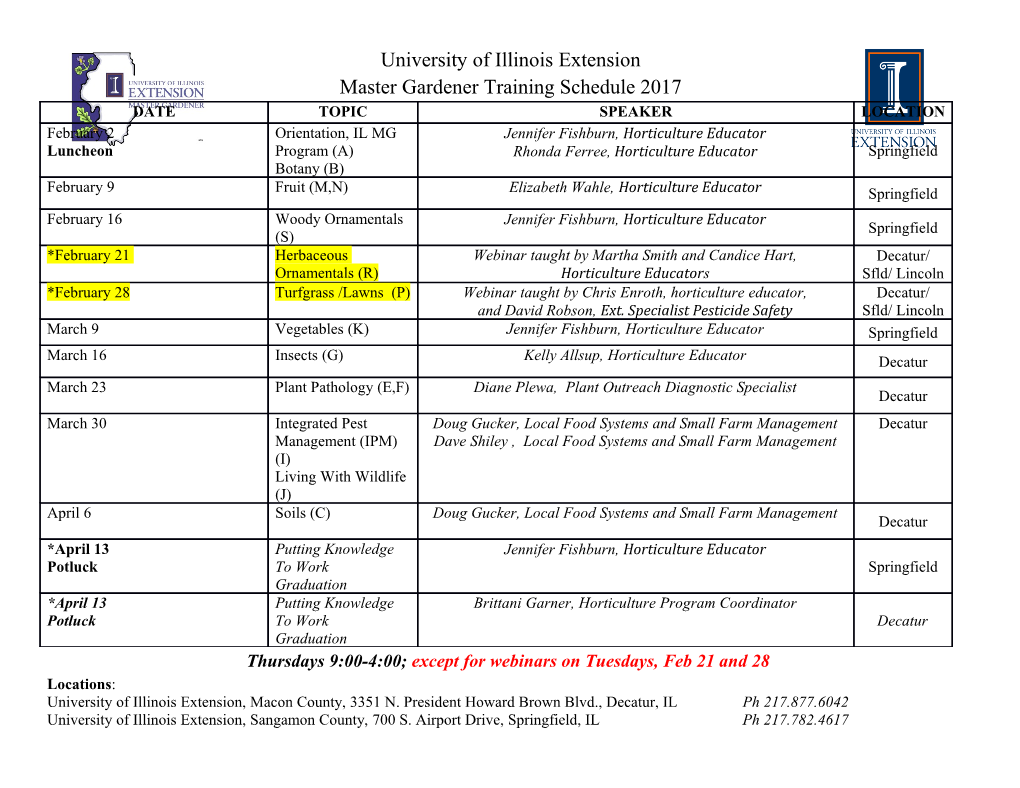
<p> Statistics Analytical Procedures (STT Consulting) April 2010</p><p>Statistical aspects in the development of analytical procedures for endogeneous serum components</p><p>Example Serum-testosterone in males</p><p>STT Consulting</p><p>April 2010</p><p> [email protected] www.stt-consulting.com</p><p>1 Statistics Analytical Procedures (STT Consulting) April 2010</p><p>Content</p><p>Which quality is desirable?</p><p>How can we proof quality?</p><p>How can we control quality?</p><p>Summary</p><p>2 Statistics Analytical Procedures (STT Consulting) April 2010</p><p>Which quality is desirable?</p><p>Physiological facts (male serum-testosterone) Reference interval (males) ~9–32 nmol/L Distribution ~log-normal; simplification for this document: assume Normal distribution Reference intervals for serum testosterone, SHBG, LH and FSH in males from the NORIP project. Bjerner J et al. Scand J Clin Lab Invest 2009;69:873-9. Within-subject biological variation (CVw) 9.3% (www.westgard.com/biodatabase1.htm).</p><p>Reference interval (statistical rehearsal) Reference interval = central 95 % of the distribution of healthy individuals; ranges from 2.5th percentile to 97.5th percentile; for normally distributed data = mean ± 1.96 x CVg (group variation) Male testosterone (simplified): Mean = 20.5 nmol/L; SDg = (32 – 9)/(2*1.96) = 5.87 nmol/L; CVg = 28.6%</p><p>1st experiment: true reference interval versus measured reference interval</p><p>Testosterone (true distribution) Testosterone (measured distribution) 0 0 5 5 1 1 0 0 y y 0 0 c c 1 1 n n e e u u q q e e r r 0 0 F F 5 5 0 0</p><p>0 10 20 30 40 0 10 20 30 40</p><p> nmol/L nmol/L</p><p>The figure on the left shows a hypothetical “true” distribution of 500 male testosterone values generated with the above mean and SDg. The figure on the right shows a measured distribution, with an analytical procedure that has a CVa of 15%# (= 3.075 nmol/L at 20.5 nmol/L; SDtot = SQRT[5.87^2+3.075^2] = 6.62 nmol/L). Note, the measured distribution is slightly broader than the true distribution (due to the fact that SDtot = 6.62 nmol/L). #”Generic” maximum CV for bioanalytical methods. Guidance for Industry: Bioanalytical Method Validation, US Department of Health andHumanServices, FDA, Center for Biologics Evaluation and Research (CBER), Rockville, MD, 2001.</p><p>3 Statistics Analytical Procedures (STT Consulting) April 2010</p><p>Observation Measurement may distort the “true” distribution of our data! The essential question arising from that is, how much distortion can we accept?</p><p>2nd experiment: distribution over time in an individual; true versus measured We take an individual with an average testosterone level = 20.5 nmol/L. Remember, CVw is 9.3% (SDw = 1.91 nmol/L). We simulate and measure the testosterone level 500 times, again with CVa = 15% (SDtot = 3.62 nmol/L).</p><p>Testosterone (true distribution) Testosterone (measured distribution) 0 0 3 3 ) ) L L / / l l 5 5 o o 2 2 m m n n ( (</p><p> e e 0 0 n n 2 2 o o r r e e t t s s o o 5 5 t t 1 1 s s e e T T 0 0 1 1</p><p>0 100 200 300 400 500 0 100 200 300 400 500</p><p>Number Number</p><p>We recognize that the measured distribution is much broader than the “true” distribution!</p><p>Axiom 1 Measurement should distort our “true” data only to an “acceptable” extent. In the in-vitro- diagnostic field, one convention is to keep the distortion less than 12%. By the variance propagation rule we obtain CVa ≤ ½ CVb (CVtot = SQRT[1^2+0.5^2] = 1.12 CVb). Note, CVb denotes any biological variation: within, group, other).</p><p>CVa ≤ ½ CVb</p><p>CVa and data distortion 2 . 2 8 . 1 t o t V C 4 . 1 0 . 1 The figure shows CVtot depends on the ratio 0.0 0.5 1.0 1.5 2.0 CVa/CVb. Ratio CVa/CVb</p><p>4 Statistics Analytical Procedures (STT Consulting) April 2010</p><p>3rd Experiment: monitoring, CVa = ½ CVw, & bias versus limits mean ± 1.96 * CVw True mean of monitored individual = 20.5 nmol/L SDtot = 2.13 nmol/L = SQRT[1.91^2+0.953^2] Limit = 1.96 * 1.91 nmol/L = 3.74 nmol/L. Lower and upper limits (red lines) = 16.76 and 24.24 nmol/L; for CVa = 0, we expect 5% of the values outside both limits (= in total 25). We investigate a situation with bias = 0 (left) and bias = +2.13 nmol/L ~10% (right)</p><p>Biology and CVa = 0.5 CVw Biology and CVa = 0.5 CVw and bias 0 0 3 3 ) ) L L / / l l o o 5 5 2 2 m m n n ( (</p><p> e e 0 0 n n 2 2 o o r r e e t t s s 5 5 o o t t 1 1 s s e e T T 0 0 1 1</p><p>0 100 200 300 400 500 0 100 200 300 400 500</p><p>Number Number</p><p>Left: With CVa = ½ CVw and bias = 0, we see somewhat more than 25 results out the red lines; that is because of the “12% compromise” we made. Right: We see that the bias of 10% moves a lot of the results outside the limits, but 1-sided (>24.7 nmol/L). So, which bias can we accept?</p><p>Axiom 2 In the in-vitro-diagnostic field, one convention is to keep the Bias ≤ ⅓ CVw in the monitoring situation#. In that situation, strictly, we do not have to worry about a bias, but over a “change in systematic error” = SE, therefore: SE ≤ ⅓ CVw. #Hyltoft Petersen P, Fraser CG, Westgard JO, Lytken Larsen M. Analytical goal-setting for monitoring patients when two analytical methods are used. Clin Chem 1992;38:2256-60.</p><p>SE ≤ ⅓ CVw</p><p>This leads us to quality specifications for CVa, SE (Bias), and TEa.</p><p>5 Statistics Analytical Procedures (STT Consulting) April 2010</p><p>Quality specifications, simple model for monitoring, male testosterone CVa ≤ ½ CVw = 4.7% (= ½ 9.3%)</p><p>SE ≤ ⅓ CVw = 3.1% (= ⅓ 9.3%)</p><p>TEa = SE + 1.645 * CVa = 11% (1.645: 1-sided out, see above)</p><p>Note: the exact model is more complicated because the values for CVa and se are valid only under the assumption that the respective other is 0 (CVa = ½ CVw, when SE = 0). See also www.stt-consulting.com >Education >Analytical Quality II.</p><p>4th Experiment: monitoring, CVa = ½ CVw & SE = ⅓ CVw versus mean ± 1.96 * CVw True mean = 21.14 nmol/L (=20.5 + 0.64, 3.1% “bias”) SDtot = 2.13 nmol/L (propagated variance of CVw and CVa) Lower and upper limits (red lines) = 16.76 and 24.24 nmol/L</p><p>Biology and CVa = 1/2 CVw and bias = 1/3 CVw 0 3 ) L / l o 5 2 m n (</p><p> e 0 n 2 o r e t s 5 o t 1 s e T 0 1</p><p>0 100 200 300 400 500</p><p>Number</p><p>We see somewhat more than expected (~13) values outside the upper limit. This is because we made some compromise for CVa and SE.</p><p>6 Statistics Analytical Procedures (STT Consulting) April 2010</p><p>Pure analytical error Naturally, when we measure the same sample repeatedly (for example, a quality control sample), we deal with analytical error, only.</p><p>5th Experiment: repeated measurement of same sample, CVa = ½ CVw & SE = ⅓ CVw versus mean ± TEa True average = 20.5 nmol/L</p><p>Exp. Average = 21.14 nmol/L (true + SE) CVa ≤ ½ CVw = 4.7% (= 0.953 nmol/L)</p><p>SE ≤ ⅓ CVw = 3.1% (= 0.64 nmol/L) TEa = 11% (= 2.26 nmol/L; LL = 18.24, UL = 22.76) Left: same scale as before Right: new scale</p><p>Measurement CVa = 1/2 CVw & bias = 1/3 CVw Measurement CVa = 1/2 CVw & bias = 1/3 CVw 0 3 4 ) ) 2 L L / / l l o o 5 2 2 m m 2 n n ( (</p><p> e e 0 0 n n 2 2 o o r r e e t t 8 s s 1 5 o o t t 1 s s e e 6 T T 1 0 1</p><p>0 100 200 300 400 500 0 100 200 300 400 500</p><p>Number Number</p><p>We see ~25 results outside the TEa limit; most of them >22.76 nmol/L. This corresponds to the construction of the TEa = SE + 1.645 * CVa.</p><p>Conclusion</p><p>We arrived at numbers for analytical quality (CVa, SE, TEa) based on the natural variation of serum testosterone. Now we have to develop a procedure with that quality.</p><p>Next question How can we demonstrate (proof) the quality of an analytical procedure?</p><p>Answer By method validation.</p><p>7 Statistics Analytical Procedures (STT Consulting) April 2010</p><p>How can we proof quality?</p><p>The method validation experiment Demonstrate CVa ≤ 4.7% Bias ≤ 3.1% TEa ≤ 11% The constraint: the number of measurements we perform for method validation. See also: www.stt-consulting.com >Statistics >Method Validation Accuracy (EXCEL).</p><p>6th experiment: repeat experiment 5 several times, with n = 20 (bias -3.1%: 19.86)</p><p>Validation (n = 20) Future 1 Future 2 4 4 4 ) ) ) 2 2 2 L L L / / / l l l o o o 2 2 2 m m m 2 2 2 n n n ( ( (</p><p> e e e 0 0 0 n n n 2 2 2 o o o r r r e e e t t t 8 8 8 s s s 1 1 1 o o o t t t s s s e e e 6 6 6 T T T 1 1 1</p><p>5 10 15 20 5 10 15 20 5 10 15 20</p><p>Number Number Number</p><p>Future 3 Future 4 Future 5 4 4 4 ) ) ) 2 2 2 L L L / / / l l l o o o 2 2 2 m m m 2 2 2 n n n ( ( (</p><p> e e e 0 0 0 n n n 2 2 2 o o o r r r e e e t t t 8 8 8 s s s 1 1 1 o o o t t t s s s e e e 6 6 6 T T T 1 1 1</p><p>5 10 15 20 5 10 15 20 5 10 15 20</p><p>Number Number Number</p><p>While in the validation experiment all results are nicely within TEa, we observe that future data may be outside TEa. The question arises: how sure are we about our estimates of CVa, Bias, and TEa when we perform 20 measurements, only?</p><p>How sure are we about our estimates? Calculate confidence intervals! >Statistics</p><p>How sure are we that our estimates are within our specifications? Perform statistical tests! >Statistics</p><p>How can we guarantee that a validation will be successful in, say 90% of the cases? Perform power analysis! >Statistics</p><p>8 Statistics Analytical Procedures (STT Consulting) April 2010</p><p>Statistics, the inevitable!</p><p>Imprecision (SDa, CVa) Note: strictly, the following is valid only for SD and not for CV. We will continue with SD, therefore! n = 20 SDa = 0.953 nmol/L (= limit SDa) Statistics: 1-sided, = 0.05</p><p>Confidence interval (from lower to upper confidence limit: LCL, UCL) (www.stt-consulting.com >Statistics >CI-calculator) LCL = 0.757 nmol/L; UCL = 1.306 nmol/L (asymmetric limits!) Observation: the “true” SDa could be quite bigger!</p><p>1-sample F -test (or Chi2-test) (www.stt-consulting.com >Statistics >Tests with estimates) “Test” SD = 0.953 nmol/L (= maximum) P = 0.54 “FAIL” (P should be <0.05) Observation: we fail the test! How small should SDa be to PASS? SDa should be = 0.695 nmol/L (try & error) (Note, UCL of 0.695 = 0.952 nmol/L) This corresponds to a “confidence” CVa = 3.4%</p><p>Power of the 1-sample F -test If we actually have SDa = 0.695 nmol/L, how often would we validate our method? G*Power software (http://www.psycho.uni-duesseldorf.de/aap/projects/gpower/) CAVE: you need the variance ratio (Var1/Var0): 0.695^2/0.953^2 = 0.532 Power = 0.544; we would pass the validation in 54%, only! How small should SDa be to pass in 90%? Calculate effect size, but NOTE that the program expects Var1 to be larger; therefore, we must do it iteratively. Ratio (Var1/Var0) = 0.3715; Var1 = 0.3715*0.908 = 0.337 >SDa = 0.581 nmol/L This corresponds to a “power” CVa = 2.8%</p><p>Conclusion When the method works with the limit CVa, the method will not be validated with reasonable n. The “confidence” CVa is not sufficient for a 90% validation chance, however, the “power” CVa is. Note, “power” CVa <“confidence” CVa <“limit” CVa!</p><p>9 Statistics Analytical Procedures (STT Consulting) April 2010</p><p>Trueness (Bias) n = 20 Mean = 21.14 nmol/L (“true” mean = 20.5 nmol/L) Bias = 0.64 nmol/L (= limit Bias) SDa = 0.581 nmol/L (= “Power” SDa) Statistics: 1-sided, = 0.05</p><p>Confidence interval (www.stt-consulting.com >Statistics >CI-calculator) LCL = 20.92 nmol/L; UCL = 21.36 nmol/L (CI = ± 0.225 nmol/L; see also below) Observation: the estimate seems very reliable!</p><p>1-sample t-test (www.stt-consulting.com >Statistics >Tests with estimates) Test level = 21.14 nmol/L (= Bias limit, 20.5 + 3.1%) P = 0.5“ FAIL” (P should be <0.05) Observation: we fail the test! How small should the bias be to PASS? Test level = 21.14, Mean = 20.9155, Difference = 0.2245 nmol/L (= CI) The bias should be <0.4155 nmol/L (by try and error) (20.9155 – 20.5) This corresponds to a “confidence” bias of ~2%.</p><p>Power of the 1-sample t-test If we actually have a Bias of 0.4155 nmol/L, how often would we validate our method? G*Power software (http://www.psycho.uni-duesseldorf.de/aap/projects/gpower/) Mean H0 = 21.14, Mean H1 = 20.9155, SD = 0.581 >Effect size = 0.3864 Power = 0.508; we would pass the validation in 51%, only! How small should Bias be to pass in 90%? Calculate effect size (= Difference/SD) = 0.679 >Difference = 0.679*0.581 = 0.3945 nmol/L The experimental average should be <21.14 – 0.3945 < 20.746 nmol/L The bias should be <0.246 nmol/L (= 20.746 – 20.5 = 0.246 nmol/L). This corresponds to a “power” Bias of 1.2%.</p><p>Conclusion Even with the “power” CVa, we can allow a Bias of 1.2%, only, to get the method validated in 90% of the cases. Note, however, the bias we can validate depends heavily on the starting bias; also, we can validate a higher bias when we reduce SDa beyond the “power” SDa.</p><p>10 Statistics Analytical Procedures (STT Consulting) April 2010</p><p>Total error n = 20 Mean = 20.5 nmol/L SDa = 0.953 nmol/L</p><p>Confidence interval The confidence interval for total error can be viewed as confidence interval of a percentile, for example, the 1-sided 95% percentile, z = 1.645 (www.stt-consulting.com >Education >Statistical Intervals). This is the same as the tolerance interval, for which factors are tabulated, or can be calculated by the use of the R-software (see same link). I choose the 1- sided factor for 95% of the population included with 95% confidence = 2.40, for n = 20.</p><p>7th experiment: simulation mean = 20.5, SDa = 0.953, n =20 Figure with z-limits 20.5 ± 1.645*0.953 (±1.568) = 18.93 & 22.07 nmol/L (blue) & tolerance- limits 20.5 ± 2.4*0.953 (±2.29) = 18.21 & 22.79 nmol/L (red).</p><p>Tolerance interval Tolerance interval Tolerance interval ) ) ) 4 4 4 L L L / / / 2 2 2 l l l o o o m m m 2 2 2 n n n 2 2 2 ( ( (</p><p> e e e 0 0 0 n n n 2 2 2 o o o r r r e e e t t t 8 8 8 s s s 1 1 1 o o o t t t s s s 6 6 6 e e e 1 1 1 T T T</p><p>5 10 15 20 5 10 15 20 5 10 15 20</p><p>Number Number Number</p><p>Because we are not sure about our estimates of mean and SDa with n = 20, we are uncertain about the future distribution of the great majority (e.g., 95%) of our data. We have to account for that by expanding z = 1.645 to k = 2.40. On the other hand, by knowing the tolerance interval with n = 20, we could account for that by reducing SDa accordingly: SDa =(1.645/2.40)*0.953 = 0.653 nmol/L. “Confidence” tolerance SDa = 0.653 nmol/L (= 3.2%).</p><p>Significance testing I am not aware of a significance test for a tolerance interval. However, we have seen that the confidence interval corresponds to the significance test in case of the null-hypothesis.</p><p>Power I am not aware of power analysis for tolerance intervals. However, according to MY OPINION, we can approximate the power by simulation.</p><p>11 Statistics Analytical Procedures (STT Consulting) April 2010</p><p>Power (1-sided tolerance interval) Remark: the following is MY OPINION!</p><p>Simulation of the distribution of the z = 1.645 centile The following R-script simulates the t-value for the distribution of the 1-sided 95% centile. See also: “Chakraborti S, Li J. Confidence interval estimation of a normal percentile. Amer Statistician 2007;61:331-6” n=20 loop=500000 T1=c(rep(0,loop)) for(i in 1:loop) { x=rnorm(n, mean = 0, sd = 1) xstd=sqrt(var(x)) xbar=mean(x) T1[i]=(1.64485-xbar-1.64485*1.01324*xstd)/(0.34944*xstd) } quantile(T1,probs=c(0.1,0.5,0.95))</p><p>Notes: C is a correction factor for s (1.01324, for n = 20), because s is a biased estimator; 0.34994 is a factor that accounts for the rest of the constants in the denominator of the above equation. </p><p>The quantile at 0.95 (= 2.086) gives us the critical t-value. When we substitute a in the equation at the right with this critical t-value, we will end up with the factor 2.4 for s. When we move the distribution by -0.746 (= 1.645 – 2.40), we expect ~50% of the t-values >2.086, the critical t-value (simulation: 54%). Now we have to find the distance that 90% of the t-values are >2.086, which would give us 90% power for the test. We find, by iteration, that this distance is ~1.31; the “power”-centile would thus be 1.645 + 1.31 = 2.96. We could account for that by reducing SDa accordingly: SDa =(1.645/2.96)*0.953 = 0.53 nmol/L. “Power” tolerance SDa = 0.53 nmol/L (= 2.6%).</p><p>12 Statistics Analytical Procedures (STT Consulting) April 2010</p><p>Comparison between desirable quality and demonstrable quality (n = 20) Desirable “Confidence” Power CVa (%) ≤ 4.7 3.4 2.8 Bias (%) ≤ 3.1 2.0 1.2 Dist. to limit# 0 1.1 1.9 TEa calculated with CVa and bias TEa (%) ≤ 11 7.6 5.8 #Note, the reduction of CVa due to confidence and power calculations can be generalized; the reduction for the bias cannot, it depends on the starting point. Therefore, we look at the “distance to the limit”. In the desirable case, we can choose a distance of 0%, in the confidence case it is 1.1%, and in the power-case it is 2.2%. When we would start with a bias = CVa, the numbers for bias would be 4.7, 3.6, and 2.8. Also, we can validate a higher bias when we reduce SDa beyond the “power” SDa.</p><p>TEa from tolerance interval Desirable “Confidence” “Power” CVa (%) ≤ 4.7 3.2 2.6 TEa (%) ≤ 11 6.4$ 5.1$ $NO bias, calculated as 1.96*SDa; we do not add bias here because the tolerance interval accounts for unknown SDa and bias.</p><p>Conclusion We cannot develop a method with “limit” performance because we will not be able to validate it with a reasonable number of measurements. We have to “keep away” from the limits, maybe we should consume ½ or ⅔ of them?</p><p>“Keep away” from the limits Let us investigate that from onother point. We know that analytical methods should be controlled during operation by statistical process control (SPC) or internal quality control (IQC). IQC should alarm us when the method deteriorates to an undesirable extent. So, we move away from our stable situation (“the null-hypothesis”) to increased values for SDa or bias (“alternative hypothesis”). IQC should tell us when we reach critical “alternative hypothesis conditions”. This means, we have to deal with power considerations. See www.stt-consulting.com “Power”.</p><p>13 Statistics Analytical Procedures (STT Consulting) April 2010</p><p>How can we control quality? Statistical Process Control (Internal Quality Control)</p><p>Assume We want to keep the TEa of our method ≤ 11%.</p><p>Can we do that with the “operating point” CVa (%) = 4.7 and Bias (%) = 3.1 (see before)?</p><p>Answer Use specialized software (www.westgard.com) Note: the following considerations are valid for the introduction of bias (drifts, shifts); increased random error requires different power analysis. </p><p>1.0 0.9 0.8 0.7 0.6 r e</p><p> w 0.5 o</p><p>P 0.4 0.3 0.2 0.1 0.0 0 2 4 6 8 Shift (k x SD)</p><p>AQA(SE) = analytical quality assurance (systematic error). The figure shows the TE line for the stable process (2 sigma performance), the TE line for the controlled process (here, with the 3s-rule), and the operating point (s = 4.7, bias = 3.1). Without going into detail, this figure says to us that we cannot control the process with the chosen operating point to achieve a TEa of 11%. The operating point should be left of the IQC line (here, the 3s line). Note, the figure is based on the power of the 3s rule for detecting bias.</p><p>Let us try the “power” values: CV = 2.8%, Bias = 1.2%.</p><p>14 Statistics Analytical Procedures (STT Consulting) April 2010</p><p>Assume TEa = 11% and “power values (n = 20)”: CV = 2.8%, Bias = 1.2%</p><p>This picture says we could control that process, but at a very high expense: with 4 control measurements and 18% false positives! </p><p>Only when we reduce CVa to 1.63%, we can control the process with a convenient rule (3s: very few false positives!) and with sufficient power (90%).</p><p>Conclusion Again, “keep away from the limits”. Ideally, we would like to have a 6 process: = (TEa – Bias)/CVa = (11 – 1.2)/1.63 = 6 (1.63 = example above).</p><p>15 Statistics Analytical Procedures (STT Consulting) April 2010</p><p>Summary Establish objective quality specifications. Keep away from the specifications; to assure that you work within specifications, you have to develop a method that is better, because of validation and quality control needs. The numbers you should strive for may be disappointingly low. In practice, you may have to make compromises. “You can’t always get what you want” (The Rolling Stones). Once developed and validated, put your procedure under statistical control!</p><p>In the end Unfortunately, you may be the only one in your surrounding that understands the logic! Then, look what everybody else does in your field, compare it with what you have learned and do it better, if necessary!</p><p>16</p>
Details
-
File Typepdf
-
Upload Time-
-
Content LanguagesEnglish
-
Upload UserAnonymous/Not logged-in
-
File Pages16 Page
-
File Size-