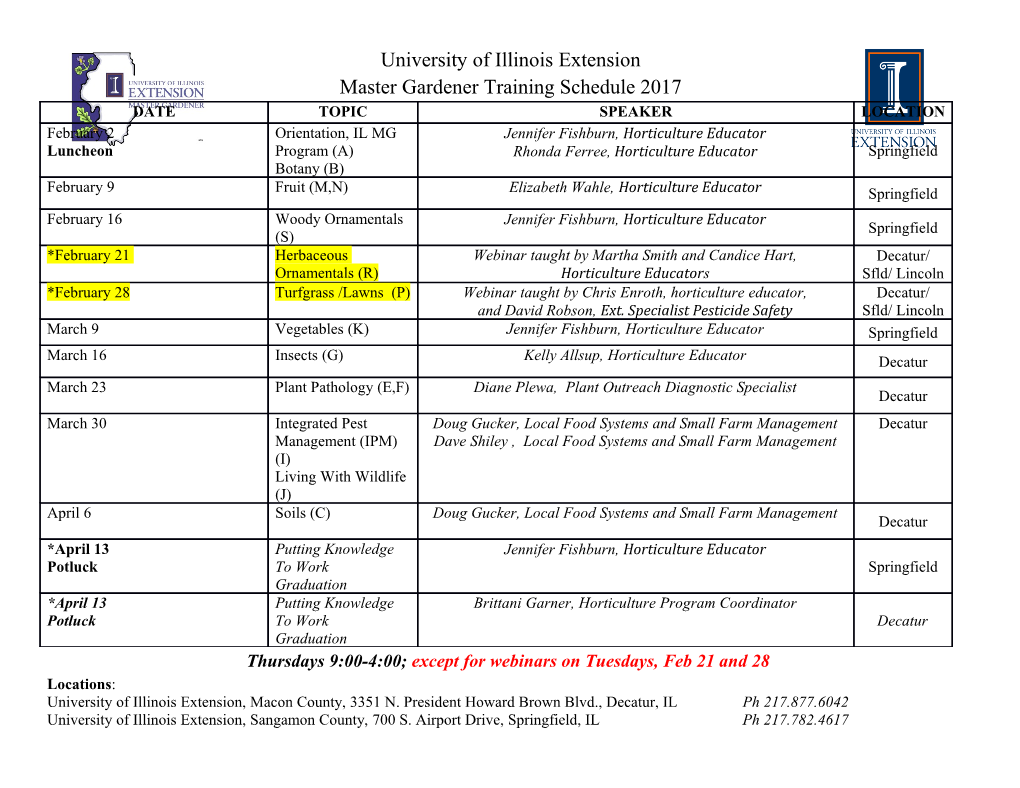
<p>Department of Statistics, Yale University STAT242b Theory of Statistics</p><p>Suggested Solutions to Homework 4</p><p>Compiled by Marco Pistagnesi</p><p>Problem 9.3c For this problem, I will use the delta method, which is faster and easier. 2 X1, , X n iid ~N (m , s ) . Following the book notation (and results), we have; 2 0 1 1 1 2 g( , ) 1.64 J n I n ; we then notice g ; n 0 1 . 6 4 2 </p><p>ˆ 2 0 1 1 1 ˆ 2 ˆ ˆ T ˆ ˆ 2 ˆ 2 2 s e ( ) ( g ) J n ( g ) 1 1 . 6 4 ˆ ( 1 . 6 4 ) n 0 1 . 6 4 n 2 2 2 where ö2 n1 X X i I do not report the numeric calculations.</p><p>Problem 9.4 ˆ We take Y= max{ X1 , , X n} and we know that Y = qMLE . Consider: P{Y-q > e} = P{ q - Y > e} = P { Y < q - e} (1) We consider for any fixed c that n P{Y< c} = P{max{ X1 , , Xn} < c} = P{ X 1 < c}创 ⋯ P { X n < c} = ( c q ) (2) Thus we use (2) to evaluate (1): n P {Y <q - e} =(( q - e) q ) We take the limit as n goes large and thus show that the MLE is consistent: n limY -q < e = lim( q - e) q = 0 . nP { } n ( ) Problem 9.6a) P N ,1 0 P N 0,1 1 . We know the MLE is q = X . Thus by the equivariance of the MLE we have ö ö X . b) given that ö ö , we can apply delta method to evaluate its st. error: ö ö ö ö se(ö) se() () se() , where (.) is the standard normal density. Since</p><p>ˆ 1 1 ˆ 1 X 1 , K , X n : N ( 0 , 1 ) I 1 ( ) 1 , hence s e ( ) s e ( ) ( X ) . n I 1 ( ) n n Thus the approximate 95% confidence interval is (ö 1.96 * se(ö)) . c) By the weak law of large numbers, is consistence. d) Because Y 1 , K , Y n : B e r ( p ) , p ( ) , we can apply the CLT to state : 1 ö ö % ()1 () Y : approxN , , hence: sµe ö . n n s e ( ˆ ) ( X ) 1 In part b) we computed n . (ö) Hence AREö,% . (Plot omitted) (ö) 1 (ö) e) From before, we have ö X . Hence, by the WLLN and its property oif preservation under continuous transformations, we have:</p><p>. X p ( X ) ˆ p ( )</p><p>But if the data are not normal, ( ) p ( X i 0 ) .</p><p>Hence, ˆ p .</p><p>Problem 5 a) The MLE of p for a binomial distribution is pˆ MLE = X n .From that we get: ö pö pö X n X n . MLE 1 MLE 2 MLE 1 1 2 2 b) We write the likelihood function: n- x n - x L p, p f x , p f x , p px1 1 p1 1 p x 2 1 p 2 2 ( 1 2) =( 1( 1 1))( 1( 1 1)) =( 1( - 1) )( 2( - 2 ) ) We take the log-likelihood function:</p><p> lnL ( p1 , p 2) = x 1 ln p 1 +( n 1 - x 1) ln( 1 - p 1) + x 2 ln p 2 +( n 2 - x 2) ln( 1 - p 2 ) . From this we can compute:</p><p> xi ni xi lnL p1, p2 , i=1,2 p p 1 p i i i 2 2 We now take the second derivatives. Note: mixed derivatives 抖 p1 p 2 and 抖 p2 p 1 are zero. We have: 2 xi ni xi 2 lnL p1, p2 2 2 , for i=1,2 pi pi 1 p i next step: 骣抖2lnLn p n- n p n 骣 2 ln L n -E琪 =1 1 + 1 1 1 = 1 and also - E 琪 = 2 p2 p 22 p1 p p 2 p 1 p 桫�1 1(1- p1 ) 1( � 1) 桫 2 2( 2 ) Thus the Fisher information matrix is:</p><p>轾n1 p 1(1- p 1 ) 0 I( p1, p 2 ) = 犏 臌 0n2 p 2( 1- p 2 ) c) We ultimately want to use the formula: T ˆ se(yˆ ) = (蜒g) Jn ( g)</p><p>To find the standard error we notice that y =g( p1, p 2) = p 1 - p 2 . We take the gradient:</p><p>轾抖g p 轾�( p p) p 轾1 T �g=1 =1 2 1 垩 ( = g) - [ 1 1] 犏抖 犏 犏 臌g p2 臌�( p1 p 2) p 2 臌-1</p><p>We also know that Jn is the inverse of the fisher information matrix (7) 2-1 2 轾n1 p 1(1- p 1) 0 轾 p 1( 1 - p 1) n 1 0 Jn =犏2 = 犏 2 臌0n2 p 2( 1- p 2) 臌 0 p 2( 1 - p 2) n 2 ˆ Taking Jn instead of Jn means we insert the estimators of the parameters instead of the real values. Thus, 轾pˆ(1- p ˆ ) n 0 轾1 se(yˆ ) =[ 1 - 1] 1 1 1 = 犏 ˆ ˆ 犏 臌 0p2( 1- p 2) n 2 臌-1 pˆ(1-p ˆ) p ˆ( 1 - p ˆ ) se(yˆ ) =1 1 + 2 2 n1 n 2 d) from before we have: ö X n X n 160 148 200 = 1.54 1 1 2 2 </p><p> pˆ1(1-p ˆ 1) p ˆ 2( 1 - pˆ 2 ) 200 160骣 160 148 骣 148 se(yˆ ) = + =琪 1 - + 琪 1 - = 0.04197 n1 n 2 200 200桫 200 200 桫 200 It is thus simple to find a 90-percent confidence interval: 1.54 1.25 0.04197 1.487,1.593 . </p>
Details
-
File Typepdf
-
Upload Time-
-
Content LanguagesEnglish
-
Upload UserAnonymous/Not logged-in
-
File Pages3 Page
-
File Size-