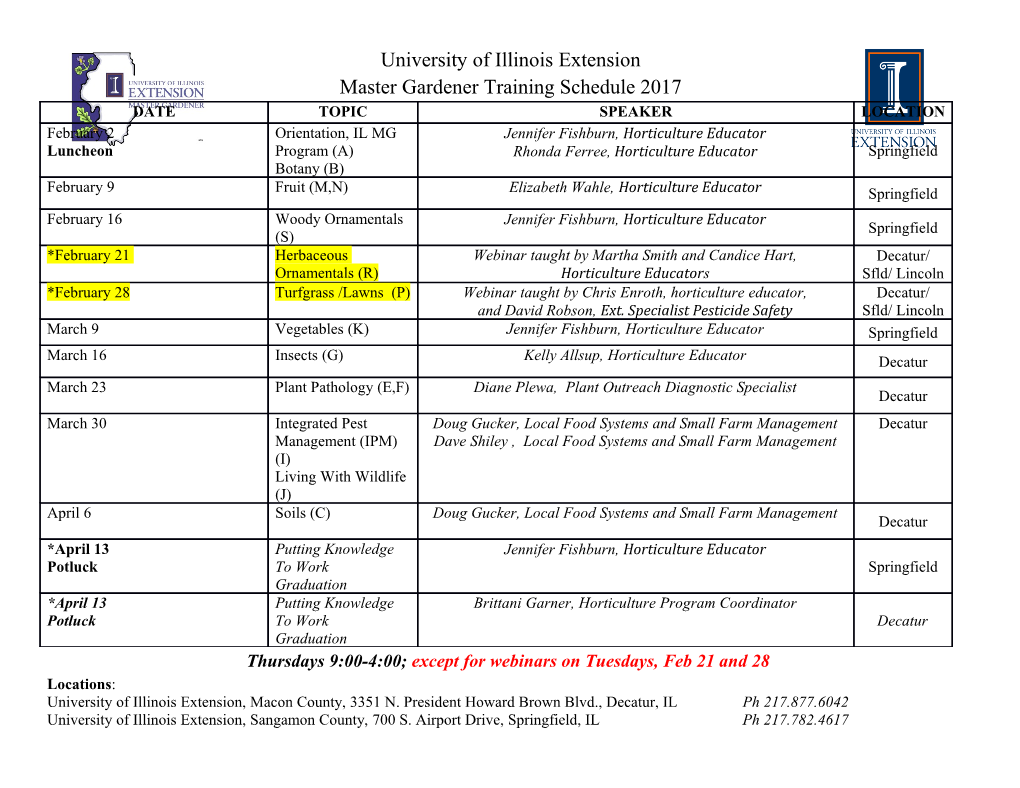
<p> IT233: Applied Statistics TIHE 2005 </p><p>Sampling Distributions</p><p>Random Sample:</p><p>A random sample is one in which each unit of the population has an equal chance of being selected in the sample.</p><p> x, x ,..., x A set of observations ( 1 2 n ) constitutes a random sample if the following are true.</p><p>1. Each observation has been selected at random from the same population.</p><p>2. The observations are independent (i.e. any selection is not influenced by the previous selections).</p><p> x, x ,..., x NOTES: To ensure that 1 2 n are indeed independent and identically distributed we follow the following notes:</p><p>1. To obtain a random sample we should be sampling from an infinite population.</p><p>2. If we are sampling from a finite population, the selection should be done with replacement. </p><p>Sample Statistic:</p><p>Any quantity that computed from a random sample is known as statistic.</p><p>Examples: Common statistics are: x 1. The sample mean: x = i n 2 ( x- x ) 2. The sample variance: S2 = i n -1</p><p>3. The sample median: x</p><p>Q, Q 4. Sample quartiles: 1 3</p><p>5. Sample range: Largest – Smallest</p><p> x - m m NOTE: The r. v. ( i ) is not a statistic. Since is unknown, we cannot determine its numerical values from a random sample.</p><p>Sampling Distribution:</p><p>Since a sample statistic is computed from a random sample, it follows that the sample statistic itself is random variable. Every random variable has a probability distribution. This distribution of a sample statistic is called its sampling distribution.</p><p>Definition: The probability distribution of a sample statistic is called a sampling distribution.</p><p>Some Sampling Distributions</p><p> Sampling distribution of x x- x Sampling distribution of the difference of two means ( 1 2 ) Sampling distribution of S2 S 2 1 Sampling distribution of the ratio of two sample variances – 2 S2 Sampling Distribution of x :</p><p>If a random sample of n observations is taken from a normal population with mean m and variance s 2 , then </p><p> m= m x</p><p> s 2 s 2 = x n</p><p> and its standard normal value z follows standard normal distribution i.e.</p><p> x - m z= : N(0,1) s n</p><p>NOTE: If the sample is drawn from unknown distribution, either finite or infinite, the distribution of x will still be approximately normal provided n is large (n >30), which is given by the following theorem:</p><p>Central Limit Theorem: If x is the mean of a random sample of size n taken from a population with mean m and variance s 2 , then</p><p> x - m z= : N(0,1) s n as n .</p><p>Applications: The sampling distribution of x could be used:</p><p> To find the probabilities concerning x To find the confidence interval for m H : m= m To test the hypothesis 0 0</p><p>Example: A random variable X has the following distribution: x 4 5 6 7 p( x )= P( X= x ) 0.2 0.4 0.3 0.1 (a) Find m and s 2 of X . m 2 (b) Find the mean x and variance s x for sample size 36. (c) Find the probability that sample mean x will be less than 5.5.</p><p>Solution:</p><p>(a) Mean m</p><p> m =E( X ) = x p i i i = 5.3</p><p>Variance s 2</p><p>2 2 E( X ) = xi p i = 28.9 i</p><p>\ 2 2 2 s =E( X ) - 臌轾 E ( X ) = 28.9 – 28.09 = 0.81</p><p> m= m (b) x = 5.3</p><p> s 2 0.81 s 2 = = = 0.0225 x n 36</p><p>(c) The distribution of X is not normal but n >30. Hence, we can take the distribution of X to be normal.</p><p>骣 5.5- 5.3 = 0.9082 \ P( X< 5.5) = P琪 Z < 桫 0.0225</p><p>= P( Z < 1.33) 0 1.33 z</p>
Details
-
File Typepdf
-
Upload Time-
-
Content LanguagesEnglish
-
Upload UserAnonymous/Not logged-in
-
File Pages4 Page
-
File Size-