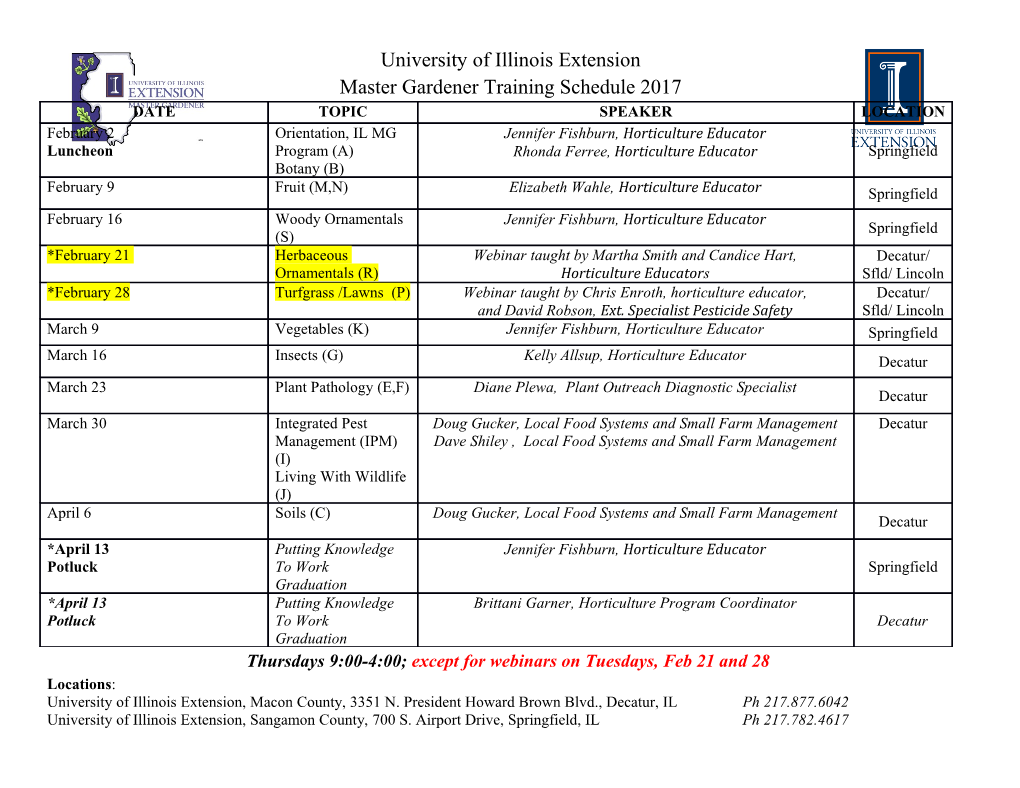
<p> On the Notion of Soft-Goals in Business Process Modeling</p><p>Pnina Soffer and Yair Wand</p><p>Abstract</p><p>Purpose: The paper aims at providing a conceptual framework based on clearly defined concepts and notions, which (a) integrates goals into process modeling and (b) specifically distinguishes goals from soft-goals or business measures. The application of this framework facilitates a systematic use of soft-goals in process design. Design: The framework is developed on the basis of Bunge’s well-established ontology. It is applied to processes taken from the SCOR supply chain reference model for demonstration and evaluation. Findings: Applying the framework to the SCOR processes resulted in a set of focused relations between soft-goals and processes, as opposed to the ones suggested originally in the SCOR model. This demonstrates the usefulness of the framework in process design. Limitations: The approach presented in the paper is still rather a theoretical framework than a fully validated procedure. It should be tested on larger-scale cases in more practical settings and evaluated accordingly. Practical implications: Applying the clearly defined concepts of the framework and the suggested analysis procedure is expected to (a) lead to focused and applicable measures tied to business process during process design, (b) provide a basis for process measurement requirements to be supported by an information system. Value: The contribution of the paper is both theoretical and practical. It provides clear-cut ontology-based definitions to concepts which so far have been assigned fuzzy and ambiguous meaning and uses these definitions for systematically tying business measures to business processes.</p><p>Paper Category: research paper</p><p>Keywords: process modeling, goals, soft-goals, process design, measures</p><p>1. Introduction The concept of goal is central in business process modeling and design. It is included in many definitions of business processes (e.g., “a business process is a set of partially ordered activities aimed at reaching a goal” (Hammer and Champy, 1994)). However, the introduction of formal constructs to incorporate the notion of goal into process modeling methods has received relatively little attention. There are two main outcomes to this situation. First, goals are often viewed as external concepts, not integrated with process models. Second, the term “goal” is being used for expressing concepts of a different meaning. Specifically, there is often no distinction between operational goals, defining a desired state to be reached by a process (e.g. “complete an order”), and strategic goals, which are more abstract objectives the organization is striving to achieve (e.g. “being responsive to market demand”). The former type can be accomplished via a given process, while the latter type of goal cannot usually be achieved by a specific process, yet it is important for the organization. Nevertheless, given that the term “goal” is used for both purposes, its use might lead to confusion among process designers and participants. Attempts to incorporate goals into process modeling in a variety of formality levels have been made in the past, addressing both operational and strategic goals. Some of these attempts rely on the Requirements Engineering (RE) literature, in which operational goals can be related to hard goals (or simply goals), forming the basis for functional software requirements, and strategic goals relate to soft-goals that set the basis for non-functional requirements. The RE literature proposes techniques for elaborating goals of the two kinds and reasoning with them. However, the fundamental concepts of goals and soft-goals are not formally defined, and may bear an ambiguous meaning. With respect to process modeling, it appears that the basic step of identifying and relating a specific soft-goal to a specific process is missing. Thus, it is not necessarily clear how to evaluate alternative process designs based on soft-goals. More generally, while assigning soft- goals to specific processes would seem straightforward and intuitive, experience shows this is not the case. We shall show later in this paper that even well-known and accepted models, such as the Supply Chain Operations Reference-model (SCOR: Stephens, 2001) (or at least a certain version of SCOR) has fallen into this trap, resulting in the definition of metrics that are not always relevant to the processes they are attached to. We believe that the key to linking process design to soft-goals is the formal definition of the notion of soft-goal and its relation to process properties.</p><p>This paper proposes a set of formally defined concepts to enable the incorporation of goals and soft-goals into process modeling and design methods. The theory underlying our approach is the Bunge-Wand-Weber (BWW) ontology (Wand and Weber, 1990), which provides a general set of concepts for representing the world. We start with a basic set of concepts that drive the mechanism of a process, including state, domain, law, stability, goal, and process. These concepts form a basis for defining the notion of soft-goals and their relationships to processes. The formal definitions lead to an analysis approach which we believe is applicable in practice. We demonstrate the approach by applying it to parts of the SCOR reference model. Once the gap of identifying relevant soft-goals and relating them to process alternatives is bridged, formal as well as informal methods that utilize soft-goals for process design can be developed. In particular, such methods can draw from the RE experience and literature.</p><p>2. Related Work Attempts to incorporate goals into process modeling include work by Kueng and Kawalek (1997), who suggest an informal approach in which goals provide a basis for process definition. They distinguish “functional goals” from “non-functional” goals. Their functional goals relate to operational as well as strategic goals, while their non-functional goals relate to properties of the model itself, such as modularity. A formally defined set of concepts, incorporating goals and processes, is provided by Khomyakov and Bider (2000), whose model is based on mathematical systems theory. Their approach to process modeling is state-oriented, viewing a process as a subset of trajectories in some state space, and a process goal as a set of conditions defining a surface in the state space. This set of concepts is extended in (Bider et. al., 2002) and used for defining a process pattern, allowing the design of generic processes that can be specialized for specific situations. The goals addressed by this approach are operational goals only, termed “functional goals”. Kavakly and Loucopoulos (1998) address business process modeling using the Enterprise Knowledge Development (EKD) framework, which entails a goal model among other views. They suggest a procedure accompanied by a set of diagrams, which sets the understanding of goals (both operational and strategic) as a basis for business process identification. This approach has its roots in the requirements engineering (RE) literature, where goal-based IS requirements have attracted a great deal of attention. RE views goals as the intentions that capture the rationale for the system to be built, and distinguishes between two categories of goals: hard goals (or simply goals) and soft-goals. While the satisfaction of goals can be clearly verified, soft-goals are defined as goals that do not have a clear-cut criterion for their satisfaction (Mylopoulos et.al., 1999; Mylopoulos et.al., 2001; Rolland, 2003). In RE hard</p><p>2 goals are generally related to functional system requirements, while soft-goals stand for non- functional requirements (e.g. flexibility) (Mylopoulos et.al., 1999; Mylopoulos et.al., 2001). RE offers techniques and methodologies that utilize goals and soft-goals in a design process. Goals and soft-goals are applied for requirements elicitation (Rolland, 2003) in combination with scenarios (Rolland, 2002) or scenarios and obstacles (Anton and Potts, 1998). Qualitative reasoning allows exploring and evaluating system alternatives on the basis of goals and soft- goals (Yu and Mylopoulos, 1996; Lamsweerde, 2001; Rolland, 2003). Formal reasoning based on formal goal-oriented notation (e.g., KAOS) enables the verification of goal refinement as well as conflict detection and analysis (Lamsweerde and Letier, 2000; Lamsweerde, 2001). Goals also serve for verifying the completeness of specified requirements (Lamsweerde, 2001; Rolland, 2003).</p><p>Given the role of goals in RE, it would seem beneficial to incorporate similar approaches or their underlying principles into business process design. Indeed, such efforts have been made. The i* RE framework has been adapted to business process redesign (Yu and Mylopoulos, 1996), employing a strategic dependency model and a strategic rational model. The strategic dependency model represents an organization as a network of strategic dependencies (goal, soft-goal, task, and resource dependency) among actors. The strategic rational model uses goals, activities, and rules to express alternative processes. In the i* framework soft-goals are used for qualitative reasoning about process alternatives, aimed at evaluating and selecting alternatives. While goals can be satisfied by a process, soft-goals by their nature are not marked by specific objectives that have to be accomplished. Hence, they are said to be satisficed1 by an alternative, meaning that an alternative has a positive contribution in terms of the soft-goal. However, despite the usefulness of the i* approach, the basic notions of goals and soft-goals are not defined formally there, and thus they remain fuzzy. In a more recent work (Giorgini et.al., 2002), an algorithm for quantitative reasoning with goal models in RE is proposed. However, it is based on weights that represent the satisfaction of a goal by a design alternative while leaving the origin of these weights obscure. </p><p>3. A Formal Process Framework 3.1 Fundamental concepts This section defines the basic concepts that drive the mechanism of a process. The theory underlying these definitions is the BWW ontology, which serves as a theoretical foundation for several works in various areas of systems analysis and design, including software concepts (e.g., Wand and Weber, 1990; Paulson and Wand, 1992) and business process concepts (Soffer et. al., 2001). According to BWW ontology, the world is made of things that possess properties. Properties can be intrinsic (e.g. height) to things or mutual to several things (e.g. a person works for a company). Things can compose to form a composite thing that has emergent properties, namely, properties not possessed by the individuals composing it. Properties (intrinsic or mutual) are represented in terms of attributes, which can be represented as functions on time. The state of a thing is the set of values of all its attribute functions (also termed state variables). When properties of things change, these changes are manifested as state changes or events. State changes can happen either due to internal transformations in things (self action of a thing) or due to interactions among things. Not all states are possible, and not all state changes can occur. The rules governing possible states and state changes are termed state laws and transition laws, respectively. </p><p>We model a process as a sequence of changes of the states of things in a domain. These changes can be tracked by relevant state variables. We shall now define the concepts that provide a formal basis for this view of a process. Domain: Part of the world of which we want to model changes.</p><p>1 The term satisficing was originally used by Simon (1981) to refer to the search for a satisfactory solution rather than an optimal one</p><p>3 By defining a process over a domain we set the scope of the process and provide a clear distinction between what would be considered external events, which are outside of the process’ control, and internal events that should be governed by the processes in the domain. State: A set of time-dependent attributes (state variables) that provide sufficient information about the domain for the purpose of modeling. Note, while the state of the domain can be described in terms of the things included in it, some of them perhaps being composites of others, for our purpose we sometimes view state variables needed to describe the domain as being part of the state of the domain. As well, we view states as being discrete, meaning that any change is from one state to another at a certain moment in time. Sub-domain: Part of the domain that can be represented by a (fixed in time) subset of the set of state variables. A state can be projected on a sub-domain by considering the sub-set of state variables describing the sub-domain. This subset defines the state of the sub-domain. Hence on “state” means a state of a domain or any sub-domain. States can be classified as being stable or unstable. The motivation to this distinction is that later on we will view the execution of a process as a sequence of unstable states that terminates when a stable state is reached. Stable state: A state that can only change as a result of an action of something outside the (sub) domain. Unstable state: A state of the (sub)domain that must change. The answer to the question of whether there exist stable states depends on the laws that exist in the domain and govern the states and their transitions. We formalize a law as: A law: A function from the set of states S into itself.</p><p>A transition law: A function from the set of possible unstable states Su into the set of states S. 2 The definition implies that a transition law is a function ON the set Su into the set of S . Implied in this definition is that the transition law is fully deterministic. Nevertheless, our model is not fully deterministic. Rather, uncertainty is captured through external events, i.e., events that are outside the process’ control.</p><p>A sequence of unstable states, transforming by law until a stable state is reached is the basic mechanism of a process: A process: A sequence of unstable states leading to a stable state. This definition does not mention the origin of the initial unstable state. This initial state, can be, in particular, the outcome of an interaction between the domain and a thing outside the domain when the domain is in a stable state. Furthermore, the definition does not mention the process goal explicitly. The following section defines and discusses the notion of goal and relates it to the definition of a process. </p><p>3.2 Goals and Soft-Goals On the basis of the fundamental concepts of our model, the following definitions incorporate goals as an integral part of a process model.</p><p>Definition: Assume a set of state variables defining a domain s=<x1,…,xn>. Let S={s | s lawful} be the set of states that are lawful by the domain state law.</p><p>Let SstS be the subset of domain stable states. Then a Goal (G) is a set of stable states SG Sst. This means that a goal is a set of states that the domain can stay in indefinitely, unless, something in the environment causes it to change. We now relate the notion of a goal to a process: Definition: A goal G will be said to be a process goal if every execution of the process terminates in G. This definition is technical in the sense that it does not provide any meaningful understanding of how a process is designed to always end in a specific subset of states. We need to</p><p>2 The definition of a transition law can be extended to a law on all states as follows: for an unstable state it is the transition law, otherwise it maps the state into itself.</p><p>4 operationalize the concept of goal so it can be related to the actual design of a process leading to it and provide practical implications. Definition: A criterion function is a function on the set of states C: S D, where D is a certain domain (of values). A criterion function is any function on the values of the state variables. It maps the state variables into a domain where a decision can be made on whether what was to be achieved by a process is indeed achieved. Examples of criterion functions are an average of certain state variable values or their distance from a target value. In many cases the mapping is on a subset of state variables that are relevant for deciding whether the process has reached its “goal”. The domain mapped into is then a sub-domain of the process domain. For example, in a manufacturing process a criterion function can map the entire set of state variables, including production time, cost, resource utilization, etc., into a sub-domain whose state variables are two Boolean variables: “Production is completed” and “Product quality is approved”. These two state variables are sufficient for determining the process termination. In general, for any practical use we may assume a domain of values, D, where the comparison operators > and = are applicable. A condition over criterion functions, Ck, can specify the subset of states which is the Goal set. Definition: A condition is a logical expression G made of simple expressions of the form:</p><p>R::= Ck rel gj, where Ck a criterion; rel{‘>’, ‘=’}; and gj Di Combined by ‘AND’ ‘NOT’ and precedences indicated by ‘( )’ We can now operationalize the definition of a goal:</p><p>SG = {s | G(C(s)) is ‘true’} Considering the manufacturing process discussed above, its goal set is {s| (Production is completed=‘true’) AND (Product quality is approved=‘true’) }.</p><p>A goal is a set of states that satisfy a condition over a criterion function. Such a set can, potentially, be attained in many different ways or paths. A process path, formally defined in the Appendix, is a set of unstable states, whose transitions are governed by the law defined over the domain, leading to a state sSG. All process paths lead to stable states that are included in the goal set. Not all state variables used in the definition of a sub-domain for which a process is defined will necessarily be affected in the process. However, state variables used in the definition of the goal should be affected. We formalize this in the following definition and lemma.</p><p>Definition: Let C be a criterion function used for the goal. Let I={1…n}.</p><p>Assume C(s)=C({xk| kIG}) where IGI (for every sSG ). We will term the set {xk| kIG} goal-defining state variables, and the set {xk| kI-IG} the indifference set of state variables (with respect to goal G). In words, the goal-defining set includes only state variables that affect the goal. In the production example described earlier the goal-defining state variables are “Production is completed” and “Product quality is approved”. All the other state variables, such as cost and time, belong to the indifference set.</p><p>The following lemma, formally stated and proved in the Appendix, connects the notion of a goal with the idea of process path. It implies that every state variable appearing in the goal set must be changeable in at least one process path. Lemma 1: For every goal-defining state variable there should be at least one path where it changes.</p><p>Hence on without loss of generality we will assume all goal-defining state variables are changed from their initial value at least once in at least one path in the process. The state variables in the indifference set may or may not be changed by the process. While considered indifferent with respect to the goal criterion function, these state variables might still have different values for different states in the goal set. It is likely that not all states in the goal</p><p>5 are equally desirable. For example, different paths of a production process, all leading to the same goal, may differ in time or in cost. The most desired state in the goal set is the one whose production cost and time are the minimal. The desirability of different goal states will be addressed below using the concept of a soft-goal. Definition: A Soft-goal is an order relation on states. By defining a soft-goal as an order relation, we can rank states according to their desirability. We call this ranking “soft-goal” since while the notion of “goal” implies something that can be attained, achieving a “better” state is a matter of improvement, not of accomplishment. Such an improvement is referred to in the RE literature as “satisficing” the soft-goal (Mylopoulos et.al., 1999). The definition of soft-goal can be operationalized in a similar way to the goal definition, as an order relation on a criterion function. The soft-goal criterion function would usually be different than the goal criterion function. For example, such criterion function may be the cost incurred by attaining the process’ goal. Clearly, the relevant state variables characterize the specific path taken to the goal state. Furthermore, while a process clearly possesses a single goal, it may be related to several (even contradicting) soft-goals. As discussed above, all state variables involved in the goal of a process must be affected by the process (from Lemma 1). Otherwise, no activation of the process will affect these variables and through them the goal. This is not necessarily the case for soft-goals. The state variables that determine the desirability of the goal states might not necessarily be affected by a given process (or even by a single process). As an example, consider the production process discussed earlier, and assume a soft-goal of “improved product quality”. The ranking of a specific goal state attained depends on state variables determined by the production process itself as well as the quality of the raw materials used, determined by other processes such as sourcing and inspection. In short, while it is obvious that a goal can only address state variables affected in the process, soft-goals can be related to parts of the domain not directly involved in a given process. Thus, soft-goals relate to the domain, not to a given process. This observation implies that the analysis of soft-goals should be addressed in a completely different manner than the analysis of process goals. Soft-goals may serve for two purposes: process design and process measurement. When designing processes the expected order relation on goal states of alternative process paths can serve for evaluating and selecting the best process path alternative. In the course of process execution actual values of state variables and criterion functions can be measured and serve for evaluating the specific occurrence of the process with respect to other occurrences or to target values.</p><p>In the rest of the paper we focus on using soft-goals for process design purposes. We begin by defining process design as follows: Given: a goal set and a soft-goal: (1) Find a (non-empty) set of paths so as to attain a state in the goal. (2) Find the best path possible with respect to the soft-goal. Two points should be noted here. First, if no soft-goal is given then all goal states are considered equivalent. Second, it is possible a process would be designed to improve more than one soft-goal. However, these soft-goals might define different orders on the goal states. In such cases, some kind of tradeoff will be needed. while our following discussion relates to one soft-goal, it can easily be applied to a combination of several soft-goals whose trade-offs are taken into account. The design objective for a process will usually entail the identification of paths that provide a “better” soft goal, namely, end in states that are higher on the order relation. Consider now the observation that a soft goal is domain-related rather than process-related, and that it might not be affected by the given process only. Thus, we need to establish a link between a given soft goal and the processes that can be designed to improve it. Definition: We will say that a soft-goal is meaningful with respect to a process iff actions taken during the process can affect the value of the related criterion function.</p><p>6 Two conditions can be derived from this definition: (1) A necessary condition for a soft-goal to be meaningful with respect to a process is that the goal set has more than one state. (2) A necessary condition for a soft-goal to be meaningful with respect to a process is that the soft-goal criterion function is dependent on the process in some sense. At this point we have not yet defined the conditions under which a criterion function can be considered process dependent. To do this, we discuss in the following section the connection between criterion functions and state variables.</p><p>3.3 Relating criterion functions to processes</p><p>This section explores the ways in which state variable can attain their values and change in processes. We then use this understanding as the basis for defining relationships between criterion functions and processes. Based on this, the relationships between soft-goals and processes are established and can serve in process design. A state variable can attain its values in a process in one of the following ways: (1) As a result of an external event to the process, namely, when a state variable in the related sub-domain changes its value as a result of an action outside the sub-domain. In this respect, the initial state of the process (which must be an unstable state) reflects a specific (“triggering”) external event (2) By a change of state which is an internal event (on one of the process paths). (3) Via dependency on state variables that change in the process. </p><p>We now formalize these notions.</p><p>Definition: Let xk be a state variable. We will say that xk is determined by a process p iff its value is affected by an internal event in (at least one path of) the process. Note that even if the value of a state variable is affected by an event, this does not necessarily imply it is known after the event. For example, the salary of an employee may be affected by the number of hours worked, but might also depend on a raise to be decided later, prior to payment. It is possible a state variable will be only partially determined by a process because it is affected by events external to the process. For example, the departure time of an airplane is partially determined by the flight scheduling process, and partially by external events (e.g. airport activities and traffic). Clearly, state variables that are part of the goal definition must be in the set of determined state variables: Lemma 2: For a given process p, every state variable in the goal must be determined by the process.</p><p>If the value of a state variable is determined by the actions of one process only, we will say it is fully determined by the process. It is possible that the value of a state variable will be determined as a result of actions in two processes. For example, the students admitted into an academic program might be determined by a criterion setting process and a candidate evaluation process. In such a case, we will say that each of the processes determines the state variable partially. More formally:</p><p>Definition: Let xk be a state variable. We will say that xk is fully determined by the process iff its value depends only on state variables that are determined by the process. We will say xk is partially determined by the process iff it is determined by the process, but not fully. It is possible that the value of a state variable that is part of the state definition of a process will be determined not by the process itself. In that case, its value will be decided by an external event (to the sub-domain of the process). If this external event is still internal to the whole domain, this would mean that another process has determined its value. For example, the color of a shirt, which obtains its existence in the process of sewing the shirt, is fully determined in</p><p>7 the process of selecting the fabric. This leads us to the notion of indirectly determined state variables. We formalize such situations using the concept of realization.</p><p>Definition: Let xk be a state variable. We will say that xk is realized in the process iff its value is attained in an internal event of the process but is only determined by the initial state or by external events. Practically, this means that the state variable is “observed” in one process, but the process does not determine it. To demonstrate, consider the shirt example of above. As long as the color of the fabric is known when the sewing begins, or is determined during the process, it will determine the color of the final product, independent of how the sewing is done. We formalize this notion as:</p><p>Definition: Let p and q be processes and xk a state variable. We will say that xk is indirectly determined by p with respect to q iff xk is determined by p and realized in q. Note, it is possible that xk is fully or partially determined by p and realized in q (but not determined by it).</p><p>Soft-goals are usually defined by criterion functions. Hence, we are interested in the dependency of criterion functions on processes. The move from discussing state variables to criterion functions is straightforward, since the functions depend on state variables. A criterion function related to a process goal is obviously process dependent (directly from Lemma 1 and Lemma 2). In contrast, since soft-goals are domain-related rather than process-related, the dependency of a soft-goal criterion function on specific processes is an issue to be analyzed. Definition: a function will be said to be process-dependent with respect to a process p iff its arguments include state variables that are determined by the process. Clearly, the dependency of a criterion function on a process relates the relevant soft-goal to that process. As is the case with state variables, dependency of criterion functions on processes can be full or partial: Definition: a function will be said to be fully process-dependent iff its arguments include only state variables that are fully determined by the process. A function will be said to be partially process-dependent iff it is process dependent but not fully. A formal form of the two above definitions is in the Appendix. As an example, the cost of executing a process is fully dependent on the process, provided the cost parameters are not subject to externally-induced changes during the process. If the process is designed to be efficient then its cost would be low. As an example of partial dependency, consider the length of a queue, which partially depends on the service process, but also on the arrival rate, which is an external event. Partial dependency can happen if the arguments of the function include state variables that are partially determined by the process or some additional state variable not determined by the process.</p><p>Partial as well as full dependency of a function on a process can either be direct or indirect. Indirect dependency is via variables that are determined by the process but are realized by another process. To see how this can happen consider the following example: a process of recruiting students to an academic program ends up with students joining the program. The outcome of the tuition collection process depends indirectly on the outcomes of the recruiting process. When a function is realized in another process, the second process can be viewed as a measurement point of the function. As an example, consider a group of weight watchers who meet once a week and weigh themselves. The function of weight lost during the week depends on how strictly a person kept his diet during the week. It is realized and measured by the weighing process.</p><p>Dependency of soft-goals on more than one process may cause difficulty in applying them for design purposes, and in tracing the effect of each individual process on the actual measured value when these processes are executed. It is therefore preferable to have as simple soft-goals</p><p>8 as possible. Sometimes, a soft-goal might depend on several processes in a way that their effects can be separated. This leads us to the notion of decomposing soft-goals. Decomposing a composite domain-related soft-goal may result in simpler soft-goals, which depend on single processes. Definition: A soft-goal is process-decomposable iff its criterion function can be represented as a combination of functions, where each function is on subset of state variables that depend on one process or a group of processes. As an example, a soft-goal of minimizing procurement costs depends on the following processes: supplier management, purchase inquiry, contract and order management, and receiving. It is actually a sum of the costs associated with each of these processes and can be decomposed into a set of soft-goals, each one dependent on a single process.</p><p>The order relations of the simple functions are not necessarily the same as the composite one. For example, a soft-goal of profitability, whose order relation is increasing, may be decomposed to a soft-goal of costs (decreasing) and revenue (increasing), determined by two different processes (production and sales).</p><p>If a soft-goal is partially dependent on a process and partially on external events, it may still be possible to decompose it to a fully process-dependent part and a part that depends on the external event. The externally-dependent part should carefully be examined to see if it is influenced by other processes in the domain. For example, purchasing cycle time is partially dependent on purchasing processes and partially on the supplier’s performance (external). However, the supplier’s performance is affected by supplier selection and by supplier relationship management.</p><p>4. Application to SCOR Model</p><p>In this section we demonstrate our approach by applying it to the SCOR model and performing a soft-goal analysis. The SCOR model is a reference model of supply chain management processes, developed and endorsed by the Supply Chain Council as a cross-industry standard. While primarily targeted for industrial use, the SCOR model has been used in quite a number of research works (e.g., Arns et. al, 2002; Humphreys et. al., 2001) as a comprehensive body of common and accepted supply chain business processes. SCOR contains three levels of process details. The top level includes five basic processes, namely Plan, Source, Make, Deliver, and Return. The second level defines categories for each of the five basic processes, according to different logistic categories (e.g. make to stock). The third level decomposes each process category into elements, which are to be decomposed into activities by companies that implement the model. The SCOR model also provides metrics and “best practices” associated with each process category and element. The metrics relate to reliability, responsiveness, flexibility, cost, and assets. The “best practices” are short descriptions of specific innovative logistic practices (e.g., Vendor Managed Inventory).</p><p>Metrics in the SCOR model are intended to measure the performance of processes. Hence they can be viewed as criterion functions used for soft-goals. Below we analyze some of the metrics defined in relation to sourcing processes in the SCOR model version 4.0. Although this is not the latest version of SCOR, it best suits our demonstration needs, as shall be explained when discussing the analysis results. The SCOR processes we address are: ES.7 - Manage supplier network ES.9 - Manage supplier agreements P2.4 - Establish sourcing plans S1.1 - Schedule product deliveries S1.2 - Receive product S1.3 - Verify product </p><p>9 S1.4 - Transfer product S1.5 - Authorize supplier payment A partial list of metrics associated to these processes is given in Table I, where X means a metric is related to a process. *** Place Table I here *** We now present the results of analyzing the soft-goals of the SCOR model, using our concepts of full and partial dependency and measurement. We demonstrate how this can be done using the following example. Consider the criterion “Supplier delivery to date performance”, related in SCOR to process S1.2 (Receive product), as shown in Table I. Indeed, it can be measured by looking at statistics that can only be obtained in process S1.2. However, the supplier performance, which is external to the domain, can only be affected by the selection of reliable suppliers, which is done in processes ES.7 (Manage supplier network) and P2.4 (Establish sourcing plans). Therefore, it is partially dependent on processes ES.7 and P2.4, and measured in process S1.2. The outcomes of the analysis with respect to all the defined metrics are shown in Table II, applying the following notation: FD – criterion function is fully dependent on the process PD – criterion function is partially dependent on the process M – value of criterion function is measured at the goal state of the process *** Place Table II here*** The analysis shows two essential types of errors in the association of soft-goals to processes: 1. A soft-goal that is partially dependent on several processes is not associated to all the relevant processes (e.g., Total source cycle time). 2. A soft-goal is associated with the process where it is measured rather than to the process(es) it is dependent on (e.g. % defective supplied). The first type of error implies that processes that affect a soft-goal are not considered with respect to it, whereas the second error type implies that processes are considered with respect to soft-goals they have no effect on. Our analysis of the metrics of version 4.0 of the SCOR model predicts that these are not effective metrics and they should be revised. Indeed, in more recent versions the metrics have been revised, although still not as systematically as we suggest below. Another straightforward observation is that the SCOR model does not distinguish between partial and full dependency of a soft-goal on a process. This distinction is important because partial dependency might imply a process cannot be designed in isolation from at least one other process. Alternatively, partial dependency can indicate cases where a soft-goal should be decomposed in order to be more focused and applicable in process design.</p><p>In order to demonstrate the effect of decomposition, consider the soft-goal of “total source cycle time”. As shown in Table II, it is partially dependent on all the processes that are considered in our analysis. Indeed, this is a high-level soft-goal that encompasses the entire sourcing cycle. Let us consider events within the sourcing process, which are the events (both external and internal) occurring while reaching goal states of sub-processes. e0 : sourcing signal is given (external event) e1 : an order is issued to the supplier (goal state of S1.1) e2 : arrival of goods from the supplier (external event) e3 : the reception of goods is completed and verified (goal state of S1.2) e4 : quality inspection is completed and the goods are verified (goal state of S1.3) e5 : the goods are transferred to their destination (goal state of S1.4) e6 : payment to supplier is completed (goal state of S1.5) The general soft-goal of total source cycle time can be expressed as:</p><p>Criterion function: TC= C(e0 ,e6) = t(e6) – t(e0) , where t(e) is the time an event occurs. A useful order relation on the criterion function is the decreasing total length of time. The soft goal can therefore be defined as “Minimize TC”. TC can be decomposed as follows: C = C1(e1) + C2(e1 ,e2) + C3(e2 ,e3) + C4(e3 ,e4) + C5(e4 ,e5) + C6(e5 ,e6) </p><p>10 Each of these components has of course its own decreasing order relation, defining a soft-goal with respect to each of them. The relationship between the components of the decomposed soft-goal to processes is presented in Table III. *** Place Table III here*** As seen in Table III, rather than having a single soft-goal that is partially dependent on a large number of processes, the result of our analysis is a set of focused partial soft-goals. Four of these soft-goals are fully dependent on a single process and measured there, which makes them easy to apply in process design. Partial dependency cases still exist, specifically in the order lead time soft-goal, where the dependency is mostly on external events, such as delivery of goods from the supplier. In this case there is a partial dependency on processes that select the supplier and set the agreements with him. Measurement is performed in the process of receiving the goods, where the information becomes available.</p><p>5. Concluding Discussion</p><p>We have presented a formal framework that defines a process model on the basis of states and state variables. We concentrated on defining and understanding soft-goals and their relationships to processes and state variables. Paradoxically, the benefit of our formal framework lies in the fact that identifying soft-goals seems to be an intuitive and straightforward task. Being so, systematic approaches might seem unnecessary. We claim such a view might lead to confusion and misidentification of relevant soft-goals. An important process design objective is to improve soft-goals by selecting a preferred design. Thus, it is important that soft-goals be defined in a correct way – linked to the processes in which they can be improved. We have presented a formal approach to analyzing the dependency of soft-goals on processes. Processes are designed to accomplish specific goals (or a defined combination of soft-goals). In contrast, a given soft-goal does not necessarily depend on specific processes. However, it is possible to analyze the dependency of a criterion function (that defines a soft goal) on processes. Specifically, we propose to (a) identify the domain soft-goals, (b) decompose them in order to minimize their partial dependency on many processes, and (c) establish dependency on processes and measurement points. Once dependency is established, soft-goals can serve for evaluating alternative process designs and execution paths. </p><p>A useful observation is that some criterion functions (metrics) can only be measured in processes that do not determine their values. This will usually happen when some decisions are made in some processes and the outcomes are “fed” into a process that only executes a certain course of action. The identification of measurement points is useful for defining information needs for process evaluation. Specifically, it can help determine which information should be collected thus supporting information systems design.</p><p>When processes are being designed with respect to soft goals, the exact ordering of alternatives according to soft goals might be only an estimate. The actual values of state variables that would be obtained in real process execution cannot be predicted precisely due to several reasons. First, while goal defining state variables often assume a limited set of values, soft-goal defining state variables might form an infinite state space. Second, even though the law in our model is deterministic, the exact state to be assumed in a single process occurrence may depend on external events, which introduce uncertainty to our model. Third, some state variables are merely parameters at the time of process design. The actual value of these parameters is set in each occurrence of the process. Some of these parameters (e.g. cost) may be fixed and not change in different process occurrences, while others (e.g. ordered quantity) are valid for a single occurrence only. All these reasons make the establishment of the exact order relation of alternative process paths at the design stage a difficult task. </p><p>11 Related work in the process design area has hardly dealt with soft-goals. The only work we are aware of is of Yu and Mylopoulos (1996), who introduced soft-goals based RE techniques to process design. That work focuses on the application of soft-goals for reasoning on process alternatives. We view our work as complementary since it deals with linking soft-goals to specific processes. Identifying these links is the first step to be taken before reasoning about the design of processes with respect to soft-goals.</p><p>Although the notion of soft-goals is “borrowed” from the RE literature, the difference between soft-goals in process design and in RE needs to be emphasized. In RE, the domain is a not-yet existing system to be designed. Thus, the design of the system can take into account the soft- goals. In contrast, process design deals with an existing organization whose existing processes are being redesigned. The soft-goals are “imposed” on an existing situation. In other words, soft-goal in RE can be applied in a “top down” manner. In process redesign they are often incorporated from the bottom up (although of course reflecting organizational objectives). Despite this difference, we believe that soft-goals can be very useful in the context of process design.</p><p>This work is a first step in incorporating goals and soft-goals into process modeling in a formal and systematic approach. The formalization can contribute to clarifying the concepts and preventing confusion. We believe that by using clear-cut definitions, inconsistencies and incompleteness in process models can be identified, and insights gained as to the relationships of all the parts of the model. We are now working on formalizing notions of process model decomposition, completeness and consistency.</p><p>References Anton, A. I., and Potts, C. (1998), “The Use of Goals to Surface Requirements for Evolving Systems”, International Conference on Software Engineering (ICSE’98), Kyoto, Japan, IEEE Press, pp. 157-166. Arns, M., Fischer, M., Kemper, P., and Tepper, C. (2002), “Supply Chain Modelling and its Analytical Evaluation“, Journal of the Operational Research Society, Vol. 53, pp. 885-894 Bider, I., Johannesson, P., Perjons, E. (2002), “Goal-Oriented Patterns for Business Processes”, Position paper for Workshop on Goal-Oriented Business Process Modeling (GBPM’02). Giorgini, P., Mylopoulos, J., Nicciarelli, E., and Sebastiani, R. (2002), “Reasoning with Goal Models”, In: Spaccapietra, S., March, S. T., and Kambayashi, Y. (ed), ER 2002, LNCS 2503, Springer-Verlag Berlin, pp. 167-181. Hammer, M. and Champy, J. (1994), Reengineering the Corporation – A manifesto for Business Revolution, Nicholas Brealey Publishing, London. Humphreys, P. K., Lai, M. K., and Sculli, D. (2001), “An Inter-organizational Information System for Supply Chain Management”, International Journal of Production Economics, Vol. 70 No. 3, pp. 245-55 Kavakli, V., and Loucopoulos, P. (1998), “Goal-Driven Business Process analysis Application in Electricity Deregulation”, in Pernici, B. and Thanos, C. (ed.), Advanced Information Systems Engineering (CAiSE’98), LNCS 1413, Springer-Verlag Berlin, pp. 305-324 Khomyakov M., and Bider, I. (2000), “Achieving Workflow Flexibility through Taming the Chaos” OOIS 2000 - 6th international conference on object oriented information systems. Springer-Verlag Berlin, pp. 85-92 Kueng, P., and Kawalek, P. (1997), “Goal-based Business Process Models: Creation and Evaluation”, Business Process Management Journal, Vol. 3 No.1, pp. 17-38</p><p>12 Lamsweerde, A.v. (2001), “Goal-Oriented Requirements Engineering: A Guided Tour”, Proc. RE’01, International Joint Conference on Requirements Engineering, IEEE Press USA, pp. 249-263 Lamsweerde, A.v., Letier, E. (2000), “Handling Obstacles in Goal-Oriented Requirements Engineering”, IEEE Transactions on Software Enginnering, Vol. 26 No. 10, pp. 978-1005 Mylopoulos, J., Chung, L., and Yu, E. (1999), “From Object-Oriented to Goal-Oriented Requirements Analysis”, Communications of the ACM, Vol. 42 No. 1, pp. 31-37. Mylopoulos, J., Lawrence, C., Liao, S., Wang, H., and Yu, E. (2001), “Exploring Alternatives during Requirements Analysis”, IEEE Software pp. 92-96 Paulson, D. and Wand, Y., (1992) “An Automated Approach to Information Systems Decomposition”, IEEE Transactions on Software Engineering, Vol. 18 No. 3, pp. 174-189 Rolland, C. (2002), “L’e-lyee: L’escritoire and Lyeeall”, Information and Software Technology, Vol. 44, pp. 185-194 Rolland, C. (2003), “Reasoning with Goals to Engineer Requirements”, ICEIS’03 – Fifth International Conference on Enterprise Information Systems, Angers, France, Invited Speeches: pp. 11-19 SCOR Reference model, Supply chain council. Available www.supply-chain.org. Simon, A. H. (1981), The Sciences of the Artificial 2nd ed., MIT Press, USA Soffer, P., Golany, B., Dori, D., and Wand, Y. (2001) “Modeling Off-the-Shelf Information Systems Requirements: An Ontological Approach”, Requirements Engineering, Vol. 6, pp.183-199 Stephens S. (2001), “Supply Chain Operations Reference Model Version 5.0: a New Tool to Improve Supply Chain Efficiency and Achieve Best Practice”, Information Systems Frontiers, Vol. 3 No. 4, pp. 471-476 Wand, Y. and. Weber, R (1990), “An Ontological Model of an Information System”, IEEE Transactions on Software Engineering, Vol. 16, No. 11, pp. 1282-1292 Yu, E., and Mylopoulos, J. (1996), “Using Goals, Rules, and Methods to Support Reasoning in Business Process Reengineering”, International Journal of Intelligent Systems in Accounting, Finance and Management, Vol. 5, pp. 1-13.</p><p>13 Appendix: Formal Form of Definitions and Lemmas </p><p>1. Process model and state variables</p><p>Definition: A process model is a quadruple MP = <S,L,SI,SG> where: S is a set of states L is a law defined on S</p><p>SI a subset of unstable states in S: the set of possible initial states SG a subset of stable states in S: the goal set</p><p>Definition: a process path is a set of states <s1,…sn> such that: sisj s1SI snSG and sk+1=L(sk) for every k{1,n-1} (where L is the law defined over the domain). </p><p>Lemma 1: For every xk kIG there should be at least one path where it changes. Proof: Assume xk does not change. Let xk=z over every path of the process. Define C’({xk| kIG-k}=C({x1,… xk-1,z, xk+1, ,xn}). Every state fulfilling the condition over C will fulfill the same condition over C’. We can redefine the criterion function to be C’.</p><p>Notation for a determined state variable: kID(p).</p><p>Lemma 2: For a given process p, if kIG(p) then kID(p). Proof: directly from the assumption derived from Lemma 1.</p><p>2. Criterion functions and processes</p><p>Definition: Let C be a criterion function, and IC={i, xi an argument of C}, then C is process p dependent iff ID(p)IC. </p><p>Definition: Let C be a criterion function, and IC={i, xi an argument of C}, then C is fully process p dependent iff ID(p)IC and IC(p)-ID(p)=. C is partially dependent on process p iff ID(p)IC and IC(k)-ID(p).</p><p>Definition: A soft-goal is process-decomposable iff its criterion function can be represented as</p><p>C(x1, x2,…xn) = C(F1(x1,…xk),…Fm(xj,…xn)). where each subset of state variables depends on one process or a group of processes.</p><p>14 Table I: Metrics Associated to processes in SCOR model Process ES.7 ES.9 P2.4 S1.1 S1.2 S1.3 S1.4 S1.5 Metric Number of Supply Sources X X Supplier Delivery to date X X Performance Product Management and X Planning Costs Source lead time X Receiving costs X Delivery Quantity Performance X Supplier On time Delivery X X X Performance Process cycle time X % Defective supplied X Verification costs X Receiving & storage costs X Time from receiving to check X issue Cost per invoice X Invoices processed without X error. Total source cycle time X X Total Product Costs X Supplier Quality Performance X X Supplier Price Performance X X Frequency that purchase X orders/contract can be altered. Average length of contracts X Cost of managing contracts X</p><p>15 Table II: SCOR metrics analysis Process ES.7 ES.9 P2.4 S1.1 S1.2 S1.3 S1.4 S1.5 Metric Number of Supply Sources FD,M Supplier Delivery to date PD PD M Performance Product Management and PD PD PD,M Planning Costs Source lead time PD PD PD PD M Receiving costs PD,M Delivery Quantity Performance PD PD M Supplier On time Delivery PD PD M Performance Process cycle time PD PD PD PD PD M % Defective supplied PD PD PD M Verification costs FD,M Receiving & storage costs FD,M Time from receiving to check PD PD,M issue Cost per invoice FD,M Invoices processed without FD,M error. Total source cycle time PD PD PD PD PD PD PD PD,M Total Product Costs PD PD PD,M Supplier Quality Performance PD PD M Supplier Price Performance PD PD M Frequency that purchase FD M orders/contract can be altered. Average length of contracts FD,M Cost of managing contracts FD,M</p><p>16 Table III: Decomposed soft-goal related to processes Process ES.7 ES.9 P2.4 S1.1 S1.2 S1.3 S1.4 S1.5 Criterion function Time to order issuing FD,M Order lead time PD PD PD M Reception verification time FD,M Quality verification time FD,M Goods transfer time FD,M Time from receiving to PD PD,M payment</p><p>17</p>
Details
-
File Typepdf
-
Upload Time-
-
Content LanguagesEnglish
-
Upload UserAnonymous/Not logged-in
-
File Pages17 Page
-
File Size-