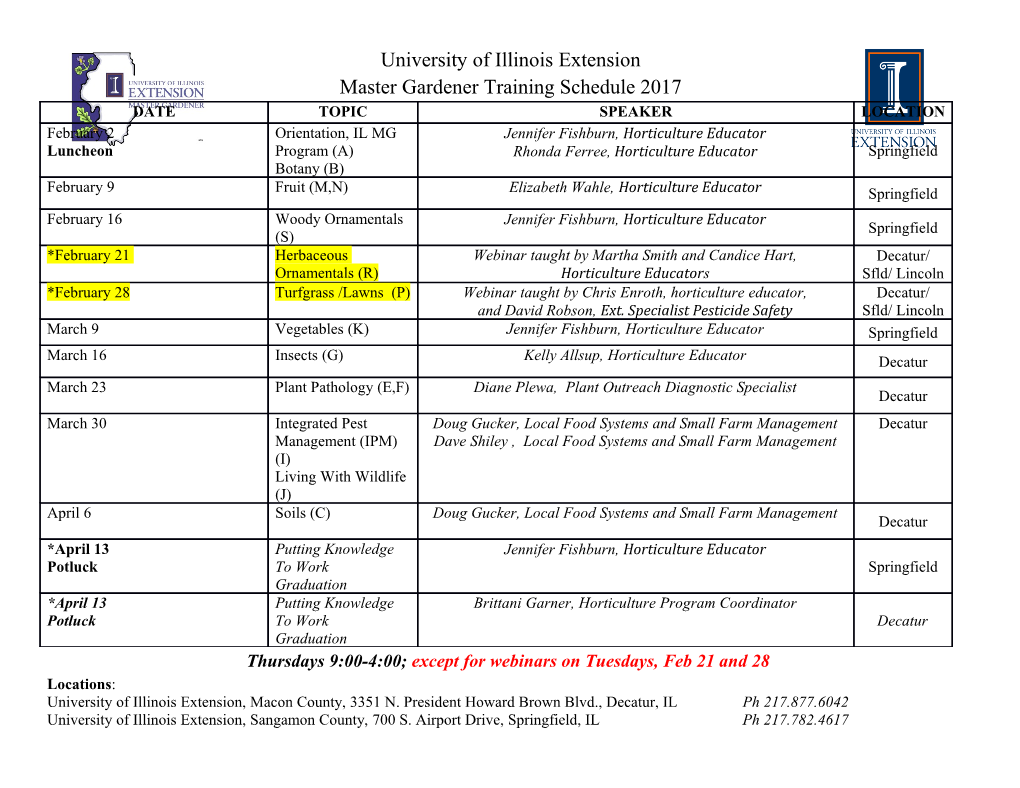
<p>P185 Lab Manual Error Analysis 1 Revised 4/17/07</p><p>Error Analysis in Physics 185 Spring 2007</p><p>Sec. Subject Page</p><p>1. Finding and categorizing errors 1 2. Combining errors 5 3. Propagating errors using direct substitution 6 4. Propagating errors using formulas 9 5. Looking for trends 12 6. Digitization and rounding error 13 7. Errors in areas 14 8. Errors from computer fit lines 16 9. Comparing to zero 19 10. Error bars on graphs 20 11. Statistics and standard deviation 21</p><p>Sections one through four cover the essentials of error analysis. Sections five through eleven are optional; sections ten and eleven are the most important of these. </p><p>1. The Basics of Uncertainty</p><p>1.1 What are “error” and “uncertainty?” </p><p>We all know that science is based on observation and measurement, and that these are imperfect. In physics we try to put a numerical value on the imperfection. This is usually stated as a range for any given measurement. For example, instead of saying that I am 179 cm tall, I might say that my height is in between 178 and 180 cm. We can express this by stating the middle of the range (179 cm) and how far above or below this you might go (1 cm up or down): “My height is 179 cm plus or minus 1 cm.” </p><p>The number “1 cm” is called the error or the uncertainty. In science, these terms both mean the same thing. In this context “error” does not mean “mistake.”</p><p>Stating something this way does not mean that I am 100% certain that my height is in the given range. There is a small possibility (about 15%) that my P185 Lab Manual Error Analysis 2 Revised 4/17/07 height could really be more than 180 cm, and the same chance that it could be less than 178 cm.</p><p>1.2 What are the basic kinds of uncertainty?</p><p>Making a mistake is one source. This is called a blunder, and since it is impossible to allow for this we usually ignore blunders. Clearly if you discover a blunder you will re-do the measurement, and if you don’t discover you will have no way of quantifying it. “Error analysis” does not take blunders into account.</p><p>The second source is random error. This is due to effects that could make your measurement either larger or smaller than the real value. For example, if you measure time with a stopwatch you may click the button too soon or too late. This will produce errors that can go either way.</p><p>Random errors do not form a trend, which means they may make your experiment less accurate but they will not distort the overall pattern of the data. We are mostly concerned with random errors in this lab.</p><p>The third source is systematic error. This is an effect that always makes your measurement either bigger or smaller than it should be, and so distorts the results. For example, if you drop a ball and measure its speed, air resistance will always slow it down. If your theory doesn’t take air resistance into account, your speeds will always be slower than predicted.</p><p>Systematic errors are often difficult to predict.</p><p>1.3 What are the sources of uncertainty?</p><p>Reading error is always present. This error is due to that fact that you cannot read an instrument with infinite precision. For example, a meter stick has millimeter markings on it. You can see which of these marks is the closest to your object, but it’s hard to estimate ranges between the marks. So you can’t read a meter stick more accurately than to within 0.5 mm.</p><p>Reading error is usually one-half of the smallest division of an instrument. If the instrument is digital, then it is half of the last digit position. For a stopwatch that has two decimals, for example, you might see</p><p> t = 3.15 s ± .005 s</p><p>Occasionally on a digital instrument the last digit or last few digits will not be stable. In this case the reading error will be at least one in the fluctuating digit, and maybe higher depending on the size of the fluctuations. P185 Lab Manual Error Analysis 3 Revised 4/17/07 The symbol for uncertainty is the Greek letter sigma: . So for the stopwatch above we can write</p><p>(t) = .005 s</p><p>You could say “The error in t is point zero zero five seconds,” or “The uncertainty on t is point zero zero five seconds,” or “Sigma of t is point zero zero five seconds.”</p><p>Calibration error comes from small imperfections in the measuring device itself. For example a meter stick might say that its calibration error is 0.2%. This means any measurement could be off by 0.2% of itself. So if you measure something have a length “L” of 45 cm, you can write</p><p>(L) = (.002) X 45 cm = 0.09 cm</p><p>Calibration errors are usually obtained from the documentation that comes with the instrument. In the end, though, it is a question of how accurate you trust the instrument to be. An old instrument may have a calibration error much larger than what the manual claims.</p><p>Other errors may be present that can’t be categorized as reading or calibration errors. Things like air resistance, friction or thermal expansion may change your results in a way you can’t predict. In this case you must estimate the size of the uncertainty these things are producing, which in some cases is a matter of intuition or just plain guesswork.</p><p>1.4 How is uncertainty presented?</p><p>A measurement should generally have an uncertainty to go along with it. It is given in “plus-or-minus” format, and the uncertainty has the same units as the quantity itself. This is called the absolute uncertainty and is written like this:</p><p> d = 32.1 cm ± 0.1 cm</p><p>It can also be stated as a percentage uncertainty: </p><p> d = 32.1 cm ± 0.3 %</p><p>Sometimes the range will be asymmetric:</p><p> d = 32.1 cm + 0.2 / -0.1 cm</p><p>Note that the absolute uncertainty and percent uncertainty are simply two different ways of stating the same information. Any source of uncertainty can be expressed in absolute form (with units) or as a percentage. P185 Lab Manual Error Analysis 4 Revised 4/17/07</p><p>The absolute uncertainty is not the same as the total uncertainty, which is what you get when you combine uncertainties from different sources. This is discussed in the next section.</p><p>1.5 How are the absolute and % uncertainties related?</p><p>To calculate the percentage uncertainty of a quantity “x”, use this formula:</p><p> (x) %Uncertainty 100 Eq. 1 x where (x) is the absolute uncertainty. An absolute uncertainty should always have units on it, and generally the same units as the original measurement. A percentage uncertainty has no units.</p><p>You may have a percent uncertainty and want to find the absolute :</p><p>%Uncertainty x (x) Eq. 2 100</p><p>Since an uncertainty represents the degree to which something is not known, it is usually rounded to one significant digit, unless that digit is a “1” or unless you have a good reason for using more.</p><p>1.6 What do I do with uncertainties?</p><p>We usually want to compare a measurement with a theory. Since these will never be exactly the same, we need to know if they’re close enough for us to say the theory works. The simplest way is to find the absolute difference between the two numbers.</p><p>Abs. Diff (x) x x MEASURED THEORY Eq. 3 Another way is to compare by percentage difference:</p><p> xMEASURED xTHEORY 980 981 %Diff 100 100 0.1% Eq. 4 xTHEORY 981</p><p>This gives you an idea of how the size of the difference (ideally it’s zero) compares with the thing being measured. This comparison is useful.</p><p>The key test is whether the theoretical value is within the range of the uncertainty. For example, say you measure the value of “g” to be g = 980 cm/s2 ± 3 m/s2. The book says the value is 981 cm/s2. You can say your measurement P185 Lab Manual Error Analysis 5 Revised 4/17/07 differed from theory by 1 cm/s2. This doesn’t really tell you much though—is that reasonable, or too much?</p><p>Your range is between 977 (980 – 3) and 983 (980 + 3). Since 981 is in between these values, your measurement agrees with theory. In general, your measurement agrees with theory if</p><p> xMEASURED (x) xTHEORY xMEASURED (x)</p><p>Of course this doesn’t “prove” your theory, it just means you haven’t disproved it, and it’s evidence in favor of the theory. The smaller the uncertainty, the more convincing a measurement is as a test of a theory.</p><p>Questions to think about:</p><p> In what circumstances would the % uncertainty be most useful?</p><p> If a measurement is more than 1 different from the theory, has the experiment been a waste of time?</p><p> Is a measurement that is less than 1 different from the theory always a “good” result that you could publish?</p><p>2. Combining Errors</p><p>2.1 How to deal with several uncertainties</p><p>Consider a meter stick measurement. It actually has two errors, reading error and calibration error. These are independent of each other, so the total uncertainty must somehow combine the two. The way to do this is simple. Let’s say we measure L to be 45 cm, with a reading error of 0.05 cm and a calibration error of 2%. Since we can’t mix apples and oranges, we need both of these errors either in cm or in %:</p><p>Absolute (cm) % Reading 0.05 0.1 Calibration 0.9 2</p><p>To combine them, take the square root of the sum of the squares, which I will abbreviate as “SRSS” from now on:</p><p>2 2 2 (L) 1 2 3 Eq. 5 </p><p>For our data we have only two errors, reading and calibration: P185 Lab Manual Error Analysis 6 Revised 4/17/07</p><p> (L) (0.05cm)2 (0.1cm)2 0.11 cm or</p><p> (L) (0.9%)2 (2%)2 2.2%</p><p>Notice that the absolute and % don’t quite match, due to rounding errors. </p><p>There may be other sources of uncertainty. For example, if you can’t hold the meter stick directly in front of the object you’re measuring, this introduces additional uncertainty. Estimating these uncertainties is usually a matter of judgment, not formulas.</p><p>If you had more errors, you would square them all, add them, and take the square root of the resulting number. </p><p>If you look at this formula you will find that in general you can ignore any error which is less than 1/3 of the largest error. This is an important time- saver.</p><p>Note that this technique applies only to several different sources of error on one single number, in this case a length measurement. You don’t use this if you have to combine several measurements, each with its own uncertainty, in a formula. For that see section 3.</p><p>Questions to think about:</p><p> Is the SRSS bigger or smaller than the sum of the uncertainties?</p><p> Why use SRSS instead of the sum?</p><p>3. Propagating Errors: Direct Substitution</p><p>3.1 What if one number depends on another?</p><p>Consider a car traveling at a steady speed of 30 m/s. Let’s assume we know the speed exactly. The car’s position is given by the very simple formula x = vt, or x = 30t. We measure the time traveled and use this to predict the position, but our clock has an uncertainty of ± 1s. Clearly this translates to an uncertainty in position of 30m, but we’d like a general way of figuring this kind of thing out. P185 Lab Manual Error Analysis 7 Revised 4/17/07 When an uncertainty in one number creates an uncertainty in another number (that depends on the first number) this is called propagation of error. In our example an uncertainty in “t” propagates through to an uncertainty in “x”.</p><p>One way of finding out how errors propagate is by direct substitution. In our example with the car, let’s say t = 50 s ± 1 s. This means the highest and lowest values for t are 51 s and 49 s. This gives us our highest and lowest values for x:</p><p> xMAX (30 m / s)(51s) 1530 m xMIN (30m / s)(49s) 1470m</p><p>This gives us a range, which we can express as x = 1500 m ± 30 m. An uncertainty of 1 s in the time propagates through to an uncertainty of 30 m in the position.</p><p>3.2 What if I have a formula?</p><p>Let’s consider a more complicated situation. An object falling in air might obey this formula for its speed:</p><p> v(t) v 0 [1 exp(kt)]</p><p>The parameter “k” depends on the air resistance of the falling object. Let’s say k -1 = 0.08 s and v0 = 15 m/s.</p><p>We want to know what the velocity will be at t = 10 s, but measuring the time gives an uncertainty of 0.5 s. Let’s make a chart of t and v:</p><p>Quantity Value Abs. Unc. Max Value Min Value t 10 0.5 v</p><p>We proceed to fill in the value for v:</p><p> v(10) (15)[1 exp((0.08)(10))] 8.26</p><p>We also fill in the max and min values for t:</p><p>Quantity Value Abs. Unc. Max Value Min Value t 10 0.5 10.5 9.5 v 8.26 P185 Lab Manual Error Analysis 8 Revised 4/17/07 Next plug the max and min values for t into the formula to find the max and min values for v: v(10.5) (15)[1 exp((0.08)(10.5))] 8.52 v(9.5) (15)[1 exp((0.08)(9.5))] 7.99 Quantity Value Abs. Unc. Max Value Min Value t 10 0.5 10.5 9.5 v 8.26 8.52 7.99</p><p>Compare the max and min values for v to v(10) to find the uncertainty. The range is to +0.26 m/s and – 0.27 m/s.</p><p>You will get slightly different numbers for the range up and the range down, but unless they differ by a large percentage it doesn’t matter—the usual method is to take the average. This gives us (v) = 0.265 m/s, which we round to 0.27 or 0.3 m/s—remember most uncertainties only have one digit anyway.</p><p>3.2 What if I have two or more variable with uncertainties?</p><p>Let’s say you’re trying to find the velocity of a car by measuring the time it takes to go between two marks (this is a common method used by police to catch speeders). We find that a car takes 6.5 s to go between marks 135 m apart.</p><p>The formula for speed is simple enough: v = x/t. Our stopwatch has an uncertainty of 0.2 s, and the distance between the marks has an uncertainty of 1 m. How do you find the range of v? </p><p>Let’s start by filling in the chart with the numbers we’ve measured:</p><p>Quantity Value Abs. Unc. Max Value Min Value x 135 1 t 6.5 0.2 v</p><p>You can fill in v = 135/6.5 = 20.8 m/s immediately. You can also fill in the max and min values for x and t:</p><p>Quantity Value Abs. Unc. Max Value Min Value x 135 1 136 134 t 6.5 0.2 6.7 6.3 v 20.8 P185 Lab Manual Error Analysis 9 Revised 4/17/07</p><p>Now you have a decision: how to combine the max and min values for these to get the max and min values for v.</p><p>In this case it’s pretty clear that to find the largest v, you need the largest x (since it’s on top) and the smallest t (since it’s on the bottom). This gives you a max value for v of 136 m / 6.3 s = 21.6 m/s. Filling in the table gives</p><p>Quantity Value Abs. Unc. Max Value Min Value x 135 1 136 134 t 6.5 0.2 6.7 6.3 v 20.8 21.6 20.0</p><p>This gives us a range of +0.8 m/s and – 0.8 m/s. This technique can be extended to any number of variables with uncertainties, but you have to be careful about whether an increase in any given variable will make the final outcome bigger or smaller. To generalize, you should always fill in the chart in this order:</p><p>Quantity Value Abs. Unc. Max Value Min Value Variable 1st 1st 2nd 2nd Variable 1st 1st 2nd 2nd result 2nd 4th 3rd 3rd</p><p>Questions to think about:</p><p> If the speed limit is 40 mph (17.9 m/s) will this guy get a ticket? Could he defend himself in court by claiming that the speed is uncertain? How about if the speed limit is 45 mph (20.1 m/s)?</p><p> Can a formula itself have an uncertainty?</p><p> What’s a surefire way of telling whether or not increasing one variable in a formula will increase or decrease the result?</p><p>4. Propagating Errors: Formulas</p><p>4.1 Is there a more sophisticated method for propagation?</p><p>Now that you mention it, there is—but it involves some basic calculus. Let’s take an example. We have an object in free-fall, so distance fallen is related to the time in the air by P185 Lab Manual Error Analysis 10 Revised 4/17/07</p><p> x (1/ 2)gt 2</p><p> dx Let’s take the derivative: gt . We can re-write this: dx gt dt . What this dt means is that if you change the time “t” by a very small amount dt, then “x” changes by a very small amount dx. We can see how this works graphically:</p><p>500 </p><p>400 </p><p>300 x(t) </p><p>200 </p><p>100 dx </p><p> dt 0 0 2 4 6 8 10 t </p><p>An error is (hopefully) a small deviation, so this technique lets us find out how a small error in t creates a corresponding uncertainty in x. We can write this in terms of uncertainties as</p><p> (x) gt (t)</p><p>Let’s say that g = 10 m/s2 and t = 4 s ± 0.5 s. This gives x (1/ 2)(10)(4)2 80m and</p><p> (x) (10m / s 2 )(4s)(0.5s) 20m</p><p>This can be generalized to any formula. If y f (x) then</p><p> df (y) (x) Eq. 6 dx</p><p>4.2 What if I have several variables? P185 Lab Manual Error Analysis 11 Revised 4/17/07</p><p>This is easy, although it’s often tedious. Just take a derivative for each variable. For example if the pressure of a gas is given by </p><p>T P nR V where T and V are the variables with uncertainties, then we have</p><p>P 1 P T nR nR T V V V 2</p><p>Note that the derivatives are now partial derivatives. If you don’t know what a partial derivative is, ask your instructor to show you. We can re-write the derivatives as</p><p> 1 T P nR T P nR V V V 2 </p><p>If we let the small variation ∂T become the uncertainty (T) we get</p><p> 1 T T (P) nR (T ) V (P) nR (V ) V V 2 </p><p>(We ignore negative signs on errors.) Since we have two sources of error, we have to combine them:</p><p>2 2 (P) T (P) V (P)</p><p>I will use the “ ” symbol as a shorthand to mean you should use the square root of the sum of the squares to add the uncertainties: </p><p> (P) T (P) V (P) Eq. 7 </p><p>In general form, if u f (x,y,z)</p><p> f f f (u) (x) (y) (z) Eq. 8 x y z </p><p>4.3 Can I just memorize these formulas? P185 Lab Manual Error Analysis 12 Revised 4/17/07 Absolutely. Most formulas you will encounter fall into a very small number of categories, so the formulas for error propagation can be memorized. For example</p><p> if y(x) Ax then (y) A (x) Eq. 9 </p><p> if y(x) Ax n then (y) nAx n1 (x) Eq. 10 </p><p>A if y(x) Aln(x) then (y) (x) Eq. 11 x</p><p> kx kx if y(x) Ae then (y) kAe (x) Eq. 12 </p><p>Keep in mind that 1/ x x 1 , and x x1/ 2 , so these work with the second formula above.</p><p>Note how a little rearrangement of the formulas gives you relative errors, which are sometimes very useful.</p><p>If you use a formula not on this list you’ll need to use derivatives.</p><p>Questions to think about:</p><p>Show that</p><p> (y) (x) (y) (x) If y(x) Ax , then and if y(x) Ax n then n y x y x</p><p> (P) (T ) (V ) P T V</p><p> Which would work better for these situations, direct substitution or analysis with derivatives?</p><p> o Sometimes you want to work backwards: from a maximum allowable uncertainty on a derived quantity, you need to figure out how much error is allowable on the basic quantities. For example, if I need to know my speed to within 1%, how much error is allowable on measurements of distance and time? </p><p> o You want to find out how high you can go on a particular variable before the error becomes too large. P185 Lab Manual Error Analysis 13 Revised 4/17/07 o You want to determine which variable contributes most to the total uncertainty over a wide range of possible values of several variables.</p><p>5. Looking for trends</p><p>5.1 Is error analysis the whole error story?</p><p>That would be too much to ask for. You also have to see if there is a general trend to your data. This can be accomplished two ways.</p><p>First, have the computer create a fit line for your theory. For example, if your object is in free-fall, you expect that the distance fallen relates to the time in the air according to x (1/ 2)gt 2 . If you let the computer do its work, it will create a parabola and most of your data points should be near this curve.</p><p>One thing you can check right away: about half your points should be above the curve and half below. A serious imbalance means you need to look further.</p><p>Next, check to see if your data points seem to generally follow the curve or if they start to depart from it at some point. If this happens there are two possibilities:</p><p>(a) Your theoretical formula is missing something or incorrect, or (b) your formula has limits, and once your experiment goes above or below these limits the theory no longer applies. Knowing the limits of your theory can be crucial to understanding a physical phenomenon.</p><p>The easiest way to see a trend is to linearize your theory. For example, if you plot your free-fall data so that t2 (not t) is on the x-axis and x is on the y-axis, the data should fall on a straight line. The human eye is very, very good at detecting departures from a straight line.</p><p>Questions to think about.</p><p> In the free-fall experiment, what would the general trend be: To follow the fit line x (1/ 2)gt 2 , or go above it, or go below it?</p><p> In the free-fall experiment, assume that the data seems to match the theory x (1/ 2)gt 2 over some range of t’s. Would you expect there to be an upper limit on the value of t where this is true? A lower limit? Why?</p><p>6. Digitization and Rounding Error</p><p>6.1 What is the error from a computerized device? P185 Lab Manual Error Analysis 14 Revised 4/17/07 A measuring device will have a calibration error and a reading error just like other devices, but is may also have an error due to the fact that it is a digital device. This means that computers measure things in discrete steps. </p><p>For example a computer may take a sample every 0.1 seconds. This means that the computer might be averaging over this period of time or it might sample at exactly one instant of time. Then you would know nothing about what happens in between the tenth-second samples.</p><p>Computers typically work by setting a range over which they will sample a quantity. For example you might have a force sensor which has a range of 0 to 10 newtons. The computer then divides this range up into a fixed number of steps, say 128 (can you guess why I chose 128?). This would mean each step is 10/128 = 0.078 N. This means the uncertainty inherent in this sampling process is ± 0.039 N. This may be considerably bigger than the reading error.</p><p>For a computer, the uncertainty is one-half the step size or the reading error, whichever is larger.</p><p>6.2 Is rounding error important?</p><p>These days we have calculators that give us lots of digits, so usually the answer is “no.” Rounding error is only important if it takes place before you get your final result.</p><p>Questions to think about:</p><p> Besides using the manual, what are some ways you could determine the size of the increment the computer is using to measure a quantity?</p><p> If you are in doubt about how many digits to round off to, would you use more digits or fewer digits? Why?</p><p> Why is rounding (i.e. significant digits) less important on numbers with uncertainties included?</p><p>7. Errors in Areas</p><p>7.1 How does the computer find the area under a curve?</p><p>Some of our experiments involve having the computer find the area under a curve, or the integral of a quantity. To do this, the computer divides the curve into thin vertical strips, finds the area of each strip, and adds up all the areas. Usually time “t” is on the horizontal axis. For example data from a force-sensor might look like this: P185 Lab Manual Error Analysis 15 Revised 4/17/07</p><p>F </p><p> t </p><p>Δt </p><p>The height of each strip if F(t) and the width is t, so the area of each box is just F(t)t. Each box in numbered. The number of a strip is called “i”, with i running from 1 to N, where N is the total number of strips. The height of a strip becomes Fi(t), or just Fi.</p><p>F </p><p> t i = N i = 1 i = 8 </p><p>The integral is the sum of all the strips:</p><p>N Area F(t)dt F t i Eq. 13 i 1</p><p>If there are N data points then the total time interval “T” is N t. </p><p>7.2 How do I find the error for the area under a curve?</p><p>Assume that the uncertainty on each F is a constant (F). The error for the area of each strip in the integral is (A) (F) t</p><p>Adding up the error for each small strip (using the SRSS sum) gives</p><p>N (Area) (F) t2 i 1 P185 Lab Manual Error Analysis 16 Revised 4/17/07 Since the ’s are all the same and the t’s are all the same, each term in the sum is the same and we can write</p><p>2 (Area) N (F) t N (F) t Eq. 14 </p><p>This can be put into a more convenient form by using T = Nt:</p><p> (F)T (Area) Eq. 15 N</p><p>You can state this in terms of relative errors:</p><p> (Area) (F) 1 Area FAVG N</p><p>This is a general result: when you have N points, the uncertainty is reduced by a factor of N . Note that I have written the area as A = FAVG T.</p><p>Questions to think about</p><p> Each data point has an uncertainty of . Can you explain why N data points wouldn’t have an uncertainty of N?</p><p> Using the computer to find an integral has another problem: the zero level, or baseline, may not actually be at zero. Can you think of a way to find the baseline and estimate its uncertainty?</p><p>8. Errors From Computer Fit Lines</p><p>8.1 Will the computer tell me the error on a fit line?</p><p>Yes it will, but not necessarily in a form that’s easy to use. The most common fit line is a straight line. The computer gives you the slope m and the intercept b, and what you want is the uncertainties (m) and (b).</p><p>A common way of comparing data to a fit line is called the variance. Suppose your data looks like this: P185 Lab Manual Error Analysis 17 Revised 4/17/07</p><p>10 </p><p>8 </p><p>6 </p><p>4 </p><p>2 </p><p>0 0 2 4 6 8 10 x </p><p>The computer generates a straight line that best fits the data. To find out how close your data points are to the fit line we go through several steps.</p><p> First find the distance from each point the fit line. For example, suppose you have a data point at (2, 3.8). The fit line is</p><p> y (0.7)x 2.0</p><p>What this means is that according to your fit line the data point should be y = (0.7)(2) + 2.0 = 3.4. Since your measured y-value is actually 3.8, the data point is off by 0.4. For the data above, the y-values are</p><p> x y Theory Diff Diff2 2 3.8 (0.7)(2)+2.0 = 3.4 0.4 0.16 4 4.4 (0.7)(4)+2.0 = 4.8 -0.4 0.16 6 7.0 (0.7)(6)+2.0 = 6.2 0.8 0.64 8 7.5 (0.7)(8)+2.0 = 7.6 -0.1 0.01</p><p> After finding all the differences, square them. This gets rid of any negative signs, and gives more weight to points that are farther away from the line.</p><p> Divide by the number of points to get an average. However, for reasons I won’t go into here, you actually divide by N – 2, where N is the number of data points.</p><p> Take the square root. The variance for the above data is P185 Lab Manual Error Analysis 18 Revised 4/17/07</p><p>0.16 0.16 0.64 0.01 s 0.7 4 2</p><p>This represents the “average” difference between your theory and your data. </p><p>To generalize this, assume you have data in the form of individual points (x, y). We label these with a subscript “i”, so you have a set of points {(xi, yi)}, where “i” runs from 1 to N, N being the total number of data points. In the above example, x1 = 2, y1 = 3.8, and y4 = 7.5.</p><p>We assume that the data is supposed to fit a theory which says that y = mx + b. In this case, the formula for the variance is</p><p>1 2 s (y i mxi b) Eq. 16 N 2 i</p><p>The variance s should be about equal to a typical uncertainty on y.</p><p>Another measure of the “goodness of fit” between your theory and the data is called Chi-squared:</p><p>1 2 (y mx b)2 Eq. 17 2 i i (y) i</p><p>The 2 value should be about equal to the number of degrees of freedom in the experiment.</p><p>8.2 If I use a fit line to find a number like “g,” what’s the error?</p><p>Many times what we want from our data is the slope or the intercept of a fit line. For example, in free-fall the speed of a falling ball is given by v = gt. If we graph v(t), then the slope of the fit line is “g.” But how accurately can we find “g” from this? You might expect it would have something to do with the uncertainty on individual measurements, but there is another factor involved. Since the fit line is going through many data points, the errors tend to cancel out and give us a more accurate value for the slope than one measurement would.</p><p>Let’s first define something you might have seen before: the standard deviation. This is a measure of how much a set of data spreads out from its average value. The symbol for the standard deviation is also , so I will write it as ({xi}) to distinguish it from (x), which is the uncertainty on a measurement of x. The standard deviation of a set of values {xi} is P185 Lab Manual Error Analysis 19 Revised 4/17/07</p><p>1 2 ({xi }) xi x Eq. 18 N i where x is the average of all the x-values. Normally this is used for finding the spread of many measurements of a single value.</p><p>In our case where the xi’s all represent different measurements, ({xi}) is meaningless, but it is useful for using the formulas below.</p><p>If your data follows a straight line of the form y = mx + b, then the uncertainties on the slope m and intercept b are given by</p><p> (y) 2 2 (m) (b) (m) x ({xi }) Eq. 19a, b N ({xi })</p><p>I have put these formula in terms of the standard deviation and the average because these functions are readily available in software packages and on calculators.</p><p>These equations are based on formulas from Bevington’s “Data Reduction and Error Analysis for the Physical Sciences,” McGraw-Hill, © 1969.</p><p>Don’t try to use these formulas by hand—let a computer do the work.</p><p>Questions to think about</p><p> If your fit line has an uncertainty on m and b, and your x-values also have an uncertainty, what is the formula for the uncertainty on y you calculate using y = mx + b? </p><p> How does the computer find the RMS error for a curve other than a line?</p><p>9. Comparing to Zero</p><p>9.1 What if the theoretical result is zero?</p><p>Consider an experiment in which two carts push off each other with springs. The initial moment of each cart is zero (since they’re moving). In theory the final momentums of the carts should also add to zero. </p><p> p1,INITIAL p2,INITIAL p1,FINAL p2,FINAL 0</p><p>Consider trying to compare the initial and final momentums using the usual formula: P185 Lab Manual Error Analysis 20 Revised 4/17/07 p 0 %Diff FINAL 100 0</p><p>We have to modify the formula in this case by comparing the final momentum to just to something that represents the approximate size of the quantities involved. In this case, averaging the two final momentums would work:</p><p>2 p %Diff FINAL 100 p1,FINAL p2,FINAL</p><p>Note the factor of 2 which gives us the average. There is no standard formula for this situation, so you have to use your judgment.</p><p>Questions to think about</p><p> If the expected result is zero, can you can still compare theory to measurement using ’s?</p><p>10. Error Bars </p><p>10.1 How do I show the size of the errors on a graph?</p><p>You have a set of data points (x1,y1 ),(x2 ,y 2 ),(x3,y 3 ) that you have graphed. You want to show the size of the uncertainties on your graph. You need to start by knowing the uncertainties on each individual point. In many cases, the uncertainties on the x-values are small and will be ignored. The uncertainties on the y-values are shown as error bars, which are simply bars going above and below the point. Each bar’s length is the size of the uncertainty.</p><p>Suppose your data looks like this:</p><p> x y (y) 2 3.8 0.5 4 4.4 0.5 6 7.0 0.5 8 7.5 1.0</p><p>The graph with error bars looks like this P185 Lab Manual Error Analysis 21 Revised 4/17/07</p><p>10 </p><p>8 </p><p>6 </p><p> y </p><p>4 </p><p>2 </p><p>0 0 2 4 6 8 10 x </p><p>Questions to think about</p><p> How do you tell how many points are within 1 of the fit line, just by looking at the graph?</p><p> How could the fit line account for the fact that the is different for each point? (i.e., require it to be closer to points with small ’s than large ones.)</p><p>11. Statistics and Standard Deviation</p><p>11.1 What if I have no way of estimating the error?</p><p>You don’t always have a book value to compare too. In one experiment we will be measuring the speed of sound cS. The experiment is repeated as one parameter (the frequency of sound) is varied, so you end up with several values for cS. Of course we can look this up in a book, but what if we couldn’t?</p><p>In this situation we expect that all the values we measure should be about the same, and cluster around the true value of cS. So we take the average of all our values as our measurement of cS, and call this c . The difference between each value of cS and the average value should give us an estimate for our uncertainty.</p><p>To find this difference we use the same procedure as we did in section 8. </p><p>First find the difference between each value and the average. Let’s say we measured cS to be 330, 333, 334, and 337 m/s in four different trials. The average is (330 333 334 337)/ 4 333.5m / s . </p><p>For the data above, the values are P185 Lab Manual Error Analysis 22 Revised 4/17/07</p><p>Measured Val. Avg. Val. Diff Diff2 330 333.5 -3.5 12.25 333 333.5 -0.5 0.25 334 333.5 0.5 0.25 337 333.5 3.5 12.25</p><p>Square all the differences, add them up, divide by the number of points to get the average, then take the square root. This gives us</p><p>12.25 0.25 0.25 12.25 RMS 2.5 4</p><p>This represents the “average” difference between your theory and your data. This is called the standard deviation. Sometimes the formula has you divide by N – 1 instead of N, but this shouldn’t make a huge difference.</p><p>The s.d. is usually abbreviated as . In theory, about 70% of your measured values should be less than 1 different from your average value.</p><p>11.2 How does the statistical error compare to other errors?</p><p>In theory, the value of the s.d. should be about the same as the uncertainty on an individual date point. If there is a big difference it’s an indication that something is wrong:</p><p> If the s.d. is much bigger than the ’s, you may have underestimated or there may be an effect on the data that you haven’t accounted for.</p><p> If the s.d. is much smaller than the ’s you may have overestimated .</p><p>Questions to think about</p><p> Which affects the s.d. more, one value that is a lot different from the average, or several values which have only small differences from the average? </p>
Details
-
File Typepdf
-
Upload Time-
-
Content LanguagesEnglish
-
Upload UserAnonymous/Not logged-in
-
File Pages22 Page
-
File Size-