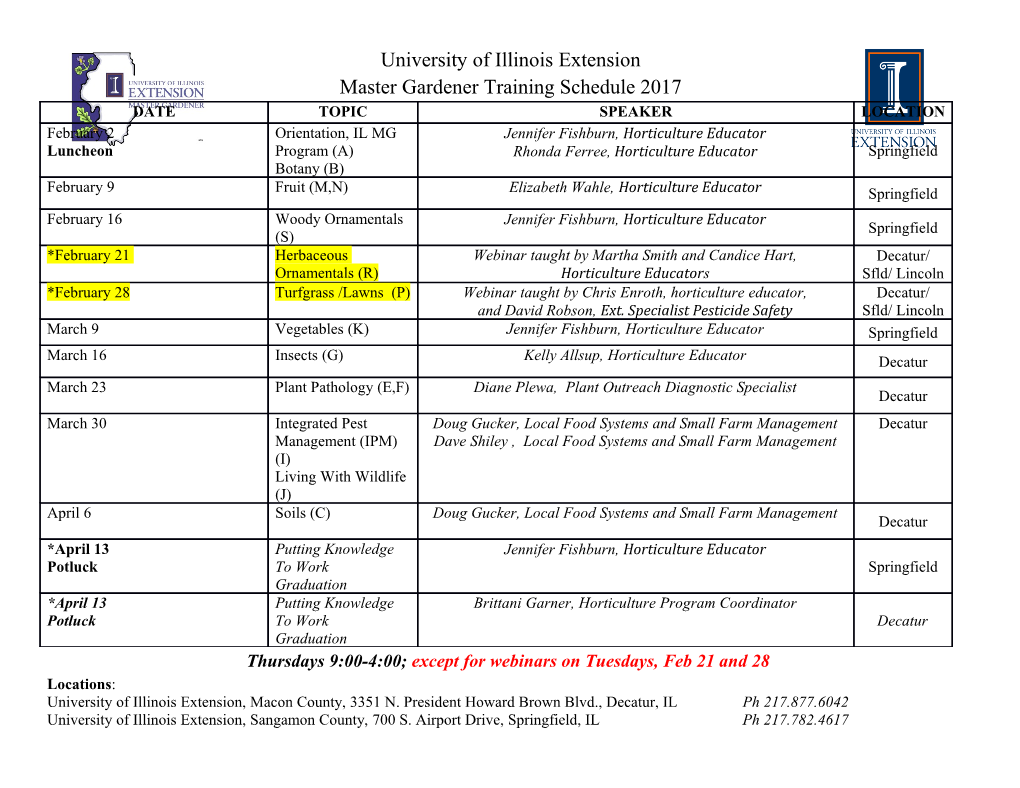
<p>Team#13767 Page 1 of 13 List</p><p>1, Introduction ------2 2, Assumption ------2 3, Analysis and solution of the model ------2 3 .1Background ------2 3 .2Establishment of model ------4</p><p>3.2.1Distribution of campsites ------5 3.2.2Cluster Analysis and Analog Simulation ------6 3 .2.3Number of contacts ------8 3 .3The establishment of comprehensive evaluation index ------9 4, Analysis of the results ------11 5, Model evaluation ------17</p><p>Team#13767 Page 2 of 13</p><p>1. Introduction</p><p>A 225-mile-river trip require camping which range from 6 to 18 nights. Passengers take either oar- powered rubber rafts, which travel on average 4 mph or motorized boats, which travel on average 8 mph. O riginally, we presume that X trips travel down the river each year during a six month period and Y camp sites on the river, distributed fairly uniformly throughout the river corridor. Differently, we need to let more trips in now, as a result, to schedule an optimal mix of trips so as to utilize the campsites in the best way possible with minimal contact with other groups of boats on the river, we should determine the schedule fairly, of varying duration and propulsion. And, no two sets of campers can occupy the same site at the same time. I n our model, we determine that · How many more boat trips could be added to the Big Long River’s rafting season. · An optimal timetable of boat trips in the season. · A piece of suggestion of determining the carrying capacity of the river.</p><p>2. Assumption</p><p>1. During the trip, groups which continue their trip leave their campsite at the same time. 2. Every boat will pull in as schedule. 3. Managers of the sites can make prediction about number of visitors next year. 4. Every camp site can be occupied by only one group. 5. In every camp site, visitors can choose the drifting way: oar or motor. 6. At night, visitors must stay at sites, and cannot to proceed. 7. Visitors have decided how many days they will enjoy before they start their trips. 8. Influence of weather is omitted. 9. When pull-in at one sites, visitor can choose a 2-day-stay to enjoy the scenery or proceed.</p><p>3. Analysis and solution of the model</p><p>3.1Background</p><p>Team#13767 Page 3 of 13 Through gathering historical data of visiting situation in 2011 and 2012, we make a table to show intuitively the visiting situations. number of 6 7 8 9 10 11 12 visiting days number of visiting groups 2 15 10 13 1 0 0 in2011 number of visiting groups 4 18 5 19 0 0 0 in2012 number of 13 14 15 16 17 18 visiting days number of visiting groups 4 4 13 5 1 1 in2011 number of visiting groups 2 4 17 4 0 0 in2012 Table.1 historical data</p><p>Fig.1 historical probability F ig.1 indicates the result that the average of 2011and 2012 probability through normalization. The vertical coordinate is weight p, and the sum of all weights is 1. We get the results. p = 0 .0419 0.2320 0.1067 0.2243 0.0072 0 0 0 .0427 0.0564 0.2106 0.0636 0.0072 0.0072 So we classify them into Group Number of days Percentage A group 6,7,8 0.3806 B group 9,10,11,12,13 0.3306 C group 14,15,16,17,18 0.2886 Table.2 classify of three groups The advantage is</p><p>1. Numbers of visitors in every group is nearly close. 2. In each group, number of visiting days is close to each other, it’s convenient to arrange the schedule. 3.2Establishment of model</p><p>Team#13767 Page 4 of 13</p><p>Fig. 2 Flow sheet</p><p>3.2.1Distribution of campsites T he purpose is distributing Y campsites to 3 visiting groups: A, B, C. Because number of visitors in these groups is nearly close, so we should do our best to guarantee cycles of boats is nearly close too. The cycle of boats depends on visiting time and shared number of campsites. For instance, the longest visiting days for A is 8 days, if the shared number of campsites is 5, the pull-in period in these campsites is 1, 1, 2, 2, 2. As a result, the cycle of boats for A group is 2 days. In this process, it is easy to find out that groups whose number of visiting days is bigger in principle of closer cycle of boats, it should have more campsites. If number of campsites for A, B, C is a, b, c, then the less difference between 8/a 13/b 18/c is, the better this plan is. After programming, we get the concrete Y, corresponded a b c and cycle of boats for each group. I n this way, we get the timetable of boats. For instance, Y = 20, we get the timetables and camp sites of 2 groups.</p><p>A B C Number of camp 4 7 9 sites Cycle of boat trips 2 2 2 Table.3 distribute of campsites</p><p>Day 1 2 3 4t 5 6 7 8 9 10 11 s A 1 1 1 1 1 1 B 1 1 1 1 1 1 C 1 1 1 1 1 1 Table.4 time table</p><p>S o we figure out that the biggest times of cycling ‘A’ group in 6 months, in other words, the maximum capacity of visitors in A group. Based on the demand proportion of visitors on 6-8 days’ visiting, we can calculate the maximum capacity on 6-8 days’ visiting. In the same way, we can get data of other groups. If the demand proportion of one way is too low, we can omit it, like the 10,11,12,17 and 18.</p><p>Visiting 6 7 8 9 10 11 12 13 14 15 16 17 18 days the 18 54 18 67 0 0 0 23 18 54 18 0 0 maximum</p><p>Team#13767 Page 5 of 13 capacity Table.5 maximum capacity</p><p>F rom the analysis above, we need to give a concrete plan of distribution. Considering the distance of travel every day is limited, we should make sure that distance everyday is certain. In case of A group, we assume the total length of this trip is 1 and A group get a campsite, so the average journey is , so the locations of campsites are known. 1/(1 +a) I n the same way, we get B and C groups’ location. W e sort the campsites and distribute Y campsites to those groups. For instance, we assume A B C group get 3 4 5 campsites, the locations of A group are {0.25, 0.5, 0.75}, of B group are {0.2, 0.4, 0.6, 0.8}, of C group are {0.167, 0.334, 0.501, 0.668, 0.835}, we get the locations. Conversely, if we make sure there are 12 campsites in all, we will know their corresponded groups. In this way, we finish the distribution of campsites.</p><p>3.2.2Cluster Analysis and Analog Simulation Cluster Analysis O ne important way for human to know the world is classify. Original classify depended on accumulated experience and related knowledge, with the rapid development of technology, demand for classify becomes higher, that’s why Numerical classification emerges. Cluster analysis is an important part in Numerical classification. A ccording to Fig.1, we classify the data in probability using Cluster analysis. F irstly, we put data through regularization by</p><p>A fter the variation, we calculate the distance coefficient matrix by</p><p>I n the process of cluster analysis, we choose elements that have minimal distance coefficient, classify their corresponded numbers of days. It’s obvious that there are 3 groups: {6, 14}, {10, 17, 18,}, {11, 12}. N ext, we calculate the weighted average of elements in a same group to get a new Regularized numerical table.</p><p>Team#13767 Page 6 of 13 Number 6,14 7 8 9 10,17, 11, 13 15 16 of 18 12 visiting days 2011 0.2667 1 0.6667 0.8667 0.0667 0 0.2667 0.8667 0.3333</p><p>2012 0.2105 0.9474 0.2632 1 0 0 0.1053 0.8947 0.2105</p><p>Table.6 regularized numerical table</p><p>According to distance coefficient, we can get the distance coefficient matrix.</p><p>Days 6 7 8 9 10 11 13 15 16 6 0 0.3465 0.1345 0.3305 0.0968 0.1133 0.0351 0.3033 0.0222 7 0.3465 0 0.2537 0.0478 0.4433 0.4592 0.3722 0.0478 0.3312 8 0.1345 0.2537 0 0.2545 0.2184 0.2389 0.1433 0.2208 0.1125 9 0.3305 0.0478 0.2545 0 0.4269 0.4411 0.3591 0.0351 0.3176 10 0.0968 0.4433 0.2184 0.4269 0 0.0222 0.0753 0.4001 0.1133 11 0.1133 0.4592 0.2389 0.4411 0.0222 0 0.0956 0.4152 0.1314 13 0.0351 0.3722 0.1433 0.3591 0.0753 0.0956 0 0.3305 0.0415 15 0.3033 0.0478 0.2208 0.0351 0.4001 0.4152 0.3305 0 0.2892 16 0.0222 0.3312 0.1125 0.3176 0.1133 0.1314 0.0415 0.2892 0 Table.7 distance coefficient matrix</p><p>W e choose the minimum to proceed cluster analysis and get the result that there turns up 2 groups: {6,14,16} {10,11,12,17,18}. After we repeat the procedures the above, we get</p><p>Numbe 6,14,16 7 8 9 10,11,12, 13 15 r of 17,18 visiting days 2011 0.2889 1 0.6667 0.8667 0.0400 0.2667 0.8667</p><p>2012 0.2105 0.9474 0.2632 1 0 0.1053 0.8947</p><p>Table.8 regularized numerical table</p><p>And Days 6 7 8 9 10 13 15 6 0 0.3870 0.1442 0.3698 0.1232 0.0407 0.3385 7 0.3870 0 0.2877 0.0542 0.5098 0.4221 0.0542</p><p>Team#13767 Page 7 of 13 8 0.1442 0.2877 0 0.2886 0.2569 0.1625 0.2504 9 0.3698 0.0542 0.2886 0 0.4904 0.4072 0.0398 10 0.1232 0.5098 0.2569 0.4904 0 0.0945 0.4604 13 0.0407 0.4221 0.1625 0.4072 0.0945 0 0.3748 15 0.3385 0.0542 0.2504 0.0398 0.4604 0.3748 0 Table.9 distance coefficient matrix</p><p>W e choose the minimum to proceed cluster analysis and get the result that there turns up 3 groups: {6,13, 14,16} {10,11,12,17,18} {9, 15}. After we repeat the procedures the above several times, we get</p><p>Days 6,8,13,14,16 7,9,15 10,11,12,17,18 2011 0.4500 0.9111 0.0400 2012 0.2500 0.9474 0 Table.10 regularized numerical table I n this way, we distribute visiting days into 3 groups and define them from the perspective of visitors’ number as hot line, usual line, unpopular line.</p><p>Analog simulation O ur model can simulate the condition of visiting using Method of Analog Simulation to guide the construct of plan and test of our model. A ccording to classify of Cluster Analysis, we can classify them as hot line (h), usual line (n), unpopular line (c). We use similar historical data to display the probability of 3 lines after Normalization.</p><p>P h= 0.2223; P n= 0.0623; P c= 0.0043;</p><p>W e assume that there come N groups’ visitors in 6 months, and display N random numbers in section (0, 1). We can determine that the random numbers are visiting days.</p><p>Days 6 7 8 9 10 11 12</p><p>Corresponde 0.0623 0.2846 0.3469 0.5692 0.5735 0.5779 0.5822 d value</p><p>Team#13767 Page 8 of 13 Days 13 14 15 16 17 18</p><p>Corresponde 0.6445 0.7067 0.9291 0.9914 0.9957 1.0000 d value Table.11 Corresponded value table</p><p>F or instance, if the random value is 0.5000, in the section (0.3469, 0.5692), it means the visitor choose a 9-day-visit. For N random numbers that can give statistics about 6-18 days’ visiting, we can proceed to establish the model in next step.</p><p>3.2.3Number of contacts C onditions in day time are complex and random, so we depend on pull-in conditions to judge number of contacts. To determine the number, we assume u and v group enjoy their trip at the same day, if u’s start campsite is in the lower reaches of the river than v’s, but the condition in pull-in camp site is opposite, it means u and v have a contact. To simplify the calculation process, we assume that 1. All boat trips in A group choose 7-day-trip. 2. All boat trips in B group choose 9-day-trip. 3. All boat trips in C group choose 15-day-trip. I n A group, the proportion of 6, 7 and 8 days’ trip is 1:1:3, it means 7-day-trip is the mean visiting type. In the same way, 9-day-trip is the mean visiting type in B group; 15-day-trip is the mean visiting type in C group. So we can guarantee the rationality of our plan. T hen we calculate number of contact according to the starting timetable. For fixed Y, we can get the camp sites in each group by the principal the above. Through analyzing the rationality of pull-in conditions, we get the rational schedule. Based on the schedule, we can list the sequence of camp sites in any groups. In the difference between two lists of sequence, if any adjacent elements’ sign is different, then they have a contact. F or instance, we assume there are 25 camp sites, A group has {1 3 5 8 12 16 21}, B has {2 4 9 13 15 17 18 20 24}, and the 7-day-trip and 9-day-trip start at the same time, the theoretical sequence is Times(day) 1 2 3 4 5 6 7 8 9 10</p><p>A group 1 3 5 8 12 16 21 26 26 26</p><p>B group 2 4 9 13 15 17 18 20 24 26</p><p>Fig.13</p><p>The number 26 means it has reached the end. </p><p>Fig.3 time--campsites </p><p>Team#13767 Page 9 of 13 F ig.3 shows the campsites the 2 groups used. The difference sequence is [-1, -1, -4, -5, -3, -1, 3, 6, 2, 0], it means they have a contact. O n the other hand, we can see the same result in the fig.3. I f they don’t start at the same time, we can fill in an 0 between the second sequence, meaning it is at the starting point. T o sum up, the number of contact is the number of adjacent elements’ sign becoming different. Furthermore, we can count the number of all contacts in our plan.</p><p>3.3The establishment of comprehensive evaluation index In our model, we use the Analytic hierarchy process to establish the comprehensive evaluation index. Symbol description · Target level--A level; criterion level--B level; plan level is C level · Average utilization rate of camps—R · Number of open days—n · Number of camps—Y · Number of visiting days in different way—k · Number of k-day-visiting teams— · Number of accepted teams—X · Number of boats’ contact—M To schedule an optimal mix of trips so as to utilize the campsites in the best way possible (A), we take account of three indexes below. · The average utilization rate of camps (B1) · The number of visitors (B2) · Encounter times of motors or oars (B3) E stablish hierarchical structure model:</p><p>Fig.4 hierarchical structure model</p><p>Team#13767 Page 10 of 13 Explanation of B level T o utilize the campsites in the best way possible, each camp must be occupied every night. </p><p>(1)The higher R is, the more reasonable C is. With this river trip becomes more and more popular, the park managers have been asked to allow more trips to travel down the river.</p><p>(2)The higher X is, the more reasonable C is. The government agency responsible for managing this river wants every trip to enjoy a wilderness experience, with minimal contact with other groups of boats on the river.</p><p>(3)The lower M is, the more reasonable C is.</p><p>Structure of pair comparison matrix U sing paired comparison method and1-9comprison of scale to construct B level’s 3*3 pair comparison matrix. Compare these 3 indexes, confirming their importance using relative scale. For the indexes, we arrange the judgment matrix A-B, the effect can be indicated as </p><p>A B1 B2 B3 B1 1 1/3 5 B2 3 1 7 B3 1/5 1/7 1 So, the matrix is</p><p>, we use the consistency test, defining consistency index CI, consistency ratio CR.</p><p>We get that X’s biggest characteristic root is b=3.0649, the corresponding feature vector is . After the normalization, we get .</p><p>So CI = 0.0325, RI = 0.58, CR = 0.0559<0.1, w can be the weight vector, which means regarding 3 aspects of weight, B2>B1>B3. In conclusion, for R, X, M, considering M is a negative index, so the compressive evaluation index can be represented as</p><p>Team#13767 Page 11 of 13</p><p>The higher g is, the more reasonable this plan is.</p><p>4, Analysis of the results For different estimated visitor groups, we give our plan using our model. For determined pre-estimated number of group, with Y becomes higher, X becomes higher too, but the span is bigger than Y’s. M rises as R falls. The variation of comprehensive evaluation index g is complex. But in fixed range, it has obvious maximum value and minimum value. According to actual situation, it’s easy to pick up the best Y to make g value highest.</p><p> r = 200 Y 15 18 20 22 25 30</p><p>X 177 195 197 197 197 197</p><p>R 69.63 64.14 58.44 53.13 46.76 38.96</p><p>M 889 441 621 799 797 2644</p><p>G 70.3984 112.7617 99.5276 85.2479 83.6144 -51.3611</p><p>Table.14 results</p><p>Fig.4 results</p><p>Fig.5 results</p><p>Fig.6 results</p><p> r = 300</p><p>Y 15 17 18 20 22 25 30 35</p><p>X 180 237 267 267 267 267 267 283</p><p>R 71.11 83.73 88.27 79.44 72.22 63.56 52.96 48.98</p><p>M 889 441 441 621 799 797 2644 2288</p><p>G 72.758 145.4895 166.2291 150.8236 136.011 133.7386 -2.0181 32.8535 6 Table.15 results</p><p>Team#13767 Page 12 of 13</p><p>Fig.7 results</p><p>Fig.8 results</p><p>Fig.9 results r=400</p><p>Y 15 18 20 25 30 34 35 37 40</p><p>X 180 270 270 270 270 270 286 395 395</p><p>R 71.11 88.82 79.94 63.96 53.3 47.03 65.60 63.58 58.81</p><p>M 889 441 621 797 2644 2652 2288 2654 2654</p><p>G 72.76 168.3 152.9 135.8 0.024 -2.300 104.3 83.31 81.98 3 1 0 1 5 Table.16 results</p><p>Fig.10 results</p><p>Fig.11 results</p><p>Fig.12 results</p><p>5, Model evaluation Fixed drifting route make mangers arrange as many camp sites as possible and convenient to manage. Besides, visitors are distributed into 3 groups: A B C, and camp sites are fixed, this arrangement can reduce conflicts and number of contacts. Furthermore, analytical Hierarchy Process gives us a chance to take account of many conditions integrally. In this way, we can determine the best number of camp sites in the end.</p><p>But we have disadvantages, like visitors’ freedom is omitted, time in each sites is limited. On the other hand, the best number of camp sites next year needs accurate prediction number.</p><p>Team#13767 Page 13 of 13 Anyway, we have more advices, for instance, if some sites are popular, managers can add more camp in the neighborhood. It can satisfy the demand and increase average utilization rate of camps.</p><p>Reference [1] Fang Peng, Ruiyan Yang, Haijun Xiao, Mingshui: Methods in Mathematical Modeling, p.90 [2] http://canyonx.com/index.php [3] Qiyuan Jiang, Jinxing Xie, Jun Ye : Mathematical Modeling, Tongji University, p.224 [4] http://wenku.baidu.com/view/ce31e26925c52cc58bd6be49.html</p>
Details
-
File Typepdf
-
Upload Time-
-
Content LanguagesEnglish
-
Upload UserAnonymous/Not logged-in
-
File Pages13 Page
-
File Size-