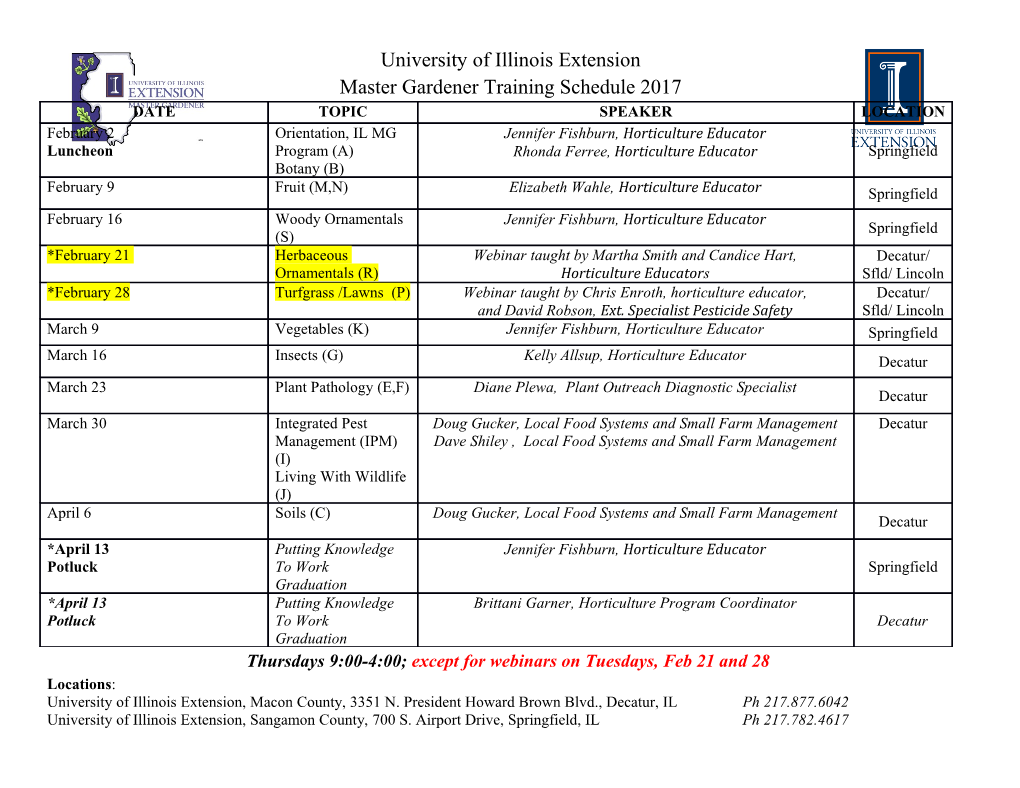
<p>Additional file 1 Feature Type and Feature Definition ICC Reference Number</p><p>SupplementaryOTHER FEATURES: Table 1. Intraclass correlation coefficients (ICC) for masked reliability assessment of computer-extracted features (n=91 pairs) Texture-based Featurefeatures: Type and Number Feature Definition ICC Reference</p><p>T3 - T8 D_BC [1-6] Fractal dimension estimated based on box-counting Range: [3, 8] SELECTED FEATURES: method; lower values correspond to coarser texture 0.895- 0.995 T9 D_M Fractal dimension estimated based on Minkowski 0.993 [3, 8] Gray level magnitude- method; lower values correspond to coarser texture based features: T10 - T17 Beta [1-8] AverageExponent gray beta value from within power ROI; law higherspectrum values analysis; Range: [9] M1 AVE correspondcharacterize to thedenser frequency region content of texture pattern; 0.9860.960- [1-3] higher values correspond to coarser texture 0.990 Gray value corresponding to the 5% region cutoff on T18 Contrast (COOC) cumulativeContrast measure density function;calculated higher from valuesco-occurrence correspond 0.998 [5-7] M2 MinCDF tomatrix; denser measure region of image local variations 0.971 [1-3]</p><p>T19 Contrast (NGTDM) RatioContrast of (95%CDF-AVE) measure calculated to (AVE-5%CDF); from Neighborhood-Gray- Related to 0.989 [1-4] skewness;tone-difference Values matrix; less than measure one correspond of image local to having M3 Balance avariations ROI that is skewed towards relatively denser values. 0.959 [1-4]</p><p>Texture-basedT20 Correlation (COOC) Measure of image linearity; larger values correspond to 0.791 [5-7] features: linear patterns Measure of image homogeneity; higher values T1T21 EnergyEntropy (COOC) correspondMeasure of to randomness being more ofhomogeneous gray level pairs 0.9800.996 [5-7][5-7]</p><p>T22 ZeroMeasure (COOC) Zero measures in co-occurrence matrix; measure of 0.982 [5-7] image homogeneity Largest number of a gray value pair in the co- T23 Skewness (COOC) occurrenceMeasure of matrix; the asymmetry measure of co-occurrenceimage homogeneity; matrix; 0.983 [5-7] T2 MaxF (COOC) higherimage values homogeneity correspond measure to being more homogeneous 0.987 [5-7] </p><p>OTHER FEATURES:T24 MeanEdgeGradient Average of edge gradient; image coarseness measure 0.997 [3, 7]</p><p>T25 MaxEdgeGradient Maximum edge gradient; image coarseness measure 0.905 [3, 7] Gray level magnitude- based features: T26 MinEdgeGradient Minimum edge gradient; image coarseness measure 0.933 [3, 7]</p><p>T27 StdDevEdgeGradient GrayStandard value deviationcorresponding of edge to gradient;the 95% regionimage cutoffcoarseness on 0.996 [3, 7] cumulativemeasure density function; higher values correspond M4 MaxCDF to denser region 0.996 [1-3] T28 Coarseness (NGTDM) Measure of image coarseness; higher values 0.953 [1-4] Graycorrespond value corresponding to coarser region to the 70% region cutoff on cumulative density function; higher values correspond MT295 70%CDFFMP toFirst denser moment region of power spectrum; spatial frequency 0.9890.972 [1-3][1-3, 7] content of parenchymal patterns</p><p>Gray value corresponding to the 30% region cutoff on cumulative density function; higher values correspond M6 30%CDF to denser region 0.982 [1-3]</p><p>Ratio of (70%CDF-AVE) to (AVE-30%CDF); Related to skewness; Values larger than one correspond to M7 Balance2 denser regions 0.842 [1-4]</p><p>The denseness measure; Negative values correspond M8 Skewness to denser region 0.981 [1-4] Rood mean square variation; quantifies the magnitude of parenchymal patterns [1-3, 7] M9 RMS 1 0.990 (cont’d) 2 Supplementary Table 2. Correlations between selected computer-extracted features (n=237 women)</p><p>Correlation with Correlation with Correlation with Correlation AVE MinCDF Balance with Energy Feature Type and Number Feature r* p-value r* p-value r* p-value r* p-value</p><p>Gray level magnitude-based features: M1 AVE 1.00 M2 MinCDF 0.60 <.0001 1.00 M3 Balance -0.77 <.0001 -0.41 <.0001 1.00</p><p>Texture-based features: T1 Energy -0.09 0.19 0.48 <.0001 -0.09 0.19 1.00 T2 MaxF (COOC) 0.03 0.69 0.43 <.0001 -0.13 0.053 0.90 <.0001</p><p>*Spearman's correlation coefficient</p><p>3 Supplementary Table 3. Sensitivity analyses of the ability of the trained classifier to distinguish between BRCA1/2 mutation carriers and non-carriers in age-matched datasets</p><p>Training dataset Testing dataset Testing dataset results</p><p>Mean paired difference in Mean probability score paired No. non- No. from trained difference Description Selected features ¥ Description carriers carriers classifier SD p-value † in PMD SD p-value † AUC SE</p><p>Age-matched testing Original training dataset* datasets PMD Alone Age-matched ~3 years 19 19 0.55 0.09 Features alone Age-matched ~3 years 19 19 0.28 0.48 0.02 -1.83 18.04 0.83 0.71 0.09 4 features selected: Features + PMD MinCDF Age-matched ~3 years 19 19 0.32 0.53 0.02 0.72 0.08 Energy PMD Alone AVE Age-matched ~1 year 17 17 0.52 0.09 Features alone Max F (COOC) Age-matched ~1 year 17 17 0.26 0.52 0.06 -0.77 19.33 1.00 0.73 0.09 Features + PMD Age-matched ~1 year 17 17 0.25 0.53 0.08 0.74 0.09</p><p>Training dataset truncated at Testing dataset truncated at upper age-limit of mutation upper age-limit of mutation 3 features selected: carriers § MinCDF carriers § Max F (COOC) Features alone Balance Age-matched ~3 years 19 19 0.18 0.39 0.055 -1.83 18.04 0.83 0.72 0.09 Features + PMD Age-matched ~3 years 19 19 0.18 0.37 0.06 0.71 0.09</p><p>* Original training set includes 70 non-carriers and 107 BRCA1/2 mutation carriers § Training dataset truncated at upper age-limit of mutation carriers (i.e., 55 years) includes 48 non-carriers and 48 BRCA1/2 mutation carriers ¥ Selected features: Percent mammographic density was not selected by the trained classifier but was forced into the models where noted † P-value for differences between age-matched pairs from Wilcoxon signed rank test AUC, area under the curve; PMD, percent mammographic density; SE, standard error</p><p>4 Supplementary Figure 1. Histogram of the number of times that each feature was selected in the 177 leave- one-case-out stepwise feature selection using linear discriminant analysis of the training dataset. Note the features M1-M9 correspond to gray level magnitude-based features, and features T1-T29 correspond to texture-based features. Two gray level magnitude-based features (i.e., M1, AVE; M2, MinCDF) and two texture-based features (i.e., T1, Energy; T2, MaxF (COOC)) were selected more than half the time (as indicated by the dashed line in the figure), and were included in subsequent Bayesian Artificial Neural Network models.</p><p>5 References</p><p>1. Huo Z, Giger ML, Wolverton DE, et al. Computerized analysis of mammographic parenchymal patterns for breast cancer risk assessment: feature selection. Med Phys 2000;27(1):4-12.</p><p>2. Li H, Giger ML, Huo Z, et al. Computerized analysis of mammographic parenchymal patterns for assessing breast cancer risk: effect of ROI size and location. Med Phys 2004;31(3):549-555.</p><p>3. Li H, Giger ML, Olopade OI, et al. Computerized Texture Analysis of Mammographic </p><p>Parenchymal Patterns of Digitized Mammograms. Academic Radiology 2005;12(7):863-873.</p><p>4. Huo Z, Giger ML, Olopade OI, et al. Computerized analysis of digitized mammograms of BRCA1 and BRCA2 gene mutation carriers. Radiology 2002;225(2):519-526.</p><p>5. Chen W, Giger ML, Li H, et al. Volumetric texture analysis of breast lesions on contrast-enhanced magnetic resonance images. Magn Reson Med 2007;58(3):562-571.</p><p>6. Haralick RM, Shanmugan K, Dinstein I. Textural Features for Image Classification. IEEE </p><p>Transactions on Systems, Man, and Cybernetics 1973;6:610-621.</p><p>7. Sonka M, Hlavac V, Boyle R. Image Processing, Analysis, and Machine Vision. Second Edition ed: PWS Publishing; 1999.</p><p>8. Li H, Giger ML, Olopade OI, et al. Fractal analysis of mammographic parenchymal patterns in breast cancer risk assessment. Acad Radiol 2007;14(5):513-521.</p><p>9. Li H, Giger ML, Olopade OI, et al. Power spectral analysis of mammographic parenchymal patterns for breast cancer risk assessment. J Digit Imaging 2008;21(2):145-152.</p><p>6</p>
Details
-
File Typepdf
-
Upload Time-
-
Content LanguagesEnglish
-
Upload UserAnonymous/Not logged-in
-
File Pages6 Page
-
File Size-