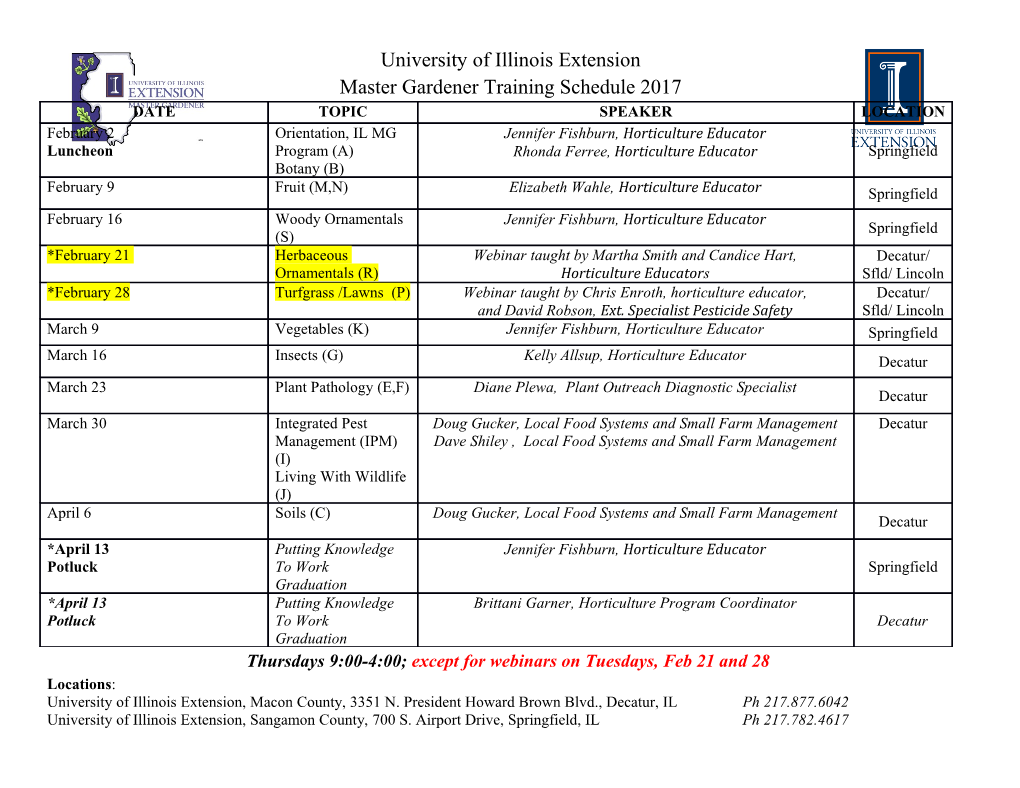
<p>Chapter 7</p><p>465 9. a. x x / n 93 i 5 b. 2 xi (xi x) (xi x) 94 +1 1 100 +7 49 85 -8 64 94 +1 1 92 -1 1 Totals 465 0 116</p><p>(x x) 2 116 s i 5.39 n 1 4</p><p>$45,500 11. a. x= S x/ n = = $4,550 i 10</p><p>S(x - x )2 9,068,620 b. s =i = = $1003.80 n -1 10 - 1</p><p>15. a. The sampling distribution is normal with</p><p>E (x ) = = 200</p><p> sx = s /n = 50 / 100 = 5</p><p>For 5, 195#x 205</p><p>Using Standard Normal Probability Table:</p><p> x - m 5 At x = 205, z = = = 1 P( z 1) = .8413 s x 5</p><p> x -m -5 At x = 195, z = = = -1 P( z < - 1) = .1587 s x 5</p><p>P(195# x 205) = .8413 - .1587 = .6826</p><p>Using Excel: =NORMDIST(205,200,5,TRUE)-NORMDIST(195,200,5,TRUE) = .6827</p><p> b. For 10, 190#x 210</p><p>Using Standard Normal Probability Table:</p><p> x 10 At x = 210, z 2 P( z 2) = .9772 x 5</p><p>7 - 1 Chapter 7</p><p> x -m -10 At x = 190, z = = = -2 P( z < - 2) = .0228 s x 5</p><p>P(190# x 210) = .9772 - .0228 = .9544</p><p>Using Excel: =NORMDIST(210,200,5,TRUE)-NORMDIST(190,200,5,TRUE) = .9545</p><p>17. a. x / n 10 / 50 1.41</p><p> b. n / N = 50 / 50,000 = .001</p><p>Use x / n 10 / 50 1.41</p><p> c. n / N = 50 / 5000 = .01</p><p>Use x / n 10 / 50 1.41</p><p> d. n / N = 50 / 500 = .10</p><p>N n 500 50 10 Use x 1.34 N 1 n 500 1 50</p><p>Note: Only case (d) where n /N = .10 requires the use of the finite population correction factor. </p><p>25. = 2.34 = .20</p><p> a. n = 30</p><p> x - m .03 z = = = .82 s /n .20 / 30</p><p>P(2.31 x 2.37) = P(-.82 z .82) = .7939 - .2061 = .5878</p><p>Using Excel: =NORMDIST(2.37,2.34,.20/SQRT(30),TRUE)- NORMDIST(2.31,2.34,.20/SQRT(30),TRUE)=.5887</p><p> b. n = 50</p><p> x - m .03 z = = = 1.06 s /n .20 / 50</p><p>P(2.31 x 2.37) = P(-1.06 z 1.06) = .8554 - .1446 = .7108</p><p>Using Excel: =NORMDIST(2.37,2.34,.20/SQRT(50),TRUE)- NORMDIST(2.31,2.34,.20/SQRT(50),TRUE)=.7112</p><p> c. n = 100</p><p> x - m .03 z = = = 1.50 s /n .20 / 100</p><p>7 - 2 Sampling and Sampling Distributions</p><p>P(2.31 x 2.37) = P(-1.50 z 1.50) = .9332 - .0668 = .8664</p><p>Using Excel: =NORMDIST(2.37,2.34,.20/SQRT(100),TRUE)- NORMDIST(2.31,2.34,.20/SQRT(100),TRUE)=.8664</p><p> d. None of the sample sizes in parts (a), (b), and (c) are large enough. At z = 1.96 we find P(-1.96 z 1.96) = .95. So, we must find the sample size corresponding to z = 1.96. Solve .03 = 1.96 .20 / n</p><p>骣.20 n =1.96琪 = 13.0667 桫.03</p><p> n = 170.73</p><p>Rounding up, we see that a sample size of 171 will be needed to ensure a probability of .95 that the sample mean will be within $.03 of the population mean.</p><p>31. a.</p><p> p(1 p ) .30(.70) .0458 p n 100</p><p> p .30</p><p>The normal distribution is appropriate because np = 100(.30) = 30 and n(1 - p) = 100(.70) = 70 are both greater than 5.</p><p> b. P (.20 p .40) = ?</p><p>.40 .30 z 2.18 P(z ≤ 2.18) = .9854 .0458</p><p>P(z < -2.18) = .0146</p><p>P(.20 ≤ p ≤ .40) = .9854 - .0146 = .9708</p><p>Using Excel:</p><p>=NORMDIST(.40,.30,.0458,TRUE)-NORMDIST(.20,.30,.0458,TRUE) = .9710</p><p> c. P (.25 p .35) = ?</p><p>7 - 3 Chapter 7</p><p>.35 .30 z 1.09 P(z ≤ 1.09) = .8621 .0458</p><p>P(z < -1.09) = .1379</p><p>P(.25 ≤ p ≤ .35) = .8621 - .1379 = .7242</p><p>Using Excel:</p><p>=NORMDIST(.35,.30,.0458,TRUE)-NORMDIST(.25,.30,.0458,TRUE) = .7250</p><p>33. a. Normal distribution</p><p>E ( p ) = .50</p><p> p(1 p ) (.50)(1 .50) .0206 p n 589</p><p> p p .04 b. z 1.94 P(z ≤ 1.94) = .9738 p .0206</p><p>P(z < -1.94) = .0262</p><p>P(.46 ≤ p ≤ .54) = .9738 - .0262 = .9476</p><p>Using Excel:</p><p>=NORMDIST(.54,.50,.0206,TRUE)-NORMDIST(.46,.50,.0206,TRUE) = .9478</p><p> p p .03 c. z 1.46 P(z ≤ 1.46) = .9279 p .0206</p><p>P(z < -1.46) = .0721</p><p>P(.47 ≤ p ≤ .53) = .9279 - .0721 = .8558</p><p>Using Excel:</p><p>=NORMDIST(.53,.50,.0206,TRUE)-NORMDIST(.47,.50,.0206,TRUE) = .8547</p><p> p p .02 d. z .97 P(z ≤ .97) = .8340 p .0206</p><p>P(z < -.97) = .1660</p><p>P(.48 ≤ p ≤ .52) = .8340 - .1660 = .6680</p><p>Using Excel:</p><p>=NORMDIST(.52,.50,.0206,TRUE)-NORMDIST(.48,.50,.0206,TRUE) = .6684</p><p>7 - 4 Sampling and Sampling Distributions</p><p>35. a. Normal distribution with E( p ) = p = .25 and </p><p> p(1 p ) .25(1 .25) .0137 p n 1000</p><p> p p .03 b. z 2.19 P(z ≤ 2.19) = .9857 p .0137</p><p>P(z < -2.19) = .0143</p><p>P(.22 p .28) = P(-2.19 z 2.19) = .9857 - .0143 = .9714</p><p>Using Excel:</p><p>=NORMDIST(.28,.25,.0137,TRUE)-NORMDIST(.22,.25,.0137,TRUE) = .9715</p><p> p p .03 z 1.55 c. .25(1 .25) .0194 P(z ≤ 1.55) = .9394 500</p><p>P(z < -1.55) = .0606</p><p>P(.22 p .28) = P(-1.55 z 1.55) = .9394 - .0606 = .8788</p><p>Using Excel:</p><p>=NORMDIST(.28,.25,.0194,TRUE)-NORMDIST(.22,.25,.0194,TRUE) = .8780</p><p>Chapter 8</p><p>x 80 13. a. x i 10 n 8</p><p> b. 2 xi (xi x ) (xi x ) 10 0 0 8 -2 4 12 2 4 15 5 25 13 3 9 11 1 1 6 -4 16 5 -5 25 84</p><p>(x x )2 84 s i 3.464 n 1 7</p><p> c. t.025 ( s / n ) 2.365(3.464 / 8) 2.9</p><p>7 - 5 Chapter 7</p><p> d. x t.025 ( s / n )</p><p>10 ± 2.9 or 7.1 to 12.9</p><p>15. x t / 2 ( s / n )</p><p>90% confidence df = 64 t.05 = 1.669</p><p>19.5 ± 1.669 (5.2 / 65)</p><p>19.5 ± 1.08 or 18.42 to 20.58</p><p>95% confidence df = 64 t.025 = 1.998</p><p>19.5 ± 1.998 (5.2 / 65)</p><p>19.5 ± 1.29 or 18.21 to 20.79</p><p>17. For the Miami data set, the output obtained using Excel’s Descriptive Statistics tool follows:</p><p>Rating</p><p>Mean 6.34 Standard Error 0.3059 Median 6.5 Mode 8 Standard Deviation 2.1629 Sample Variance 4.6780 Kurtosis -1.1806 Skewness -0.1445 Range 8 Minimum 2 Maximum 10 Sum 317 Count 50 Confidence Level(95.0%) 0.6147</p><p>The 95% confidence interval is x margin of error</p><p>6.34 0.6147 or 5.73 to 6.95</p><p>Sx 2600 21. x =i = = 130 liters of alcoholic beverages n 20</p><p>S(x - x )2 81244 s =i = = 65.39 n -1 20 - 1</p><p>7 - 6 Sampling and Sampling Distributions</p><p> t.025 = 2.093 df = 19</p><p>95% confidence interval: x t.025 (s / n)</p><p>130 2.093 (65.39 / 20) </p><p>130 30.60 or 99.40 to 160.60 liters per year</p><p>35. a. p = 281/611 = .4599 (46%)</p><p> p(1 p ) .4599(1 .4599) b. z 1.645 .0332 .05 n 611</p><p> c. p ± .0332</p><p>.4599 .0332 or .4267 to .4931</p><p> p(1 p ) (.53)(.47) 43. a. Margin of Error = z 1.96 .0253 / 2 n 1500</p><p>95% Confidence Interval: .53 .0253 or .5047 to .5553</p><p>(.31)(.69) b. Margin of Error = 1.96 = .0234 1500</p><p>95% Confidence Interval: .31 .0234 or .2866 to .3334</p><p>(.05)(.95) c. Margin of Error = 1.96 = .0110 1500</p><p>95% Confidence Interval: .05 .0110 or .039 to .061</p><p> d. The margin of error decreases as p gets smaller. If the margin of error for all of the interval estimates must be less than a given value (say .03), an estimate of the largest proportion should be used as a planning value. Using p* .50 as a planning value guarantees that the margin of error for all the interval estimates will be small enough.</p><p>Chapter 9</p><p>1. a. H0: 600 Manager’s claim.</p><p>Ha: > 600</p><p> b. We are not able to conclude that the manager’s claim is wrong.</p><p> c. The manager’s claim can be rejected. We can conclude that > 600.</p><p>3. a. H0: = 32 Specified filling weight</p><p>Ha: 32 Overfilling or underfilling exists</p><p>7 - 7 Chapter 7</p><p> b. There is no evidence that the production line is not operating properly. Allow the production process to continue.</p><p> c. Conclude 32 and that overfilling or underfilling exists. Shut down and adjust the production line.</p><p>5. a. The Type I error is rejecting H0 when it is true. This error occurs if the researcher concludes that young men in Germany spend more than 56.2 minutes per day watching prime-time TV when the national average for Germans is not greater than 56.2 minutes. </p><p> b. The Type II error is accepting H0 when it is false. This error occurs if the researcher concludes that the national average for German young men is 56.2 minutes when in fact it is greater than 56.2 minutes.</p><p> x 14 12 23. a. t 0 2.31 s/ n 4.32 / 25</p><p> b. Degrees of freedom = n – 1 = 24</p><p>Upper tail p-value is the area to the right of the test statistic</p><p>Using t table: p-value is between .01 and .025</p><p>Using Excel: p-value = TDIST(2.31,24,1) = .0149</p><p> c. p-value .05, reject H0.</p><p> c. With df = 24, t.05 = 1.711</p><p>Reject H0 if t 1.711</p><p>2.31 > 1.711, reject H0.</p><p> x 44 45 25. a. t 0 1.15 s/ n 5.2 / 36</p><p>Degrees of freedom = n – 1 = 35</p><p>Lower tail p-value is the area to the left of the test statistic</p><p>Using t table: p-value is between .10 and .20</p><p>Using Excel: p-value = TDIST(1.15,35,1) = .1290</p><p> p-value > .01, do not reject H0</p><p> x 43 45 b. t 0 2.61 s/ n 4.6 / 36</p><p>Lower tail p-value is the area to the left of the test statistic</p><p>7 - 8 Sampling and Sampling Distributions</p><p>Using t table: p-value is between .005 and .01</p><p>Using Excel: p-value = TDIST(2.61,35,1) = .0066</p><p> p-value .01, reject H0</p><p> x 46 45 c. t 0 1.20 s/ n 5/ 36</p><p>Lower tail p-value is the area to the left of the test statistic</p><p>Using t table: p-value is between .80 and .90</p><p>Using Excel: p-value = TDIST(1.20,35,1) = .8809</p><p> p-value > .01, do not reject H0</p><p>31. H0: 47.50</p><p>Ha: > 47.50</p><p> x 51 47.50 t 0 2.33 s/ n 12 / 64</p><p>Degrees of freedom = n - 1 = 63</p><p>Upper tail p-value is the area to the right of the test statistic</p><p>Using t table: p-value is between .01 and .025</p><p>Using Excel: p-value = TDIST(2.33,91,1) = .0110</p><p>Reject H0; Atlanta customers are paying a higher mean water bill. </p><p>37. a. H0: p .125</p><p>Ha: p > .125</p><p>52 b. p = = .13 400</p><p> p- p .13- .125 z =0 = = .30 p0(1- p 0 ) .125(1 - .125) n 400</p><p>Upper tail p-value is the area to the right of the test statistic</p><p>Using normal table with z = .30: p-value = 1.0000 - .6179 = .3821</p><p>Using Excel: p-value = 1-NORMSDIST(.30) = .3821</p><p>7 - 9 Chapter 7</p><p> c. p-value > .05; do not reject H0. We cannot conclude that there has been an increase in union membership.</p><p>43. a. H0: p ≤ .10</p><p>Ha: p > .10</p><p> b. There are 13 “Yes” responses in the Eagle data set.</p><p>13 p = = .13 100</p><p> p- p .13- .10 z =0 = = 1.00 c. p0(1- p 0 ) .10(1 - .10) n 100</p><p>Upper tail p-value is the area to the right of the test statistic</p><p>Using normal table with z = 1.00: p-value = 1 - .8413 = .1587</p><p>Using Excel: p-value = 1-NORMSDIST(1) = .1587</p><p> p-value > .05; do not reject H0.</p><p>The statistical results do not allow us to conclude that p > .10. But, given that p = .13, management may want to authorize a larger study before deciding not to go national.</p><p>Chapter 10</p><p>54 42 11. a. x 9 x 7 1 6 2 6</p><p>2 (xi x1 ) b. s1 2.28 n1 1</p><p>2 (xi x2 ) s2 1.79 n2 1</p><p> c. x1 x 2 = 9 - 7 = 2</p><p>2 2 s2 s 2 2.282 1.79 2 1 2 n1 n 2 6 6 d. df 2 2 2 2 9.5 1s2 1 s 2 1 2.28 2 1 1.79 2 1 2 n11 n 1 n 2 1 n 2 5 6 5 6 </p><p>Use df = 9, t.05 = 1.833</p><p>2.282 1.79 2 x x 1.833 1 2 6 6</p><p>7 - 10 Sampling and Sampling Distributions</p><p>2 2.17 (-.17 to 4.17)</p><p>xi 111.6 13. a. x1 9.3 n1 12</p><p>2 (xi x1 ) 71.12 s1 2.54 n1 1 12 1</p><p>xi 42 x2 4.2 n2 10</p><p>2 (xi x2 ) 18.4 s2 1.43 n2 10.1</p><p> b. x1 x 2 = 9.3 - 4.2 = 5.1 tons</p><p>Memphis is the higher volume airport and handled an average of 5.1 tons per day more than Louisville. Memphis handles more than twice the volume of Louisville.</p><p>2 2 s2 s 2 2.542 1.43 2 1 2 n1 n 2 12 10 c. df 2 2 2 2 17.8 1s2 1 s 2 1 2.54 2 1 1.43 2 1 2 n11 n 1 n 2 1 n 2 11 12 9 10 </p><p>Use df = 17, t.025 = 2.110</p><p>2 2 s1 s 2 (x1 x 2 ) t .025 n1 n 2</p><p>2.542 1.43 2 5.1 2.110 12 10</p><p>5.1 1.82 (3.28 to 6.92)</p><p>15. 1 for 2001 season</p><p>2 for 1992 season</p><p>H0: 1 2 0</p><p>Ha: 1 2 0</p><p> b. x1 x 2 = 60 - 51 = 9 days</p><p>9/51(100) = 17.6% increase in number of days.</p><p>7 - 11 Chapter 7</p><p> x1 x 2 0 (60 51) 0 t 2.48 c. s2 s 218 2 15 2 1 2 n1 n 2 45 38</p><p>2 2 s2 s 2 182 15 2 1 2 n1 n 2 45 38 df 2 2 2 2 81 1s2 1 s 2 1 18 2 1 15 2 1 2 n11 n 1 n 2 1 n 2 44 45 37 38 </p><p>Using t table, p-value is between .005 and .01.</p><p>Exact p-value corresponding to t = 2.48 is .0076</p><p> p-value .01, reject H0. There is a greater mean number of days on the disabled list in 2001. </p><p> d. Management should be concerned. Players on the disabled list have increased 32% and time on the list has increased by 17.6%. Both the increase in inquiries to players and the cost of lost playing time need to be addressed.</p><p>31. a. p1 = 150/250 = .46 Republicans</p><p> p2 = 98/350 = .28 Democrats</p><p> b. p1 p 2 = .46 - .28 = .18</p><p>Republicans have a .18, 18%, higher participation rate than Democrats.</p><p> p1(1 p 2 ) p 2 (1 p 2 ) c. z.025 n1 n 2</p><p>.46(1 .46) .28(1 .28) 1.96 .0777 250 350</p><p> d. Yes, .18 .0777 (.1023 to .2577)</p><p>Republicans have a 10% to 26% higher participation rate in online surveys than Democrats. Biased survey results of online political surveys are very likely.</p><p>33. a. p1 = 256/320 = .80</p><p> b. p2 = 165/250 = .66</p><p> c. p1 p 2 = .80 - .66 = .14</p><p> p1(1 p 1 ) p 2 (1 p 2 ) .14 z.025 n1 n 2</p><p>7 - 12 Sampling and Sampling Distributions</p><p>.80(1 .80) .66(1 .66) .14 1.96 320 250</p><p>.14 .0733 (.0667 to .2133)</p><p>35. a. H0: p1 - p2 = 0</p><p>Ha: p1 - p2 0</p><p> p1 = 63/150 = .42</p><p> p2 = 60/200 = .30</p><p> n p n p 63 60 p 1 1 2 2 .3514 n1 n 2 150 200</p><p> p p .42 .30 z 1 2 2.33 1 1 1 1 p1 p .3514 1 .3514 150 200 n1 n 2 </p><p> p-value = 2(1.0000 - .9901) = .0198</p><p> p-value .05, reject H0. There is a difference between the recall rates for the two commercials.</p><p> p1(1 p 1 ) p 2 (1 p 2 ) b. p1 p 2 z .025 n1 n 2</p><p>.42(1 .42) .30(1 .30) .42 .30 1.96 150 200</p><p>.12 .1014 (.0186 to .2214)</p><p>Commercial A has the better recall rate.</p><p>7 - 13</p>
Details
-
File Typepdf
-
Upload Time-
-
Content LanguagesEnglish
-
Upload UserAnonymous/Not logged-in
-
File Pages13 Page
-
File Size-