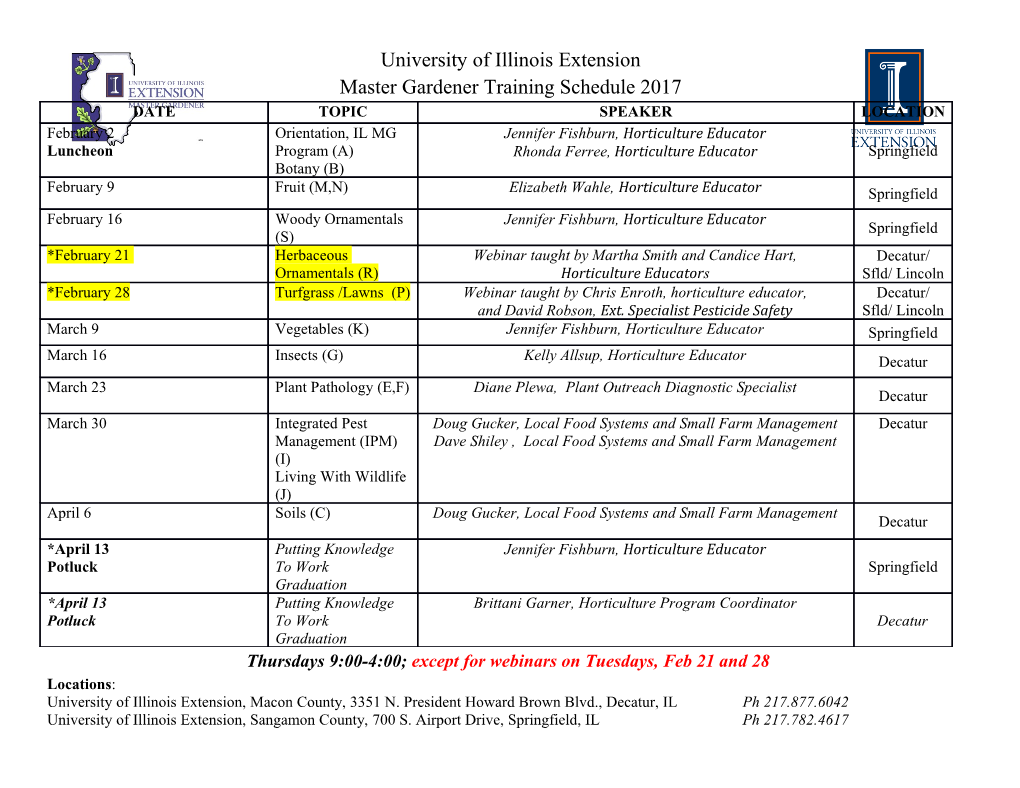
<p> Implications relevant to CFA model misfit, reliability, and the Five</p><p>Factor Model as measured by the NEO-FFI</p><p>Gilles E. Gignac*</p><p>University of Western Australia</p><p>Timothy C. Bates</p><p>University of Edinburgh</p><p>Kerry L. Jang</p><p>University of British Columbia</p><p>ABSTRACT</p><p>Several attempts to model the NEO PI-R/NEO-FFI as a FFM have failed to achieve adequate CFA model fit, which has prompted some personality researchers to suggest serious problems with SEM. In this paper, it was hypothesized that a portion of the model misfit associated with the NEO-FFI may be due to intra-dimensional correlated residuals, which was expected to result in upwardly biased estimates of internal consistency reliability. Based on a latent variable modeling approach to the estimation of internal consistency reliability, the degree of reliability overestimation was found to range from .02 to .06. Because the consequences can have implications for internal consistency reliability estimation, it is recommended that researchers attend to evidence of model misfit, rather than dismissing the issue as a problem with SEM.</p><p>*Corresponding Author: School of Psychology University of Western Australia 35 Stirling Highway Crawley, Western Australia 6009 AUSTRALIA email: [email protected]</p><p>Accepted 5/3/2007 CFA and NEO 2</p><p>Implications Relevant to CFA Model Misfit, Reliability, and the Five Factor </p><p>Model as Measured by the NEO-FFI</p><p>It is well established that both unrestricted (EFA) and restricted (CFA) factor analysis have direct corollaries to classical test theory (CTT) and internal consistency reliability (McDonald, 1999), where reliability is defined as the ratio of true score variance to total variance (Lord & Novick, 1968). Estimates of internal consistency reliability are frequently estimated via Cronbach’s α, which can be formulated as</p><p> k 2 *COV a = е S 2 ,COV where k = number of indicators in the scale, COV = mean inter-item covariance, and</p><p>2 е S , COV = the sum of the square variance/covariance matrix (Cortina, 1993). </p><p>However, it is important to note that the application of Cronbach’s α is based on three assumptions (McDonald, 1970): (1) that the error variances of each item is unrelated to the true score variance; (2) that the factor loadings of the congeneric model are equal (tau-equivalence); and (3) that the errors are uncorrelated with each other.</p><p>The first assumption is primarily of theoretical interest, because the assumption is necessarily satisfied through use of any conventional true score/error variance decomposition procedure. In contrast, tau-equivalence is probably rarely achieved in practice, consequently, Cronbach’s α would be expected to be a ‘lower bound’ estimate of internal consistency reliability (Bentler & Woodward, 1983). </p><p>However, Reuterberg and Gustafsson (1992) found that the biasing effect of violating the assumption of tau-equivalence on reliability tends to be negligible (i.e., <.02). In contrast, the biasing effect of violating the assumption of uncorrelated errors can be CFA and NEO 3 quite large, contingent upon the amount of variance associated with the correlated errors (Raykov, 2001). Based on McDonald (1970), Raykov (2001) and Hancock and </p><p>Mueller (2001), the internal consistency reliability of an equally weighted composite estimated within a factor analytic framework may be formulated as: </p><p> k 2 ( l i ) еi=1 wA = k k 2 ( l i ) + d ii еi=1 еi=1</p><p> where λi = standardized factor loading, δii = standardized error variance. In the event that one or more correlated errors are observed within a factor model solution, the internal consistency reliability of the corresponding equally weighted composite may be formulated as:</p><p> k 2 ( l i ) еi=1 wB = k k ( l ) 2 + d + 2 d е i е ii е ij i=1 i=1 1Ј i< jЈ k</p><p> where λi and δii are defined as above and δij = standardized error covariances. Thus, the greater the amount of positive variance associated with the correlated errors within a factor model, the greater the amount of upward bias in the estimate of internal consistency reliability, all other things being equal. Cronbach’s α, ωA, and ωB, will yield the same estimates only when the underlying factor solution is tau-equivalent and the error terms are uncorrelated.</p><p>Confirmatory Factor Analysis and the NEO PI-R/NEO-FFI</p><p>Although the popularity of confirmatory factor analysis (CFA) has increased over the last decade or more, some dissatisfaction with the method has emerged. For instance, McCrae, Zonderman, Costa, Bond, and Paunonen (1996) tested via CFA the </p><p>Five Factor Model (FFM) based on the 30 facets of the NEO PI-R (Costa & CFA and NEO 4</p><p>McCrae,1992). Various combinations of FFMs were tested, which either excluded or included salient cross-loading facets, as well as correlated or uncorrelated personality dimensions. However, McCrae et al. (1996) failed to confirm the FFM based on TLI values of .52 to .83, i.e., below the demarcation criterion of .95 to indicate satisfactory fit for incremental close-fit indices (Hu & Bentler, 1999). Consequently, McCrae et al. (1996) concluded that there were “…serious problems with CFA itself when used to examine personality structure” (p. 563). Of what these CFA problems consist the reader is left to surmise, because McCrae et al. (1996) did not offer any suggestions and, instead, opted to endorse the plausibility of the FFM based on factor congruence coefficients derived from factor loadings obtained from a Procrustes rotation. The model misfit associated with FFM as measured by the NEO PI-R is not unique to the </p><p>McCrae et al. investigation, as others have also been reported (e.g., Borkeneau & </p><p>Ostendorf, 1990; Church & Burke, 1994; Vassend & Skrondal, 1997). Failures to confirm the FFM of personality via CFA have also been reported based on the NEO-</p><p>FFI, which is a 60-item short form of the NEO PI-R. For example, Mooradian and </p><p>Nezlek (1995) reported a NFI = .66 based on a sample of 601 undergraduates for an orthogonal FFM. Others have also failed to confirm the FFM as measured by the </p><p>NEO-FFI (e.g., Egan, Deary, & Austin, 2000; Parker, Bagby, & Summerfeldt, 1993; </p><p>Tokar, Fischer, Snell, & Harik-Williams, 1999), which has prompted further support for the contention that CFA likely suffers from serious problems and was of doubtful use to personality researchers.</p><p>The possibility that the FFM may not be an adequate representation of the covariance between the 30 facets within the NEO PI-R (or the 60 items of the NEO-</p><p>FFI) does not appear to have been entertained by McCrae et al. Nor was the possibility that the model misfit may have implications for the estimation of internal consistency reliability of the composite scores associated with the five personality CFA and NEO 5 dimensions. Despite the fact that many investigators have tested the CFA model fit of the FFM, no published investigation has yet to assess the model fit of the five factors, individually. If it were observed that the individual subscales associated with the </p><p>NEO-FFI were associated with inadequate model fit, it would follow that some intra- dimensional inter-item covariance remained unmodeled, which would violate the uncorrelated errors assumption associated with internal consistency reliability estimation (Lord & Novick, 1968), and possibly result in upwardly biased estimates of internal consistency reliability. An important advantage associated with modeling the FFM dimensions of the NEO FFI is that they are associated with only 12-items each (i.e., relatively small number). Thus, the contention that the poor CFA fit associated with FFM personality measures may be due to the large number of items included in such models (e.g., Raykov, 1998) would be substantially militated. </p><p>Method</p><p>Participants and Measure</p><p>The total sample was based on the combination of two independent samples. </p><p>One of the samples (N = 639) consisted mostly of university students, as well as some individuals from the general community (mean age = 26.13, SD = 10.08). The other sample consisted of 460 twin pairs (N = 920). However, for the purposes of not violating the independence assumption, only one twin was selected for the purposes of analyses, which yielded a sample of 460 individuals (mean age = 33.7, SD = 13.9). </p><p>Using a statistical procedure published by DeCarlo (1997), 22 multivariate outliers were identified and deleted, which resulted in a total sample of 1077. For the purposes of cross-validation, two samples (N= 538 & N=539) were selected from the initial sample of 1077 participants using the random selection algorithm in SPSS. All analyses were based on the NEO-FFI, which is the 60-item short form of the NEO PI- CFA and NEO 6</p><p>R (Costa & McCrae, 1996). Note that the 60 items within the NEO-FFI were recovered from the NEO PI-R for the purposes of the following analyses.</p><p>Data Analytic Strategy</p><p>The following analyses proceeded in three stages. First, each of the five dimensions of the NEO-FFI were modeled, individually, as single-factor models. An evaluation of the model fit was performed and ωA was estimated, irrespective of possible violations of the ‘no correlated errors’ assumption. Next, within each single- factor model, correlated residuals were added to the model successively, based on the modification indices (i.e., Lagrange multiplier), until both the TLI and CFI for each dimension tested individually achieved an approximate value of .950 (i.e., such that both CFI and TLI were within the range of .940 to .960), which is considered to be an indication of adequate model fit (Hu & Bentler, 1999). Two absolute close fit indices were also reported (SRMR and RMSEA), under the expectation that a well-fitting model would be associated with absolute close-fit values less than .08 (Hu and </p><p>Bentler, 1999). Estimates of ωB were then calculated based on the satisfactory-fitting model solutions. Finally, for the purposes of evaluating the replicability of the correlated errors, the models identified within the satisfactory-fitting conditions </p><p>(sample one) were re-tested on the sample two data and the corresponding ωA and ωB re-estimated on the sample two factor solutions. A correlated residual was considered replicated if was observed to be statistically significant and in the same direction within both sample solutions. Further, the mean absolute difference between the respective residuals was also calculated. All models were identified/scaled by constraining the latent variable variance to 1.0 and tested via AMOS 5.0.</p><p>Results CFA and NEO 7</p><p>The model fit statistics/indexes associated with each model for each dimension are summarized in Table 1. It can be observed that all five of the single-factor models </p><p>(i.e., each dimension tested individually) were associated with poor levels of model fit, which suggested the possibility of correlated errors. The completely standardized factor loadings and errors associated with each item for each dimension are presented in Tables 2 (Neuroticism), 3 (Extraversion), 4 (Openness), 5 (Agreeableness), and 6 </p><p>(Conscientiousness). The standardized correlated error terms added to each model to achieve satisfactory fit are presented in Tables 7 and 8. </p><p>The results associated with the cross-validation analyses (sample two) are presented within the same Tables (right side) for each respective dimension. It can be observed that two dimensions (i.e., Neuroticism and Agreeableness) were associated with a perfect level of replication (as defined in the Method section). Overall, the mean level of replication across the two samples for the satisfactory fitting models was calculated to be 92.0%, suggesting a high level of correspondence. Further, the mean absolute difference between the respective correlated residuals ranged from .04 to .05 across the five dimensions. Thus, overall, the correlated error terms were found to be highly replicable.</p><p>The biased and unbiased estimates of internal consistency reliability are reported in Table 9. It can be observed that the ωA estimates associated with the poor- fitting model solutions were all biased upwards, in comparison to the satisfactory model ωB estimates (see Note under Table 9 for an example of the calculations). More specifically, across all five dimensions (for sample one), the mean internal consistency reliability estimate was .83 for the poor fitting models versus .79 for the satisfactory fitting models. Thus, the mean difference (Δω) between the poor-fitting models and the satisfactory-fitting models was equal to .04. Extraversion was associated with the largest Δω difference at .06. As can be seen in Table 9 (right side), CFA and NEO 8</p><p> the sample two mean ωA and ωB estimates corresponded exactly to the sample one mean ωA and ωB estimates.</p><p>Discussion</p><p>The hypothesis that the CFA model misfit associated with the NEO-FFI would have implications with respect to internal consistency reliability was supported. </p><p>Specifically, the ωB estimates associated with the well-fitting factor model solutions were all lower than the comparable ωA estimates derived from the poorly fitting models. </p><p>The consequence of violating the uncorrelated errors assumption had the smallest effect on the Neuroticism domain. Specifically, the reduction in reliability from the poor-fitting model to the satisfactory-fitting model amounted to .02 (sample one). The majority of the correlated error variance was attributable to two items, 71 and 11, which exhibited a residual correlation of .41. An examination of the item content of items 71 and 11 revealed that the two items could probably be considered redundant. That is, both items include words such as ‘sad’, ‘depressed’, ‘lonely’, and </p><p>‘blue’ in virtually identical contexts. Given the great degree of similarity between these two items, it is perhaps not surprising that it was necessary to add a covariance link between their residuals to help achieve adequate CFA model fit.</p><p>In contrast to Neuroticism, the Extraversion modeling results demonstrated the largest biasing effect with respect to internal consistency reliability, which amounted to a difference in reliability of .06 (i.e., .83 vs. .77). Expressed as a correlation coefficient, the difference of .06 was equal to .25 (i.e., √.06), which is close to a moderate effect size (Cohen , 1992). Further, within three of the five dimensions, the reliability estimates were reduced to below .80, the minimum level of reliability recommended for basic research (Nunnally & Bernstein, 1994). Thus, previously published Cronbach’s α estimates associated with the NEO-FFI should probably not CFA and NEO 9 be viewed as lower-bound estimates of reliability (see Bentler & Woodward, 1983). </p><p>Rather, they should be viewed as upwardly biased estimates. Consequently, the reliability estimates which approached the .80 demarcation criterion (E, O, & A) should probably not be given the benefit of the doubt. </p><p>It is perhaps surprising that two comprehensive quantitative reviews of the internal consistency reliability of the scores derived from popular measures of the Big </p><p>Five/FFM did not mention the assumptions associated with estimating internal consistency reliability (i.e., Caruso, 2000; Viswesvaran & Ones, 2000). Based on 20 samples, Caruso (2000) estimated mean Cronbach’s alphas of N=.83, E=.75, O=.65, </p><p>A=.67 and C=.80. These estimates would only be expected to be accurate to the degree that the individual estimates included in the review were based on data which did not violate the uncorrelated errors assumption. Based on the results of this investigation, this assumption is likely untenable. Thus, it is probable that the estimates reported by Caruso (2000) and Viswesvaran & Ones (2000) are overestimates. </p><p>It may be argued that many of the correlated errors observed in this investigation were relatively large and that an attempt should be made to interpret their substantive meaningfulness. It may be argued further that the correlated error variance should be modeled as nested latent factors to reflect their substantive nature. </p><p>However, with respect to internal consistency reliability, it is irrelevant whether the correlated errors do or do not represent “substantive” variance, because modeling the correlated errors as nested latent variables within the global factor would nonetheless result in a reduction in the estimate of internal consistency reliability associated with the composite scores (see Gignac, Palmer, & Stough, in press). Thus, despite the fact that some of the correlated errors identified in this investigation may have represented narrower facet level variance, the conclusion that the poor-fitting single-factor CFA and NEO 10 solutions yielded upwardly biased estimates of internal consistency for the domain level aggregated scores would, nonetheless, remain unchanged. </p><p>Past contentions that CFA was seriously flawed as a technique may be questioned by the results of this investigation, as the individual FFM personality dimensions based on only 12 items failed to be associated with adequate model fit. </p><p>That is, latent variables with 12 observed variables, putatively measuring a single unidimensional factor, should probably not be considered excessively large. Several item level investigations in the broad area of individual differences have used CFA and achieved adequate model fit, based on scales with approximately 12 items or more (e.g., Guppy et al., 2004; Haase & Prapavessis, 2004; Noar, 2003; Tanaka & </p><p>Hubba, 1984). Thus, the problem alluded to by McCrae et al. (1996) may be unrelated to any technical limitation with SEM/CFA. Instead, the problem may reside within the</p><p>NEO’s as yet poorly understood (over)expansiveness. </p><p>In conclusion, personality researchers who may be working with measures of personality associated with unacceptable levels of CFA model-fit are encouraged to consider developing measures (and theories) with a narrower focus than would otherwise be compatible with the Big Five or the FFM. Such a view is consistent with </p><p>McGrath (2005), who contended that constructs in personality psychology may be excessively complex. From this perspective, it is recommended that future personality factor analytic research focus on addressing the individual “measurement” related elements (items and facets) of the psychometric measures of the FFM. That is, each proposed facet/dimension should be examined and refined, individually, according to both theory and empirical CFA results. Such a process would be consistent with the two-step procedure commonly endorsed in SEM research (see Anderson & Gerbing, </p><p>1988). Until this is achieved, it makes little sense to evaluate the FFM based on models that incorporate all five dimensions, simultaneously. Ultimately, the CFA and NEO 11 demonstration and acceptance of the unacceptable levels of model fit associated with comprehensive measures of personality may lead to alternative formulations and measures of personality, which, in turn, can and should be tested via CFA for the purpose of confirmation or disconfirmation. This process should be considered a healthy aspect of science, generally, and an element conducive to the robust evolution of personality theory and measurement, specifically.</p><p>References Anderson, J.C., & Gerbing, D.W. (1988). Structural equation modeling in practice: A review and recommended two-step approach. Psychological Bulletin, 103, 411-413.</p><p>Bentler, P.M., & Woodward, J.A. (1983). The greatest lower bound to reliability. In H. Wainer & S. Messick, (Eds). Principals of modern psychological measurement: A Festschrift for Frederic M. Lord (pp. 237-253). Hillsdale, NJ: CFA and NEO 12</p><p>Erlbaum.</p><p>Borkeneau, P., & Ostendorf, F. (1990). Comparing exploratory and confirmatory factor analysis: A study on the 5-factor model of personality. Personality and Individual Differences, 11, 515-524.</p><p>Caruso, J. C. (2000). Reliability generalization of the NEO personality scales. Educational and Psychological Measurement, 60(2), 236-254.</p><p>Church, A.T., & Burke, P.J. (1994). Exploratory and confirmatory tests of the Big Five and Tellegen's three- and four-dimensional models. Journal of Personality and Social Psychology, 66, 93-114.</p><p>Cohen, J. (1992). A power primer. Psychological Bulletin, 112, 155-159.</p><p>Cortina, (1993). What is coefficient alpha? An examination of theory and applications. Journal of Applied Psychology, 78(1), 98-104</p><p>Costa, P.T., & McCrae, R.R. (1992). Revised NEO Personality Inventory (NEO-PIR) and NEO Five-Factor Inventory professional manual.Odessa, FL: Psychological Assessment Resources.</p><p>DeCarlo, L.T. (1997). On the meaning of kurtosis. Psychological Methods, 2(3), 292- 307.</p><p>Egan, V., Deary, I., & Austin, E. (2000). The NEO-FFI: Emerging British norms and an item-level analysis suggest N, A and C are more reliable than O and E. Personality and Individual Differences, 29, 907-920.</p><p>Gignac, G.E., Palmer, B., Stough, C. (in press). A confirmatory factor analytic investigation of the TAS-20: Corroboration of a five-factor model and suggestions for improvement. Journal of Personality Assessment.</p><p>Guppy, A., Edwards, J. A., Brough, P., Peters-Bean, K.M., Sale, C., & Short, E. (2004). Journal of Occupational and Organizational Psychology, 77(1), 39- 62.</p><p>Hancock, G. R., & Mueller, R. O. (2001). Rethinking construct reliability within latent variable systems. In R. Cudeck, S. du Toit,& D. Sorebom (Eds.), CFA and NEO 13</p><p>Structural equation modeling: Present and future—A festschrift in honor of Karl Jöreskog (pp. 195-216). Lincolnwood, IL: Scientific Software International. </p><p>Haase, A.M., & Prapavessis, H. (2004).Assessing the factor structure of and composition of the Positive and Negative Perfectionism Scale in sport Personality and Individual Differences, 36(7), 1725-1740.</p><p>Hu, K-H, & Bentler, P.M. (1999). Cutoff criteria for fit indexes in covariance structure analysis: Conventional criteria versus new alternatives. Structural Equation Modeling, 6(1), 1-55.</p><p>Lord, F.M., & Novick, R. (1968). Statistical theories of mental test scores. Readings, MA: Addison-Wesley.</p><p>McCrae, R.R., Zonderman, A.B., Costa, P.T., Bond, M.H., & Paunonen, S.V. (1996). Evaluating replicability of factors in the revised NEO personality inventory: Confirmatory factor analysis versus procrustes rotation. Journal of Personality and Social Psychology, 70(3), 552-566.</p><p>McDonald, R.P. (1970). The theoretical foundations of principal factor analysis, canonical factor analysis, and alpha factor analysis. British Journal of Statistical and Mathematical Psyhcology, 23(1), 1-21.</p><p>McDonald, R.P. (1999). Test Theory: A unified treatment. Mahwah, New Jersey: Lawrence Erlbaum.</p><p>McGrath, R. E. (2005). Conceptual complexity and construct validity. Journal of Personality Assessment, 85(2), 112-124.</p><p>Moordian, T.A., & Nezlek, J.B. (1995). Comparing the NEO-FFI and Saucier's Mini- Markers as measures of the Big Five. Personality and Individual Differences, 21(2), 213-215.</p><p>Noar, S.M. (2003). The role of structural equation modeling in scale development. Structural Equation Modeling, 10(4), 622-647.</p><p>Nunnaly, J.C., & Bernstein, I.H. (1994). Psychometric Theory. Sydney: McGraw-Hill. CFA and NEO 14</p><p>Parker, J.D.A., Bagby, R.M., & Summerfeldt, L.J. (1993). Confirmatory factor analysis of the revised NEO personality inventory. Personality and Individual Differences, 15, 463-466.</p><p>Raykov, T. (1998). On the use of confirmatory factor analysis in personality research. </p><p>Personality and Individual Differences, 23, 291-293</p><p>Raykov, T. (2001). Bias in coefficient alpha for fixed congeneric measures with correlated errors. Applied psychological measurement, 25(1), 69-76.</p><p>Reuterberg, S-E, & Gustafsson, J-E. (1992). Confirmatory factor analysis and reliability: Testing measurement model assumptions. Educational and Psychological Measurement, 52, 795-811.</p><p>Tanaka, J.S., Huba, G.J. (1984). Confirmatory hierarchical factor analyses on psychological distress measures. Journal of Personality & Social Psychology, 46(3), 621-635.</p><p>Tokar, D.M., Fischer, A.R., Snell, A.F., & Harik-Williams, N. (1999). Efficient assessment of the five factor model of personality: Structural validity analyses of the NEO Five Factor Inventory (Form S). Measurement and Evaluation in Counseling and Development, 32(1), 234-239.</p><p>Vassend, O., & Skrondal, A. (1997). Validation of the NEO Personality Inventory and the five-factor model. Can findings from exploratory and confirmatory factor analysis be reconciled? European Journal of Personality, 11(2), 147-166.</p><p>Viswesvaran, C., & Ones, D.S. (2000). Measurement error in the "Big Five Factors" personality assessment: Reliability generalization across studies and measures. Educational and Psychological Measurement, 60(2), 224-235. CFA and NEO 15 Table 1 Summary of Maximum Likelihood Model Fit Statistics/Indexes Sample 1 Sample 2 Fit Level χ2 (df) TLI CFI SRMR RMSEA χ2 (df) TLI CFI SRMR RMSEA Neuroticism Neuroticism Poor 279.59(54)* .872 .895 .050 .088 200.21(54)* .916 .931 .943 .071 Satisfactory 128.71(50)* .952 .963 .036 .054 100.91(50)* .968 .976 .033 .044 Extraversion Extraversion Poor 338.27(54)* .783 .823 .067 .099 310.03(54)* .801 .837 .064 .094 Satisfactory 104.68(43)* .941 .962 .039 .052 132.78(43)* .912 .943 .043 .062 Openness Openness Poor 278.96(54)* .813 .847 .058 .088 288.68(54)* .809 .844 .059 .090 Satisfactory 102.25(49)* .951 .964 .039 .045 116.53(49)* .940 .955 .041 .051 Agreeableness Agreeableness Poor 202.22(54)* .850 .877 .054 .071 300.59(54)* .743 .790 .070 .092 Satisfactory 88.78(45)* .947 .964 .038 .043 161.37(45)* .854 .901 .054 .069 Conscientiousness Conscientiousness Poor 321.93(54)* .836 .866 .062 .096 271.33(54)* .870 .894 .055 .086 Satisfactory 126.38(45)* .940 .959 .042 .058 137.05(45)* .934 .955 .040 .062 *p<.05; Null model χ2: N=2220.63; E=1670.50; O=1535.06; A=1271.20; C=2066.91(sample 1); N=2189.87; E=1637.67; O=1569.87; A=1238.68; C=2106.76(sample 2); all df=66. CFA and NEO 17</p><p>Table 2 Completely Standardized MLE Parameter Estimates for Poor- and Satisfactory- Fitting Single-Factor Models: Neuroticism Sample One Sample Two Poor Satisfactory Poor Satisfactory Items λ δ λ δ λ δ λ δ 136 .44 .81 .44 .81 .48 .77 .48 .77 86 .56 .69 .62 .62 .65 .58 .66 .56 91 .65 .58 .68 .54 .65 .58 .66 .56 41 .69 .52 .66 .56 .73 .47 .72 .48 76 .56 .69 .55 .70 .60 .64 .58 .66 26 .64 .59 .62 .62 .58 .66 .57 .68 6 .52 .73 .52 .73 .50 .75 .50 .75 221 .68 .54 .66 .56 .68 .54 .68 .54 1 .38 .86 .39 .85 .44 .81 .45 .80 61 .66 .56 .68 .54 .61 .63 .62 .62 71 .71 .50 .67 .55 .70 .51 .67 .55 11 .69 .52 .65 .58 .69 .52 .66 .56 7.18 7.58 7.14 7.65 7.31 7.45 7.25 7.53 2 51.55 50.98 53.44 52.56 ωA=.87 ωB=.85 ωA=.88 ωB=.85 Note. All factor loadings were statistically significant (p<.05).</p><p>Table 3 Completely Standardized MLE Parameter Estimates for Poor- and Satisfactory- Fitting Single-Factor Models: Extraversion Sample 1 Sample 2 Poor Satisfactory Poor Satisfactory Items λ δ λ δ λ δ λ δ 177 .72 .48 .74 .45 .77 .41 .78 .39 CFA and NEO 18</p><p>87 .53 .72 .53 .72 .58 .66 .58 .66 147 .44 .81 .43 .82 .47 .78 .46 .79 237 .53 .72 .55 .70 .54 .71 .55 .70 197 .45 .80 .40 .84 .42 .82 .38 .86 227 .58 .66 .58 .66 .54 .71 .54 .71 107 .61 .63 .63 .60 .54 .71 .56 .69 142 .58 .66 .54 .71 .53 .72 .50 .75 37 .55 .70 .47 .78 .57 .68 .49 .76 67 .42 .82 .37 .86 .43 .82 .38 .86 122 .62 .62 .59 .65 .62 .62 .58 .66 162 .41 .83 .42 .82 .41 .83 .41 .83 6.44 8.45 6.25 8.62 6.42 8.46 6.21 8.65 2 41.47 39.06 41.22 38.56 ωA=.83 ωB=.77 ωA=.83 ωB=.77 Note. All factor loadings were statistically significant (p<.05).</p><p>Table 4 Completely Standardized MLE Parameter Estimates for Poor- and Satisfactory- Fitting Single-Factor Models: Openness Sample 1 Sample 2 Poor Satisfactory Poor Satisfactory Items λ δ λ δ λ δ λ δ 88 .14 .98 .13 .98 .19 .96 .19 .96 93 .40 .84 .41 .83 .43 .82 .44 .81 163 .39 .85 .40 .84 .35 .88 .35 .88 98 .72 .48 .69 .52 .71 .50 .67 .55 128 .69 .52 .65 .58 .69 .52 .64 .59 188 .74 .45 .65 .58 .76 .42 .68 .54 78 .14 .98 .18 .97 .03 1.00 .05 1.00 108 .35 .88 .35 .88 .28 .92 .28 .92 28 .28 .92 .30 .91 .32 .90 .34 .88 203 .59 .65 .56 .69 .59 .65 .57 .68 CFA and NEO 19</p><p>23 .64 .59 .63 .60 .67 .55 .67 .55 173 .65 .58 .69 .52 .65 .58 .69 .52 5.73 8.73 5.64 8.90 5.67 8.70 5.57 8.88 2 32.83 31.81 32.15 31.02 ωA=.79 ωB=.74 ωA=.79 ωB=.74 Note. Parameters in bold were not statistically significant (p>.05).</p><p>Table 5 Completely Standardized MLE Parameter Estimates for Poor- and Satisfactory- Fitting Single-Factor Models: Agreeableness Sample 1 Sample 2 Poor Satisfactory Poor Satisfactory Items λ δ λ δ λ δ λ δ 4 .56 .69 .55 .70 .57 .68 .53 .72 64 .40 .84 .31 .90 .48 .77 .40 .84 39 .51 .74 .50 .75 .51 .74 .51 .74 14 .62 .62 .58 .66 .66 .56 .62 .62 229 .57 .68 .60 .64 .47 .78 .50 .75 19 .45 .80 .47 .78 .38 .86 .41 .83 109 .50 .75 .50 .75 .46 .79 .49 .76 59 .51 .74 .53 .72 .51 .74 .55 .70 74 .65 .58 .60 .64 .65 .58 .58 .66 104 .47 .78 .48 .77 .38 .86 .38 .86 164 .35 .88 .35 .88 .29 .92 .26 .93 44 .44 .81 .43 .82 .36 .87 .33 .89 6.03 8.88 5.90 9.01 5.72 9.15 5.56 9.30 2 36.36 34.81 32.72 30.91 ωA=.80 ωB=.76 ωA=.78 ωB=.73 Note. All factor loadings were statistically significant (p<.05). CFA and NEO 20</p><p>Table 6 Completely Standardized MLE Parameter Estimates for Poor- and Satisfactory- Fitting Single-Factor Models: Conscientiousness Sample 1 Sample 2 Poor Satisfactory Poor Satisfactory Items λ δ λ δ λ δ λ δ 35 .67 .55 .63 .60 .62 .62 .60 .64 40 .51 .74 .57 .68 .51 .74 .55 .70 200 .48 .77 .45 .80 .56 .69 .52 .73 110 .62 .62 .61 .63 .68 .54 .66 .56 15 .59 .65 .59 .65 .70 .51 .69 .52 85 .77 .41 .81 .34 .74 .45 .76 .42 50 .62 .62 .59 .65 .63 .60 .60 .64 135 .60 .64 .59 .65 .62 .62 .62 .62 55 .63 .60 .59 .65 .56 .69 .54 .71 130 .55 .70 .54 .71 .53 .72 .52 .73 45 .51 .74 .48 .77 .51 .74 .50 .75 70 .48 .77 .46 .79 .51 .74 .51 .74 7.03 7.80 6.91 7.92 7.17 7.65 7.07 7.76 2 49.42 47.75 51.41 49.98 ωA=.86 ωB=.83 ωA=.87 ωB=.84 Note. All factor loadings were statistically significant (p<.05). CFA and NEO 21</p><p>Table 7 Completely Standardized MLE Residual Correlations for Satisfactory-Fitting Single-Factor Models: Neuroticism, Extraversion, Openness Neuroticism Extraversion Agreeableness Sample Sample Sample Correlated Items 1 2 |dif| Correlated Items 1 2 |dif| Correlated Items 1 2 |dif| 71 11 .41 .33 .08 177 87 .25 .18 .07 203 23 .37 .34 .03 26 221 .25 .20 .05 37 67 .26 .23 .03 88 163 .20 .25 .05 41 76 .22 .20 .02 197 142 .25 .17 .08 188 78 -.14 -.04 .10 136 6 .20 .14 .06 197 227 .22 .26 .04 128 188 .33 .31 .02 147 237 .18 .14 .04 98 188 .24 .28 .04 87 197 -.16 -.13 .03 142 37 .24 .21 .03 37 122 .23 .25 .02 197 37 .15 .10 .05 67 122 .17 .20 .03 237 162 -.15 -.02 .13 1.08 .87 .05* 1.64 1.59 .05* 1.00 1.14 .05* 2* 1.17 1.74 2* 3.28 3.18 2* 2.00 2.28 Note. Parameters in bold were not statistically significant (p>.05); * = Mean |dif|. CFA and NEO 22</p><p>Table 8 Completely Standardized MLE Residual Correlations for Satisfactory-Fitting Single- Factor Models: Agreeableness and Conscientiousness Agreeableness Conscientiousness Sample Sample Correlated Items 1 2 |dif| Correlated Items 1 2 |dif| 104 44 .25 .32 .07 200 110 .26 .24 .02 4 64 .25 .30 .05 25 55 .28 .27 .01 64 59 .07 .05 .02 110 50 .22 .17 .05 59 164 -.13 -.13 .00 200 15 .18 .15 .03 64 109 .15 .05 .10 135 45 .20 .17 .03 14 74 .20 .20 .00 40 110 -.19 -.12 .07 64 39 .13 .08 .05 40 85 -.24 -.18 .06 229 104 -.14 -.09 .05 55 70 .15 .04 .11 74 164 .14 .18 .04 25 30 .15 .11 .04 .92 .96 .04* 1.01 .85 .05* 2* 1.84 1.92 2* 2.02 1.70 Note. Parameters in bold were not statistically significant (p>.05); * = Mean |dif|. CFA and NEO 23</p><p>Table 9</p><p>Summary of Reliability (ωA and ωB) Estimates for Samples One and Two Sample 1 Sample 2</p><p>ωA ωB Δω ωA ωB Δω N .87 .85 .02 .88 .85 .03 E .83 .77 .06 .83 .77 .06 O .79 .74 .05 .79 .74 .05 A .80 .76 .04 .78 .73 .05 C .86 .83 .03 .87 .84 .03 X .83 .79 .04 .83 .79 .04 Note. As an example, the Neuroticism ωB estimate associated with the satisfactory- fitting solution (sample 1) was calculated as 50.98 / (50.98+7.65+1.17)=.85, where 50.98 and 7.65 were obtained from the bottom of Table 2 (i.e., ∑2 and ∑) and 1.17 was obtained from the bottom of Table 7 (i.e, 2*∑).</p>
Details
-
File Typepdf
-
Upload Time-
-
Content LanguagesEnglish
-
Upload UserAnonymous/Not logged-in
-
File Pages23 Page
-
File Size-