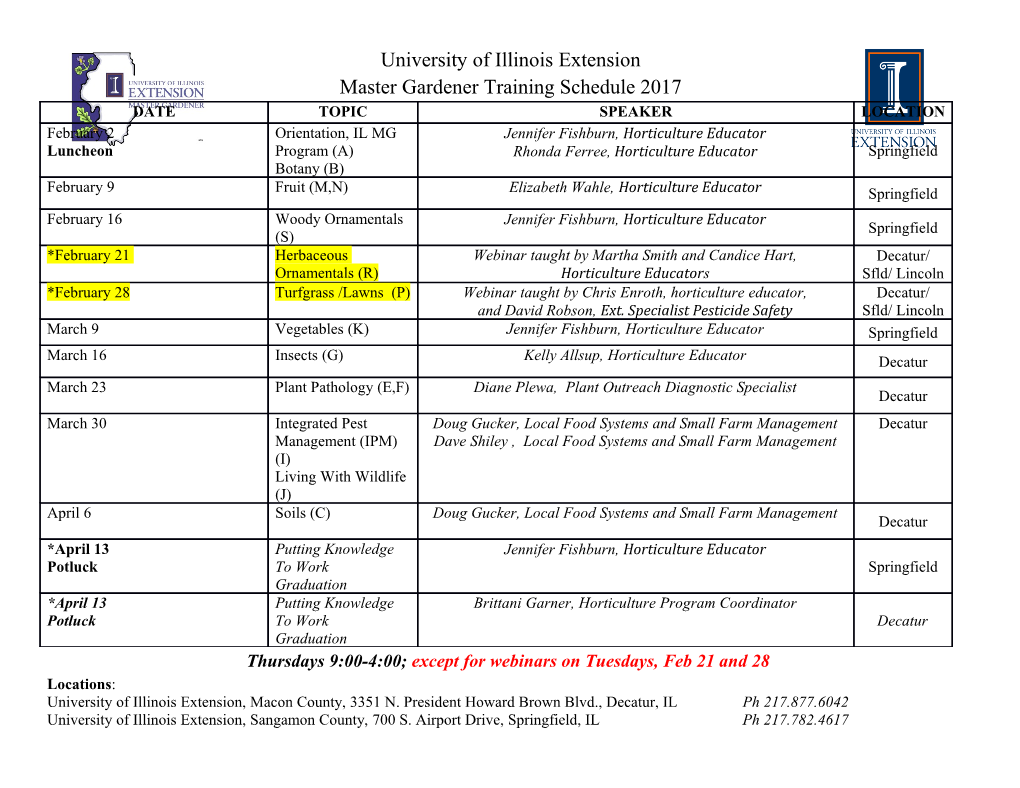
<p>Exploring new ways of Startup Performance Information Management</p><p>Enabling Startups to Manage their Performance Information within Supportive Startup Ecosystems</p><p>Tiemen Roest Master of Business Informatics Information and Computing Sciences, Utrecht University January 2016 Name: Tiemen Roest Contact: [email protected] </p><p>Title: Exploring new ways of Startup Performance Information Management. Enabling Startups to Manage their Performance Information within Supportive Startup Ecosystems</p><p>Research period: April 20st, 2015 – January 31st, 2016 Date of defence: January 28st, 2016</p><p>Utrecht University, Information and Computing sciences Master: Business Informatics 1st Supervisor: Dr. Slinger Jansen 2nd Supervisor: Prof. dr. Sjaak Brinkkemper</p><p>Host institution: Holland Startup B.V. Supervisor: Maurice Bakker Acknowledgements First I want to say many thanks to my both supervisors. Thanks to Slinger Jansen as my guide concerning the scientific part of the research, where his critical academic point of view kept me on track concerning the quality, in the semi-academic world of startup. However since he is a real startup-enthusiastic as well, he has provided many ideas and directions to consider, always with a lot of enthusiasm. Also many thanks to Maurice Bakker, my supervisor at the host company, the venture builder Holland Startup, for providing me the opportunity to do research in my favourite field, which is on startups and entrepreneurial behaviour, and at a fantastic company, which also definitely kept me motivated to finish my thesis on time. His practical point of view on startups, from his broad experience, made me aware of how startups behave and which stakeholders could be important to consider as subjects of research. Most important, I felt being a lucky guy with such enthusiastic supervisors. Also thanks to Sjaak Brinkkemper, who was willing to be my second supervisor and therefore assessing my thesis from a critical standpoint, which contributes to the quality of the research and my experience and knowledge on delivering a well-written thesis. I also must say special thanks to my girlfriend (currently also being my fiancé), Renata Karzijn, for keeping motivated all the time, and for her patience, especially when writing my thesis during the holidays was required. Also many thanks to all of my ‘colleagues’ at Holland Startup for the great time I had together with them during the period of doing my research. Robbert Jan Hanse and Rene Roskamp as experienced guys in entrepreneurship, information systems, metrics, and such. All the other guys as well, the young and brilliant entrepreneurs and researchers. All of them provided me with helpful feedback and made me stay motivated during the whole research period.</p><p>Abstract Different methods of company building exist, both traditional and non- traditional. The non-traditional method, the Lean Startup, combines iterative Agile development and Lean manufacturing, to an iterative build, measure and learn cycle, in order to enable building fast growth companies, i.e. startups. To support and guide trough the startup building process, several startup building programs (e.g. incubators, accelerators, and venture builders) evolved since the 2000 Internet bust. Monitoring and managing the risks in the startup building processes within these programs require Performance Measurement. Performance measurement has shifted the last decades from mainly Financial Accounting to a merely broader measurement of startups, referred to as Innovation Accounting. The traditional investing world can be shifted as well, by applying traditional success factors (similar to risk factors), which can be categorized on Team, Product and Market, and Startup, to a more non- traditional (Lean Startup) perspective on the startup lifecycle and its related success factors. Literature research and field research on startup building organizations and startup investors result in a framework providing insights and metrics on startup building and its performance measurement. Metrics can be divided on category into two types, General and Stage dependent. Metrics can be actionable or vanity metrics. The resulting SPIM Framework consisting of the Stakeholders, General and Stage dependent metrics, and a Framework Application Model, enables Startup Performance Information Management (SPIM) for the different stages and stakeholders in the startup lifecycle.</p><p>Contents 1. Introduction “If you can’t measure it, you can’t manage it” – Peter Drucker1 “It is the framework which changes with each new technology and not just the picture within the frame” – Marshall McLuhan2 1.1. Startup Building Organizations In 2005 the ‘accelerator program’ Y Combinator selected eight companies for their first batch of companies (i.e. startups) they would accelerate to growth, through intensive guidance (Miller & Bound, 2011). Y Combinator therefore is considered by Miller & Bound (2011) as the first official accelerator program, followed by Techstars in 2007, and from that moment in time, a lot of other accelerator programs are established, both successful and not successful initiatives. Besides accelerators, other forms of startup building programs do exist, focusing on different parts of the startup lifecycle: incubators to support only the early-stage of a startup, venture builders to cover the complete startup lifecycle (Miller & Bound, 2011; Radojevic-Kelley & Hoffman, 2012; Rohé, 2012). The three different forms of startup building can also be diversified in industry-focus (for example: healthcare startups), restrictions in their participant application (for example: university-affiliated startups) or focussed on product usage or complementary products (for example: using Microsoft) (Cohen & Hochberg, 2014). 1.2. Performance Measurement Challenges How do these accelerators and other building programs look like, i.e. in which ways are they building their startups? What criteria are used to measure how successful these startups are, to estimate, increase and perhaps predict their success? For example, how do you know if a startup is on track reaching their Product/Market fit milestone and when to act if it seems the startup does not reach its milestone? Because, as Peter Druckers’ quote says, measuring is necessary in order to manage the way you run your business, i.e. managing the path to (potential) success through realising improvements. Performance measurement systems like accounting systems have been a central topic in previous research, on both financial reporting as well as on management accounting (Davila and Foster, 2005). Davila and Foster (2005) describe how still from the early 2000s the research towards management accounting in younger (i.e. early-stage) firms has begun to receive attention. In these firms, little systems, architecture, or any kind of frameworks are in place. However, decisions on the central topics within a company, supported through management accounting systems, build up the operational framework of a company. Therefore the need for insights in better (management) accounting systems in early-stage companies (i.e. startups) seems clear. 1.3. Lack of Research To know how the young firms (in case of this research: venture builders consisting of internally located startups) look like and how to improve them, first a theoretical</p><p>1 Writer, professor (Frankfurt University) and consultant in Organizational Theory and Management</p><p>2 Philosopher of communication theory and a public intellectual background on these programs is needed, since Cocca and Alberti (2010) describe that to be able to develop a tool to do performance measurement, you need to understand the needs of a small and medium size enterprise (SME) in detail, gaining insights in their characteristics (which obviously also applies to venture builders and startups as being SMEs). However, as claimed by Cohen and Hochberg (2014), unfortunately little research is done in the field of startup building programs, both descriptive and result oriented, caused by the heterogeneity as well as lacking definitions of the startup building programs. Therefore, this research will try to provide clearer insights in how startup- building organizations like these programs look like, in an exploratory way, both on the content (the startups and their building methods and techniques) as well as the environment (i.e. ecosystem) they operate in. Obviously also a lot of startups are built without the support of these programs, but this research will focus on those who are particularly built within the organized building programs. 1.4. Scientific Relevance Obviously, as mentioned, the performance and measuring this performance at the startups being build within a program is important, both for the existence and performance of the startup-building program itself. As research shows, building programs (thus venture builders and investors too) interpret the performance of startups on several company portfolio criteria, in order to know which startups can apply for their program and are worth the investment (Chang, 2013; Megginson and Weiss, 1991). However, still low growth small or medium sized businesses do not use as many customer metrics for example, and their performance management systems are often not based on best practices from the literature (Cocca and Alberti, 2010; Ates et al., 2013). Steve Blank (2012) refers to Evidence Based Entrepreneurship and Investor Readiness to describe respectively the importance of the knowledge of how a startup is performing, related to the knowledge where startups should be in order to meet the investing criteria. Previous research on startup success is, among others, done by Cann, Brinkkemper, and Jansen (2012). They have done an extensive research towards the key decisions in the startup phases that might have contributed to success within sixteen large Dutch software companies. Although they did include theory on startup phases, success factors and investing criteria, their research focuses mainly on the key decisions of the entrepreneurs of traditionally built companies, concerning shaping the company, the product development, entering the market and going international, influenced the company success. Marshall McLuhan as quoted above the start of the introduction was right: changes in technology (and methods) from, for example, traditional to non-traditional, needs new frameworks. Therefore, the main goal of this research is to provide more insights in the startup ecosystem criteria for performance measurement, tied to the different startup phases, from both the information management and performance measurement points of view, in a Startup Performance Information Management (SPIM) Framework. The underlying goal of this research is to contribute to transparency and knowledge within startup ecosystems, to in the end strengthen the relationships between stakeholders and startups within those ecosystems. Which particular criteria need to be considered as important by startups and investors, will be based on literature and evaluated in the environment of the ecosystem. Research on the investment decision process of Business Angels (BA’s) shows that criteria can be unknown or ignored when assessing a startup, where BA’s tend to rely on their gut feeling instead of relying on a formal startup evaluation approach, which makes the evaluation process quite unclear (Maxwell, Jeffrey & Lévesque, 2011). The potential economic impact of startups makes that same problem concerning to Maxwell et al. (2011) whereas they describe, based on other research, for example 95% of the entrepreneurs in Canada fail to attract BA funding, similar as observed in the U.S. an the U.K.. Maxwell et al. (2011) ascribe that substantial amount of failure to misunderstanding of the decision process. Also research on the investment decision process of Venture Capitalists (VCs) shows entrepreneurs and VCs are facing similar decision process knowledge problems. As Miloud, Aspelund and Cabrol (2012) describe through strong findings of actual Venture Capitalists’ (VC) valuations, for example the factors Industry structure, Founder/Team and Network, consisting of several specific criteria, are the most important ones, unfortunately they have not found a relative importance yet between those factors. Therefore they suggest further research as required in order to use these highly relevant findings to become beneficial to startups and their investors. 1.5. Scope The framework will consist of information and data both for venture builders as well as for external stakeholders (in this research: investors), focused on Software-as-a-Service (SaaS) startups. It mainly aims at quicker and easier provision of useful insights in the company portfolio of a venture builder (which consists of a management team and individual startups) as well as improving the assessment and prediction of success of the startups involved in those startup-building programs, which is relevant to investors as well. Therefore, the venture builder can be seen as the primary stakeholder, where the investors are the (most important) secondary stakeholders. Baum and Silvermann (2004) describe financial intermediaries such as VC firms as the dominant ones shaping the environment (i.e. ecosystem) the startups (or venture builder) operate in. To put the importance of the different roles into perspective, the research also will describe how the level of dominance of a particular stakeholder depends on the stage of a startup. Although the framework tries to improve the information and data management (performance measurement) in and around all kinds of (SaaS) startups, keep in mind that the framework is not built to serve as a ‘one-size-fits-all’ solution. Also, the performance measurement cannot be seen as a smaller version of tools being already developed for large enterprises, like Cocca and Alberti (2010) warn for in the context of inappropriate design of SME tools. Therefore it will focus on startups particularly in the SaaS domain. 1.6. Research Structure The structure of the research will be build upon one main research question, separated into three parts with six research sub-questions. The main research question:</p><p>The first part is the startup part, necessary in order to gain insights in the characteristics of startups and the venture builder ecosystem they operate in. The first question to answer is what startup building methods do exist (SRQ 1). Followed by how a startup lifecycle is structured (phases and stages) (SRQ 2). The second part concerns the descriptive question on how the environment of a startup looks like, the venture builder ecosystem (SRQ 3), elaborating on the stakeholders and their relationship with a startup being built within a venture builder. The third part will focus on the startup performance information, the corresponding metrics and existing startup information systems, which might support the startup building process within the venture builder ecosystem. It starts describing why and how SPIM supports startup building (SRQ 4). Followed by describing which existing information systems could support SPIM (SRQ 5). Each of the chapters concerning the research questions will close with a summary on those particular chapters. All previous questions sum up to a whole description of startups and their ecosystem performance information demand, where the remaining question is how that information can be modelled into a SPIM framework, consisting of both a SPIM stakeholders and metrics overview, as well as a related application model (SRQ 6). </p><p>2. Research Method After the topic is introduced in the previous chapter, this chapter will elaborate on which research methods will be used. A schematic overview of the subsequence of the different methods is depicted in figure 1, designed according to the Process Deliverable Diagram modelling technique developed by Weerd and Brinkkemper (2008). The research will first start with an exploratory literature research to provide a theoretical background on startup building, the venture building ecosystem, funding, success criteria, and performance information management. This theory will provide information on framework requirements, which will be described in the framework requirements section. The requirements will be completed through an extensive field research in two fields. First, (internal) interviews in the field of venture building itself: at venture builders, incubators and accelerators. Second, (external) interviews on other startup stakeholders. Due to limitations of the research period, the scope of these external stakeholders only includes the investors as a target group, whereas Business Angels (informal investors) and Venture Capitalists (formal investors) are selected as focus groups within the investor ecosystem, therefore excluding other forms of informal investing, like family & friends, crowdfunding, etc. (see chapter. 3.5 for a more detailed view on a startup ecosystem). Besides the limitation mentioned, the investors are selected due to their important (or even necessary) role in the startup lifecycle, since financing events are necessary to startup survival and do have a positive effect on firm growth as well as predicting growth (Davila, Foster & Gupta, 2003). The qualitative interview results will be transcribed, coded and used as a basis to formulate a grounded theory, based on a comparison method, comparing the different stakeholder groups to each other (Boeije, 2002; Singer, Sim, & Lethbridge, 2008). The field research will be executed in an iterative way: the findings will be processed in the framework during the field research.</p><p>Fig. 1 - Research method</p><p>3. Theoretical Background To start providing the background of this particular research, this chapter will provide the existing relevant theory on venture building and the related information management and performance measurement. The theory will be discussed in three separated parts: first on what a startup and venture building actually is, secondly on business information management and thirdly why business performance measurement is considered as crucial in managing venture building. 1.7. Defining a Startup Before discussing startups and their building process, a definition of a startup is needed. Different definitions are used in the recent years. Blank and Dorf (2012) define a startup as “an organization formed to search for a scalable and repeatable business model”. Graham (2012) defines a startup as “a company designed to grow fast”. Both terms in essence relate startup to growth, through scaling and repeating. Seen from a sales perspective is selling something to a big market to reach rapid growth what a startup differentiates from a traditional business (Graham, 2012). Blank covered the difference in an overview, see figure 2, which shows the transition, or difference, between a startup, which is considered in the experimentation phase, working on a scalable business model, to a revenue generating company. The main parts changing are the main company focus, finances and human resources.</p><p>Fig. 2 - Startup to Company transition process (Blank, 2012) Startup Commons, a global non-profit initiative to support governments in startup ecosystem building, managing, measuring and benchmarking, also contributes to startup insights by (among others) providing the overview as shown in figure 2 (Startup Commons, 2015). The figure shows how a startup evolves from Ideation (identifying a potential idea/product) to Establishing (achieved great company growth). These stages will be discussed in more detail when discussing several similar startups lifecycle descriptions in chapter 3.2. The bumpy line symbolizes the major entrepreneurial factor ‘uncertainty’ over a substantial period of time. As described in the introduction, this research aims at providing more clarifying insights at what happens during that period and how to act while under a certain level of uncertainty, both applicable to internal as well as external stakeholders, which will be elaborated on in the pivot section in 3.3.1.</p><p>Fig. 3 – Startup Development phases (Startup Commons, 2015) Defining entrepreneurship will give some additional insights in the background and effort related to building a startup. Gelderen, Thurik and Bosma (2006) refer to the person undertaking the activities of creating a business as the nascent entrepreneur, where nascent entrepreneurship is the definition for the effort needed to found a business. Shane and Venkataraman (2000, p.218) define entrepreneurship as “the scholarly examination of how, by whom, and with what effects opportunities to create future goods and services are discovered, evaluated, and exploited”. </p><p>1.8. Startup Building Methods</p><p>3.1. Traditional Methods Two traditional used building methods, originating from software development, are the Waterfall development method and the Agile development method (Larman & Basili, 2003) The main difference is the iteration frequency. The waterfall method as a stepwise approach, avoids processing changes during (at least) the first development round (Larman & Basili, 2003). The opposite is the agile development approach, functioning as an iterative approach, focussed on processing and communicating feedback every step of the process, to adapt rapidly to changing consumer requirements, in order to do incremental delivery of the software (Fowler & Highsmith, 2001). The agile approach is important in the transition to the recent (iterative) lean development methods, as will be disused in the next section. Advantages and disadvantages of both methods are described in table 1. The overview is included in order to have a better understanding of the history of the recently developed methods. Waterfall (stepwise) Agile (iterative) Advantages Documented requirement gathering Less time & costs on requirement gathering </p><p>Customer feedback simultaneously with development Disadvantages Changing requirements require Unstructured documentation changes Table 1 – Waterfall Development versus Agile Development (Fowler & Highsmith, 2001; Larman & Basili, 2003)</p><p>3.2. Non-Traditional Method: Lean Startup Royce (1970) already figured out that, besides analysis and coding, the development process of large software systems require a lot of extra steps, contributing to waste of effort rather than the main goal: "[While] many additional development steps are required, none contribute as directly to the final product as analysis and coding, and all drive up the development costs” (Royce, 1970, p.1). That is what the most recent company building methodology, the Lean Startup, also is about. The term Lean was used for the first time in the Japanese industry, as in Lean manufacturing. A lean manufacturing system is focused on value creation for the end customer, considering the expenditure of resources for other goals, as waste. This lean manufacturing originates from Ohno, who introduced the Toyota Production System in Japan, aiming primarily at cost reduction (or, waste elimination), introduced in the U.S. in 1984 (Shah and Ward, 2007). However, this lean method is focused on manufacturing. In the process of company building the lean method also evolves, when in 2005, Steve Blank published his book called ‘the Four Steps to Epiphany: Successful Strategies for Products that Win’. He introduced the new methodology on company building, called Customer Development. This methodology is, like lean manufacturing, focussed on only using resources to reach you primary goals, in the case of company building defined by blank Blank as finding primarily the right market, through Customer Discovery and Customer Validation in an iterative way (Blank, 2006). Eric Ries further defined the Lean Startup process, combining customer development (Steve Blank, The Four Steps to Epiphany), Agile software development methodologies, and Lean manufacturing practices into a framework for developing products and businesses quickly and efficiently (Croll and Yoskovitz, 2013, p.12). He published his findings in ‘The Lean Startup: How Today's Entrepreneurs Use Continuous Innovation to Create Radically Successful Businesses’ (Ries, 2011). This renewed Lean Startup building approach methodology created a whole new movement in the startup world, where even business schools adapt their curricula to teach this particular approach (Blank, 2013). Core of the method is ‘Build -> Measure -> Learn’—the process by which order you do everything, from establishing a vision to building product features to developing channels and marketing strategies, as shown in figure 2. The faster your organization iterates through the cycle, the more quickly you’ll find the right product and market. Main part of that cycle building the Minimum Viable Product (MVP), necessary to perform what Eric Ries calls ‘innovation accounting’, which helps you objectively measure how you’re doing (Ries, 2011). In addition to that cycle, the Lean Analytics methodology is developed, as a practical guide to quantify your innovation, by providing metrics for the different stages, “getting you closer and closer to a reality check—in other words, to reality itself” (Croll and Yoskovitz, 2013, p.13). Thus within the Lean Startup cycle, Lean Analytics focuses on measuring the outcome of the stages (notify that not only the measure-stage is captured by Lean Analytics). As the methodology says: “If you measure better, you’re more likely to succeed” (Croll and Yoskovitz, 2013, p.12). This chapter covered the evolution of the different building approaches over time. To see how this latest developments work in practice, the next chapter will describe the lifecycle of a startup, including the different existing lean-based lifecycle frameworks. 1.9. Startup Lifecycle How does a startup life cycle look like? Which stages contribute to fast growth, in order to be a startup according to the startup definitions? Which phases can a startup life be divided in? This section will first elaborate on the stages, or, the frameworks representing the stages, after which will be elaborated on the three main phases of a startup.</p><p>3.3. Startup Lifecycle Stages Different frameworks are created over the last decade (see figure 4): Maurya (2010) created the Lean Canvas, followed by the Lean Startup by Ries (2011), McClure (2007) created the five Pirate Metrics stages AARRR, Ellis (2009) the three-tier Growth Pyramid, and most recently Croll and Yoskovitz (2013) the five Lean Analytics Stages. As shown by the framework overview below (figure 5), there is a lot of overlap in the existing frameworks. An important part missing, due to the design of this particular figure, but worth to mention, is the iterative way (characteristic of the Lean approach) in which all the steps of the different methods are executed.</p><p>Fig. 4 – Startup lifecycle/building frameworks (Croll and Yoskovitz, 2013) Another relevant startup-building framework is developed in the Startup Genome project by Marmer, Herrmann, Dogrultan, Berman, Eesley & Blank (2011), who created four top level stages called the Marmer Stages, based on the Four Steps to Epiphany, developed by Blank (2006). The next section will elaborate on the reason this framework is included and its content, first an overview of these particular stages will be provided (figure 6).</p><p>Fig. 5 – The four Marmer Stages (Marmer et al., 2011) The researchers of this research have created a new building methods overview, based on figure 5, to summarize all the frameworks as described above in one picture (see figure 7). Also, three main phases (represented by three milestones), according to Maurya (2012), are added at the right side of the picture, to increase the overall understanding of the several stages. And the Exit is included as a general ‘end-stage’, like is mentioned as being the last step in figure 5. Where Exit means: going public through an Initial Public Offering (IPO) or being acquired by another company (Davila et al., 2003). The picture immediately shows that milestones do fit to some of the frameworks, but not to all of them (especially Sean Ellis’ Growth Pyramid). Due to this inconsistency can be concluded that the content of the startup stages and target phases are not always as consistent relative to other frameworks as their names do expect.</p><p>Fig. 6 – Overview of all the relevant lifecycle frameworks (stages & phases) Before discussing the three main milestones of the startup lifecycle, two of the six frameworks will be highlighted in detail here: first the Lean Analytics framework, followed by the Startup Genome framework. The reason for highlighting in particular these frameworks are chosen here based on their goals and novelty, in line with this research). </p><p>3.3.1. Lean Analytics stages The Lean Analytics framework, developed by Croll and Yoskovitz (2013), focuses on startup performance measurement in particular, i.e. startup analytics (therefore, this framework will be used for defining metrics in the remaining theory). Lean Analytics consists of five stages (as depicted in figure 2). (1) The goal of the Empathy stage is to identify a need, which can be solved, and where people tell they are willing to pay for a solution. Most of the results in this first stage are qualitative, some of which can be quantified. Part of the result of this stage is also known as ‘Problem/Solution Fit’ (Maurya, 2012), which will be discussed later. (2) The Stickiness phase aims at proving that a proposed solution (a MVP, not an end product), solves the problem and keeps people coming back. This stage can be quantified through measuring engagement. During this stage, you are reaching ‘Product/Market Fit’ (Maurya, 2012), which will be discussed later. (3) The next stage is Virality, which means the user growth from existing users to new users. Measuring that virality can tell the stakeholders whether user acquisition and growth can be accomplished. (4) The Revenue stage is about the money a user (customer) brings in. The core equation is the money that customer brings in minus the cost of acquiring that customer. For example: SaaS businesses can use the calculation resulting in the CLV:CAC ratio, the Customer Lifetime Value versus the Customer Acquisition Cost. (5) The last stage is Scale. From now on you can focus on large growth, for example by entering new markets. From now on more metrics at the same time needs to taken into account. Set up metrics on strategy, tactics and implementation, aligned to a consistent set of goals. The exact metrics for the particular Lean Analytics stages will be elaborated on in more detail in chapter 3.6 when discussing startup performance measurement.</p><p>3.3.2. Marmer stages The second framework worth highlighting is the Startup Genome project framework, i.e. Marmer stages, consisting of four stages (see figure 2)(Marmer et al., 2011). This framework stages will be used in the remaining for the reason of simplicity and clarity of both the naming and content of the stages, compared to the other frameworks. Stage 1. It starts with Discovery of the problem: is the identified problem a meaningful problem and is anybody interested? That is what is called the Problem/Solution Fit. The discovery stage also covers the development of a MVP, Minimum Viable Product: a product with just enough functionality to test assumptions. An alternative definition on MVP is provided by Maurya (2012), as: “the minimum feature set that let’s you start learning about customers”. Stage 2. The discovery phase is followed by Validation of the proposed solution, resulting in feedback and money or attention, in order to refine the product and do first analytics. This validation is the search for Product /Market Fit. Stage 3. In the Efficiency phase the business model is improved to increase efficiency, through, for example, creating viral (i.e. self-sustaining) growth, according to Blank (2006, p.16) as ‘customer creation’: “creating and driving end user demand”. Stage 4. The Scale phase is all about big ‘aggressive’ growth, through, for example, massive customer acquisition and hiring the first employees, which Blank (2006, p.16) defines as ‘company building’: "transitioning the organization from one designed for learning and discovery to a well-oiled machine engineered for execution”.</p><p>3.4. Startup Lifecycle Phases As shown in figure 3, Problem/Solution Fit (P/S Fit), Product/Market Fit (P/M Fit) and Growth are in general the three main milestones (or targets) a startup focuses on, as being three phases a startup is in. These milestones are important in the research since they represent measurable outcomes, which is a major part of this research. The first target to reach is Problem/Solution Fit: validating “there is a problem worth solving” (Maurya, 2012). Since Maurya (2012) argues that Problem validation, Solution validation and Discovery cover that same validation process, the Problem/Solution Fit in figure 3 can be considered as reached at the point in time depicted by the first horizontal border. The Product/Market Fit phase is about “building something people want and validate your business model” (Maurya, 2012). Since Retention, MVP iteration, (partly) Stickiness and Validation align to the defined phase of Product/Market Fit, the second milestone in figure 3 is drawn after this four phases. After Product/Market Fit is found, startup growth, scaling the startup, is the next phase. (Maurya, 2012). More on the three phases, including their associated techniques will be discussed in more detail here. </p><p>3.4.1. Problem/Solution Fit A startup ideally starts with a problem, not primarily an idea; therefore you need to identify the problem behind the idea to be able to work on a real problem (Maurya, 2012). This is done through the ‘Discovery’ of a potential customer segment (Marmer et al., 2011), also defined as Customer Discovery (Blank, 2006). The main part of Customer Discovery is hypotheses testing: state a customer and problem hypothesis and get in contact with these potential customers to test through interviews whether the hypothesis holds or does not hold (this is called: an experiment). Which potential customers to test, are stated in the ‘diffusion of innovations’ as the ‘Early Adopters’ (Rogers, 2010). Rogers already in 1963 started with his research and founded the theory of how innovations are adopted by a potential market. See figure 6 for the innovation adoption theory distribution and lifecycle.</p><p>Fig. 7 – Innovations Distribution and Lifecycle (Rogers, 2010) Thus, in this phase a startup needs to identify and validate who the innovators will be. If you have executed enough experiments to say you have significantly identified your customer segment, their problem, and a vision on a possible solution, Problem/Solution Fit is reached (Maurya, 2012; Blank, 2006). Interview results are obviously qualitative, where chapter 3.6. on performance measurement will describe metrics to validate these results quantitatively. Besides identifying the customer segment and potential solution, the P/S Fit can be used to build the test group before launching the MVP in de next phase, and deciding what goes into that MVP (Croll & Yoskovitz, 2013).</p><p>3.4.2. Product/Market Fit As the name says, this is the search for validating your product in the market you are targeting (Maurya, 2012). In the previous phase, the startup identified the customer segment and a possible solution. This solution needs to be validated in the market, the reason why the stage similar to this phase is called the ‘Validation phase’ (Marmer et al, 2011). Therefore, industry expert and senior investor Andreessen (2007) states the following: “The only thing that matters is getting to Product/Market fit”. Also Blank (2012) highlights the importance of Product/Market fit in the startup building process. As can be seen in 3.3.1., Ellis (2009) defines Product/Market fit as the basis of a startup. To test whether the startup has reached that validation of Product/Market fit, you need to know your customer segment as defined in the Problem/Solution fit stage and you start building your MVP, as described in 3.3.1. as “the minimum feature set that let’s you start learning about customers” (Ries, 2011; Maurya, 2012). Both industry experts and venture capitalists Feld and Horowitz agree on 4 P/M fit myths when discussing P/M fit for SaaS companies (Feld, 2015): - Myth #1: Product market fit is always a discrete, big bang event - Myth #2: It’s patently obvious when you have product market fit - Myth #3: Once you achieve product market fit, you can’t lose it. - Myth #4: Once you have product-market fit, you don’t have to sweat the competition</p><p>3.4.3. Growth The growth phase consists of Efficiency and Scale (Marmer et al., 2011), quite similarly to respectively the Viral and Scale stage of Croll and Yoskovitz (2013). Ellis (2009) uses the broad description ‘Stacking the odds’, although this one will not be used in this research due to its unspecific character. Research shows that assuming too early Product/Market Fit is found, is one of the leading factors for failure. Marmer et al. (2011) define this problem as pre-mature scaling: assuming you have found Product/Market Fit and go to the Scale phase, or in that case the Growth phase. Marmer et al. (2011, p.5): “ Premature scaling is the most common reason for startups to perform worse. They tend to lose the battle early on by getting ahead of themselves.” This same problem is defined by Croll and Yoskovitz (2013) as pre-mature virality: assuming there is Product/Market Fit and leaving the Stickiness phase going to the Virality (i.e. Growth) phase. Deloitte and THNK (2015) also found out in a research among 400.000 startups, that scale-ups, top-performing startups with 10M dollar revenue take twice the time a startup takes on entering a market, in order to be better prepared for growth. Therefore, this research considers the Efficiency stage as defined in Marmer et al. (2011) as a necessary part of the Growth phase , where, as chapter 3.3. describes, the business model will be “improved to increase efficiency, through, for example, creating viral (i.e. self-sustaining) growth”. This first stage in the growth phase therefore focuses on making the startup ‘growth- ready’, in order to avoid pre-mature scaling. Two methods to stabilize/ the business model for sustainable growth is through choosing the right ‘Engine of Growth’, a concept defined by Ries (2011), consisting of three possible engines (depending on the business model): the Sticky, Viral and Paid Engine of Growth. Sticky focuses on retention, keeping customers coming back on the long term, Viral focuses on the product advertising itself, through word- of-mouth, and Paid focuses on using the value created by new customers to pay acquisition for new customers (Ries, 2011). Croll & Yoskovitz (2013) are approaching the same kinds of engines through their Virality phase, distinguishing Inherent virality, Artificial virality and Word-of-mouth virality. For the three kinds of virality, they also included some examples. Inherent virality for example happens when a business promotes users to share specific content with colleagues, who also can install the software to have a better view of the content. Artificial virality for example is when inherent virality is combined with rewarding its’ users to share the content, which could be additional functions for promoting a service on social media. Word-of-mouth can use blogs for example, to spread the word. The Scale stage within the Growth phase just focuses on large growth (Croll and Yoskovitz, 2013), as Blank defines as (2006, p.16) “transitioning the organization from one designed for learning and discovery to a well-oiled machine engineered for execution”. This stage will not be elaborated on in detail.</p><p>3.5. Pivots As mentioned earlier, the phases of the four different Marmer stages are, as the Lean Startup approach prescribe, iterative: based on measuring and learning. This same approach therefore applies in the search for reaching the main milestones in the three different main phases. Through using that iterative approach, based on information (qualitative) or data (quantitative) measurement, a startup team is able to make a (fundamental) change in the business, seeing that a hypothesis they formed about one of the topics in the definition, is based on the wrong assumptions. This is what is called a ‘pivot’. A pivot is defined by Ries (2011) as “a structured course correction designed to test a new fundamental hypothesis about the product, strategy, and engine of growth”. As written, knowledge about pivots is necessary, because firstly, this explains the bumpy line figure 2 showed in section 3.1., secondly, is important to keep in mind while using and reading schematic figures (like figure 3 and figure 4) which are a model of how reality occurs and thirdly, provides an understanding in the necessity of performance measurement to avoid making fundamental mistakes during startup building, i.e. reducing risk. The importance of making those pivots, i.e. adapting to the information/data you received through measurement, is one of the major findings on startup success of Marmer et al. (2011, p.5): “ Startups that pivot once or twice times raise 2.5x more money, have 3.6x better user growth, and are 52% less likely to scale prematurely than startups that pivot more than 2 times or not at all.” Like the definition of Ries provided above, a pivot in this context is defined as “a major change in the business. For example a new market or a new value proposition.” (Marmer et al., 2011, p.25). The next question is, what measures (i.e. metrics) are needed for monitoring whether a startup reaches the necessary three milestones described in the previous section, in order to manage the transition through the different stages from being a startup towards an established company? Before discussing those measures, the stakeholders (startup building programs and the ecosystem they operate in) who demand for access to information on those measures, will be discussed in the next two chapters. </p><p>1.10. Startup Building Organizations As mentioned in the introduction, little research is done in the field of startup building organizations, and Cohen and Hochberg (2014) do also claim that definitions on startup building organizations are unclear. This section tries to elaborate on the different forms of organizations that support startup building (the incubators, accelerators and venture builders), followed by a short description of the ecosystem where a startup operates in. History Before 2000, in the 80’s and 90’s ventures were built with help of research labs. After the Internet bust of 2000, most angel investors reduced their investments to decrease their risk. However, the starting ventures still needed their capital for launching and growing their business. This gap was the trigger of the emerging of investment firms, known as accelerators. These accelerators were led by experienced and successful entrepreneurs, to coach and mentor new ventures to reduce risk and failure rate (Radojevic-Kelley & Hoffman, 2012). Global examples of accelerators are TechStars in Colorado, Y Combinator in San Francisco, Rocket Internet in Berlin and StartupBootcamp in Amsterdam. Incubator or Accelerator As mentioned, there is little descriptive research on building programs, resulting in confusing definitions of incubators and accelerators (terms are even used interchangeably) (Cohen & Hochberch, 2014). However, this section tries to elaborate on the differences between both forms. An incubator in majority of the cases is an organization providing office space to starting ventures, and in some occasions access to support and/or a business network; a facilitating role towards ventures considered started from scratch, with the ability to grow (the fragile phase) (Miller & Bound, 2011). Accelerators however, are fully committed organizations, as a partnership, through investing in business and product development (Fishback, Gulbranson, Litan, Mitchell & Porzig, 2007). Accelerators are defined by Cohen and Hochberg (2014, p.4) as “a fixed- term, cohort-based program, including mentorship and educational components that culminates in a public pitch event or demo-day”. Cohort-based since they process batches of a pre-defined maximum number of startups per program period. Demo day is the final program day, designed for investors to come and see the startups, where founders can pitch their startup resulting in a chance to launch their product and service (Miller and Bound, 2011). One of the critics on the accelerator approach is that finishing a 90 days program is not an insurance that the company is strong enough to survive on its own, resulting in failure (Miller and Bound, 2011). Therefore, another program is created, the Venture builder. Venture builder The other form of building companies is through a venture builder. It is an extended form, supporting both incubation and acceleration, and even beyond acceleration, towards growth. Venture builders are the ones providing ideas, supposed to be built by other persons; therefore these persons will become co-founders of the starting venture, i.e. startup. You closely work with/for them, in an entrepreneurial environment, for an unknown period of time (Rohé, 2012). Venture builders do not only incubate, they also accelerate and go even further, beyond acceleration to growth. The Management Team (MT) within such a venture builder can be considered as the primary stakeholder of a startup. Definitions on building organizations like a venture builder are unclear as Cohen & Hochberch (2014) found. A new definition therefore on a venture builder could be: an organization with startup ideas designed to guide entrepreneurs in building a startup for an unknown period of time. Table 1 provides a complete overview of the differences. Incubator Accelerator Venture builder Idea You bring your idea and Investing cash for equity in In the majority of the cases, ideally already your return (usually max. € it’s not your idea and not team. 20k). your company: it’s theirs. Resources They give supporting Sometimes providing You’re a share incentivized resources, maybe some Infrastructure in a CEO/COO/etc. money, infrastructure program-like setup. You work for/with “them” in and advice trough a a Entrepreneurial mentor network. environment. Time period Runs (usually) a limited Runs a limited period of Runs for an indefinite period period of time. time. of time. Example Harvard Innovation-Lab Y Combinator Rocket Internet Table 2 - Different organizational forms of venture support/building (Rohé, 2012) 1.11. Startup Building Ecosystem</p><p>3.6. Ecosystem Overview Observations at the research host company as case company show the structure of the ecosystem a venture builder operates in (figure 7). Universities are involved to recruit co- founders from, Corporates to cooperate with regarding to corporate entrepreneurship, Investors to receive investments from, a local Economic Board to receive investments and other resources from, the (primarily) local Government supporting local ecosystems and defining and regulating legislation on entrepreneurship, the Suppliers are partners supplying resources necessary for startups to grow (for example: a developer team) and finally the Customers are the individuals or businesses required to buy the startup products or services. As described in 3.4., the primary stakeholder of a startup is the venture builder Management Team. The secondary stakeholder, the investor, will be discussed in the next section.</p><p>Fig. 8 – Venture Builder Ecosystem overview 3.7. Investors As mentioned in the introduction, this research will, besides the Venture Builder as the intern stakeholder, only focus on one external stakeholder group in the ecosystem, namely the Investors. This section will describe why they play such an important role, what types of investors are involved in the startup-building field and the role of the risk factor. Before diving into those details, some background from a startup perspective. </p><p>3.7.1. Investments and Startup Growth Hellman and Puri (2000) have found that venture-backed companies are more innovative companies compared to non-venture-backed companies. They have made a distinction between firms as innovators, which are “the first to introduce new products or services for which no close substitute is yet offered in the market”, and firms acting as imitators, which are “also engaged in relatively new products and technologies, but they are not the first movers in their markets, and therefore tend to compete on aspects other than innovation” (Hellmann & Puri, 2000, p2.) The results suggest that innovators rather than imitators do receive funding. They also found that funding is positively related to shorten the time to market, especially for the innovators. Davila et al. (2003) found that VC’s involvement increase reputation and skills for a startup. The signal of passing through the screening of a venture capitalist and receiving funding is perceived powerful by the stakeholders in the ecosystem, also inside the startup itself. Result of these positive factors is easier attraction of high quality employees, increase in the number of customers as well as an advantage in making deals with external parties. Based on a database of 494 companies in Silicon Valley, Davila et al. (2003) have found that there is a significant difference in growth of startups without venture capital, compared to startups that received venture capital (see Figure 9). The positive consequence of receiving funding is important to keep in mind. Notion: since it is hard to define growth according to revenue or even profit increase for early-stage startups, growth in their research is defined as the increase of people working for a startup (Davila et al., 2003). </p><p>Fig. 9 – Startups with venture-capital vs. Startups without venture-capital (Davila et al, 2003)</p><p>3.7.2. Investor Types Hellmann and Puri (2000) distinguish six different types of funding: self-financing entrepreneurs, corporations, banks, government, angels (wealthy private individuals) and venture capitalists. This section consists of two parts: it starts with the first four types which will be discussed shortly, mainly based on Hellmann and Puri (2000), followed by the latter two types, the business angels and venture capitalists, which will be discussed more in detail, Self-financing is financing from the founders, their families and their friends. Corporate investors may also be in good position to invest and add value, due to for example their knowledge about a particular market. However, Hellman and Puri (2000) also mention that incentive problems may occur, and bureaucracy of a corporate is frequently seen as a reason for less usefulness of such a corporate investor. Here the distinction between financial and strategic types of investors comes into play (Hellmann, 2002). The incentive problems occur when corporates invest mainly for strategic reasons, where financial returns are not that important as for other types of investors. To provide an insight of how large the portion of strategic investors is, Hellman (2002) describes a survey done by Yost and Devlin (1993), who found that 93% of all the corporate investors invest for strategic returns as their main objective. Banks can be seen as a more infrequent provider of funding (Hellmann & Puri, 2000). They invest through loans and sometimes through ‘wholly owned subsidiaries’. Hellmann and Puri (2000) point out that regulatory constraints are the main reason why banks are more conservative investors. A special type of bank is an investment bank: they are particularly focussed on future transactions (an IPO for example). Also government do funding, but typically they are entirely passive and their funding mainly consists of grants (Hellmann & Puri, 2000). Now the first group stakeholders are described, the ones less important for this research, the two other stakeholders will be described in more detail: the Angel investors (interchangeably terms used in literature are Private Investors or Business Angels, which will be used from now on) and Venture Capitalists. </p><p>3.7.3. Business Angels vs. Venture Capitalists This section will start shortly describing both groups, based on previous literature. First the Business Angels, followed by a description of the Venture Capitalists. The second part will elaborate more on the differences through comparison. Business Angels Business Angel investors independently diversify their wealth through investing in new companies. They act in a less formal way compared to the institutional investors, for example, they mostly do not have any staff for supervising their investments and to find deals they mostly rely on their pre-existing networks. And for many of them it’s not their main professional activity. Important to keep in mind that the group of Angel investors can be considered as a heterogeneous group, therefore drawing conclusions is a delicate process. Venture Capitalists Hellman and Puri (2000) provide eleven characteristics of Venture Capitalists, the relevant ones in this research context will be highlighted here. . Venture Capitalists are full-time professional investors who invest for their partnership funds . Venture capitalists tend to closely follow the technology and market developments in their area of expertise in order to stay in the deal flow and to be able to make an informed investment decision . Before making an investment, they carefully scrutinize the founders and their business concepts . They also continuously monitor their companies, both formally through participation at the board level and informally . As monitors and through their access to private information, like banks, they can help provide certification to outside stakeholders . They can provide valuable mentoring and strategic advice for the entrepreneurs and they frequently assist companies in providing business contacts and recruiting senior managers . They often take an active role in guiding the exit decision, such as influencing a company’s initial public offering</p><p>Similarities and Differences Ehrlich, Noble, More and Weaver (1994) did a literature review focused on similarities and differences between Business Angels (BAs) and Venture Capitalists (VCs). More recent research is from Maxwell et al. (2011), on Business Angels early stage decision-making. This section will describe the similarities and differences between the two types of investors. Similarities they found in theory are first on the market or technology type, where they tend to invest both in markets or technologies they are familiar with. And second on the time-period, where both BAs and VCs prefer to liquidate their investment in period of five to ten years. Both of the groups invest money in exchange for equity (Ehrlich et al., 1994; Maxwell et al., 2011). Differences they found were among others in stage and size of investment, the ownership of the investment money, geographic location of the firm, and the motivation to invest in a particular company. We will discuss those differences in more detail, mainly the investment stage and size. Investment stage First, the investment stages. Before reading the stage descriptions, be aware that definitions of stages differ in literature (similar problem as it is the case in the various startup lifecycle descriptions in 3.3.). BAs invest much more frequent, twenty times as many as VCs do. Therefore, they invest more money accumulated, but they tend to invest much more smaller amounts per investment, and in a earlier stage than VCs do, where in later stages the perceived risk is much lower than in the early stage (which factor will be discussed in more detail later on) (Maxwell et al., 2011). Ehrlich et al. (1994) also found that the company stages BAs tend to be involved in through funding are mainly the seed and startup stages. Also the U.S. Angel Capital Association (2014) figured out that indeed most business angels (or, angels in groups) mainly invest in the early stage of a company, a large portion in the seed stage and much fewer investments in the later stages.</p><p>Fig. 10 – Preferred Business Angel Investment stages (Angel Capital Association, 2014) Closing remark on the investment stages is concerning the naming of the stages: the stages are consistently different in the (traditional) investing field (like in figure 9), compared to the stages in the (non-traditional) Lean Startup lifecycle descriptions (as described in sections 3.3. and 3.4.). Investment size Second, the different sizes of investment deals. The investment stage and investment size are closely related. If a company grows over time, they are likely to need a larger amount of funding. An interesting difference occurs when looking closely at how investment size has changed over time. In early investment research, done in 1994, the investment size for the early stages was less than $500.000. If the required investment increases beyond that number, VCs involvement increases. In the first stages of investment, they found that the amounts of BA investment ranges between $100.000 and $300.000, where VCs tend to participate in a range of $1 million to $3 million (Ehrlich et al., 1994). However, as research in 2014 shows, the BA investment size is increased to $1 million, however, more important, the VC investment size also has changed, starting from 4 million (Angel Capital Association, 2014). Important to keep in mind is that the results apply for the US market, where VCs are continuously growing caused by a huge growth in the startup population attracting increased investments (figure 10). An example is the increased Series A amounts of investments in startups at Y Combinator (the example accelerator in 3.4.): on a sample of 92 startups, the average Series A size in 2014 is five times larger than an average Series A in 2008 (Mattermark, 2014). </p><p>Fig. 11 – Increased funding sizes by stage over the last decade (Mattermark, 2015) It seems positive that funding deal sizes are increasing, related to the positive influence on startup growth as discussed earlier. However, the Angel Capital Association found when VCs are able and willing to invest larger amounts of money, due to their growing funds, and BAs do not tend to invest larger amounts per investment deal, a funding gap appears, as shown in figure 11 (Angel Capital Association, 2014). This gap can result in a increasing toughness of survival for startups during their transition process from startup to company, since receiving financing influences the startup continuity and growth (as described before, in figure 8).</p><p>Fig. 12 – Investors per stage, including the Funding Gap (Angel Capital Association, 2014) Remaining Differences One of the other distinctions Maxwell et al. (2011) make is that BAs tend to invest their own money, because they are those wealthy individuals Hellmann and Puri (2000) talk about, where VCs tend to invest other people’s money. Geographic location is point of difference too. Where BAs tend to look at startups within 50 miles of where they live, VCs focus on seeking startups in specific areas like major cities where they are able to network with other VCs as well (Ehrlich et al., 1994). The motivation to invest differs for both groups where BAs are more likely to help a family member or friend or deriving non-monetary appreciation as a result of assisting other entrepreneurs in building companies, since they (mostly) were entrepreneurs themselves before they started investing (Ehrlich et al., 1994; Hellmann & Puri, 2000). Mason and Harrison (2002) compared BAs to VCs. They found that BAs focus more on avoiding bad investments, which is related to less ability for diversification. However, their gains from their investments are high. That could be caused by a better selection process or by their higher degree of involvement through for example value adding by knowledge. BAs have more entrepreneurial experience, whereas VCs have more investing experience. They also found that in terms of meeting frequency, BAs meet companies less often before investing than VCs do. As the characteristics describe, investors are closely involved in the company. They evaluate and monitor the companies intensively and continuously. The next chapter on Performance Measurement will discuss in detail which criteria are being used by both types of investors. Before that, one other important phenomenon needs some description. That is the factor of ‘risk’, which stands for the risk of losing your investment money invested in a startup.</p><p>3.7.4. Investment Risk and Startup Success As described earlier, Ruhnka and Young (1987) already described that particular risk phenomenon (Figure 11). Interesting to see is that already did research in 1987 investigating stages of company development, as can be seen in figure 12, where they have segregated a startup in phases. Slightly comparable to the four stages of Marmer et al. (2011), as described in chapter 3.3., and an additional fifth stage, the Exit. </p><p>Fig. 13 – Risk of Loss of Investment (Ruhnka & Young, 1987) As Ruhnka & Young (1987) does the risk decrease over the period of the life of a startup, related to information availability and therefore an improved ability to predict the startup success. An example of an implication of risk related to investor actions, is one of the findings of Kaplan and Stromberg (2004) who analysed 67 investments by 11 VC’s, where a higher (internal, i.e. team and product) risk is related to: the VC desiring more control, VC’s providing higher compensation to the entrepreneur and a higher contingency of a financing round. But why is that risk phenomenon that important to investors (and to the startups as their subjects)? Previous research towards investing criteria, consider venture capitalists as experts on the evaluation of companies, and the factors of risk defined by those VC’s are directly interpretable as success factors for those companies (Tyebjee and Bruno, 1984; Franke, Gruber, Harhoff and Henkel, 2008). Therefore this research will consider risk factors as a necessary part to be involved in this research, needed in the descriptive as well as the practical implications in in the following sections. Startup Evaluation As Franke et al. (2008) describe, research towards startup evaluation criteria has a long history, started in 1970 already, and still considered as a topic of interest in the present. They structured the evaluation criteria of previous research, in order of relative importance of several criteria, in a range from 1974 until 1999. This theoretical background will focus on literature in startup evaluation research from 2000 until now. The risks (i.e. evaluation criteria) appear in different categories, Maxwell et al. (2011) found. They listed four different risk categories (see figure 15), where Endogenous consists of the internal risk effects and Exogenous consists of the external risk effects. As discussed, these factors are equal to startup evaluation factors when establishing a potential relationship with those particular startups as a stakeholder, e.g. an investor.</p><p>Fig. 14 – Risk categories and related Endogenous and Exogenous examples (Maxwell et al., 2011) In an overview consisting of previous research on company evaluation can be seen that these risk factors are similar to the success factors (see table 3). The sources are chosen based on authority of the authors, diversity in investor type (including BA and VC), startup type (including digital and biotech), and publication year (> 2000). Author(s) Perspectives Factors Order / Weight Miloud et al Industry Organization economics Product differentiation n.a. 2012 Industry growth rate n.a. Venture Capitalists Recource-based view Industry experience n.a. Top Management experience n.a. Startup experience n.a. Top Management team (TMT) n.a. Team completeness n.a. Network theory Network size n.a. Baum & Silvermann Alliance capital Downstream alliances n.a. 2004 Upstream alliances n.a. Biotech startups Horizontal alliances n.a. Intellectual capital Patent applications n.a. Patents granted n.a. Human capital (TMT) Size TMT n.a. President no of roles n.a. President entrepreneurial activity n.a. President entrepreneurial abilities n.a. Maxwell et al V1 – Market potential Is there a large market for this product? A Large market potential (i.e. over $20 million) 2011 (market size) B Medium market potential (i.e. over $5 million) Business Angels C Unable to predict — likely less than $5 million. V2 – Product adoption Will customers in target market easily adopt this A Customers will easily adopt product or service (market share) product? B Benefits harder to identify, some adoption issues C No clear benefits, or major adoption issues V3 – Protectability How easy will it be for other people to copy the A Product patented or significant other barrier (profitability) product or service? B It will not be easy to replicate. C Anyone could copy it easily. V4 – Entrepreneur experience Does management have direct and relevant A Significant relevant experience (reputation) experience? B Limited experience, but appropriate knowledge C No evidence of required experience V5 – Product status Product ready for market, or major work required A Finished product (for technology risk) before it ships? B Design complete all technical issues addressed C Needs more research and development V6 – Route to market Is there a realistic marketing plan and route to A Realistic marketing plan / distribution partner (for operational risk) market? B Options identified — no agreements in place C Limited thought given to distribution issues V7 – Customer engagement Is a first customer identified? Does product meet A Customers in place, or committed to purchasing (for market risk) need? B Customers engaged in development project C No first customers identified. V8 – Financial projections Profitable and sustainable cash flow? A Sound business model and cash management (for financial risk) B Unclear profitability, limited cash management C No evidence of profit or cash management Where [V1 - V4 : Forecasted venture value (ROI), aggregated to Va] [V5 - V8 : Risk assessment values, aggregated to Vb] No trade-off between Va & Vb Kaplan & Stromberg Reason to Invest (Strength) Risk of Investment (Weakness) 2004 Quality of management 59,7% 61,2% Venture Capitalists Performance to date 26,9% 7,5% Funds at risk/downside 19,4% 13,4% Internal Factors Influence of other investors 6,0% 6,0% VC portfolio fit and monitoring cost 17,9% 14,9% Valuation 20,9% 19,4% Market size and growth 68,7% 31,3% Competition and barriers to entry 32,8% 40,3% External Factors Likelihood of customer adoption 29,9% 22,4% Financial markets and exit conditions 16,4% 7,5% Product and/or technology 40,3% 31,3% Difficulty of Execution Business strategy/model 53,7% 50,7% Gompers et al Success Probability/Prediction 2010 Entrepreneur - prior Second/later venture vs. First venture 20,9% vs. 25,0 % Venture Capitalists experience Entrepreneur-Company prior success Successful serial vs. Failing serial vs. First-time 30,6% vs. 22,1% vs. 20,9% Company-Entrepreneur prior success Successful serial vs. Failing serial vs. First-time 30,9% vs. 21,2% vs. 17,1% VC experience & Successful serial Experienced vs. Less experienced 32,4% vs. 31,9% entrepreneur VC experience & Failed serial Experienced vs. Less experienced 25,9% vs. 17,7% entrepreneur VC experience & First-time Experienced vs. Less experienced 20,9% vs. 14,2% entrepreneur Market timing (i.e. predicted success) Industry success rate High vs. Low 31,4% vs. 25,0% Managerial skill (i.e. residual success) Past success High vs. Low 34,9% vs. 26,6% Table 3 - Research overview on company success/risk factors of the last decade What immediately can be noticed are the comparable categories of startup success factors over the years of research. These success factors will be categorized into factors taking their priority into consideration. The first main category is the Team factor, represented by factors like top management experience, team completeness, size top management team, quality of management, entrepreneur prior success, etc. The second main category is the Product & Market, represented by factors like product differentiation, patents, product status, industry growth rate, several types of alliances, market potential, product adoption, competition and barriers to entry, etc. The third main category is the sum of the activities/results within the startup itself, which will referred to as the Startup, represented by factors like cash flow, business strategy/model, etc. As Davila et al. (2003) describe does the due diligence process, the investors’ evaluation/screening process of a startup, focus in a very detailed manner on the different factors as described above. Another moment of startup evaluation is the so-called ‘post- money valuation’, which is “the standard way of valuing firms in the venture capital industry”, after a financing round has taken place (Hellman, 2002, p.287). Hellman (2002) however, points out the lack of reliability of a post-money valuation, since it does not reflect the support a investor provides, which is an intangible company asset and therefore hard to evaluate.</p><p>3.7.5. Performance Monitoring Gompers (1995) describes why capital infusions, i.e. financing rounds, the most powerful control mechanism is for a venture capitalist, doing a major review of the progress, the due diligence and their decision to continue with follow-up funding. Therefore, the duration of a financing round can be considered as a metric for the monitoring intensity. For example: the shorter the financing rounds are, the greater the information need for monitoring a startup and entrepreneur’s progress is. Monitoring can be considered as valuable, useful to track the progress of a firm and possibly cut off financing in future rounds when there is negative information about the future of a firm. Gompers (1995) also found that monitoring intensity decreases due to increases in financing duration and tangibility of assets within a firm. Gompers (1995) does also describe the claim of venture capitalists on generating information and providing services as being as important as their financing, in contrast to what entrepreneurs believe. Early-stage startups do not have a track record, and therefore no historical information to provide. Thus does Gompers (1995) argue, VC’s should invest in those companies to increase the companies’ information oversight since they lack that particular information oversight. Gompers (1995) also found that firms in industries with significant growth opportunities and high R&D intensity results in close monitoring. Monitoring costs can be substantial for a VC when reading reports takes time away from other activities (Gompers, 1995). Although VCs monitor companies frequently, entrepreneurs still do have private information about their company. The frequency of monitoring checks is researched by Gorman and Sahlman (1989), through a survey among 49 VCs in 1984. They found that between financing rounds the lead VC visits the portfolio company once a month, for about four to five hours on an average visit. The other non-lead VCs do their check-up less frequently, typically once a quarter, for about two to three hours on an average visit. VCs request for monthly reports, and do not prefer to become involved in the management of the portfolio company. Gompers (1995) concludes that corporate control can be seen as “a fundamental concern of investors”. Davila & Foster (2005) indicate that the involvement of venture capital becomes mostly for the first time the request for formal monitoring. According to Gorman and Sahlman, this can be related to monitoring (i.e. agency) costs. Therefore, this is the point in time that through encouragement of VCs, the use formal information systems to monitor the startup performance comes into play. This is part of the professionalization VCs bring to early-stage startups they invest in (Hellman & Puri, 2000). Figure 14 shows the findings of Davila and Foster (2005) and Hellman and Puri (2000) on increasing adoption of Information Systems (ISs) after a startup receives VC funding. </p><p>Fig. 15 – Percentage of companies adopting different types of ISs since receiving VC funding (Davila & Foster, 2005) 1.12. Startup Performance Information Management How will startups survive and grow in the programs and ecosystems described in the previous section? What relevant information is needed and available to monitor and manage the startup lifecycle? The previous section on monitoring startup success factors will be elaborated in this chapter. In addition to that, different stakeholders (different types of investors in this research) require different types of information. This section will discuss Startup Performance Information Management (SPIM) to support defining, measuring and communicating progress of startups through their lifecycle. </p><p>3.8. Defining Performance Measurement The online business dictionary defines Information Management as the “application of management techniques to collect information, communicate it within and outside the organization, and process it to enable managers to make quicker and better decisions” (Business Dictionary, 2015). Many terms are used in processes concerning information management within companies, thus first some clarification between the two most relevant ones, Business Intelligence and Business Performance Management, is needed. Business Intelligence stands for the process and information to help managers reviewing the performance of an organization, whereas Business Performance Management focuses on both the quality of the information and the performance of an organization (Chaffey & Wood, 2004). Bringing the definitions on Information Management and Business Performance Management together makes Performance Information Management the collection and communication of the performance information produced through the execution of Business Performance Management, in order to make quicker and better decisions. In the context of this research those terms will be merged into a new term, in order to improve clarity concerning that context: Startup Performance Information Management (SPIM). SPIM is introduced before as the main topic of this research, where Performance Measurement serves as the process behind collecting data within SPIM. To make information management easier and faster by digitalizing it, i.e. digitalizing the management processes, Information Systems are developed. Laudon and Laudon (2006) did research towards managing ‘the digital firm’. However, they point out that before digitalizing firms, i.e. implementing information systems, “one must understand the problems they are designed to solve, their architectural and design elements, and the organizational processes that lead to these solutions” (Laudon & Laudon, 2006, p.8). Therefore this chapter will focus on two parts. First the exploration of the needs for performance measurement for internal and external stakeholders. This can be seen as a theoretical background of a hypothetical performance information management system, in order to be able to provide the second part, a high-level advice on a design approach for that particular information system. 1.1.1. Performance Measurement Goals Why performance measurement? To improve startup (or ‘business’) performance, performance measurement is a crucial element (Taticchi, Tonelli & Cagnazzo, 2010). Also taking into consideration that, as described in the previous section, collecting information through information management is a crucial part of making quicker and better decisions. Franco-Santos, Kennerley, Micheli, Martinez, Mason, Marr, Neely (2007, p.9) define the performance measurement objectives as: “organizations measure their performance in order to check their position (as a means to establish position, compare position or benchmarking, monitor progress), communicate their position (as a means to communicate performance internally and with the regulator), confirm priorities (as a means to manage performance, cost and control, focus investment and actions), and compel progress (as a means of motivation and rewards)”. Wickman (2011) describes eight company (internal) advantages of having numbers on performance measurement in a company: 1. Numbers cut through murky subjective communication between manager and direct reports. They become a communication tool between manager and direct reports, creating the basis of comparison, unemotional dialogue and results. 2. Numbers create accountability. When you set a number, everyone knows what the expectation is. Accountability begins with clear expectations and nothing is clearer than a number. 3. Accountability people appreciate numbers. Wrong people in the wrong seats usually resist measurables. Right people in the right seats love clarity. Knowing the numbers they need to hit, they enjoy being part of a culture where all are held accountable. 4. Numbers create clarity and commitment. When an employee is clear on his or her number and agrees that he or she can achieve it, you have commitment. There is no grey area. 5. Numbers create competition. There’s nothing wrong with a little pressure. 6. Numbers produce results. What gets watched improves. 7. Numbers create teamwork. When a team composed of the right people in the right seats agree to a number to hit, they ask themselves “how can we hit it,” creating camaraderie and peer pressure. 8. You solve problems faster. When an activity-based number is off track, you can attack it and solve the problem proactively; unlike with an end-result based number that shows up after it’s too late to change it. In addition, the use of hard data cuts through all of the subjective and emotional opinions that create murkiness and lengthen the amount of time it takes to make the right decision. These eight advantages clearly explain how metrics contribute to clear communication between employees and drives to company success. The Startup Genome Project, in collaboration with the Stanford University and Berkeley University, performed an in- depth research into the success factors of 650+ web startups located in Silicon Valley, from 2004 till now. Their first key finding also highlights the importance of performance measurement, in this case combined with mentoring and proven methods usage (Marmer et al., 2011, p.5): “ Founders that learn are more successful: Startups that have helpful mentors, track metrics effectively, and learn from startup thought leaders raise 7x more money and have 3.5x better user growth.” The way to track these metrics is what performance measurement is. Performance measurement consists of measures defined by Neely, Mills, Gregory and Platts (1995, p.1) as: “metrics used to quantify the efficiency and/or effectiveness of action”. Note: from now on those measures will be referred to as ‘metrics’.</p><p>3.9. Financial-to-Innovation Paradigm Shift In traditional companies, Neely (2002), identified three different business perspectives (i.e. business functions), which can be used for performance measurement: The Accounting perspective, Marketing perspective and Operations perspective. However, as discussed in chapter 3.1, startups transitioning towards high growth companies do not have these mature business functions in place yet as traditional companies do have. Instead, as described in previous chapters, the Lean Startup building approach focuses primarily on the early stage of a company, when creating and managing the startup, to get the desired product to the customer as quick as possible, using innovation accounting: objectively measuring how you are doing (Ries, 2011). Maurya (2012) does also describe the movement where accounting has shifted from Financial accounting, focused on the cost and revenue part, used for late-stage companies, to Innovation Accounting, focused on the early stage of a company. Therefore, the Lean Startup loop is created as an approach to continuously test and measure whether the hypotheses (i.e. assumptions) of a startup, about their problem, customers and solution, can be proven by customer discovery and validation (Blank & Dorf, 2012). To eliminate uncertainty as much as possible, the Measure part is included in the iterative loop of Lean Startup as shown in chapter 3.2 (Ries, 2011). An interesting link to the changing paradigm shift and the investors perception on these paradigms is one of the findings of Marmer et al. (2011, p.13), who found that “better performing VCs understood that startups are a search process for product market fit and a scalable business model. As a result, they drew conclusions based on more subtle data points (..)”. In line with the performance measurement as described in the introduction of this section, this proves the need for (non-traditional) performance measurement during startup building. </p><p>3.10. Metrics Next reasonable question following from preceding theory then will be: which metrics can be considered as relevant for measuring the performance of startups? And how to apply those metrics, what is the usability of those metrics? Therefore this chapter will first elaborate on the metrics itself, followed by describing how to focus on the right metrics. The metrics descriptions will be divided into two parts: the general metrics and the stage-dependent metrics. General aims at the metrics involved at all stages of a startup, where stage-dependent metrics are about metrics only tied to one or (maximum) two specific phases. Both sections will conclude with a metric overview.</p><p>3.10.1. General Metrics The general metrics are divided into three main categories, aligned to the risk/success factors as found in section 3.5.2.: Team, Product & Market and Startup. Team The startup team is one of the main variables of interest in a research from Deloitte and THNK, among a population of 400.000 companies, founded since 2005, over 24 countries (Deloitte & THNK, 2015). In their research they focused on the scale-ups and unicorns among these startups, where they define a scale-up as a company with a revenue level of 10 million dollar in their fifth year, and being classified as a unicorn requires a valuation above 1 billion dollar (like Facebook, Google, and Über). The set of global unicorns consists only of 104 startups at the time of research (early 2015), where nowadays 144 unicorns do exist (late 2015) (CBInsights, 2015). - Size A team of at least two persons versus a single founder starting the company is the case in approximately 50% of the scale-up population (Deloitte & THNK, 2015). - Experience One of the major findings is that 78% of the scale-ups is founded by a team consisting of at least one person with corporate or entrepreneurial experience. In the case of the unicorns, that’s even the case in 89% of their teams. For the remaining startups, called survivors, the number is 57%. Having industry specialists on board is perceived as effective since 61% of the scale-up leaders in the UK state this as a grow accelerating team component. For the unicorns, even 85% of the teams have at least one experienced specialist on board (Deloitte & THNK, 2015). - Background The university dropout becoming a billionaire by launching a startup is rarely the case. Most of the founders of scale-ups have an academic degree, e.g. a bachelor diploma, master diploma or PhD (Deloitte & THNK, 2015). Product & Market - Existing Alternatives As Chaffey and Wood (2004) describe, one of the goals of management information gathering is being aware of the external environment and respond to activities in that particular environment, monitoring for example competitor activity like new product releases. In line with Blank and Dorf (2012) above, this research will focus mainly on entering new markets, a continuously monitoring for new entrants on a particular (new) market will be useful, according to Chaffey and Wood (2004). However, the competitor focus also receives critics from Lean startup focused leaders, whereas Maurya (2012) focuses more on existing alternatives used by customers than primarily on competitors. Especially in new markets, competitors enter that particular market from the moment of initial startup success, thus not at the early early-stage (or seed stage) of a startup (Maurya, 2012). Therefore this metric is called existing alternatives instead of the traditional competitor focus. - Target Market As discussed in 3.3.2.2., Product/Market cannot be considered being a bing bang event or something you cannot lose as a startup. Therefore, the search for the right target market will be discussed both as a general metric as well as a stage-dependent metric. As Blank & Dorf (2012) show in figure 14, the market of a startup can be distinguished into three different types: TAM corresponds to the whole ‘universe’, Served Available Market (SAM) corresponds to the reach of a startup Sales channel, and Target Market describes the number of startup prospects, i.e. potential buyers of your product. Blank and Dorf (2012) suggest in their theory that startups need to focus on the latter only. These markets are quantifiable in terms of size for existing markets, however, most startups focus in particular on new markets, where they don’t know who their target customers will be (Blank & Dorf, 2012). This is where the search for the business model in the startup definition in 3.1. corresponds to. Therefore the key is not primarily competing, like discussed at the previous metric, but getting an understanding where a large customer base exists and how those customers behave (Blank & Dorf, 2012). Startup This section will describe which metrics are involved as startup general metrics, concerning financials and the business model. - Financials Just since some years, more knowledge about useful metrics for early-stage startups evolved. Where traditional measures were for example profit and loss, balance sheet, cash-flow forecast, etc., early-stage startups are more focused on testing customer and market hypotheses rather than these (quite static) financial metrics (Blank & Dorf, 2012). Blank and Dorf (2012) argue that a startup only needs to focus on a few financial metrics: cash-burn rate (or burn-rate), number of months’ worth of cash left (defined by Ries (2011) as ‘runway’), short-term hiring plans and time needed to reach the cash-flow break-even point. - Pivots As 3.3.1. describes, the number of pivots is crucial, where startups pivoting once or twice are much more successful than startups pivoting more than two times or pivoting not at all. According to Ries (2011) and Marmer et al. (2011), business changes can be considered as pivots when there is a major change in the business (e.g. a new market) - Learning Since theory as discussed in 3.6.1. shows that tracking metrics effectively and learning from startup thought leaders (i.e. mentors and usage of proven building methods) leads to better growth, these both learning parts can be considered as relevant to track if they are used in the startup daily activities as well. Tracking metrics effectively sounds subjective, however theory has made a distinction between vanity and actionable metrics, it is possible to measure whether metrics are used in an effective way (3.6.3.5. will elaborate on picking the right metrics). Also checking whether a startup receives help from mentors and usage of proven building methods is easy to check.</p><p>3.10.2. Conclusion This section will describe the quantify equivalent of the metrics described in the preceding theory above, on Team, Market, and Startup. Team . Size: no. of founders . Experience: corporate/entrepreneurial/none; no. of industry specialists . Background: academic degree Market . Existing Alternatives: no. of existing alternatives . Target Market: no. of potential customers Startup . Financials: burn rate; runway; short-term hiring plan; time-to-break-even . Pivots: no. of pivots . Learning: effective metrics implemented; mentor help + usage of proven building methods </p><p>3.10.3. Stage-Dependent Metrics Based on 3.3.2., this section will discuss the relevant metrics in the order of the four stages of the Startup Genome framework (Discovery, Validation, Efficiency, Scale), which are tied to the three thresholds (i.e. milestones) defined by Maurya (2012) (Problem/Solution fit, Product/Market fit, Growth). Each of these parts will first provide a short recap on the particular phase, followed by providing potential metrics. As proposed in 3.3.1., the Lean Analytics framework will be incorporated here, to use the best practices (metrics) from that particular (state-of-the-art) framework, since they particularly focus on startup performance measurement, i.e. measuring the different phases of the Lean Startup method. Discovery To measure whether a startup has reached the P/S Fit, i.e. as 3.3.2. describes, a startup has to discover (i.e. identify) the problem and the customer segment experiencing that particular problem, executing experiments is the major activity of this phase. The results experiments results are mainly qualitative (Croll & Yoskovitz, 2013). To have data to base the next action upon, an entrepreneur needs to verify those results quantitatively on people he assumes as being prospects. Therefore McClure (2007) relates the metric Acquisition to this particular phase, as the metric to monitor an increasing (potential) user base, i.e. prospects. Qualitative research done in a scientific manner, as the method part of this research describes in chapter 2, uses extensive processing steps in order to form a grounded theory derived from interviews. The Lean Startup movement has developed their own way of qualitative research, in order to be as quick as possible and still paying much attention to interview results. Two ways of using an actionable metric to quantify the qualitative results within the P/S fit phase are provided by Maurya (2013) and Croll and Yoskovitz (2013). The first one, is the Customer Factory created by Maurya (2013), using four of the five AARRR (pirate) metrics as defined by McClure (2007) (see figure 16). From these P/S Fit phase-tailored metrics, the most important rate to measure whether the startup is on track on reaching P/S Fit, is the Revenue, in this particular case 20%, symbolizing what Maurya (2013) calls the ‘production-rate’, i.e. problem/solution interview success-rate. This rate is derived from the percentage of persons interviewed who want to pay for a potential solution, as part of the group of persons interviewed about a possible solution, the Activation number, which is the remaining part of the first step, the problem interviews, which is described by the Acquisition number. A Business-to-Business (B2B) startup requires other numbers than a Business-to-Consumer (B2C) startup, an example could be that a B2B startup requires 100 interviews to start with, where a B2C startup needs 1000 interviews to start with (Maurya, 2013). Referral describes the number of prospects referred to by persons interviewed in the solution interviews, which can be used as acquisition prospects for the next iteration. After enough iteration steps the startup needs to reach an 80% ‘production rate’ as a signal for reaching P/S Fit, which in the example of figure 16 is 20% (Maurya, 2013). </p><p>Fig. 16 – Example Problem/Solution fit interview conversion metrics The second possible way of verifying your P/S Fit discovery in a quantitative manner is provided by Croll and Yoskovitz (2013). Scoring a list of six questions can tell a startup whether the problem they want to solve is painful enough to solve. Attachment A shows the whole list including the scores; one example is provided in table 4 (see next page). Question 1. Did the interviewee successfully rank the problems you presented? Score The interviewee ranked the problems with strong interest Yes 10 (irrespective of the ranking) He couldn’t decide which problem was really painful, but he was still really interested Sort of 5 in the problems No He struggled with this, or he spent more time talking about other problems he has 0 Table 4 – First out of six question to calculate the interview score in the P/S Fit (Croll & Yoskovitz, 2013) The sum of the six question scores can tell whether a startup has found a P/S Fit, explained by a score of a range between 0 and 35, where a score of at least 31 proves P/S Fit is found. Assuming a startup has discovered P/S Fit, the next phase can be entered, which will be the validation of an MVP in order to reach P/M Fit. Validation As 3.3.2. describes, this phase is about validating your potential solution, explained by terms like MVP iteration (Ries, 2008), Stickiness (Croll & Yoskovitz, 2013) and Validation (Marmer et al., 2011). This phase mainly focuses on testing the MVP, therefore data on MVP testing is the core part (Croll & Yoskovitz, 2013). MVPs can appear in different types (video’s, prototypes, etc.). To narrow down the focus on MVPs in order to be able to provide example metrics, the SaaS focus of this research will be used. A SaaS MVP could be a prototype of the potential solution, for example, an investing platform, accessible trough the internet, reaching the target customer segment via associated channels, which could be the mailing-list of the customer segment the startup already gathered in the Discovery phase. The startup can measure and learn how its’ MVP is doing, by tracking users’ Activation and Retention, in SaaS solutions measured by the so-called metric on Engagement (McClure, 2007; Croll & Yoskovitz, 2013). The metrics are, compared to the previous phase, of increased usefulness due to their quantitative character. When should a startup consider P/M Fit as reached? Maurya (2012) advocates using the threshold Ellis (2009) has defined, proven through usage among 100+ startups. The startup has to ask their prospects the following multiple-choice question: “How would you feel if you could no longer use product X?” His rule dictates prospects to choose one of the following answers: 1. Very disappointed 2. Somewhat disappointed 3. Not disappointed (It isn’t really that useful) 4. N/A – I no longer use Product X P/M Fit can considered as being reached “at least 40% of users saying they would be “very disappointed” without your product”, whereas his research shows that startups scoring below 40% always struggle on their following lifetime (Ellis, 2009). Gross (2015) did research among 100+ startups started within Idealab, a venture builder organization, and presented his findings at a TEDTalk. He found out that finding the right market at the right time in matters most, compared to the idea and the execution of that particular idea: “execution definitely matters a lot. The idea matters a lot. But timing might matter even more. And the best way to really assess timing is to really look at whether consumers are really ready for what you have to offer them” (Gross, 2015). As the above has shown, the first customers start using the product in this stage. Startup performance metrics provided by Croll and Yoskovitz (2013) are shown in table 5. Which are comparable to the characteristics of Acquisition and Activation (McClure, 2007). Note that revenue-related (monetizing) metrics are not applied by the authors yet. Metric Description Attention How effectively the business attracts visitors Number of visitors becoming free or trial users (first conversion), if you’re relying Enrolment (conversion) on one of these models to market the service Stickiness Number of customers using product How many users leave in a given time period. Churn Bottom-line: for early-stage companies churn should be 5%, to consider business as sticky. Feature Utilization What features are used and therefore ask yourself what to build next into your MVP. Table 5 – Usage validation metrics (Croll and Yoskovitz, 2013) Skok (2013), SaaS expert and five-time serial entrepreneur, currently VC, describes the acquisition and activation metrics as funnel metrics, where conversion is the link between the several metrics. Therefore, the previous P/S Fit related Customer Factory (Maurya, 2013), can be used in this P/M validation stage as a visualization of funnel metrics as well. The funnel metrics can be used in forward planning, for example calculating how many sales people are required in order to attract more visitors and turning them into users and possibly paying customers. After finding (the first) P/M Fit and having the first metrics in place, a startup can proceed to the next phase, Efficiency. Efficiency This particular phase is included, as discussed in 3.3.2., to avoid pre-mature scaling (Marmer et al., 2011) or pre-mature virality (Croll & Yoskovitz, 2013), which are described as one of the major reasons for startup failure. If a startup invests in user growth, but still has a high churn (i.e. low retention), return on investment will be (dangerously) low (Croll & Yoskovitz, 2013). Therefore, this phase will focus on increasing efficiency, by creating self-sustaining growth (i.e. virality). However, still do Croll and Yoskovitz (2013) warn for only focussing on reaching that efficiency/virality, on the expense of engagement: getting new users is great, however losing engagement is possible when new users are different from your first users, the early adopters. This corresponds to Skok (2013), who defines three main goals of SaaS businesses: Acquiring customers, Retaining customers and Monetizing customers. He provides metrics for startups to monitor how they are doing in reaching these three goals. The Efficiency stage needs to focus on Retaining customers effectively, as Skok (2013) describes as avoiding churn through negative-churn, to be able to recover revenue from loosing through churn, in order to keep a startup growing. A method to enable negative churn is for example through upselling when growing. Therefore a startup needs to identify the churn problem in time, in order to be able to define a roadmap (strategy) for negative-churn on time (Skok, 2013), in the Efficiency stage. Ries (2011) provided a method to realise self-sustaining growth, through the so-called Engine of Growth (EoG) (Ries, 2011), or similar the different types of Virality (Croll & Yoskovitz, 2013), as 3.3.2.3. describes. Stakeholders want to know for this particular stage whether user acquisition and growth can be accomplished as 3.3.2. describes. Therefore, Croll and Yoskovitz (2013) define the key quantifying measurement here as the ‘viral coefficient’: the growth number of new users per existing user. Where a viral coefficient above 1 means that every single user is inviting at least another user. Besides focusing on that particular coefficient, the cycle-time of virality is important too, which explains the time it takes for a user to invite another user, which determines fast or slow growth in the user base (Croll & Yoskovitz, 2013). Since prospects are turned into users and possibly even paying users in this phase, when the MVP is validated and the product is live, from now on financial metrics (like Revenue) need to be monitored as well. The metrics are listed in table 5, some including bottom-lines describing what numbers represent a healthy SaaS business. Those bottom-line numbers are based on Skok (2013) and Croll and Yoskovitz (2013) (the latter also use the experience of Skok to form their theories). Metric Description How much money a customer brings in within a given time period. Revenue-per-customer Bottom-line: Grow 20% from year to year, trough: series of tiered offerings and easy upselling path. Total (recurring) Monthly Recurring Revenue (MRR) and Annual Recurring Revenue (ARR). Revenue Number of users becoming paying customers, and how many of those switch to a Conversion higher-paying tier Churn How many users and customers leave in a given time period. You have to see a clear path to getting churn below 2% if you want to scale significantly. There must be a roadmap in place explaining the strategy for negative churn for the Negative churn roadmap next phase. Methods: product expansions, upselling, and cross-sells, to your current customer base. Customer Acquisition How much it costs to get a paying user Cost (CAC) Customer Lifetime How much customers are worth from cradle to grave Value (CLV) The CLV to CAC ratio, meaning the ratio of the costs of customer acquisition and CLV : CAC the value a customer brings into the company. (value) Bottom-line: CLV > 3 x CAC. In optimal form higher than 3, sometimes even 7 or 8. A time-bound CLV:CAC ratio is about profitability and cash flow. CLV : CAC Bottom-line: Months to recover CAC < 12. A well-performing startup needs to (time) recover from the CAC within 12 months after acquiring that particular customer, where many of the best recover in 5-7 months. Larger businesses can afford to have a longer recovery-time. Billings The amount that you have invoiced that is due for payment shortly. Uptime and Reliability How many complaints, problem escalations, or outages the company has. Table 6 – SaaS startup metrics - Efficiency (Croll & Yoskovitz, 2013; Skok, 2013) A calculation example on recovering from CAC till a customer breakeven point is reached, is provided by Croll and Yoskovitz (2013). They calculate that the CAC of $14 is recovered through monthly revenue of $2.45 in (14/2.45) 5.7 months to customer breakeven, which is related to burn categorized in general metrics. Scale This phase will add extra metrics to the existing phase. The metrics a startup already tracks from the previous phases can be put into an accounting system. And, metrics that were seen as being distractions before become useful now. For example: metrics concerning competitors. Therefore, metrics provided by Croll & Yoskovitz are on now also focused on the ecosystem. Metric Description A path to negative churn needs to be in place to recover revenue from loosing through churn, in order to scale a startup. Negative Churn Bottom-line: a startup needs to get 2% of the paying subscribers to increase what they pay each month. What makes customers increase their spending, and how often that happens, Up-selling focused on up-selling: upgrades, maintenance, licensing-deals, etc. What makes customers increase their spending, and how often that happens, Cross-selling focused on cross-selling: extra related useful products, services. Traffic in APIs show how your data or services are integrated on the web into Application Programming other modules or applications. That network effect is important in supporting Interface (API) traffic growth. The number and effectiveness of ecosystem channel relationships, on for Ecosystem Channel example resellers selling your product for you, is an important factor to enable a Relationships network effect and therefore supports growth. The number of relevant competitors now becomes important, since from this Competitors phase on it is signalled to others you do sell the right product in the right market. Support costs in order to be able to serve your existing customers in doing Support Costs maintenance, becomes a relevant metric now. The ability to deliver what is promised, or, are you in control as a software Compliance supplier. Can be reported quarterly for example. Entering new markets. Could be the ones already existing, or creating the market Market Expansion where it does not exist yet. Total number of FTEs of the new sales people supporting growth (scaling-up) at Sales Force most of the above metrics Table 7 - SaaS startup metrics – Scale (Croll & Yoskovitz, 2013; Skok, 2013) 3.10.4. Conclusion This part has clarified the insights in what happens in each stage of a startup, aiming at metrics to support increasing the understanding of startup performance for both entrepreneurs as investors. A clear development shown through metrics can be seen from the first stage, Discovery, focused on interviews, to Validation, merely focused on testing the MVP, to Efficiency, which metrics show preparation for Scale to avoid pre-mature scaling, and in the end Scale, which focuses more on fast growth and the ecosystem. The following chapters will first describe what systems are currently used to track what happens in the startup stages, followed by data concerning startup stakeholders considerations on relevant startup evaluation factors or metrics, closed by a chapter where it all will be concluded in a framework functioning as a metrics base, making the theoretical defined metrics applicable. </p><p>1.13. Performance Measurement Systems </p><p>3.11. Performance Measurement System Goals To perform business performance measurement, a system to perform that task is necessary. That business performance measurement system functions as a management system (management system is a term for all kind of management assisting systems, like business performance measurement systems). What are the internal organizational benefits of implementing those business performance systems as management systems? Antonio Davila, George Foster and Ning Jia (2010) did research on ways of building sustainable high-growth startup companies. They prove that at a certain point in time, the so-called ‘entrepreneurial crisis’, where 50-100 employees are involved, organization management has to transform from ‘personal’ to ‘professional’. In this increasing so-called ‘professional’ management, management systems play a critical role (Davila et al., 2010). As 3.5.2. describes, VCs are able to bring that professionalization to early-stage startups, resulting in the increasing adoption of information systems. Little background on the purpose of a information system, will be provided first. Besides internal organizational information, information systems also can provide information on the external world of customers, suppliers, etc. The Hawley committee (Hawley, 1995) defined several information groupings, like market and customer information, business process information, etc. According to Chaffey and Wood (2004, p.14), these groupings can be used by information systems to: 1. Research demand for new products – customers in different markets can be surveyed for their needs for products. 2. Exchange information with partners such as suppliers as part of their operational processes. 3. Communicate messages about brands and products externally. 4. Monitor and control operating processes for efficiency and improve them to save time or money. 5. Sense what is happening in the external environment and respond accordingly through their strategy and tactics. For example, it can monitor competitor activity such as the introduction of new products or the winning of new contracts. Davila and Foster (2005) found out that financial planning systems are the earliest set of systems adopted. However, as the startup lifecycle and the corresponding metrics have shown in the previous chapters, traditional financial planning systems should not be the earliest set of systems, since they are not sufficient in managing the product and market focused performance information in the early-stage. This is related to the paradigm shift currently happening, from Financial focus to Innovation focus, as is described in 3.6.2. Therefore it might be useful to clarify definitions on such (traditional) management systems first, where Management Accounting Systems (MAS), focused on the financial aspects of a company, can be defined as a subset of Management Control Systems (MCS) (Davila & Foster, 2005). The advantage Davila and Foster (2005) found is the positive association between the adoption of management control systems and startup growth. Related is the CEOs position, where adopting fewer information systems result in shorter tenures for CEOs. Therefore, the execution of business performance measurement, in the industry also known as business performance management (BPM), needs a management (control) system, taking all different company stages into consideration. A detailed definition on performance measurement system and related goals and methods is provided by Franco-Santos et al. (2007, p.9): “A performance measurement system enables informed decisions to be made and actions to be taken because it quantifies the efficiency and effectiveness of past actions through the acquisition, collation, sorting, analysis, interpretation, and dissemination of appropriate data.” Useful requirements for such a system are provided by Stair, Reynolds and Chesney (2008, p.239), describing seven characteristics of Executive Support Systems (ESS): Tailored to individual executives Are easy to use Have drill-down abilities Support the need for external data Can help with situations that have a high degree of uncertainty Have a future orientation Are linked with value-added business processes</p><p>3.12. State of the Art As discussed in chapter 3.6. on general and stage-dependent metrics, this research uses the four Startup Genome-stages. This section will elaborate on the available tools designed to reach the associated milestones P/S Fit, P/M Fit, and Growth as quick as possible, taking into consideration it needs to fit early-stage startups. Not only do the tools fulfil requirements on early-stage fit, the performance information in the tool needs to be available to external viewers (i.e. stakeholders) too. All of them are online available. The state-of-the-art consists of mainly three tools (or frameworks), developed by leaders within the lean startup movement, to guide a startup through their early-stages process (Maurya, 2012; Ries, 2011): First the Lean Canvas (Maurya, 2012), second the Validation Board (Lean Startup Machine, 2012) and third the Lean Dashboard (Maurya, 2013). A fourth tool looked very useful, but since they are releasing at the moment this research is done, no evaluation can be done yet. 1. Lean Canvas The Lean Canvas, depicted in figure 17, is designed by Maurya (2012), as an adoption from the Business Model Canvas, to primarily enable entrepreneurs to clearly capture that what is most uncertain and most risky in a startup, for both the product and market side of a startup. The sequence a startup defines the Lean Canvas is as follows: (1) Problem (2) Customer Segments, (3) Unique Value Proposition (UVP), (4) Solution, (6) Channels, (6) Revenue Streams, (7) Cost Structure, (8) Key Metrics, and (9) Unfair Advantage (Maurya, 2012). Some of the concepts will be highlighted here. Keeping the Solution box small compared to the Problem box corresponds to the concept of a MVP instead of a detailed solution and the importance of focusing on the problem a startup wants to solve. Key metrics are important concerning defining a few key actions (or key macro metrics) that matter, in order to be able to focus. Unfair Advantage is also known as competitive advantage, whereas Maurya (2012) notes that few startups have a true Unfair Advantage from day one. The UVP is different than the Problem: the UVP is the marketing promise of combining the problem and solution. The model can be used in different stages of a startup, Maurya (2012) argues, where the risks documented evolve over the lifecycle of a startup. </p><p>Fig. 17 – The Lean Canvas (Maurya, 2012)</p><p>2. Validation Board The Validation Board, or Experimentation Board, is used to document the testing of hypotheses, i.e. documenting the validation process of experiments. It provides a structured overview, focused on the main Lean Canvas parts, Customer, Problem and Solution, thereby driving the entrepreneurs’ behaviour towards ‘getting out of the building’ and talking to potential customers, to validate the hypotheses (i.e. assumptions). The process is done step by step through the Pivots at the upper half, to be able to test the assumptions in an iterative way. Fig. 18 – Validation Board (Lean Startup Machine, 2012) 3. Lean Dashboard. The Lean Dashboard, also developed by Maurya (2013), is like the Validation Board designed to do experimentations in a structured manner, however this tool is combined with the Customer Factory. The Customer Factory is described in 3.6.3.3. when discussing metrics concerning P/S Fit. The dashboard is shown in figure 19, an example filled in for a SaaS startup in the P/M Fit phase. The process starts on the upper level by defining how many customers are needed to reach the Scale target, from which P/M Fit is a smaller version, and P/S Fit an even smaller version. A Scale target of 2000 customers is a customer production rate is derived from the key metric of $10M ARR, where the revenue stream is $200 MRR, with a customer LTV of 2 years, which means the need for approximately 2000 new customers/year. The experiments show the validation of the different experiments, which are time-bound since the entrepreneur can appoint an end-date to each objective and experiment. The metrics show the numbers and conversion of the leads, users, customers, and referrals (new leads). The completed experiments show the validated or invalidated experiments. Fig. 19 – Lean Dashboard (Maurya, 2013)</p><p>The three online tools as described in this section support a part of the Lean Startup methods in their own way, based on best practices, whereas two of them are developed by a startup thought leader (Maurya). However, since they only support a part of the process, they cannot be considered as solutions feasible to all the stages, in particular concerning SPIM and its related metrics implementation.</p><p>4. Data Now the underlying theory as discussed in the previous chapter will be used in this chapter, to support the results: the interview data and the framework. This chapter will firstly describe the interview data: the descriptions of data collection, data processing and analysis method, followed by the data. Secondly, the framework based upon the theory and interview results will be presented. 1.14. Qualitative Methods</p><p>4.1. Data Collection As described in chapter 2, qualitative data is gathered through interviews, in order to build a grounded theory on the topic of SPIM within a startup ecosystem. The interview protocols are attached to this research in the appendices, specified for the different stakeholder groups: Appendix B on Building Programs (Incubators/Accelerators/Venture Builders) and Appendix C on Investors (Business Angels/Venture Capitalists). The interviews are semi-structured, i.e. consisting of both specific questions aiming at short answers as well as open questions aiming at unexpected responses, which tend to be more highly interactive and provide the ability to the researcher to clarify questions (Singer, Sim & Lethbridge, 2008). However, interviews are time and cost consuming, concerning scheduling, attending the meeting, and transcribing and coding the audio recordings (Singer et al., 2008). This resulted in a much longer period of time invested in this specific part of the research than anticipated in planning the research milestones. </p><p>4.2. Data Quality First some elaboration on which stakeholders participated, and how the selection process is done. Four different categories of stakeholders are included: one consists of experts in the startup field and three consist of ecosystem stakeholders. The experts will be used as a starting point of the research, exploring which scope would be appropriate, which stakeholders needs to be considered as relevant, etc. The goal concerning ecosystem stakeholders is to enhance existing startup and stakeholder theories, by bringing new insights to the field of research. To optimally serve the research, several criteria need to be met. The experts need to have experience in doing research towards large populations of startups over multiple years. The criteria used for the ecosystem stakeholders obviously are different. There must be at least one set of building organizations (incubators, accelerators or venture builders), which have a track-record in building multiple startups over multiple years. Also needed is one set of Business Angels and one set of Venture Capitalists, who both can show a proven track-record, i.e. have invested in multiple startups over multiple years, including investing in at least one SaaS startup. As the theory discussed, Business Angels belong to a group of considerable heterogeneous wealthy individuals. In order to be able to draw conclusions on such a wide group of individuals, formal founded Business Angel networks will be used to gather data from. These criteria are needed in order to be able to justify usage of the data, through guaranteeing quality of that data. The right quantity of the data in order to be useful to include in the research is subjective, either little field notes while doing an interview, or an explorative unstructured interview will not be considered as useful. Table 7 below, shows the descriptive data on the quantitative data gathered through the interviews, including three data sources which are excluded after analysing the data, based on the quantitative criteria mentioned above. Stakeholder Type Company Interviewee Occupation Investment size Company Age Interview (euros) (years) time (min) Building Program INC 1 Head Europe Relations 21.000 (GBP) < 10 35 Incubator INC 2 Incubation Manager N.a. > 10 60 INC 3 Coach N.a. < 10 40 INC 4 Business Analyst N.a. N.a. 30 Investor BA 1 Partner 200K - 1M > 10 25 BA (matching) BA 2 Director 50K - 2.5M < 10 55 BA 3 Director Unknown < 10 55</p><p>Investor VC 1 Investment Manager 100K – 500K Unknown 75 VC (seed - growth) VC 2 Managing Partner 100K – 500K Unknown 60 VC 3 Investment Manager 2.5M - 10M < 10 40 VC 4 Director 1M- 5M < 10 60 Expert Expert 1 Scientific Director N.a. N.a. 35 Expert 2 Business Analyst N.a. N.a. 40 Performance Tool Tool Business Analyst N.a. < 10 80 Company</p><p>Table 8 – Descriptive data (including excluded sets)</p><p>4.3. Data Analysis Method The qualitative interview results are transcribed and coded, in order to be able to formulate a grounded theory, based on analysis and conceptualization of the coded data. Some interview recordings are transcribed using Microsoft Word and VLC media player, and some by using the qualitative research tool NVIVO. The coding of those transcripts is based on both selective coding (pre-formed codes), in line with the structure of the interviews, which are tied to the concepts used in the research questions, as well as coding open to the context and conditions of the interview (post-formed codes) to support the emergent nature of grounded theory. The level of coding is detailed, on a sentence level, since the number of participants is small and still to be able to get most out of the data. The analysis and conceptualization is done through practices derived from literature on information systems qualitative research methods: cross-case analysis of data slices, in order to compare the different stakeholders within similar and between the different groups (stakeholder types functioning as cases), to build the theory (Urquhart, Lehmann & Myers, 2010). 1.15. Data</p><p>4.4. Concepts Overview Before diving into the detailed results, an overview of the used codes (i.e. nodes or topics) will be provided. This is included prior to the data belonging to these particular codes, to provide a preview on the topics and corresponding structure as emerged and used in the interview data. The nodes of the data set are shown in table 8, consisting of nodes and sub- nodes, for the stakeholders, the experts, descriptive data on the framework, and general tips, hints and references received during the interviews. Building Programs Business Angels Venture Capitalists Stakeholder focus Stakeholder focus Stakeholder Focus Startup Sectors Startup Sectors Startup Sectors Startup Stages Startup Stages Startup Stages Building Methods Engagement Engagement Investor Involvement Governance (Board/MT/Coach) Governance (Board/MT/Coach) Program Reporting BA Knowledge VC Knowledge Establishing Relationship Establishing Relationship Formal Agreements Formal Agreements Exit Strategy Exit Strategy SPIM SPIM SPIM SPIM before SPIM before SPIM before Assessment Information Assessment Method Assessment Method SPIM after Assessment Information Assessment Information Reporting Format Team SPIM after Reporting Frequency Product + Market Reporting Format Reporting Request Funding Size Reporting Frequency Information SPIM after Information Intervention Reporting Format Information Asymmetry Reporting Frequency Intervention Information Information Asymmetry Intervention BA (network) Assessment Experts Framework Tips/Hints/References Research Focus General Stakeholders Choosing Stakeholders Startup Population Startup Type Research goals Building Methods Coaching Evaluation Criteria Table 9 – Interview Data Structure Two main topics are Stakeholder (or Research) Focus and SPIM. The first main topic, Stakeholder (or Research) Focus, aims at the type and maturity of startups a stakeholder or expert is involved in. The second main topic, SPIM, obviously is about the performance information management, both after and before a stakeholder-startup relationship starts. The other topics are stakeholder-specific topics. For building programs, those are the Building Methods, Investor Involvement and Program Reporting. For investors the Engagement and Establishing a Relationship are important. For Business Angels in particular, Usage BA Funding and BA (network) Assessment are two very specific topics emerged in the data. What interview data belongs to the different code groupings will be discussed in the next section. </p><p>4.5. Interview Data This section will elaborate on the data, by describing what qualitative data is collected, in a structured way of conceptualization. The first part of the interview data will be on the three primary startup stakeholders (Building Programs, BAs, VCs), using the distinctive character of ‘main topics’ (Stakeholder Focus and SPIM) and ‘stakeholder-specific topics’ as a structure. The second part will consist of a short description on the remaining groupings (Experts, Framework and Tips/Hints/References). Although this section conceptualizes the raw data, it still is a lot of data. Therefore the subsequent section (4.2.3.) will summarize the most important part, the stakeholder part (including some references to the remaining groupings). </p><p>4.5.1. Stakeholder 1 - Building Programs [Incubators] 4.5.2. Stakeholder 2 - Investors [Business Angels] </p><p>4.5.3. Stakeholder 3 - Investors [Venture Capitalists] 4.6. Summary This section will consist of a summary of the data presented in the previous section, in order to provide quicker insights in the data. Stakeholder Focus . Stages o Incubators: early early-stage / early-stage. o BAs: seed/early stages. Define scope as: from vague idea to detailed business plans. o VCs: seed/early stages/late stage. Define scope as: from detailed business plan to at least market-fit. Stakeholders’ definitions on stages differ, hard to tie them to exact phases. . Sectors o Incubators unknown. o BAs: B2B seems most common. Mostly rely on expertise; however still 40% in unknown sectors. Then syndicate with expert investors is important. o VCs: Prefer one sector, however change is needed. Larger VCs broader portfolio. SaaS specialisation seems new for Dutch VCs.</p><p>SPIM before . Assessment Method o Incubators: unknown. o BAs: Tend to focus on the entrepreneur and his business plan o VCs: Already are monitoring the complete startup for multiple months. o BAs: Mainly focusing on meetings o VCs: Execute additional formal steps, like due diligence, checklists, talking to customers and competitors. The focus on assessment parts is different. The way of getting in contact is quite similar for both types of investors. . Assessment Information o INC 2: applicant screening on Fit & Viability, less focus on Team. Expert 2: Team for large Dutch accelerators most important. o BAs: Assess on (prioritized) factors. First feeling on Team, second the Product & Market estimation, and finally on appropriateness of Funding size, which can be objectively assessed. In the end, emotions are key in BA matching. o Most VCs: Team is the most important risk/success factor. Can be its perseverance and VCs feeling on it (subjective) or completeness (objective). Product and Market focuses on traction, growth rate, and product metrics, depending on startup stage. o BA + VC: both agree on optimistic character of business plans, i.e. sales stories, and state that proof of assumptions is something entrepreneurs need to use to receive funding. Example: VC 4 states that 95% of business plans or not realistic in terms of targets. VC 2 considers open invoices as an important characteristic of a startup. Corresponds to ‘Billings’ in stage-dependent-metrics. In general: assessment factors align with Expert 1, stating both criteria as well as gut feeling are involved. However, Expert 2 emphasises on using criteria through quantitatively assessing team (experience in leadership and academics, team size, team completeness, and timing). SPIM after . Format & Frequency o Incubators: Consider performance measurement as important and necessary. Lean startup methods are used to communicate information. Formal reports are considered as not suitable, therefore mostly it is done through conversations, in bi- weekly incubator meetings and meetings with coaches. Since progress is key, INC 2 wants to get more insights in the startup process, where quantification is considered as useful. o BAs: Different approaches, however in general a bi-weekly, monthly and/or quarterly overview of management issues, financials, etc. are required. The information they need to see is proof on the claims they make and just how the startups are doing. o VCs: Require monthly financial reports and quarterly management reports. Could be every format, no standardization is used. Dashboards including KPIs are defined in the official agreements, where larger portfolios may require higher-levels of detail. Milestones are an important part. </p><p>. Reporting Information o BAs: Less data and somewhat abstract on factors and metrics to measure. o VCs: Have more clear descriptions on KPIs (i.e. metrics), some which are included in the SPIM framework too. VC 4 believes these KPIs only apply to later stages, where even Efficiency sometimes can be still qualitative. According to what is stated in the assessment information part, the quantitative measurement of the Product & Market might be important, but VC 4 (and partly VC 2) claims Team as being the biggest risk factor for all stages.</p><p>. Information Asymmetry o BAs + VCs: Not considered as a problem, merely a characteristic of the relationship, where startups have detailed process or specific technological knowledge an investor does necessarily benefit by knowing it. o VCs: VCs characterized by investing in more mature startups: (1) simply can be too much information to handle as a VC and (2) recognize that even for CEOs it might be a challenge to get the right information. VCs therefore need to have a critical view on information provided. </p><p>. Intervention o Incubators: Focuses mainly on the personal level, where meetings are used to monitor progress, and 1-on-1 coaching can be a tool for intervention. o BAs: Focuses primarily on the performance of the management team, which can be replaced. o VCs: Mention budgets, revenue and costs as variable to obtain startup control. And, since VCs mostly participate in a supervisory board, mostly they are partly responsible for startup performance as well, and therefore are required to take action when that performance gets worse. o BAs + VCs: Participate until the moment when it turns out too bad and intervention does not help, then they pull the plug out and further participation stops.</p><p>. Intervention o Incubators: unknown. o BAs: Primarily want to add value, whereas their experience might be needed to participate (temporarily) in a startup. The group varies in BAs participating multiple days a week in a startup to BAs sharing their experiences and thoughts concerning the startup once a month. o VCs: Require more formal positions, like most of them fulfil a supervisory board position (depending on startup maturity), in order to act as a sounding board as well as a decision maker, together with other commissioners (not necessarily shareholders). VCs’ added value concerning knowledge is mainly in the assessment phase, where specific market knowledge is required. Engagement is different for BAs and VCs mainly in terms of informal versus formal. </p><p>Establishing Relationships . Formal Agreements o Incubators: unknown. o BAs: unknown. o VCs: Agreements consist of for example board positions and 100-day plans. Careful milestone setting is key in the process: a revenue number as milestone, for example, is a perverse incentive since revenue can be easily increased through hiring sales people. </p><p>. Exit Strategy o Incubators: unknown. o BAs: unknown. o VCs: Exit strategy is a part VCs differ in opinions. VC 2 believes exit strategy is a required part of the company assessment and agreements, whereas VC 3 believes exit strategy is something to only discuss as a venture capitalist team internally. VC 3 thinks it might be strange to discuss the strategy with the startup while knowing they just started their cooperation for (on average) five or six years, not foreseeing what will happen within these years. Only VC interviews contain data on this topic. This is probably due to two reasons: (1) the formal character of this topic, and (2) incubators and BAs are not participating anymore at the moment a company has its exit. Tips/Hints/References Most important in this part is the data retrieved from interviewing VC 4, who according to data on SPIM can be considered as most mature in evaluating and monitoring non-traditional startups. VC 4 states little is known in the Dutch ecosystem on SaaS startup investing in particular, compared to foreign investors. </p><p>5. SPIM Framework After describing the theoretical background on SPIM and the interview data focused on SPIM, this chapter will provide the resulting framework on SPIM. The framework, originating from a conceptual model, consists of a two-parts SPIM Framework (stakeholders overview and metric-base) and a practical SPIM Framework Application Model. As is described in the research method description in chapter 2, the framework is build upon two sources: the theoretical background and interview data. 1.16. Conceptual Model Before the first framework is developed, a conceptual model open to theoretical findings is designed, called the Startup Ecosystem Information Demand Framework (SEIDF). This particular conceptual model is depicted in figure 22.</p><p>Stakeholder (X)</p><p>X1 X2 … Xn</p><p>Startup Stage (Y) ? Y1</p><p>Y2</p><p>…</p><p>Yn</p><p>Fig. 20 – Conceptual Model for SEIDF In order to enable the conceptual model as being a starting point for the search for the final framework, a couple of questions related to the research questions are used: 1. Which Stakeholders are represented by columns {X1, X2, …, Xn }? 2. Which Startup Stages are represented by rows {Y1, Y2, …, Yn }? 3. Which information is demanded by different stakeholders {X} in the different startup stages {Y}, represented by the cells {[X1Y1], [X2Y2], …, [XnYn]},? Optional (related to research time left) are the following questions: 4. What information system (IS) (or IS design) enables optimal use of the SEIDF? 5. Which RISK/ROI-ratios are related to the different startup stages? 1.17. The Framework</p><p>5.1. Origination The first theoretical findings led to the first framework, therefore based purely on concepts derived from theory, which is the SEIDF (v. 0.1) (attached to this research in Appendix D). After conducting the interviews, the SEIDF evolved to v. 0.2, v. 0.3, and v. 0.4 (attached to this research in Appendices E, F, and G), to an SPIM framework, consisting of two parts: the first framework part focusing on stakeholders, and the second (main) framework part focusing on startup performance information. Both frameworks are depicted in respectively figure 21 and figure 22 (due to space both added after this chapter).</p><p>5.2. Framework Description First remark to make is that the final result differs from the preceding conceptual model and SEIDF frameworks. The main difference is the stakeholders and their specific information demand, in one framework, caused by the changes over time due to interview results. It turned out stakeholders are not specific enough in metrics they are focused on, only the stages they are involved in. Therefore the SEIDF with metrics tied to stakeholders changed to separate stages-stakeholder and stages-metrics framework parts. Still all parts are based on both the theoretical background as well as the interview results,</p><p>5.2.1. Part 1: SPIM Stakeholder Framework The first framework part is the SPIM Stakeholder Framework, representing which different startup stakeholders are involved in which particular startup stages (table 9). The upper part represents the four Marmer stages representing the main startup-building processes (Discovery, Validation, Efficiency, and Scale), and the corresponding three main milestones a startup wants to reach (the search for Problem/Solution Fit, Product Market Fit and Growth). The factor Time (in months) is based upon findings concerning average time per stage, from the research among startups by Marmer et al. (2011) as 6.2 described. Obviously that number of months mostly can be seen as an impression how long the stages can take instead of being a rigid guide, since those numbers are average numbers. Also part of it is the Startup focus activities part (where reaching milestones also does belong to). The bottom part represents the main part of the framework: which Stakeholders belong to which Startup stages, tied to investment risk percentage per stage, as being discussed in 3.5.2. All components are based on both the theoretical background as well as the interview results.</p><p>Startup stages Discovery Validation Efficiency Scale</p><p>Problem/Solution Fit Product/Market Fit Startup focus Growth actitvities search search (information) Problem clear First channels Solution Hiring Customer Segment clear MVP building Revenue stream in place Negative churn in place MVP testing Cost structure in place Market expanse Solution definition Improving Channels Table 10 – Framework on Startup Stages – Startup Stakeholders Engine of Growth Negative Churn </p><p> roadmap</p><p>B u Incubator i l Accelerator d i n g</p><p>O r g a n Venture Builder i Stakeholders z a t i o n s I n Business Angel(s) v e s t Venture Capitalist(s) o r s Investment Risk 66,2 % 53.0 % 33.7 % 20.1% One extra remark is needed concerning the iterative character of the processes. As is discussed before in this research, pivots for example are common in building a well- performing startup. Therefore, processes are not interpretable that linear as they are visualized. The arrows at the Validation phase visualize the same aspect: it marks the iterative character of MVP building and testing. </p><p>5.2.2. Part 2: SPIM Metrics Framework (i.e. SPIM Metrics Base) The upper part of the second part, consisting of the startup stages, milestones and focus activities are similar as in the previous Stakeholder Framework. However, since this framework focuses in particular on performance measurement within SPIM, the rows represent the Stage-dependent Metrics and General Metrics, to in the end serve as a SPIM metrics base. The framework can be used first to check where a startup is when checking the activities. Concerning those activities, a startup, startup-building organisation, and external stakeholders are able to do a first evaluation of a startup using the related metrics (i.e. KPIs) to check both how a startup performed in previous periods, both in the short-term (stage-dependent metrics) as well as the long-term (general metrics). Also, the stage-dependent and general metrics can be used to monitor the startup performance for respectively on a short-term basis as well as on a long-term basis, both enabling intervention on the short-term as well as enabling more accurate prediction for the future, in order to be able aligning the building and investing strategy to those results. Startup stages Discovery Validation Efficiency Product/Market Fit Problem/Solution Fit search search Startup focus Problem clear First channels Solution Customer Segment clear MVP building Revenue stream in place actitvities MVP testing Cost structure in place (information) Solution definition Improving Channels Engine of Growth Negative Churn roadmap</p><p>Customer Survey Statistics (%) Attention (# visitors) Customer Interview Score (0-35) Enrolment (%) Validation Production + Conversion rates Customer Lifetime Value (CLV) (€) (approaches/week -> interviews/week) Revenue (per customer, or MRR, or ARR) Interview Production + Conversion rates # customers (problem interview -> solution interview -> sale) Billings (no) Stickiness (# users) User Acquisition Cost (€) Customer Acquisition Cost (CAC) (€) Metrics MVP acceptance score (%) CLV: CAC (ratio) Churn (users/month) Churn (customers/month) Stage-dependent Feature Utilization (%) Uptime & Reliability (%) Engine of Growth (ratio) (data) Up-selling (frequency) Cross-selling (frequency) API traffic (%) Ecosystem Relationships (no.) Ecosystem Effectiveness (%) Competitors (no.) Support Costs (€) Compliance (%) New Markets (no.) Sales Force (FTE)</p><p>The next page will continue on the Framework, where the General Metrics will be discussed. Startup stages Discovery Validation Efficiency Scale Metrics - Mgmt. Team Size (no.) general Mgmt. Team Experience (corporate/entrepreneurial/none) (data) Mgmt. Team Background (academic degree) Existing Alternatives (no.) Burn Rate (cash/week,-/month) Runway (months) Short-term hiring plans (yes/no) Time-to-break-even (months) Pivots (no.) Learning (metrics quality) Learning (mentor help) Learning (Lean method(s) usage) Funding Raised (€) FTE's (no.) SPIM Framework continued:</p><p>5.3. Framework Application Before being able to provide advice on a particular information system aiming at enabling the implementation of all these metrics within a startup ecosystem, or even developing such a system, one necessary phenomenon will be described first: a method to be able to choose out of all these metrics the useful ones, at distinct times and situations, i.e. picking the right metrics. First the goals and reasons of picking those appropriate metrics will be described, followed by an application model to support that particular process.</p><p>5.3.1. Background Picking the Right Metrics All those metrics in the previous sections seems to be useful. However, after describing all those metrics for the four different stages, this research will also include more on focus: the Table 11 – Startup Performance Information Management (SPIM) Framework main goal is to raise awareness among researchers, entrepreneurs and investors on the importance of picking the right metrics at the right time, instead of using as much metrics (i.e. data) as possible, resulting in the end in the risk of drowning in all that data. Therefore, as Maurya (2012) and other industry experts describe, it is important of knowing which metrics can be considered as actionable metrics and which ones as vanity metrics. Difference is where the actionable metrics’ observed results are tied to specifically and repeatable actions. Vanity stands for the opposite, a metric also defined to observe results, but which is not usable to base actions on. An example of how the lean startup methods can lead to failure will be described first. The lean startup methods have space left for pivots, since is a highly iterative approach (3.2.2.). The risk of such an approach and the characteristics of entrepreneurs may lead to try new things, instead of using a methodical way to eliminate risk (Croll & Yoskovitz, 2013). For example, trying each of the engines of growth to obtain growth, instead of focussing by choosing just one engine of growth and stick to that one (Ries, 2011). Focus is considered by Croll and Yoskovitz (2013) as the key to startup success, therefore they initiated a discipline they call the One Metric That Matters (OMTM), applicable within the lean startup methods. They define it as “the one number you’re completely focused on above everything else for your current stage” (Croll & Yoskovitz, 2013, p.56). It is like choosing out of the most important metrics (Key Performance Indicators) one a startup will focus on. That approach provides guidance in the web of all different metrics for all different business models at different stages. Picking the right OMTM, changing over time, is effective for four different reasons (Croll & Yoskovitz, 2013, p.58): - It answers the most important question you have. The entrepreneur needs to identify the riskiest part of the business, and that’s where the most important question lies. When the entrepreneur knows what the right question is, he will know what metric to track in order to answer that question. - It forces you to draw a line in the sand After the entrepreneur identified the key problem on which he wants to focus, he needs to set goals. - It focuses the entire company. Display your OMTM prominently through web dashboards, on TV screens or in regular emails. - It inspires a culture of experimentation Experimentation is what all of the lean startup methods encourage. When everyone rallies around the OMTM and is given the opportunity to experiment independently to improve it, it is a powerful force. Four characteristics (i.e. quality criteria) of a good metric are defined by (Croll & Yoskovitz, 2013, p.9-11). A metric must be: - Comparative: Being able to compare a metric to other time periods, groups of users, or competitors, helps you understand which way things are moving. - Understandable: If people can’t remember it and discuss it, it’s much harder to turn a change in the data into a change in the culture. - A ratio or a rate: Ratios or rates tend to be easier to act on, they are inherently comparative (see the first characteristic above) and they are good for comparing opposing factors. - Changes the way you behave: What will you differently based on changes in the metric? If a startup has chosen its OMTM, what is next? To gain insights in making such a OMTM actionable, Graham (2012) will be used. Graham (2012), founder of Y Combinator, the example accelerator described in 3.5., wrote an essay on startups and growth. He also advocates the importance of startup focus, and therefore describes growth rate as the number every founder always should know. It is the measure of a startup to see if you are doing well or badly. In the Y Combinator accelerator program, growth rate is measured each week. Partly because of the limited time period of the building program, partly because feedback every week can keep startups on the right path in the early-stage. He argues that the primary metric you need to focus on is Revenue, and the secondary best metric is the number of active users when a startup is not charging money initially (Y Combinator guides startups which already found P/M fit and therefore making revenue) (Graham, 2012). A startup needs to pick the growth rate they think they can hit for that week, and fully focus on that rate. Bottom line for the weekly growth rate is 5-7%, exceptionally well is a growth rate of 10% per week. Lower than 5% tells the founders they have not figured out the riskiest parts of the business model. Correspondence on that growth rate takes place each week, to be able to change directions in time, or continue growing on that particular growth rate (Graham, 2012) Although a weekly growth rate focuses obviously on weekly growth, it still affects the long- term decisions. Graham (2012) uses the example of hiring a programmer to implement a new feature, to perhaps reach the growth rate in a month when the startup did not reach it that particular week. Such a decision is only allowed when the startup (a) does not miss its’ growth rate number in the short term, and (b) the startup is worried enough of not keeping to hit the growth rates without a new hire. This decision reflects the positive influence of forcing a startup to discover new ideas as part of an evolutionary process, to in the end staying on track concerning reaching the identified growth rate (Graham, 2012). 5.3.2. SPIM Framework Application Model As the previous section discussed, the appropriate weekly picked metrics (OMTMs) bring focus to startup founders (and other stakeholders), which is considered as key in startup success. Therefore this chapter will close with an application model, based on Croll and Yoskovitz (2013), describing a method supporting stakeholders in order to bring the ‘picking the right metrics’ approach into practice. An important note on this SPIM Framework Application Model is that it primarily aims at application of the stage-dependent metrics and less at general metrics, since the latter always remain the same for every startup stage and therefore less relevant in the weekly iterative process of picking the right metrics.</p><p>Fig. 21 – Method supporting picking the right metrics The method depicted in figure 23 shows an iterative, Lean Startup corresponding approach, to enable picking a (weekly) metric: the OMTM. Which supports the testing of hypothesis through measuring results on the OMTM tied to that particular hypothesis. Bottom-lines are useful in determining whether a hypothesis can be validated or invalidated. The cycle time preferably is a weekly period. Both the prescribed growth rate and cycle time-period are corresponding to best practices from Y Combinator as described in the previous section. This part will elaborate on the steps in the model. (1) The process starts with formulating the hypothesis, as the core of the iterative Lean Startup process. (2) Then a related OMTM needs to be picked from the SPIM Metrics base, based on two startup characteristics: the Business Model, in this particular research SaaS, however further research can extend to other business models, and the Stage a startup is in. (3) This metric needs to be checked on the quality criteria as described 5.3.1.. (4) Thereafter a bottom-line will be used in order to avoid unbound data measurement. As a bottom-line, two possible ways exist: the weekly growth rate of 5-7% for a particular metric as described in the previous section, or keeping track of the Lean Analytics bottom-lines related to the metrics as described in 3.6.3.3 (however the latter merely are characterised by long term measurements). However, the iterative character of the model does also suggest space for improvements, where an improved bottom-line might be more appropriate in some situations than theory provides. (5) Measuring the results enables the startup stakeholders to prove whether the formulated hypothesis from the first step can be considered as (6a) validated or (6b) invalidated. (6a) Validation leads to (7) communicating the results to the stakeholders involved, where after the cycle can be repeated with a newly formulated hypothesis. However, when a hypothesis is considered as invalidated (6b), two options are available to the stakeholder: - The stakeholder (8) gives up and (7) communicates this event to the stakeholders involved, where after a new and maybe more realistic hypothesis will be formulated. - The stakeholder returns back in the process to (5) measuring for another time- period (preferably week) until the formulated hypothesis can be considered as validated.</p><p>6. Conclusions This chapter will provide conclusions on the research. The different research questions will be answered in order to provide an answer to the central question in this research, which is: </p><p>The first part of the research is the startup building part, consisting of three research questions, to gain insights in the characteristics of startups, their building process and the ecosystem they operate in. The first question to answer is what startup building methods do exist. The second question deals with the structure of the startup lifecycle (phases and stages). Both will be answered simultaneously, since they are that much interrelated, however there might be a slight difference between stages serving as building method components and phases referring to the startup lifecycle. Where after an answer will be provided to the third question concerning building organizations. Startups were built through traditional methods (like waterfall) before, where currently non- traditional methods (like the Lean Startup) have taken over. From the different (non- traditional) building methods (i.e. frameworks), the six most common known ones are included, conceptualized through different stages. Most method stages are named differently, however the content of the stages target mostly similar parts, since they are mostly based on the Lean Startup method (Ries, 2011). The ones selected for further use in this research are the Lean Analytics method, developed by Croll and Yoskovitz (2013) and the Marmer stages, developed by Marmer et al. (2011). Important to keep in mind is the iterative character of the different frameworks, since pivots, i.e. major changes in the business, are normal. Marmer et al. (2012) even found that startups pivoting once or twice raise more money, have better user growth and are less likely to scale prematurely than startups pivoting more than 2 times or not at all. The three main milestones Problem/Solution Fit, Problem/Market Fit and Growth (Maurya, 2012) align seamlessly to the four Marmer stages. Therefore both frameworks are used in the target SPIM framework. Reaching the P/S Fit milestone means you have significantly identified your customer segment and a vision on a possible solution. Reaching P/M Fit means the solution, in this stage represented by an MVP, is sufficiently validated in the market. What follows is the Growth phase, where its first important part is preparation for the growth, i.e. Scale phase, through the Effiency phase. This is highly important considering on of the major findings of Marmer et al. (2011), and Croll & Yoskovitz (2013) stating that premature scaling, assuming there is Product/Market Fit and leaving the Validation/Stickiness phase too early, by going to the Scale/Virality phase, emerged as the most common reason for startups to fail. Interview results show the focus of venture-building organizations towards using those (non- traditional) methods and lifecycle descriptions, whereas other stakeholders, investors for example, communicate using their own methodologies. They are less used to the Lean Startup terms and more convenient using terms like for example Seed, Early-Stage, and Late-Stage. Theory shows the methodologies to describe such a perspective on startup lifecycles is used for a couple of decades already, Ruhnka and Young for example (1987), and therefore can be determined as being a more traditional perspective. The third question is to search for a detailed descriptive background on the startup ecosystem, e.g. the building organizations and the related stakeholders. As the introduction described, Cohen and Hochberg (2014) claim little research is done in the field of startup building programs, both descriptive and result oriented. Therefore the research has separated and described three types of startup building organizations, the Incubator, Accelerator, and Venture Builder, whereas for the last one a ecosystem diagram and a first definition is provided. The ecosystem consists of different types of stakeholders, where the research has focused on the Investors. Findings on the positive association between receiving funding and startup growth are described. The investor types this research focuses on are: BAs, the independent wealthy individuals, and VCs, the professional investment funds. BAs tend to focus on the Seed and Early Stage phases of a startup, where VCs tend to invest later, in Early Stage and Later Stages. Important finding is the investment risk factors similarity to startup success factors. The research overview of risk factors provided a prioritized categorization for those success factors, which are: (1) Team, (2) Product & Market, and (3) Startup. Findings from interviews show similar distinctions in success factors categories. Those risk (or success) factors needs monitoring, however early-stage companies do not have historical information to provide to investors for most of the different factors, which also is stated by stakeholders through the interviews. Therefore Gompers (1995) argues that investors need to invest in monitoring, where Davila and Foster (2005) found that involvement of VCs brings formal monitoring (information systems) for the first time to startups. In line with these findings, this particular research has tried to figure out a way of bringing that formal monitoring to startups earlier, when, for example, still in the Discovery or Validation phase. Therefore, the term ‘SPIM’, Startup Performance Information Management emerged. The second part of the research elaborated on that type of information management, SPIM, which is the performance measurement and information management of startups. This part elaborates on SPIM, the search for supporting information systems, and a final framework as a result of both the first and the second part of the research. The fourth research question deals with the definition, purpose and content of SPIM. SPIM is defined by bringing several definitions together. SPIM is the collection and communication of performance information produced through the execution of Business Performance Management, in order to make quicker and better decisions. To implement information systems in order to digitalize this process, first an understanding of the underlying problems, technical elements and the organizational processes is needed; therefore this research started with describing the startup and related building organizations background. The main goal of the research is to improve startup performance, where indeed Taticchi et al. (2010) argue performance measurement is a crucial element. Eight advantages of performance measurement are described in the same chapter, explaining how metrics contribute to both clear communication between employees and company success. The focus in performance measurement has shifted from a mainly measurement of financials towards a more measurement of innovation. This can be seen as a shift corresponding to the (apparently still existing) difference between the (traditional) investor perspective on startups and the (non- traditional) venture-building organizations perspective on startups. The metrics derived from research are divided into general metrics and stage-dependent metrics. General are the ones involved at all stages of a startup, divided in line with the success factor categorization from previous literature: Team, Product & Market, and Startup. Whereas stage-dependent are the ones especially designed to be used for one or more stages, divided in line with the four Marmer stages (Discovery, Validation, Efficiency and Scale). Croll and Yoskovitz (2013) consider focus as key to startup success; therefore picking the right metric (OMTM) and focusing on growth for that metric is a necessary part of the process of using SPIM in startups. The fifth question tries to search for an answer concerning SPIM supporting information systems (i.e. tools). Davila and Foster (2005) found a positive association between the adoption of management control systems and (at least) two startup characteristics: startup growth and CEO tenure length. Therefore the section on existing SPIM tools is included, consisting of descriptions on the Lean Canvas, Validation Board and Lean Dashboard, chosen based on several requirements. All of them support a part of the Lean Startup methods in a certain way, however therefore not being solutions for all the processes, in particular SPIM concerning metrics. After the interview results are included, the last research question can be answered. The sixth research question deals with searching for a way of modelling the SPIM information in a SPIM framework. That process started with a conceptual model, which has evolved over the time of research towards the final SPIM framework, consisting of two parts, one on the stakeholders and one on the SPIM itself. The first part consists of the four Marmer startup- building stages, three Lean lifecycle phases (or milestones), corresponding startup focus activities and stakeholders tied to these stages and phases. The second part focuses on SPIM, consisting of the four Marmer startup-building stages, three Lean lifecycle phases (or milestones), corresponding startup focus activities too. But in this framework the stage- dependent and general metrics tied to these stages and phases are included, in order to enable SPIM, which supports performance information management and growth of startups within venture builder ecosystems.</p><p>7. Discussion This chapter will discuss the limitations and provide recommendations for further research. 1.18. Limitations Startups built through Lean methods (non-traditional), as well as related building organizations, are not common subjects in scientific research yet, as claimed by Blank (2013) and Cohen and Hochberg (2014). Therefore this research can be considered mainly as exploratory research, exploring non-traditional concepts, combining it with traditional perspectives on these concepts. The largest limitation therefore was concerning formulating the right and feasible research questions taking the (then partly unknown) scope in consideration. One of the main limitations related to this aspect, is finding the right stakeholders for relevant data collection. Finding individual Business Angels is hard and perhaps might be not sufficient in order to represent the heterogeneous BA market. Therefore BA networks are used as representatives for population. However, these network founders know less about the topic of how BAs monitor the performance of startups after the investment is done. Some field of tension on formulating conclusions on BAs emerged there. Also concerning the stakeholders, a limitation is the fact that besides the (relative young) host company, venture builders are not common in the Dutch startup ecosystem, let alone experienced ones. Therefore, only building programs could be the subjects of interview, whereas incubators were easier to approach as accelerators were: none of the (multiple) approached accelerators had the ability to cooperate in this research. The main research question therefore is answered for supportive startup ecosystems instead of venture builders in particular. A related ecosystem limitation is considering the external validity, whereas only data on Dutch stakeholders is gathered, and none of the conclusions therefore are directly applicable on non-Dutch stakeholders. Which corresponds to the findings in data on VC 4, who mentions that this research, partly consisting of SaaS investing, needs to involve foreign investors, concerning knowledge and experience aspects on SaaS. For example, only data on VC 4 shows they are using the currently recommended metrics, which also are the ones described by the modern blogs nowadays, since VC 4 mentions he dived into recent theory. Also on the general metrics not much non-traditional approaches, for example quantification, are used. For example, only Expert 2 talked about quantification of Team aspects. Feedback concerning those kinds of issues is not gathered through a second round after the first round of interviews. The only verification done afterwards through email is on the tools VC 3 uses. Another main limitation, related to the exploratory character of the research, is concerning the way the framework is formed. After doing interviews, the framework seems to be not that much focused on stakeholders anymore, like the conceptual model was, since not all of them are familiar with the non-traditional ways startups are being build with currently, and the corresponding metrics. Therefore, tying the stakeholders and their exact information demand to the different stages for those stakeholders is hard, where the latter even more, is impossible when taking significance of conclusions into consideration. The research tried to come up with two parts of a complete framework, one on stakeholders and one on performance information. Perhaps, tying different abstraction levels for different stakeholders to information would be more convenient. Due to the limited research timeframe, unfortunately research on which Information System would enable digitalizing the use of SPIM, and therefore contribute to startup growth, could not be executed. 1.19. Further Research This section will provide recommendations for further research. The first important aspect to elaborate on in future research is the non-traditional startup movement, the Lean Startup, related to both new ways of SaaS startup building as well as new perspectives investors are going to need when investing in these specific startups. Another recommendation is on including other streams of theory, which could be for example on the use of Knowledge Management theory: the theory of Nonanka might be useful in describing how to transform implicit to explicit information, especially regarding startup team aspects. The third recommendation is concerning the stakeholders, whereas the perspective of entrepreneurs themselves on the framework might be interesting, in order to improve this first SPIM framework. Also a feedback session on the SPIM framework with Building Organizations and Investors would provide a more accurate framework. Another recommendation is further research into the framework metrics: the level of detail, data quality/privacy, metrics focus, and further implications. Concerning this level of metrics/data detail requested by stakeholders, some of them mentioned a difference in detail level of information: VCs with large portfolios might focus more on high-level financials compared to VCs with small portfolios, due to simply a difference in level of involvement and available monitoring time. Rules concerning quality/privacy rules will be interesting in further research as well, when further evaluating and implementing the framework. The aspect concerning, focus: BAs with less technological knowledge might focus only on business model metrics, and less on product metrics, as interview tips suggest. Another aspect to elaborate on in further research is the usage of metrics tied to the prediction of startup success. Some bottom-lines representing a healthy startup already are provided in this research, although further research might lead to more and proven bottom-lines on the long-term in order to be useful for predictions. The last recommendations is on practical implications of the SPIM framework, where research is needed in order to investigate whether it could serve as a foundation for a SPIM Information System in order to bring performance measurement, startup growth, information management systems, and improved stakeholder involvement into practice.</p><p>8. References Angel Capital Association (2014). Important Things to Know About Angel Investors. Retrieved September 2015, from http://www.angelcapitalassociation.org/data/Documents/Resources/ Andreessen, 2007. The pmarca guide to startups. Retrieved July 2015 from http://pmarchive.com/guide_to_startups_part4.html. Baum, J. A., & Silverman, B. S. (2004). Picking winners or building them? Alliance, intellectual, and human capital as selection criteria in venture financing and performance of biotechnology startups. Journal of business venturing, 19(3), 411-436. Business Dictionairy (2015). Information Management. Retrieved June 2015 from http://www.businessdictionary.com/definition/information-management.html. Blank, S. (2006). The Four Steps to the Epiphany: Successful Strategies for Products that Win. CA: Cafepress.com. Blank, S. & Dorf, B. (2012). The startup owner’s manual: The step-by-step guide for building a great company. CA: K&S Ranch. Blank, S. (2013). Why the lean start-up changes everything. Harvard Business Review, 91(5), 63-72. Blank (2013a). Reinventing Life Science Startups – Evidence-based Entrepreneurship. Retrieved June 2015 from http://steveblank.com/2013/08/21/reinventing-life-science-startups- evidence-based-entrepreneurship-2/ Blank (2013b). It’s Time to Play Moneyball: The Investment Readiness Level. Retrieved June 2015 from http://steveblank.com/2013/11/25/its-time-to-play-moneyball-the-investment- readiness-level/ Boeije, H. (2002). A purposeful approach to the constant comparative method in the analysis of qualitative interviews. Quality and quantity, 36(4), 391-409. Cann, van R., Jansen, S., & Brinkkemper, S. (2012). Software Business Start-up Memories: Key Decisions in Success Stories. NY: Palgrave Macmillan. CBInsights (2015). The Unicorn List: Current Private Companies Valued At $1B And Above. Retrieved December 2015, from https://www.cbinsights.com/research-unicorn-companies. Chaffey, D., & Wood, G. (2004). Business information management: improving performance using information systems. Essex: Prentice-Hall. Chang, C. (2013). Portfolio Company Selection Criteria: Accelerators vs Venture Capitalists. Claremont McKenna College Senior Theses. Paper 566. Retrieved December 2015 from http://scholarship.claremont.edu/cmc_theses/566 Cocca, P., & Alberti, M. (2010). A framework to assess performance measurement systems in SMEs. International Journal of Productivity and Performance Management, 59(2), 186-200. Cohen, S., & Hochberg, Y. V. (2014). Accelerating startups: The seed accelerator phenomenon. Available at SSRN 2418000. Croll, A., & Yoskovitz, B. (2013). Lean analytics: Use data to build a better startup faster. CA: O'Reilly Media. Davila, A., & Foster, G. (2005). Management accounting systems adoption decisions: evidence and performance implications from early-stage/startup companies. The Accounting Review, 80(4), 1039-1068. Davila, A., Foster, G., & Gupta, M. (2003). Venture capital financing and the growth of startup firms. Journal of business venturing, 18(6), 689-708. Davila, A., Foster, G., & Jia, N. (2010). Building sustainable high-growth startup companies: Management systems as an accelerator. California Management Review, 52(3), 79–105. EF (2015). Our Programme. Retrieved June 2015 from http://www.joinef.com/our- programme/. Ehrlich, S. B., De Noble, A. F., Moore, T., & Weaver, R. R. (1994). After the cash arrives: A comparative study of venture capital and private investor involvement in entrepreneurial firms. Journal of Business Venturing, 9(1), 67-82. Ellis, S. (2009). The Startup Pyramid. Retrieved June 2015 from http://www.startup- marketing.com/the-startup-pyramid/ Feld, B. (205). The Illusion of Product/Market Fit for SaaS Companies. Retrieved July 2015 from http://www.feld.com/archives/2015/01/illusion-product-market-fit-saas-companies.htm Fishback, B., Gulbranson, C. A., Litan, R. E., Mitchell, L., & Porzig, M. A. (2007). Finding Business' Idols': A New Model to Accelerate Start-Ups. Fowler, M., & Highsmith, J. (2001). The agile manifesto. Software Development,9(8), 28-35. Franco-Santos, M., Kennerley, M., Micheli, P., Martinez, V., Mason, S., Marr, B., ... & Neely, A. (2007). Towards a definition of a business performance measurement system. International Journal of Operations & Production Management, 27(8), 784-801. Franke, N., Gruber, M., Harhoff, D., & Henkel, J. (2008). Venture capitalists' evaluations of start‐up teams: trade‐offs, knock‐out criteria, and the impact of VC experience. Entrepreneurship Theory and Practice, 32(3), 459-483. Gorman, M., & Sahlman, W. A. (1989). What do venture capitalists do?. Journal of business venturing, 4(4), 231-248. Graham, P. (2012). Startup = growth. Retrieved June 2015 from http://www.paulgraham.com/growth.html Gompers, P. A. (1995). Optimal investment, monitoring, and the staging of venture capital. Journal of finance, 1461-1489. Gross, B. (2015). The single biggest reason why startups succeed. Retrieved December 2015, from https://www.ted.com/talks/bill_gross_the_single_biggest_reason_why_startups_succeed/. Hawley, R. (1995). Information as an asset: the board agenda. Information management and technology, 28(6), 237-239. Hellmann, T. (2002). A theory of strategic venture investing. Journal of Financial Economics, 64(2), 285-314. Hellman, T., & Puri, M. (2000). The interaction between product market and financing strategy: The role of venture capital. Review of Financial studies,13(4), 959-984. Kaplan, S. N., & Strömberg, P. E. (2004). Characteristics, contracts, and actions: Evidence from venture capitalist analyses. The Journal of Finance,59(5), 2177-2210. Larman, C., & Basili, V. R. (2003). Iterative and incremental development: A brief history. Computer, (6), 47-56. Laudon, K. & Laudon, J. (2006). Management Information Systems: Managing the Digital Firm, 9th ed. New Jersey: Prentice Hall. Lean Startup Machine (2012). Validation Board. Retrieved December 2015 from https://www.leanstartupmachine.com/validationboard/ Marmer, M., Herrmann, B. L., Dogrultan, E., Berman, R., Eesley, C., & Blank, S. (2011). Startup Genome Report Extra: Premature Scaling. Mason, C. M., & Harrison, R. T. (2002). Is it worth it? The rates of return from informal venture capital investments. Journal of Business Venturing, 17(3), 211-236. Mattermark (2014). For Y Combinator Startups, the Average Series A Round in 2014 is 5X Larger Than in 2008. Retrieved December 2015, from http://mattermark.com/y-combinator- series-a-average-round-size/ Mattermark (2015). A Decade of VC Funding History: Trend Analysis 2005 to 2014. Retrieved December 2015 from http://mattermark.com/a-decade-of-vc-funding-history-trend- analysis-2005-to-2014/ Maurya, A. (2010). Running Lean: A Systematic Process for Iterating Your Web Application from Plan A to a Plan That Works. CA: O'Reilly Media. Maurya, A. (2013). The Customer Factory. Received October 2015 from http://leanstack.com/new-book-the-customer-factory/ Maxwell, A. L., Jeffrey, S. A., & Lévesque, M. (2011). Business angel early stage decision making. Journal of Business Venturing, 26(2), p. 212-225. McClure, D. (2007). Startup Metrics for Pirates: AARRR!!!. Retrieved June 2015 from http://www.slideshare.net/dmc500hats/startup-metrics-for-pirates-long-version Megginson, W. L., & Weiss, K. A. (1991). Venture capitalist certification in initial public offerings. Journal of finance, 879-903. Miller, P., & Bound, K. (2011). The Startup Factories: The rise of accelerator programmes to support new technology ventures. NESTA. Miloud, T., Aspelund, A., & Cabrol, M. (2012). Startup valuation by venture capitalists: an empirical study. Venture Capital, 14(2-3), p. 151-174. MyGlobalIT, 2013. Waterfall vs Agile Models in Software Development. Retrieved May 2015 from http://www.myglobalit.com/blog/waterfall-vs-agile-models-software-development. Neely, A.D., Mills, J.F., Gregory, M.J., Platts, K.W. (1995). ‘Performance measurement system design: a literature review and research agenda’, International Journal of Operations and Production Management, 15(4), 80–116. Neely, A. D. (2002). Business performance measurement: theory and practice. Cambridge: Cambridge University Press. Graham, P. (2012). Startup = growth. Retrieved June 15 from http://paulgraham.com/growth.html Radojevich-Kelley, N., & Hoffman, D. L. (2012). Analysis of accelerator companies: An exploratory case study of their programs, processes, and early results. Small Business Institute® Journal, 8(2), p. 54-70. Ries, E. (2011). The Lean Startup. New York, NY: Crown Business. Rohé, B. (2012). The Definition Of #Berlin Incubators, Accelerators And Company Builders Might Get You Confused. Retrieved May 2015 from http://www.makeastartup.com/the- definition-of-berlin-incubators-accelerators-and-company-builders-might-get-you-confused- 2-new-founder-programs-startups/ Rogers, E. M. (2010). Diffusion of innovations. Simon and Schuster. Startup Commons (2015). Startup Development phases. Retrieved June 2015 from http://www.startupcommons.org Singer, J., Sim, S. E., & Lethbridge, T. C. (2008). Software engineering data collection for field studies. In Guide to Advanced Empirical Software Engineering, 9-34. London: Springer. Skok, D. (2013). SaaS Metrics 2.0 – A Guide to Measuring and Improving what Matters. Retrieved October 2015 from http://www.forentrepreneurs.com/saas-metrics-2 Stair, R. M., Reynolds, G., & Chesney, T. (2008). Fundamentals of business information systems. Boston: Cengage Learning. Taticchi, P., Tonelli, F., & Cagnazzo, L. (2010). Performance measurement and management: a literature review and a research agenda. Measuring business excellence, 14(1), 4-18. Tyebjee, T. T., & Bruno, A. V. (1984). A model of venture capitalist investment activity. Management science, 30(9), 1051-1066. Urquhart, C., Lehmann, H., & Myers, M. D. (2010). Putting the ‘theory’ back into grounded theory: guidelines for grounded theory studies in information systems. Information systems journal, 20(4), 357-381. Van de Weerd, I., & Brinkkemper, S. (2008). Meta-Modeling for Situational Analysis and Design Methods. In Handbook of Research on Modern Systems Analysis and Design Technologies and Applications, 35–54. Hershey: Idea Group Publishing. Wickman, G. (2012). Traction: Get a Grip on Your Business. Dallas: BenBella Books, Inc.. Appendix A – Discovery Interview Question 1. Did the interviewee successfully rank the problems you presented? Yes The interviewee ranked the problems with strong interest 10 (irrespective of the ranking) Sort of He couldn't decide which problem was really painful, but he was 5 still really interested in the problems No He struggled with this, or he spent more time talking about other 0 problems he has 2. Is the interviewee actively trying to solve the problems, or has he done so in the past? Yes He's trying to solve the problem with Excel and fax machines. You 10 may have struck gold Sort of He spends a bit of time fixing the problem, but just considers it the 5 price of doing his job. He's not trying to fix it. No He doesn't really spend time tackling the problem and is OK with 0 the status quo. It's not a big problem 3. Was the interviewee engaged and focused throughout the interview? Yes He was hanging on you every word, finishing your sentences, and 8 ignoring his smartphone. Sort of He was interested, but shows distraction or didn't contribute 4 comments unless you actively solicited him. No He tuned out, looked at his phone, cut the meeting short, or 0 generally seemed entirely detached--like he was doing you a favour by meeting with you 4. Did the interviewee agree got a follow-up meeting/interview (where you'll present your solution)? Yes, without being He's demanding the solution "yesterday" 8 asked to Yes, when you He's OK with scheduling another meeting, but suddenly his calendar 4 asked him to is booked for the next month or so. No You both realize there's no point showing him anything in terms of 0 a solution 5. Did the interviewee offer to refer others to you for interviews? Yes, without being He actively suggested people you should talk to without being 4 asked to asked. Yes, when you He suggested others at the end, in response to your question. 2 asked him to No He couldn't recommend people you should speak with. 0 6. Did the interviewee offer to pay you immediately for the solution? Yes, without being He offered to pay you for the product without being asked, and 3 asked to named a price. Yes, when you He offered to pay you for the product. 1 asked him to No He didn't offer to buy and use it. 0</p><p>Appendix B – Interview Protocol (Startup Building Organizations) Introduction - Graduation Research Business Informatics master, Utrecht University. - Research Goals o Startup and Stakeholders insights . Startup lifecycle . Stakeholders o Startup Ecosystem Information Management insights . Providing stakeholders better and possibly new insights into startup de building & investing - Show Target Framework - Reason to interview that particular interviewee</p><p>Stakeholders 1. Which stakeholders are the most important ones in the startup building process?</p><p>Startup lifecycle - General 2. In which stage of their lifecycle is a startup when applying to your program? 3. Which type of startups do you build? / For which sectors?</p><p>- Method 4. Which startup building method do you use for venture building? 5. What makes this particular method suitable for you? 6. To which extent are you, as an incubator/accelerator, planning your milestones?</p><p>Stakeholder [building organization] Information 7. What information/data are you asking for in the different stages of your startups? To which extent Quantitative / Qualitative? 8. Which consequences are the result of that data? For example: Go’s/No-go’s, etc.? 9. How is the team formed / assessed, before/shortly after applying for the programme? 10. How do you monitor team performance during their stay at your startup-building program/organization? </p><p>Stakeholder [Investor] Information 11. Are there any investors involved in the process, and if so, from which point in the startup lifecycle are they involved? 12. Are these investors Business Angels or Venture Capitalists? 13. What information/data do these investors ask for? 14. To which extent is that information/data different from what you are asking for? 15. Which role is assigned to investors within a startup?</p><p>Appendix C – Interview Protocol (Investors) Introduction - Graduation Research Business Informatics master, Utrecht University. - Research Goals o Startup and Stakeholders insights . Startup lifecycle . Stakeholders o Startup Ecosystem Information Management insights . Providing stakeholders better and possibly new insights into startup de building & investing - Show Target Framework - Reason to interview that particular interviewee</p><p>Stakeholder(s) Role 1. Are the (framework) included stakeholders the most important ones in the startup building process? 2. In which stage of their lifecycle is a startup when applying to your program? 3. Which startup sectors do you invest in?</p><p>Startup Performance - Before Investment 4. What information (or factors) do you need to evaluate an unknown startup on? (Check mentioning Startup & Team) 5. How do you do that evaluation research? (Check Informal meeting(s), Due diligence, etc.)</p><p>- After Investment 6. What information/data are you asking for in the different stages of your startups? (Check - Basic: Example. No. of customers, Revenue, Profit & Loss (snapshot) - Advanced: Data driven, customer data (real-time KPIs) (period) ) 7. To which extent is that information/data different or less from what a startup has?</p><p>8. Which consequences do that data result in? For example: Investment follow-up/stop Engagement 9. Do you participate in the Board/Management Team to closely monitor the performance? 10. Do you coach the startups/teams?</p><p>Closing 11. Do you have any remarks/tips? Appendix D - First SDEIF (version 0.1) Appendix E – Second SDEIF (version 0.2) Appendix F – Third SDEIF (version 0.3)</p><p>Appendix G – Fourth SDEIF (version 0.4) </p>
Details
-
File Typepdf
-
Upload Time-
-
Content LanguagesEnglish
-
Upload UserAnonymous/Not logged-in
-
File Pages70 Page
-
File Size-