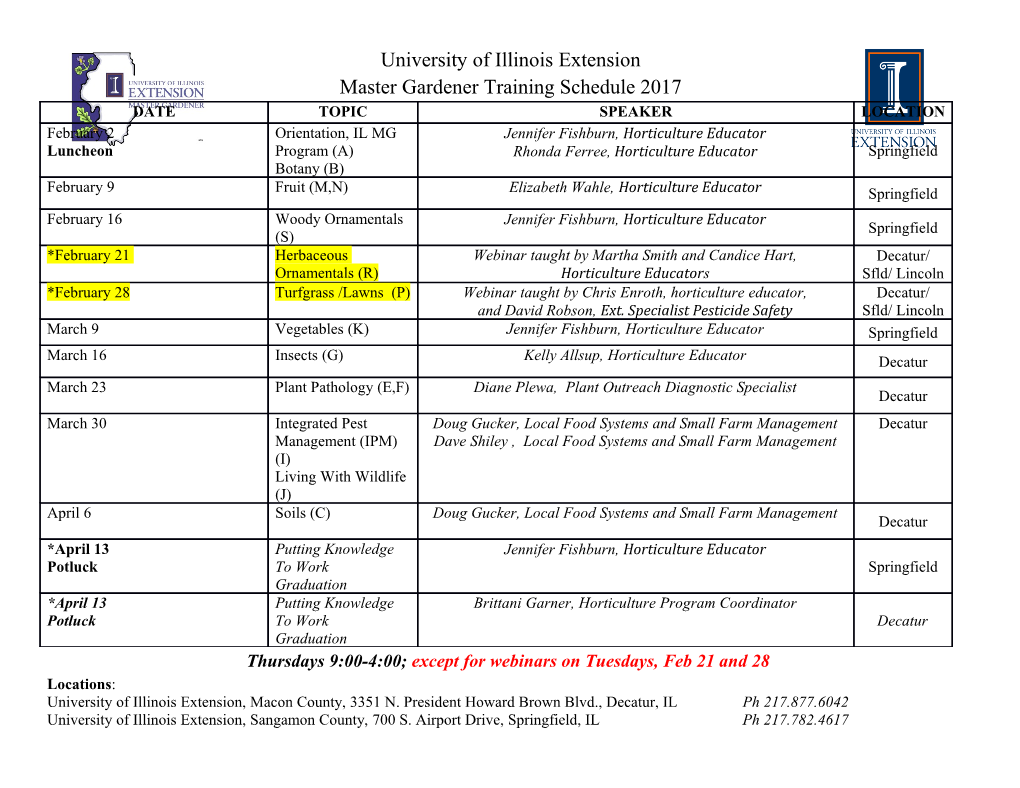
<p>A GIS Framework to Forecast Residential Home Prices</p><p>Submitted by</p><p>Mak Kaboudan & Avijit Sarkar School of Business, University of Redlands, Redlands, CA 92399, USA</p><p>Corresponding author: Mak Kaboudan e-mail: [email protected] Tel: (909) 748-8772; fax: (909) 335-5125;</p><p>November 20, 2006 A GIS Framework to Forecast Residential Home Prices</p><p>Mak Kaboudan, Avijit Sarkar School of Business, University of Redlands, Redlands, CA 92399, USA</p><p>Abstract. In this paper we estimate spatiotemporal models of average neighborhood single family home prices to use in predicting individual property prices. Average home- price variations are explained in terms of differences in average neighborhood house attributes, spatial attributes, and temporal economic changes. Models adopting three different neighborhood resolution definitions are estimated using quarterly panel data over the period 2000-2005 in four cities from four different counties in Southern California. Our results suggest that forecasts obtained using city neighborhood average price equations have advantage over forecasts obtained using equations estimated from city disaggregated data. </p><p>Keywords: Spatiotemporal models; models with panel data; estimating microeconomic data. JEL classification: C21; C23; C81</p><p>1. Introduction</p><p>This paper introduces a new way of modeling residential home prices that may help produce more accurate and timely forecasts of them. Accurate and timely forecasts of home prices clearly help home owners, developers, financial institutions, and government agencies make better decisions. For many decades, hedonic price models demonstrated that the price of a house is mainly dependent on its attributes. Those attributes typically included house characteristics such as building square footage, number of bedrooms, </p><p>2 number of bathrooms, age of the house, lot square footage, etc. Ball (1973) provides a review of the early literature. Recent use of geographic information systems (GIS) helped add spatial attributes such as distance to schools, distance to parks, distance to city business center, neighborhood ethnic mix, neighborhood median family income, etc. when modeling home prices. Can (1998) provides a spatial analytical framework to use when accounting for neighborhood effects. Including spatial variables along with housing attributes in specifications of hedonic models clearly adds a new dimension of complication to the statistical estimation of home price models. Harveston and </p><p>Pollakowski (1981) addressed concerns about the functional form to use in their estimation. The complication is mainly due to spatial autocorrelation. Like temporal autocorrelation, spatial autocorrelation reduces the efficacy of forecasts obtained when using standard statistical modeling techniques. Prior work that addressed spatial dependence either considered geographical coordinates as explanatory variables in the price model (Clapp, 2003) or modeled the regression residuals spatially (Basu and Thibodeau, </p><p>1998). Most existing models – both strictly hedonic or those that address spatial dependence </p><p>– focus on parsimony of estimated equations and produce out-of-sample predictions of prices for homes sold during the same time period. The sample to forecast and measure model efficacy typically consists of a withheld percent of the sample of data available to conduct the research. This means that the time dimension is absent in those models and as a result the forecasts are less useful when making future decisions since prices of homes change over time.</p><p>The method of modeling residential home prices proposed in this paper aims to move a step closer to producing parsimonious home price models that take into consideration </p><p>3 housing attributes, spatial attributes, and temporal economic changes. Temporal economic changes (especially changes in mortgage rates) have had evident significant effect on real (or inflation adjusted) home prices. Therefore statistical estimation efforts should deal with aggravated statistical problems of spatial autocorrelation (between spatial attributes) and of estimating panel (or cross-sectional-time-series) models. No attempt is made in this paper to introduce new methodology to resolve any of the two problems. Logical manipulations are used to circumvent spatial correlation; and existing methodology is used to resolve problems with estimating panel data. Logical manipulations mainly involve redefining the scope of the dependent variable and therefore the independent variables. Rather than estimating a model of individual property prices, a model that estimates average neighborhood home prices is considered instead. This logical manipulation is possible if it is assumed that homes in a specific neighborhood have similar attributes. </p><p>Modeling average neighborhood prices is new. Most studies focus on modeling individual home prices. If hedonic models explain variations in price levels “on average”, perhaps average home prices should be explained instead of individual home price levels.</p><p>Further, specifying and estimating average rather than individual home price functions is logical if spatial dependency between contiguous homes exists. Basu and Thibodeau </p><p>(1998) explain that spatial correlation is a likely phenomenon when dealing with individual home prices. The correlation is because nearby properties are probably constructed about the same time, share location attributes, and typically have similar structural features. Some studies focus on median prices as in Zhou (1997). While the </p><p>4 median price may be used instead of average price, the median price may fail to represent homes in its neighborhood accurately if that median-priced house happens to be atypical. </p><p>Modeling the average neighborhood price calculation may help “smooth out” the effects of unusual homes. To estimate the average neighborhood price model using panel data, existing methodology suggests use of a generalized least square method (GLS) (Pindyck and Rubinfeld, 1998). </p><p>With neighborhood average prices the dependent variable in equations to estimate, it is necessary to define what is meant by a “neighborhood”. Studies that focus on modeling hedonic submarkets of home prices provide suggestions that may help in defining neighborhoods. Goodman and Thibodeau (2003) use zip code districts, census tracts, and city market segmented by quality of public education to evaluate neighborhood effects on individual home prices. Bourassa et al. (2003) use geographical areas defined by real estate appraisers. Fletcher et al. (2000) found that there is no agreement in the literature on what is best when defining submarkets. Because our objective is to predict the average price of homes sold during a given time period in a neighborhood, it is only logical to define a neighborhood as one that has a statistically large enough number of contiguous sold houses that hopefully have similar attributes. Two existing definitions of submarkets may therefore be suitable to use when defining neighborhoods: census tracts and postal zip codes. While census tracts divide a city into submarkets for administrative purposes and while zip codes divide it for some postal delivery objective function, they provide clear definitions of what may be acceptable neighborhood size and boundaries. This study adds a novel definition of neighborhoods to the aforementioned. Neighborhoods are</p><p>5 also defined using the county assessor’s parcel numbers (APN). An APN is a nine digit id of land parcels assigned by the county when a parcel of land is subdivided (at least in several western US states). Contiguous subdivisions are assigned consecutive numbers. </p><p>For example, if a parcel of land that is 25 acres large gets subdivided into 50 potential home sites, the 50 new lots get new parcel numbers that relate to the original 25-acre lot number. To elaborate, assume that before the subdivision, the 25-acre parcel was assigned the APN 0300-100-00 at some time in the past. After subdivision, the new 50 lots are assigned new sequential numbers that would be something like: 0300-100-10, </p><p>0300-100-20, etc., which clearly relate to the original. If this is the case, using the APN’s first four digits of properties in a city (like 0300, 0301, etc.) provides a definition of neighborhoods that contain a fairly large number of contiguous houses. Selection of the number of digits to use is dependent on the size of the city. The objective when selecting such number is that the number of homes per neighborhood satisfies a minimum level imposed by statistical theoretical constraints. (Results provided later in this paper suggest choosing that number such that neighborhoods contain ten to 30 homes.) </p><p>Average neighborhood price models are different from standard hedonic price models and from models of housing submarkets. Average price models utilize a much smaller number of observations that hopefully smooth out of effects of unusual house attributes on estimated coefficients. Standard hedonic price models utilize a huge number of individual property observations regardless of the effects of unusual house attributes. </p><p>Hedonic models of submarkets estimate a different price equation for each market segment still using individual home prices. Each equation thus has a lower the number of </p><p>6 observations than standard hedonic models but requires estimating a larger number of equations; one for each submarket.</p><p>Adopting any of the three neighborhood definitions to obtain an average price of a home was never used before. It is not possible without a GIS framework to identify the neighborhoods clearly. This idea of modeling home prices assumes that each neighborhood has an imaginary average house that sells at a price that is determined by an average square footage, with an average number of bedroom, etc. Besides reducing spatial correlation problems, this averaging process provides a consistent definition of a neighborhood that can be easily reproduced. Variations in the average price may then be explained by the average home attributes, average spatial attributes, and temporal changes in mortgage rates and average median income. This paper explores the idea of modeling average neighborhood prices by applying it to four cities in Southern </p><p>California. Section 2 contains a description of the neighborhood resolutions for which the price equations are estimated. Section 3 and 4 introduce the data and methodology, respectively. Comparisons between standard hedonic model results of individual property prices and results from models of average neighborhood prices are in section 5. Section 6 has the conclusion.</p><p>2. Neighborhood Resolutions</p><p>Appropriate neighborhood resolutions are defined using a GIS framework that clusters houses possibly possessing similar attributes. The framework applies the three spatial resolutions: census tract (CT), assessor’s parcel number (PN), and by zip code (ZIP). CT </p><p>7 follows U.S. Census Bureau assigned numbers. All houses sold during a given quarter within a given census tract number belong to a neighborhood. Only the leftmost 4 digits of a PN in cities subject of this study define a neighborhood. ZIP+1 code (a subset of </p><p>ZIP+4) is the third resolution. ZIP+1 is used because using five-digit ZIP numbers produced only two neighborhoods for some cities. Addresses of homes sold over the study period (2000-2005) in four cities each in a different county in Southern California were geocoded in ArcGIS 9.1. Neighborhood polygons were delineated according to each of the three resolutions. CT, PN, and ZIP+1 neighborhoods in Burbank of Los Angeles </p><p>County are in Figure 1(a), (b), and (c), respectively. CT, PN, and ZIP+1 neighborhoods in Carlsbad of San Diego County are in Figure 2(a), (b), and (c); while those of Redlands of San Bernardino County and Riverside of Riverside County are in Figure 3 and 4, respectively. Given that CT was developed to satisfy city-management administrative objectives and that ZIP+1 was designed to satisfy the objective of maximizing efficiency of postal service, PN is expected to work best.</p><p>(a) CT Neighborhoods (b) PN Neighborhoods (c) ZIP+1 neighborhoods Figure 1. Resolutions of neighborhoods in Burbank of Los Angeles County</p><p>8 (a) CT Neighborhoods (b) PN Neighborhoods (c) ZIP+1 neighborhoods Figure 2. Resolutions of neighborhoods in Carlsbad of San Diego County</p><p>(a) CT Neighborhoods (b) PN Neighborhoods (c) ZIP+1 neighborhoods Figure 3. Resolutions of neighborhoods in Redlands of San Bernardino County</p><p>(a) CT Neighborhoods (b) PN Neighborhoods (c) ZIP+1 Neighborhoods Figure 4. Resolutions of neighborhoods in Riverside of Riverside County</p><p>9 3. The Data </p><p>A detailed data set containing individual sales and attributes of homes sold in the four selected counties in Southern California was obtained from DataQuick (2005). Not all cities in the four counties had consistent data and some had incomplete data. Complete data with consistent variables were identified for four cities Burbank (BB), Carlsbad </p><p>(CB), Redlands (RD), and Riverside (RS). Six years (2000-2005) of available data for the four cities are selected. Only six years are used because they cover a period of time with approximately consistent lending rules. It is a period when banks facilitated borrowing with new lending conditions such as interest only payments and other lending rules that led to historically relatively low down payments and low monthly mortgage payments. </p><p>The period (2000-2005) is thus selected to minimize structural changes in lending rules that may render inconsistent model estimation results. Data of the first five years (2000-</p><p>2004 inclusive) were used to fit different price models for each city. Data for 2005 would then be used to test the efficacy of one-year-ahead forecasts the models deliver. </p><p>Successful models then predict the unknown prices for 2006. </p><p>4. Methodology</p><p>Similar to standard hedonic individual price models, multiple regression methods apply when estimating average price models. The average neighborhood price is the dependent variable and the vector of attributes provides the set of independent variables. Because the data of average neighborhood prices and attributes is a combination of cross-sectional and time series observations or panel data, standard OLS multiple regression are not suitable as mentioned earlier. The method to use for panel data is the random-effects (or </p><p>10 error-components) model. Random-effects models are estimated as generalized least squares (GLS) regressions after estimating an OLS equation. The OLS residuals are used to obtain cross-section, time-series, and combined error variance components. The OLS </p><p> residuals t = ui + vt + wit, the three error components, respectively, are then used to obtain the GLS parameters estimates. GLS applies when heteroscedasticity and autocorrelation problems (that typically characterize panel data) are detected. For more on random-effects models, the reader is referred to Maddala (1971).</p><p>Many explanatory variables were initially explored to explain average neighborhood price variations. To obtain parsimonious regression equations, a combination of forward and backward selection procedure stepwise routine (Eckerd, 1985) is useful. To produce robust models, the stepwise routine produces a best model from all variables based on their statistical significance. Alternatively, using the stepwise routine, variables with estimated coefficients not significantly different from zero or with illogical signs were eliminated iteratively before the equation was re-estimated. One variable was deleted in each iteration until all estimated coefficients left are significantly different from zero at about the 5% level and had a logically acceptable sign. Accordingly, the variables that ultimately populated the estimated equations are: SSF = average house square footage; </p><p>BD = average number of bedrooms; AHI = average minimum household income; MRt-2 = mortgage rate lagged two quarters where t = 1,…, T quarters; MRt-3 = mortgage rate lagged three quarters; MRt-6 = mortgage rate lagged six quarters. SSF and BD values were available from the DataQuick data sets. MR data were obtained from Federal Home </p><p>Loan Mortgage Corporation's (Freddie Mac) and were adjusted for inflation using the </p><p>Los Angeles-Riverside-Orange County, CA all urban CPI. Because income data is not </p><p>11 available by household, AHI was approximated using the prices of homes and standard lending rules. Standard lending rules mandate a minimum down payment of 20% of the amount needed to purchase a house. Mortgage payments are typically around 30% of a home-buying household’s annual income. Using these rules and the average price of homes four quarters before a current quarter (the time needed to actually complete a purchase from the time a decision is made to buy a house), income for a current quarter was approximated. The loan amount lagged one year was computed as: LAt = home pricet-4 * 0.8. LA was then used to approximate the average monthly mortgage payment </p><p>(PMT), where </p><p> k 轾(MRt- 4 /12)*(1+ MR t - 4 /12) PMTt= LA t 犏 k . (1) 臌 (1+ MRt- 4 /12) - 1 where k = loan duration (360 months for 30-year fixed loan). Since the approximate annual payments (APt = PMTt*12) are 30% of a household’s annual income (It),</p><p>It= AP t / 0.3. (2)</p><p>With i = 1, …, n houses sold in a neighborhood during tth quarter, the approximate average annual neighborhood household income (AHIt) is</p><p>1 n AHIt= I t . (3) n i= 1</p><p>AHI thus approximates annual household income for a neighborhood i such that prices of houses sold a year ago determine the level of income needed to purchase a house in the current quarter. Given that AHI is different for each neighborhood, the variable plays two roles. In addition to being a measure of income needed to purchase a house in each neighborhood based on the average prices of homes four quarters earlier, it is also a spatial variable that distinguishes between neighborhoods for other reasons.</p><p>12 5. Comparison of Results</p><p>A comparison between results of estimating average prices and individual prices is presented here to show whether averaging prices does help produce better estimates of the equations and/or better forecasts. First, GLS hedonic individual homes price levels models for each city’s are obtained. Average neighborhood price models follow.</p><p>Comparisons are first made between estimated models then between forecasts the different models deliver. Comparison of the estimated models is based upon the coefficient of determination (R2) and the mean absolute percent error (MAPE) of fitted values. Comparisons between the forecasts are based upon prediction MAPE (PMAPE) and Theil’s U-statistic. Theil’s U is defined as</p><p>1 2 (Y- Yf ) F f f U = (4) 2 1 1 2 邋Yf + Yf Ff F f where Y = observed values to forecast ex post, Y = their forecasted values, and the forecast horizon f = 1, …, F periods. If U = 0, the model is delivering a perfect forecast; while if U = 1, the model has no predictive power.</p><p>The estimated GSL equations using individual and using average home prices in each of the defined neighborhoods for each city follow. The p-values are reported in parentheses below the estimated coefficients. </p><p>Burbank - BB:</p><p>Using individual home prices for the entire city:</p><p>RPt = 141.181 + 76.455 SSFt + 0.259 CAt + 11.45 NGt + 3.191 LSFt – 7.271 DVAt + 1.834 AHIt (0.00) (0.00) (0.005) (0.009) (0.003) (0.018) (0.00)</p><p>13 – 10.944 MRt-2 – 10.918 MRt-3 – 8.475 MRt-6 + 1.378 SCDt – 1.168 PAt (5) (0.00) (0.00) (0.00) (0.00) (0.00)</p><p>Using average neighborhood prices:</p><p>CT: APt = 121.5 + 93.65 SSFt + 0.79 CAt + 1.64 AHIt - 12.295 MRt-3 - 16.405 MRt-6 (6) (0.00) (0.00) (0.00) (0.00) (0.00) (0.00) PN: APt = 153.8 + 94.4 SSFt + 0.52 CAt + 5.08 LSFt + 0.845 AHIt (0.00) (0.00) (0.03) (0.006) (0.005) - 6.56 MRt-3 - 11.7 MRt-5 - 13.53 MRt-6 (7) (0.023) (0.001) (0.00) ZIP: APt = 105 + 98.17 SSFt + 1.05 CAt + 1.46 AHIt - 10.84 MRt-3 - 16.86 MRt-6 (8) (0.00) (0.00) (0.00) (0.00) (0.00) (0.00)</p><p>Carlsbad - CB:</p><p>Using individual home prices for the entire city:</p><p>RPt = 151.182 + 97.512 SSFt + 1.051 CAt + 13.47 NGt + 1.987 AHIt (0.00) (0.00) (0.001) (0.00) (0.00) </p><p>– 12.05 MRt-2 – 5.886 MRt-3 – 15.306 MRt-6 + 2.141 SCDt – 21.293 PAt (9) (0.00) (0.022) (0.00) (0.00) (0.00)</p><p>Using average neighborhood prices:</p><p>CT: APt = 160.2 + 88.1 SSFt + 1.845 AHIt - 10.37 MRt-2 - 14.79 MRt-5 - 5.36 SCDt (10) (0.00) (0.00) (0.00) (0.00) (0.00) (0.00) PN: APt = 146.3 + 39.8 SSFt + 9.8 BDt + 3.14 AHIt - 16.44 MRt-2 - 9.59 MRt-6 - 20.98 PAt (11) (0.00) (0.00) (0.06) (0.00) (0.00) (0.019) (0.003) ZIP: APt = 95.4 + 62.99 SSFt + 2.73 AHIt - 9.17 MRt-2 - 9.23 MRt-3 - 16.18 PAt (12) (0.00) (0.00) (0.00) (0.005) (0.019) (0.016)</p><p>Redlands - RD:</p><p>Using individual home prices for the entire city:</p><p>RPt = 82.425 + 44.634 SSFt + 0.079 CAt + 5.28 NGt + 0.457 LSFt – 6. 275 DVAt + 2.165 AHIt (0.00) (0.00) (0.034) (0.01) (0.00) (0.054) (0.00)</p><p>– 6.3234 MRt-2 – 5.809 MRt-3 – 6.3095 MRt-6 – 1.139 PAt (13) (0.00) (0.00) (0.00) (0.00)</p><p>Using average neighborhood prices:</p><p>CT: APt = 113.8 + 52.17 SSFt + 0.35 LSFt + 1.7 AHIt - 7.02 MRt-2 (0.00) (0.00) (0.06) (0.00) (0.002) - 5.52 MRt-5 - 7.83 MRt-6 - 0.38 PHt (14) ( (0.007) (0.00) (0.00) </p><p>14 PN: APt = 67.1 + 46.07 SSFt + 2.56 AHIt - 8.31 MRt-2 - 3.68 MRt-3 - 3.82 MRt-6 (15) (0.00) (0.00) (0.00) (0.00) (0.03) (0.03)</p><p>ZIP: APt = 59 SSFt + 0.54 CAt + 2.15 AHIt - 11.12 MR2 + 0.26 PHt (16) (0.00) (0.00) (0.00) (0.00) (0.03) </p><p>Riverside - RS:</p><p>Using individual home prices for the entire city:</p><p>RPt = 86.276 + 33.374 SSFt + 0.266 CAt + 1.582 NGt + 0.55 LSFt – 6.19 DVAt + 2.988 AHIt (0.00) (0.00) (0.005) (0.009) (0.00) (0.048) (0.00)</p><p>– 4.424 MRt-2 – 7.578 MRt-3 – 5.079 MRt-6 - 0.494 SCDt + 1.776 PAt (17) (0.00) (0.00) (0.00) (0.00) (0.00)</p><p>Using average neighborhood prices:</p><p>CT: APt = 51.14 + 17.14 SSFt + 3.63 AHIt - 3.5 MRt-2 – 5.88 MRt-3 (18) (0.00) (0.00) (0.00) (0.001) (0.00) </p><p>PN: APt = 67.8 + 24.33 SSFt + 3.04 AHIt - 3.20 MRt-2 - 5.8 MRt-3 - 2.82 MRt-6 (19) (0.00) (0.00) (0.00) (0.00) (0.00) (0.00)</p><p>ZIP: APt = 60.97 + 21.11 SSFt + 3.52 AHIt - 3.1 MRt-2 - 5.36 MRt-3 - 3.08 MRt-6 (20) (0.00) (0.00) (0.00) (0.00) (0.00) (0.00)</p><p>In equations (15) – (20), SSF = average structure square footage; BD = average number of bedrooms; CA = average construction age; LSF = average lot square footage; AHI = average minimum household income needed to purchase a house; MR = mortgage rate; </p><p>PA = percent of African American population in a neighborhood; PH = percent of </p><p>Hispanic population in a neighborhood; SCD = average distance to nearest school in the neighborhood. All estimated coefficients have signs consistent with logical expectations and are significantly different from zero at the 5% level of significance (except for one – </p><p>BD in (10) that is significant at the 6%). Estimation (over 2000-2004) and forecast (for </p><p>2006) statistics of the above equations are in Table 1.</p><p>Table 1 Estimation and forecast comparative statistics Estimation Statistics Forecast Statistics Obs. R2 MAPE DW Obs. U PMAPE</p><p>15 BB Individual Prices: 3189 0.78 15.73 1.76 794 0.09 13.94 Neighborhoods: CT 260 0.86 8.96 1.57 76 0.05 7.76 PN 146 0.91 8.35 1.63 42 0.06 8.53 ZIP 221 0.85 10.19 1.62 61 0.07 11.47 CB Individual Prices: 1230 0.81 19.10 1.62 215 0.10 17.38 Neighborhoods: CT 117 0.91 17.35 1.86 25 0.10 14.95 PN 276 0.88 13.19 1.77 60 0.06 10.09 ZIP 264 0.89 14.43 1.75 57 0.09 13.53 RD Individual Prices: 3393 0.78 17.83 1.70 788 0.12 15.56 Neighborhoods: CT 155 0.89 11.75 1.74 40 0.09 12.53 PN 242 0.91 11.34 1.86 63 0.08 11.17 ZIP 216 0.91 9.00 1.50 60 0.09 10.78 RS Individual Prices: 3571 0.71 14.65 1.70 901 0.11 17.45 Neighborhoods: CT 178 0.86 7.61 1.77 38 0.09 10.65 PN 260 0.87 6.95 1.54 52 0.07 11.47 ZIP 344 0.83 8.01 1.60 77 0.06 10.37</p><p>The results in Table 1 on estimation statistics provide a comparison between the number of observations used to obtain each equation (obs.), the R2, MAPE, and the Durbin-</p><p>Watson statistic. The coefficients of determination (R2) for using individual price data are all lower than those of the average neighborhood price equations. The MAPE statistics also confirm that the average price equations may have the advantage. The DW statistics are persistently below the critical 2.0 level suggesting slight positive autocorrelation persisting. Forecast statistics provide comparisons between the number of observations, the U-statistic, and the prediction MAPE (PMAPE). For all four cities, the average neighborhood price equations show forecast statistics suggesting improvements over the individual home price equations. Generally, the 2005 predictive powers using PN resolution models are better than the other two. </p><p>16 To test which equation produces the better 2005 forecasts of individual home prices for each city’s neighborhood resolution, we test the null hypothesis that predictions of prices using the average price equation ≤ predictions of the same prices using the individual price equation. It is assumed here that the average price equations can be equally useful in predicting individual home prices. Using the PMAPE statistic, the test can be rewritten as:</p><p>Ho: PMAPE1 - PMAPE2 ≤ 0 where PMAPE1 = PMAPE obtained when predicting 2005 individual prices using an average price equation and PMAPE2 = PMAPE obtained when predicting the same prices using an individual price equation. The test statistic to use is:</p><p>PMAPE- PMAPE z = 1 2 s2 s 2 (21) 1+ 2 F F</p><p>Where s1 = variance of PMAPE1, s2 = variance of PMAPE2, and F is the sample of 2005 predicted ex post. </p><p>Table 2 Comparison of individual home price 2005 forecasts </p><p>PMAPE1 PMAPE2 z-score p-value 1-tailed BB PN 14.14 13.94 -0.10 0.46 CT 22.76 13.94 4.59 0.00 ZIP+1 22.99 13.94 4.71 0.00</p><p>CB PN 20.22 17.38 0.65 0.26 CT 17.51 17.38 0.03 0.49 ZIP+1 18.09 17.38 0.17 0.43</p><p>RD PN 16.29 15.56 0.57 0.29 CT 20.47 15.56 3.92 0.00 ZIP+1 20.37 15.56 3.71 0.00</p><p>RS</p><p>17 PN 19.87 17.34 1.84 0.03 CT 19.68 17.34 1.67 0.05 ZIP+1 18.78 17.34 1.02 0.15</p><p>The comparison of the test results are in Table 2. Although PMAPE1 > PMAPE2 in all situations, the null is not rejected at the 5% level of significance for PN in three of the four cities. This means that it is possible to obtain predictions of individual home prices using the average price equations that are not significantly different from those obtained using the individual price equations. </p><p>The better neighborhood models may now be used to determine the future of housing prices. They are used to predict average neighborhood prices in 2006 assuming that houses sold in 2005 were resold in 2006. Predicting 2006 prices is possible without having to predict any of the explanatory variables; the income variable is lagged one year and because mortgage rates are easily adjusted to account for increases that occurred in the first half of 2006. The 2005 and 2006 forecasts were then used to compute expected price changes between the two years. Year-over-year expected quarterly changes in average neighborhood price levels using the PN equations is reported in Table 3. PN is selected since it was best according to the statistics in Table 2. The results in Table 3 suggest that home prices in 2006 are expected to rise only in BB and decrease otherwise. </p><p>Figures 5 (a) – (d) compare actual real average neighborhood prices with the ex post forecasts for 2005. Figures 6 (a) – (d) compare average neighborhood ex post price forecasts for 2005 with ex ante price forecasts for 2006.</p><p>Table 3 2006 over 2005 quarterly expected % price changes BB CB RD RS Q1 9.20 -4.22 4.78 3.06</p><p>18 Q2 0.89 -3.08 -1.86 -1.40 Q3 0.08 -6.47 -1.96 -5.80 Q4 2.22 -6.75 -2.38 -5.76</p><p>RP 2005 ex post Forecast</p><p>$'000</p><p>500</p><p>400</p><p>300</p><p>200</p><p>100 1 1 1 1 1 1 1 1 1 1 2 2 2 2 2 2 2 2 2 2 2 3 3 3 3 3 3 3 3 3 3 3 4 4 4 4 4 4 4 4 4 4 Quarter (a) BB-PN 2005 actual and forecasted values</p><p>RP 2005 ex post Forecast $'000 700 600 500 400 300 200 100 0 1 1 1 1 1 1 1 1 2 2 2 2 2 2 2 3 3 3 3 3 3 3 3 4 4 4 4 4 4 4 Quarter (b) CB-PN 2005 actual and forecasted values</p><p>19 RP 2005 ex post Forecast $'000 500</p><p>400</p><p>300</p><p>200</p><p>100</p><p>0 1 1 1 1 1 1 1 1 2 2 2 2 2 2 2 2 2 3 3 3 3 3 3 3 3 3 4 4 4 4 4 4 4 Quarter (c) RD-PN 2005 actual and forecasted values</p><p>RP 2005 ex post Forecast $'000 250</p><p>200</p><p>150</p><p>100</p><p>50 1 1 1 1 1 1 1 1 1 1 1 1 1 2 2 2 2 2 2 2 2 2 2 2 2 2 3 3 3 3 3 3 3 3 3 3 3 3 3 4 4 4 4 4 4 4 4 4 4 4 4 4 Quarter (d) RS-PN 2005 actual and forecasted values</p><p>Figure 5. Actual and predicted 2005 real average neighborhood prices.</p><p>2005 ex post Forecast 2006 ex ante Forecast $'000 500</p><p>400</p><p>300</p><p>200</p><p>100 1 1 1 1 1 1 1 1 1 1 2 2 2 2 2 2 2 2 2 2 2 3 3 3 3 3 3 3 3 3 3 3 4 4 4 4 4 4 4 4 4 4 Quarter (a) BB-PN 2005 and 2006 forecast comparison</p><p>20 2005 ex post Forecast 2006 ex ante Forecast $'000 700 600 500 400 300 200 100 0 1 1 1 1 1 1 1 1 2 2 2 2 2 2 2 3 3 3 3 3 3 3 3 4 4 4 4 4 4 4</p><p>(b) CB-PN 2005 and 2006 forecast comparison Quarter</p><p>2005 ex post Forecast 2006 ex ante Forecast $'000 500</p><p>400</p><p>300</p><p>200</p><p>100</p><p>0 1 1 1 1 1 1 1 1 2 2 2 2 2 2 2 2 2 3 3 3 3 3 3 3 3 3 4 4 4 4 4 4 4</p><p>(c) RD-PN 2005 and 2006 forecast comparison Quarter</p><p>2005 ex post Forecast 2006 ex ante Forecast</p><p>$'000 200</p><p>150</p><p>100</p><p>50 1 1 1 1 1 1 1 1 1 1 1 1 1 2 2 2 2 2 2 2 2 2 2 2 2 2 3 3 3 3 3 3 3 3 3 3 3 3 3 4 4 4 4 4 4 4 4 4 4 4 4 4 (d) RS-PN 2005 and 2006 forecast comparison Quarter</p><p>Figure 6. Ex post predicted 2005 versus ex ante 2006 real average neighborhood prices.</p><p>21 Ideally forecast statistics should be compared with those reported in the literature. </p><p>However, reported results found do not use consistent dependent variables or report the same statistics. MSE cannot be compared since they are dependent on relative prices of homes in different areas and time periods analyzed. Only MAPE can be compared. A comparison of the statistics found is in Table 4. There is a main difference between the results in Table 3 and the results in Table 2. The results in Table 2 belong to forecasts of future prices. Those in Table 4 are predictions of prices of homes sold in the same period as the data used in model estimation.</p><p>Table 4. Comparison with literature forecast statistics Sample MAPE Gençay and Yang (1996) 50 12.3 Gençay and Yang (1996) 100 16.7 Fletcher et al. (2000) 525 19.8 Bourassa et al. (2003) 200 14.8</p><p>6. Concluding Remarks</p><p>This paper proposed a novel specification strategy to forecast residential home prices. </p><p>Rather than estimating a model to forecast prices directly, an equation to estimate an average neighborhood price is adopted instead. The proposed method implicitly generalizes subtleties about neighborhood attributes. Neighborhoods were defined according to census tract (CT), the county assessor’s parcel number (PN), and the ZIP+1 code (ZIP+1). CT and ZIP+1 codes are well established resolution definitions. The PN aggregation is justifiable since parcel numbers are typically assigned sequentially to contiguous parcels of land before the construction of a house. Therefore, PN- neighborhoods are assumed to include homes that have similar houses and akin spatial attributes. Average price equations were specified as a function of the average house </p><p>22 attributes, neighborhood attributes, spatial differences, and mortgage rates taken at different temporal lags. GLS, a standard parametric modeling technique that applies in the case of panel data, was used to find that model which forecasts best. Estimated equations utilized five years (2000-2004) of data. Average prices were best explained average square footage of houses, average household income needed to purchase a property, and different lags of real mortgage rates. The best models were then used to forecast prices of homes sold in 2005 ex post and 2006 ex ante assuming that houses sold in 2005 are sold again in 2006. Those models forecasted individual home prices sold in </p><p>2005 in the four cities reasonably well. The forecast statistics were consistent with those found in other studies. They also predicted logical changes for 2006 over 2005. </p><p>Predictions of price changes in 2006 suggest that real estate prices are expected to decline in three of the four cities studied.</p><p>This paper contributed in two directions. It introduced a new neighborhood averaging of prices and attributes strategy that may have some merit and might warrant further investigations. Further, while most models in the literature “predict” same-period sale prices, the models presented in this paper were used to “forecast” future period prices. </p><p>Acknowledgement</p><p>This work would not have been possible without the support of the University of </p><p>Redlands, School of Business. Grants were generously provided to purchase the data from DataQuick.</p><p>23 References</p><p>Ball M (1973) Recent empirical work on the determinants of relative house prices. Urban Studies 10: 213-233</p><p>Basu A, Thibodeau TG (1998) Analysis of spatial autocorrelation in house prices. Journal of Real Estate Finance and Economics 17: 61-85</p><p>Bin O (2004) A prediction comparison of housing sales prices by parametric versus non- parametric regressions. Journal of Housing Economics 13: 68-84</p><p>Bourassa SC, Hoesli M, Peng VS (2003) Do housing submarkets really matter? Journal of Housing Economics 12: 12-18</p><p>Can A (1998) GIS and spatial analysis of housing and mortgage markets. Journal of Housing Research 9: 61-86</p><p>Clapp J, and Giaccotto C (2002) Evaluating house price forecasts. Journal of Real Estate Research 24:1-26</p><p>Clapp J, Kim H, and Gelfand A (2002) Predicting spatial patterns of house prices using </p><p>LPR and Bayesian smoothing. Real Estate Economics 30: 505-532</p><p>Clapp JM (2003) A semiparametric method for valuing residential locations: Application to automated valuation. Journal of Real Estate Finance and Economics 27: 303-320</p><p>Clapp J (2004) A semiparametric method for estimating local house price indices. Real Estate Economics 32: 127-160</p><p>Cukierman A (1979) The relationship between relative prices and the general price level: a suggested interpretation. The American Economic Review 69: 444-447</p><p>DataQuick</p><p>24 Dubin R (1988) Estimation of regression coefficients in the presence of spatially autocorrelated error terms. Review of Economics and Statistics 70: 466-474</p><p>Eckert J (1985) Modern modeling methodologies. In: Woolery A and Shea S (eds.) Introduction to computer assisted valuation. Oelgeschlager, Gunn and Hain in association with the Lincoln Institute of Land Policy, Boston, pp. 51-83</p><p>Fletcher M, Gallimore P, Mangan J (2000) The modeling of housing submarkets. Journal of Property Investment and Finance 18: 473-487</p><p>Fotheringham S, Brunsdon C, and Charlton M (2002) Geographically Weighted </p><p>Regression: The Analysis of Spatially Varying Relationships. John Wiley and Sons, </p><p>West Essex, England</p><p>Gençay R, Yang X (1996) A forecast comparison of residential housing prices by parametric versus semiparametric conditional mean estimators. Economic Letters 52: 129-135</p><p>Getis A and Ord K (1992) The analysis of spatial autocorrelation by use of distance </p><p> statistics. Geographical Analysis 24: 189-206</p><p>Goldfeld S and Quandt R (1965) Some tests for homoscedasticity. Journal of The </p><p>American Statistical Association 60: 539-547</p><p>Goodman AC, Thibodeau TG (2003) Housing market segmentation and hedonic prediction accuracy. Journal of Housing Economics 12: 181-201</p><p>Haining R (2003) Spatial Data Analysis. Cambridge University Press, Cambridge.</p><p>Longley P, and Batty M (1996) Spatial Analysis: Modelling in a GIS Environment. </p><p>GeoInformation International. Cambridge, UK</p><p>25 Halverson R, Pollakowski H (1981) Choice of functional form for hedonic price equations. Journal of Urban Economics 10. 37-40</p><p>Hill R (2004) Constructing price indexes across space and time: the case of the European Union. The American Economic Review 94: 1379-1410</p><p>Limsombunchai V, Gan C, and Lee M (2004) House price prediction: Hedonic price model vs. artificial neural network. American Journal of Applied Sciences 1:193-201</p><p>Mason C and Quigley J (1996) Non-parametric hedonic housing prices. Housing Studies </p><p>11: 373-385</p><p>Officer L (1978) The relationship between absolute and relative purchasing power parity. The Review of Economics and Statistics 60: 562-568</p><p>Pindyck R and Rubinfeld D (1998) Econometric Models and Economic Forecasting.</p><p>Irwin McGraw-Hill, Boston</p><p>Rossini P (2000) Using expert systems and artificial intelligence for real estate </p><p> forecasting, Sixth Annual Pacific-Rim Real Estate Society Conference, Sydney, </p><p>Australia, </p><p> http://business2.unisa.edu.au/prres/Proceedings/Proceedings2000/P6A2.pdf.</p><p>Rubin D (1992) Use of forecasting signatures to help distinguish periodicity, randomness,</p><p> and chaos in ripples and other spatial patterns. Chaos 2: 525-535</p><p>Schabenberger O, Gotway C (2005) Statistical Methods for Spatial Data Analysis. Chapman and Hall/CRC, Boca Raton</p><p>Shiller R (1993) Measuring asset value for cash settlement in derivative markets: hedonic repeated measures indices and perpetual futures. Journal of Finance 48: 911-931</p><p>26 White H (1980) Heteroskedasticity-consistent covariance matrix estimator and a direct test for heteroskedasticity. Econometrica 48: 817-838</p><p>Zhou Z (1997) Forecasting sales and price for existing single-family homes: a VAR model with error correction. Journal of Real Estate Research 14:155-168</p><p>27</p>
Details
-
File Typepdf
-
Upload Time-
-
Content LanguagesEnglish
-
Upload UserAnonymous/Not logged-in
-
File Pages27 Page
-
File Size-