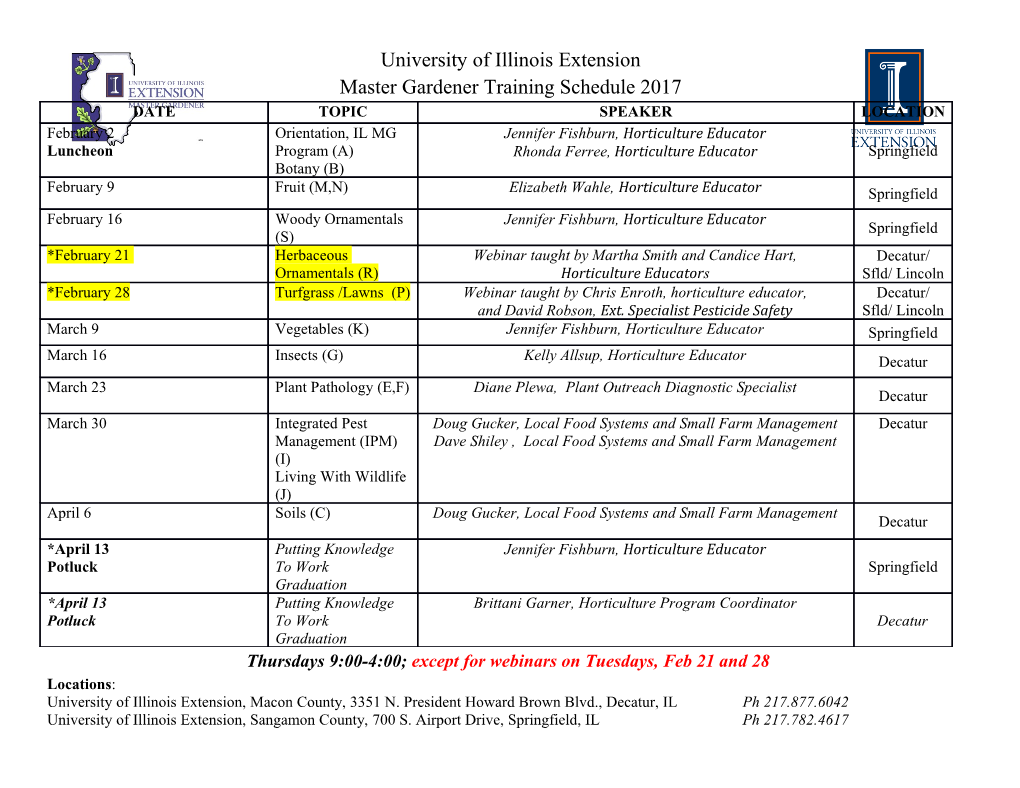
<p>Supplement: A Genome-Wide Association Study of Anorexia Nervosa Boraska … Bulik</p><p>Table of Contents</p><p>Supplemental Methods...... 2 QC of directly typed variants in discovery dataset...... 2 Imputation...... 2 Figure S1: Distribution of imputation accuracy scores ...... 3 Figure S2: Concordance of masked genotypes...... 3 Genome-wide association analysis...... 3 GWAS meta-analysis QC...... 4 Ancestry informative markers...... 4 Gene-based association test...... 4 Network analysis...... 4 AN subtype analyses...... 5 Expression studies...... 5 Supplemental Results...... 6 Meta-analysis results of the discovery dataset...... 6 Figure S3: QQ plot...... 6 Figure S4: Manhattan plot...... 7 Gene-based association test results...... 7 Network analysis results...... 7 Figure S5: Network analysis...... 8 Supplemental Discussion...... 8 Discussion of Genetic Loci...... 8 Supplemental References...... 12 Supplemental Tables...... 22</p><p>1 Supplemental Methods</p><p>QC of directly typed variants in discovery dataset The following sample-level QC criteria were applied: a) all SNPs were converted to forward strand; b) sample call rate exclusion threshold of 99%; c) cross-checking of reported sex; d) heterozygosity exclusion threshold of ±3 standard deviations (SD) from the mean; e) multidimensional scaling analysis (MDS) was performed using genome-wide set of directly genotyped common SNPs, plots were visually inspected and an exclusion lists with samples of non-European ancestry were generated. MDS plots with cases and controls matched by ancestry were also generated to inspect their compatibility and ancestry matching. All samples not matching case and control ancestry within each of 15 discovery dataset were excluded; f) identity by state (IBS)/ identity by descent (IBD) statistics were calculated to identify duplicated and related samples (exclusion threshold >0.05). All samples failing sample-level QC were removed within each case and control dataset separately prior to performing SNP-level QC. The SNP-level QC step was also carried out in each case and control dataset separately under the following criteria: a) SNP call rate threshold of 99%; b) deviation from Hardy-Weinberg Equilibrium (HWE) in controls (exact HWE p<1x10-4). All SNPs that failed individual SNP-level QC steps were removed. Low frequency and rare SNPs (<0.05) were excluded from cases and controls separately. Differential missingness between cases and controls was investigated within each stratum and SNPs that showed deviation visualized by quantile-quantile (QQ) plots were excluded. All subsequent association analyses were performed using merged case-control post- QC datasets. All QC steps were performed using PLINK.1 Imputation Two additional SNP filtering steps were performed prior to imputation. First, SNPs with ambiguous allele coding (A/T or C/G) were removed as the strand of these variants can be difficult to resolve. Second, variants not present in the HapMap3 release 2 reference panel were removed as they can not inform the imputation process. Remaining variants were then strand- matched to the reference data and imputation was carried out using Impute2. Post imputation QC consisted of the exclusion of variants with MAF < 0.05 or info score < 0.4 within each dataset. The effect of each of these filtering steps is shown in Table S2. We assessed the adequacy of imputation in multiple ways. We show the distribution of imputation accuracy metrics (as captured by the INFO score) in each dataset and across a range of minor allele frequencies (Figure S1). As expected, the info scores are consistently high (>0.8).</p><p>2 Figure S1: Distribution of imputation accuracy scores. Figure S2: Concordance of masked genotypes</p><p>We carried out an analysis of genotype concordance by masking directly genotyped variants and imputing them as though they had not been directly assayed. We present the results of this analysis (Figure S2). As expected, genotype concordance remains very high across the full range of examined minor allele frequencies and is consistently high (>0.8) in all studied populations.</p><p>Genome-wide association analysis Eleven association analyses were conducted per stratum: one with no principal components (PC) adjustment and the others adjusted for up to 10 PCs. PCs were calculated using EigenSoft.2 A list of the most informative 54 independent ancestry informative markers was created based on Tian et al.,3 to distinguish North-South European and Northern European substructure, and also based on the Wellcome Trust Case Control Consortium population stratification SNP list.4 Differences in allele frequencies of these 54 SNPs were examined between cases and controls in each stratum in each of the 11 association analyses as an additional test of ancestry matching between cases and controls (i.e., in addition to the MDS analysis already performed under standard QC). A Bonferroni corrected p-value of 0.0009 was considered significant evidence of population stratification. There were no SNPs reaching Bonferroni corrected p-value threshold in the analyses without adjustment for all cohorts except for the French dataset. For the French dataset, no ancestry marker showed association in the analysis adjusted for the first PC. We also constructed QQ plots for all 11 association analyses for all 15 discovery datasets and visually inspected them. There were no qualitative differences between QQ plots for 11 analyses within each case-control group, i.e., there were no inflations in test statistics in the analyses without adjustment, in comparison to the ones adjusted for up to 10 principal components (PCs). To cross check the adequacy of our approach to population stratification, we computed PCs on 41,838 independent (r2<0.2) SNPs directly genotyped in all cases and controls and performed individual association analyses adjusting for the first 3 PCs. We then performed meta-analysis -12 across 15 datasets and found equivalent results (GC=1.01, Pmin=1.66x10 at rs4957798 when 12 adjusting for the first 3 PCs) to the ones we report under Results (GC= 1.024, Pmin=1.67E- at rs4957798, Supplementary Table S5). </p><p>3 GWAS meta-analysis QC We examined all SNPs with p<10-4 and sample size greater than 1,000 to check for false positives due to genotyping error or other artefacts. We investigated cluster plots and exact HWE p<1.0x10-4 in cases or controls separately. Cluster plots were examined in studies with available intensity data using Evoker.5 Poorly genotyped/called SNPs were excluded within each dataset and meta-analyses using clean datasets were repeated. Results were graphically represented using QQ and Manhattan plots. The combined results presented here are not corrected for overall GC. Ancestry informative markers Replication samples in this study came from 15 populations of which 12 were of European ancestry and de novo genotyped for the prioritized set of SNPs. Replication cases and controls were carefully matched by country and collection center during collection phase. To additionally check for ancestry matching between cases and controls within each of the 12 replication datasets we included 27 ancestry-informative markers (AIMs) for genotyping. These markers were selected on the basis of our discovery dataset that contained samples from 15 populations of European ancestry. We performed principal components analysis (PCA) on these samples and found that the top two PCs aligned with perpendicular geographical axes (rho=0.90 for PC1 vs. latitude, rho=0.59 for PC2 vs. longitude). We identified markers that were contributed highly to these PCs, and found that even as few as 27 markers were sufficient to predict the ancestry of the samples well. For more information, please see reference.6 Gene-based association test We used VEGAS7 to conduct a gene-based association test using all variants in the GWAS. Briefly, VEGAS assigns SNPs to genes and uses all individual variant test statistics within a gene to calculate a gene-specific test statistic. VEGAS uses a simulation-based approach to account for gene length and LD. The Bonferroni-corrected p-value threshold is p<2.8x10-6. Network analysis We examined the genomic context within 0.5 Mb either direction of each of the 20 most statistically significant SNPs using Ensembl (version 70),8 and identified the two directly adjacent genes in each case. Of these 20 SNPs, we found two pairs of SNPs with the same adjacent genes. We found 3 SNPs with only one gene within the stated boundaries, and one SNP that lay close to three genes. Altogether this produced 34 candidate genes (Supplementary Table S4). In order to visualize the connections between this gene list, we created a network representing these 34 genes. Each node represents a gene. Genes adjacent to the same SNP are represented by nodes of the same color. Edges within the network represent connections between the genes. We obtained a number of different connections: 1. Co-citation in the same study. These data were obtained using the citations for each gene on Ensembl (release version 70)8 and the NHGRI database.9 2. Implication in the same psychiatric disorder. These data were obtained as in (1) 3. Implication in the same metabolic disorder. Data were obtained as in (1) 4. Implication in body shape phenotypes such as height, weight, etc. Data obtained as in (1). 5. Protein-protein interactions. These were obtained using the String-db database.10</p><p>4 In order to compare the connectedness of this network to one obtained by chance, we simulated one million random sets of 34 genes and repeated the analysis. We then calculated the network connectivity of each node in the network using two clustering coefficients, L and w, both for the observed network and for the simulated networks. W is a measure of local weighted connectivity, as described by Kalna et al.11 The second coefficient, Lj, is a measure of global connectivity. This is simply the average distance from node j to all other nodes. In the case where a node has no neighbors, the node is not included in the calculation. In order to test whether the 34 candidate genes are significantly more closely connected than would be expected by chance, we created random sets of 34 genes, and compared the clustering coefficients in each case. As it was not computationally feasible to investigate every gene in the simulated datasets using Ensembl and String-db, we restricted this analysis to the NHGRI database. AN subtype analyses AN restricting subtype included individuals who maintained low body weight solely through caloric restriction and increased energy expenditure. AN binge/purge subtype included individuals who also reported binge eating and/or purging via vomiting or laxatives. Expression studies RNAseq experiments sequence fragments of cDNA, which are then mapped to individual gene transcripts. This provides a fragment count, usually expressed in Fragments per Kilobase of exon Per Million fragments (FPKM) values, which correlates with relative gene expression. We analyzed RNAseq data from whole brain tissue samples from 12 different mouse strains. Analysis was performed as follows: 1. Fastq files were downloaded from the European Nucleotide Archive.12 ENA run IDs and strain information for each dataset are presented below. Mouse strains used, along with the relevant ENA accession IDs. Mouse Strain ENA ID A/J ERP000038 AKR/J ERP000037 BALB/cJ ERP000039 CBA/J ERP000043 DBA/2J ERP000044 NOD/ShiLtJ ERP000046 NZO/HILtJ ERP000047 129P2/OlaHsd ERP000034 SPRET/EiJ ERP000049 129S5SvEvBrd ERP000035 WSB/EiJ ERP000050 C57BL/6NJ; 2 samples ERP000041</p><p>2. The tophat13 and bowtie14 software packages were used to find the read alignments for each fastq file. 3. The samtools15 and HTseq16 software packages were then used to count the number of alignments per gene.</p><p>5 4. Count values were then normalized to account for the depth of sequencing and the transcript length, using the following formula: 1 0 9 * t o t a l _ c o u n t _ r e a d s N o r m a l i s e d _ c o u n t _ v a l u e s t o t a l _ m a p p e d _ r e a d s * t r a n s c r i p t _ l e n g t h Transcript lengths were obtained using the Ensembl GTF annotation (Version 63).8 The top 20 SNPs by statistical significance were used to identify candidate genes. We examined the genomic context within 0.5 Mb either direction of each SNP using Ensembl (version 70), 8 and identified the two directly adjacent genes in each case. Of these 20 SNPs, we found two pairs of SNPs with the same adjacent genes. We found 3 SNPs with only one gene within the stated boundaries, and one SNP that lay close to three genes. Altogether this produced 34 human candidate genes. 32 mouse orthologues of these genes were identified using Ensembl (version 70).8 Significant SNPs are shown with adjacent genes and mouse orthologues in Supplementary Table S4.</p><p>Supplemental Results</p><p>Meta-analysis results of the discovery dataset</p><p>Figure S3: QQ plot</p><p>6 Figure S4: Manhattan plot</p><p>Gene-based association test results There were no gene signals surpassing genome-wide significance levels (at p<2.8x10-6) for VEGAS. Two adjacent genes were associated with p<10-4: TMEM37 (P=4.0x10-5) and SCTR (P=4.7x10-5). The most significant variant in both genes was rs12479137. Its proxy rs2254122 (r2=1) was followed up in independent cohorts and resulted in a replication P=0.870. </p><p>Network analysis results We formulated a network using data on a number of interactions between the genes adjacent to the most significantly associated SNPs, (Supplementary Figure S5), and found that the genes form two distinct clusters, with a number of outliers. Many are closely related in this network, implying that they may be functionally related. Several of the genes are associated with body- shape or metabolic disorders (Supplementary Figure S5.b-d). Further, we find that these genes are significantly more closely related than would be expected by chance. We found a significantly shorter average pathway between genes (P=0.005) and significantly tighter clusters (P=0.03) when using the AN genes compared to one million randomly generated sets of 34 genes.</p><p>7 Figure S5: Network analysis A Network view of 34 genes. Nodes represent 34 genes adjacent to the top 20 statistically significant SNPs. Edges represent connections between the nodes. A) A network showing all identified connections between the nodes. B) A network showing genes implicated in the same psychiatric disorders. C) A network showing genes implicated in the same metabolic disorders. D) A network showing genes implicated in body shape phenotypes. </p><p>Supplemental Discussion</p><p>Discussion of Genetic Loci Here, we briefly describe functions of genetic loci identified through our study. The majority of reported genes are expressed in the brain and have already been associated with a neurodegenerative or neurodevelopmental disorder. </p><p>8 SOX2OT is a non-coding RNA that is expressed in several regions of the human brain.17 By applying the Logic Mining method to a gene expression dataset from transgenic mice, Sox2ot gene was identified as potential biomarker for the early and late stage of Alzheimer's disease neurodegeneration.18 SOX2OT was also suggested to have a role in the etiology of myopia.19 PPP3CA gene (also known as calcineurin) is also expressed in several regions of human brain (USCS site - GNF Expression Atlas 2 Data from U133A and GNF1H Chips). This gene has been implicated in the etiology of Alzheimer's disease. It is a serine-threonine phosphatase that dephosphorylates tau, as tau hyperphosphorylation is one of hallmarks of Alzheimer's disease.20 Moreover, altered expression of novel alternatively spliced PPP3CA isoforms were found in brain regions of postmortem Alzheimer's disease patients.21 The PPP3CA gene is differentially expressed between patients with frontotemporal degeneration and controls.22 Genetic variants in PPP3CA have been associated with human endurance exercise phenotype traits.21 CSGALNACT1 (also known as ChGn) plays a role in chondroitin sulfate biosynthesis in cartilage but is also ubiquitously expressed in various other tissues.25 Chondroitin sulfate proteoglycans are likely to be regulatory molecules in the process of axonal degeneration/regeneration is in the peripheral nervous system and it was shown that CSGALNACT1 missense mutations are associated with pathogenetic mechanisms in peripheral neuropathies.26 SYN2 (synapsin II), a member of the synapsin family, encodes for neuronal phosphoprotein found in synaptic vesicles that can modulate synaptic transmission and plasticity. 27 Because of its role in synaptogenesis and the modulation of neurotransmitter release, this gene was suggested to be involved in several neurological and neuropsychiatric diseases including epilepsy and schizophrenia. In addition, SynII knock-out (KO) mice showed autism-related behavioral abnormalities.32 SPATA13 (also known as ASEF2) has a role in cell migration and it was shown that it is required for aberrant migration of colorectal tumor cells.33 SPATA13 was shown to be part of the pathway that regulates microtubule and actin dynamics involved in brain lamination.34 NCAM2 (also known as OCAM) belongs to the immunoglobulin superfamily, is primarily expressed in the brain and is important for the organization of axons and dendrites in the olfactory system.35 NCAM2 was suggested to carry a genetic risk for development of Down syndrome35 and Alzheimer’s disease (AD).36 In addition, the polymorphism found to be associated with changes in cerebrospinal fluid levels lies near NCAM2 gene.37 A case-report of a man with pervasive developmental disorder bearing deletion of 19 genes, including NCAM2, points to the possible role of this gene in autism and other neurobehavioral disorders.38 Also, some prostate and breast cancer cell lines showed differential expression of NCAM2 in comparison with healthy cells. PRKAR2B (also known as RII-BETA) encodes for a regulatory subunit of protein kinase A (a central component of the cAMP pathway which is important for a variety of cellular functions) and it is abundantly expressed in adipose tissue and brain. Studies in KO mice suggested an important role of this gene in regulating energy balance and adiposity.43 PRKAR2B KO mice have markedly diminished white adipose tissue, elevated metabolic rate and body temperature resulting in lean phenotype43 and are resistant to diet-induced obesity, insulin resistance and dyslipidemia.41 Moreover, deletion of PRKAR2B decreases body weight and increases energy expenditure in the obese, leptin-deficient mice.44 PRKAR2B was also found to be down-regulated in obese subjects when compared to lean ones.45 In addition, PRKAR2B regulates voluntary consumption of alcohol46 and PRKAR2B KO mice drink significantly more ethanol and are </p><p>9 resistant to ethanol-induced sedation.47 In brain, mRNA for calcium-calmodulin-dependent protein kinase IIbeta is elevated in the frontal cortex of cases with schizophrenia.48 PRKAR2B has also been shown to mediate adverse effects of antipsychotic medication49 and cataleptic behaviour induced by haloperidol50 This gene has been associated with several other disorders. For example, susceptibility locus for knee osteoarthritis lies in a linkage disequilibrium block that contains PRKAR2B gene.51 Also, reduced expression levels of this gene were observed in osteoarthritis cartilage compared with control cartilage.52 Nuclear PRKAR2B levels are found to be abnormally high in systemic lupus erythematosus T cells. PRKAR2B was also suggested to influence homocysteine levels.55 CTNNA2, alpha N-catenin 2, is associated with the cadherin cell adhesion molecules.56 In mouse, it is expressed almost exclusively in the nervous system and has a key role in stability of synaptic contacts. In primates, CTNNA2 is expressed in all layers of adult dorsolateral prefrontal cortex and in all neuronal subregions of the hippocampal formation.58 A potential role of CTNNA2 in development of several neurodegenerative diseases has been proposed. CTNNA2 was suggested to be a susceptibility gene for schizophrenia59-61 and it was shown that smoking influences on differential regulation/expression of CTNNA2 in patients with schizophrenia.62 GWAS and linkage studies identified CTNNA2 as a novel risk gene for adult ADHD and addiction vulnerability63 and late-onset Alzheimer’s disease.64 A GWAS of excitement-seeking personality trait also identified CTNNA2 as a risk gene suggesting its role in hyperactivity, substance use, antisocial and bipolar disorders.65 A clinical manifestation of a deletion encompassing CTNNA2 gene includes mental retardation, speech delay and gait abnormalities.66 A genome-wide epigenetic study showed age-related DNA methylation changes of the CTNNA2 gene.67 This gene was also suggested to contribute to gene expression variability in humans.68 OR7E156P is an olfactory receptor, family 7, subfamily E, member 156 pseudogene. CUL3 is part of E3 ubiquitin ligase complex and has a role in polyubiquitination and degradation of important regulatory proteins in all eukaryotes.69-71 It has been shown to have a role in endolysosomal trafficking pathway.69 Research in Drosophila provided clues that Cul3 is component of the Drosophila circadian clock72 and that it is important for setting the sleep homeostat and a dopaminergic arousal pathway.73 Cul3 ubiquitin ligase complex was also shown to mediate neuronal differentiation in zebrafish74 and to play critical role in many developmental processes in all metazoan animals through Hedgehog (Hh) signalling.75 The role of this gene in etiology of several diseases has been suggested. The expression of Cul3 in mice is protective against formation of progenitor cells that may transform into tumor-initiating cells.76 Breast cancer progression has been shown to be regulated by a novel mechanism that includes Cul3- SPOP complex.77 Mutations within CUL3 gene cause hypertension and electrolyte abnormalities, as shown by exome sequencing of patients with pseudohypoaldosteronism type II.78 FAM124B was suggested to be involved in the pathogenesis of CHARGE syndrome (an autosomal dominant multiple malformation disorder) and neurodevelopmental disorders.79 It was also shown that DNA methylation state of FAM124B is affected by estrogen and progesterone receptor status in breast cancer.80 ZNF833P is a zinc finger protein 833, pseudogene non-coding RNA. COL4A5 encodes alpha-5 subunit of type IV collagen.81 It is associated with X-linked Alport syndrome, characterized by progressive nephritis, hearing loss, and ocular changes.82-86 Protein interactions between Alzheimer's disease beta A4 precursor protein and collagen type IV have been observed.87 COL4A5 was suggested to be a candidate gene in the development of </p><p>10 subungual exostosis.88 The expression of COL4A5/COL4A6 was shown to be down-regulated in colorectal cancer due to hypermethylation of their promoter region.89 CNTNAP2 gene encodes contactin-associated protein-like 2 which is a member of the neurexin superfamily of proteins that are involved in cell to cell interactions in the nervous system90,91 This is one of the largest genes in the human genome; it encompasses 1.5% of chromosome 7.91 CNTNAP2 gene has been widely investigated and associated with multiple neurodevelopmental disorders and psychiatric traits, especially with autism spectrum disorders. Other traits include obsessive-compulsive disorder and Gilles de la Tourette syndrome99 developmental language disorders,100-102 focal epilepsy,103 epilepsy, schizophrenia, bipolar disorder,108 Pitt-Hopkins-like mental retardation,109 Morvan syndrome,110 major dimensions of personality,111 reading-related measures, selective mutism and social anxiety-related traits,114 adolescent idiopathic scoliosis,115 pseudoexfoliation syndrome.116 CNTNAP2 gene is found to be downregulated by STOX1A in the hippocampus of Alzheimer's disease patients.117 The involvement of this gene in the regulation of diet-induced obesity in mice has also been observed.118 Aberrant methylation of CpG islands within promoter of CNTNAP2 gene was found in pancreatic cancer cells.119 Expression study detected expression of this gene in mouse brain including the limbic system, which is considered to be involved in social behaviour, and in all cortical layers of the temporal lobe of the human brain. Mice KO of the Cntnap2 gene are characterised by behavior similar to autism spectrum disorders, these mice have reduced number of interneurons and display abnormalities with neuronal migration and neuronal network activity.121 MRI scan of human brains of healthy carriers of this gene showed altered structural brain connectivity.122 ATP2B1 gene encodes the plasma membrane calcium ATPase isoform 1 and plays a role in regulation of cytoplasmic calcium. ATP2B1 (also known as PMCA1) has a distinct distribution in the rat brain in that it is expressed at high levels in the forebrain and in CA1 region of hippocampus, followed by the midbrain, but has lower expression in the cerebellum, suggesting an independent regulation of Ca2+ homeostasis in different neurons.123 Genetic variants mapped in or near the ATP2B1 gene were found to be associated with blood pressure and hypertension in people of European and East Asian origin,124 coronary artery disease in Han Chinese,125 and serum magnesium levels and hypomagnesemia.126 This gene showed reduced expression in tissues taken by endoscopic pinch biopsies from Ulcerative colitis patients127 and in primary oral squamous cell carcinomas.128</p><p>11 Supplemental References</p><p>Supplemental Tables</p><p>12</p>
Details
-
File Typepdf
-
Upload Time-
-
Content LanguagesEnglish
-
Upload UserAnonymous/Not logged-in
-
File Pages12 Page
-
File Size-