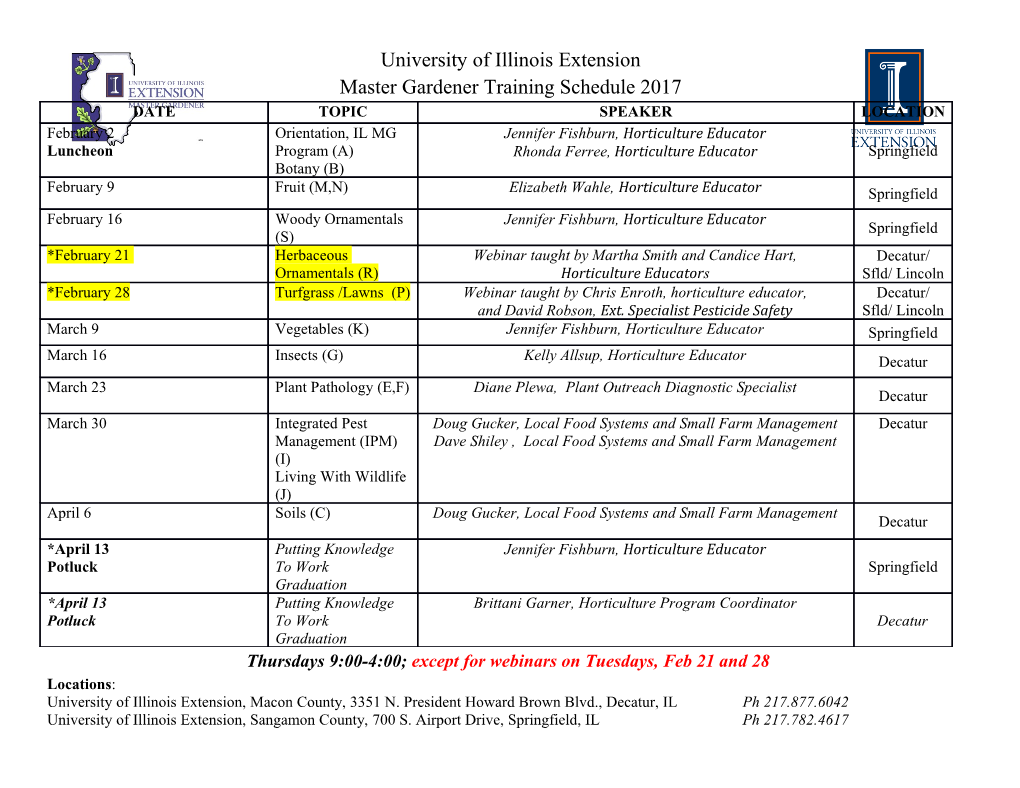
<p>C OHORT STUDIES </p><p>INTRODUCTION</p><p>Definition...... 95 Conceptual Model...... 95</p><p>TYPES OF COHORT STUDY Concurrent studies (Prospective cohort studies)...... 97 Nonconcurrent studies (Retrospective cohort studies)...... 98</p><p>METHODOLOGICAL ASPECTS Calculating the sample size...... 98 Sampling: recruitment and monitoring...... 98 Evaluation of exposure...... 100 Evaluation of outcome...... 101</p><p>ANALYSIS OF RESULTS Measurements of association between risk factors and disease...... 102 Person-time...... 103 Survival analysis...... 103 Stratification...... 104 Multivariable model...... 106</p><p>ADVANTAGES AND LIMITATIONS...... 107</p><p>CHECKLIST FOR DESIGN OF A COHORT STUDY...... 109</p><p>ADDITIONAL READING...... 110</p><p>EXERCISES...... 111</p><p>DATA FILE DICTIONARY...... 138 Cohort studies</p><p>INTRODUCTION</p><p>Definition</p><p>A cohort study (also called prospective, longitudinal, incidence or follow-up study) is a type of study in which the investigator observes and analyzes the relationships between the presence of risk factors and the development of diseases in a population group over time. The participants are followed-up over a pre-established period of time, depending on the outcome of interest, in order to identify changes in the frequency of occurrence of the disease associated with the presence of a given risk factor(s). The unit of observation is the individual, and the follow-up allows detection of the changes that occur among any or all the participants in the study.</p><p>The term cohort comes from the Latin cohors, which meant a tenth of a legion of Roman soldiers, who marched together in the military campaigns of the Empire. In epidemiology the term identifies a group of persons who have a given experience in common.</p><p>Conceptual model</p><p>The conceptual model of a cohort study is relatively simple: a sample representative of the population to be studied is selected and information on some risk factor or characteristic of interest is obtained. This sample is divided into two distinct groups:</p><p>(1) Those who are (or could be) exposed to the risk factor or characteristic of interest</p><p>(2) Those who are not (or could not be) exposed to the risk factor or characteristic of interest.</p><p>These groups are followed over time to determine which of their members will develop the event to be studied (infection, disease or other health problem) and whether prior exposure is related or not to the occurrence of that event. The basic objective of a cohort study, therefore, is to identify the effects of exposure on the incidence of outcome (Figure 1).</p><p>Figure 1 - Scheme of a cohort study Time Direction</p><p>Disease Exposed No Disease Persons Population Without Disease Disease Unexposed No Disease</p><p>Adapted from Beaglehole et al., 1993 95 Cohort studies</p><p>The simplest designs consist of at least two groups, one of exposed and the other of unexposed individuals, so that the occurrence of disease can be compared. As opposed to an intervention design, the exposure factor(s) is not controlled by the investigator; the investigator only observes and measures the potential risk factor (exposures) under investigation. In more complex designs several groups may be formed for different degrees and durations of exposure.</p><p>The record of the occurrence of a health problem (infection, disease, or deaths) are used to calculate incidence and death rates, which are the basic measurements of the risk of developing a disease or of dying. These rates can be calculated:</p><p>(1) Among those exposed and not exposed to the risk factor of greatest interest;</p><p>(2) Among those individuals exposed to different levels of the risk factor and for different periods of time; and </p><p>(3) Among those exposed to a combination of those factors.</p><p>It can also be determined whether any variations in the levels of exposure during the period of follow-up have changed the estimates. Thus, cohort studies provide a basic measurement of the risk associated with different levels and types of exposure, which makes them very important in epidemiology.</p><p>The inferences about cause-effect associations drawn from a cohort study are more consistent than those deriving from a case-control study. In the latter, conclusions are often limited by the conceptual model, errors in the selection of cases and controls, whether or not the study sample is representative of the source population, and errors in the collection of information. Hence it is desirable, whenever possible, for a disease-risk factor association found in a case-control study to be confirmed by a cohort study.</p><p>Cohort studies have been used to investigate the natural history of diseases and to study characteristics of their transmission and maintenance. For tropical diseases they are generally done in rural areas and are conducted over a long period of time in areas with high prevalence/incidence frequencies of the disease of interest (e.g. schistosomiasis, leishmaniasis, etc). Studies are initiated by an epidemiological survey to identify possible risk factors (socioeconomic, demographic, and biological) associated with the disease among the population. In a longitudinal prospective study asymptomatic and symptomatic individuals are followed-up, together with a cohort of uninfected participants. Patients are evaluated periodically, and may receive treatment when available; a long follow-up would allow to identify prognostic markers for evolution of the disease. In addition to providing a clinical evaluation of patients, these studies can estimate the prevalence of a disease in the population, estimate the incidence and identify risk factors for infection and disease evolution. </p><p>TYPES OF COHORT STUDY</p><p>96 Cohort studies</p><p>Cohort studies can be conducted in two different ways: (1) As concurrent or prospective cohort studies (2) As nonconcurrent or retrospective cohort studies In concurrent studies individuals are selected by having or not the exposure to the risk factor under study and are followed-up for a defined period of time. In non-concurrent (historical) cohort studies the investigator looks at the past to select comparison groups based on past exposure in a given time and follows them over time, generally to the present, by a variety of methods. As can be seen in Figure 2, these two types of study are distinct and involve different methodological aspects.</p><p>Concurrent or prospective cohort study Figure 2 - Difference between a hypothetical concurrent and nonconcurrent studies based on the time of selection of exposed and unexposed participants. Year of study start: 1996</p><p>Concurrent Defined Nonconcurrent population 1996 1976</p><p>Exposed Unexposed</p><p>2016 Disease No Disease Disease No Disease 1996</p><p>Adapted from Gordis, 1996 In concurrent or prospective cohort studies the epidemiologist identifies and selects the groups exposed and not exposed at the time when the study begins and monitors them for a given period of time in the future.</p><p>Example 1: Cohort studies can be used to estimate the risk associated with intensity of water contacts and schistosomiasis transmission and disease. </p><p>Example 2: The risk associated with use of injectable drugs, unprotected sexual and other potential risk factors to HIV/AIDS can be estimated by following-up and comparing groups of individuals exposed or not to those risks.</p><p>In some studies the exposure may have been taking place before the investigation begins, in which case the duration and intensity of that exposure may influence the outcome.</p><p>97 Cohort studies</p><p>When possible the duration of exposure should be estimated by interviews or a test. In many situation, however, it is impossible to determine the exact onset of exposure.</p><p>Example 1: Chronic malnutrition and pulmonary infection. Example 2: Leishmaniasis infection and risk of developing clinical disease. In both cases, the duration of the exposure may influence the risk of developing of the outcome, but this information is hard to collect. </p><p>The duration of follow-up depends on the outcome and objectives of the study. For infectious diseases of brief incubation period, results can be achieved in a short period of time, whereas in those of long incubation or latency period, monitoring usually goes on for a fairly long time. Studies of long duration are operationally more complex, of high cost, difficult to keep standard procedures, and are subject to a loss of participants over time. Example: A large concurrent cohort study was carried in Brazil to evaluate the influence of perinatal, demographic, environmental, food and welfare factors on child health. A cohort was established by all children born in a given city in 1982. Several years of follow-up were conducted to monitor the occurrence of a variety of health problems.</p><p>Nonconcurrent or retrospective cohort study</p><p>In nonconcurrent or retrospective cohort studies, groups of individuals who were exposed and unexposed at some point in the past are identified; those groups are then “followed or monitored,” usually through the recent past or present (or even into the future). This is a study that begins in the past, at the time when the exposure occurred, but retains the basic principle of the cohort study: observations from the exposure in the direction of the event.</p><p>Nonconcurrent studies can rarely be done on a general sample of the population; the investigator would need access to information on past exposure to the risk factor (from the ontset of the study). This information allows for the identification of samples of exposed and unexposed participants and for the follow-up of the selected individuals. Example: participants of a study on possible food poisoning can be selected from among those who have and have not participate/eaten in a given buffet. For long-term exposure in the past the availability of information can be very limited and will rely on medical or company records. For outcome which are easy to identify such as a severe disease or death the information can be more available.</p><p>Due to the time and resources needed to conduct a prospective cohort study, the choice of epidemiological design is often between a retrospective cohort study and a case-control study. These studies share the advantage that it is not necessary to wait a long time for cases of a disease to turn up. For rare diseases, the case-control study design is ideal. In the case of exposures of low prevalence, the retrospective cohort study may be the only design capable of providing a sufficient number of exposed participants.</p><p>METHODOLOGICAL ASPECTS</p><p>Calculating the sample size Based on EPIINFO the following parameters are required:</p><p>98 Cohort studies</p><p>(1) Ratio of persons exposed to those not exposed [usually one to one]. (2) Minimum relative risk (RR) to be detected: an value for the RR which, if detected, will warrant the conclusion that there is an association between the risk factor and the disease. (3) The frequency of the outcome in the unexposed group (p0): estimated on the basis of a review of the literature. (4) The level of significance (0.05)- a given association between a risk factor and an outcome would have occurred by change only once in 20 . (5) The power of the test (1-beta): 80% or 90%, meaning a 10% or 20% probability of concluding that an association between risk factor is not related to the outcome when it actually is. </p><p>Example: Calculation of the size of a sample for a one-year prospective cohort study designed to study the incidence of tuberculosis among HIV positive individuals who are PPD positive or PPD negative.</p><p>(1) Ratio of exposed to unexposed participants: 1:1 (2) Value of the risk to be detected: 2 (3) Frequency of the event in those not exposed: 8.0% (4) Value of alpha: 0.05 (5) Power of the test: 0.80</p><p>The sample needed to conduct this study will be 283 PPD(+) and 283 PPD(-) HIV + individuals.</p><p>Note that: </p><p>(1) The smaller the RR the study is designed to detect the larger the required sample size; (2) The sample size varies according to the specified parameters. </p><p>The feasibility of a study will depend on (a) the financial resources and time available, and (b) minimum risk considered of clinical or biological significance.</p><p>Sampling: Recruitment and monitoring</p><p>The eligibility criteria for inclusion in a study must defined in the protocol. A participant, when selected, should be free of the disease of interest or another disease that is a consequence of the exposure of interest. It is also important to establish the time and intensity of the exposure to which the participants have been (or are being) subjected.</p><p>If the participants are selected as a sample of the population and classified as whether they have or not the exposure, then the study is one of internal comparison. The use of internal comparison groups (1) increases the probability that the members of both cohorts belong to similar population groups, and (2) implies that they will be subjected to the same procedures during the monitoring period, and so have the same chance of the disease being detected.</p><p>99 Cohort studies</p><p>When no internal comparison is available and the unexposed group is selected as a sample of the general population the two group may differ with regard other risk factors and quality of monitoring.</p><p>The major operational difficulty in a cohort study is to maintain a high rate of compliance with the follow-up. Special procedures may be required to assure periodic contacts with the participants in the sample in order to minimize losses. Every effort should be made to ensure their participation without violating the conditions under which they were recruited.</p><p>In a cohort study some proportion of the participants will always be lost during follow-up for different reasons. Special data analysis methods are used to account for losses in the follow-up. In studies in which the outcome is death, it is almost always possible to obtain information on lost participants (whether alive or dead) from death certificates of family information. When the endpoint is developing a disease, the participants who remain with the study can be compared with those lost to follow-up in respect to some of the baseline characteristics. The possibility that a bias have not been introduced in a study is reinforced if those characteristics are similar in both groups, that is, no evidence of differential loss is detected.</p><p>The quality of the information is a critical aspects in nonconcurrent cohort studies, when the information on exposure and monitoring is from long time ago. Methods of diagnosis or of identification and measurement of exposure may also have changed over time, making comparisons difficult or impossible.</p><p>Evaluation of exposure</p><p>Failure to assign participants to the correct exposed and unexposed groups seriously compromises a study. The techniques used to measure exposure can vary considerably from study to study and depend on the different risk factors. Exposure is obtained from interviews and questionnaires, laboratory tests, clinical and biological evaluations, and medical histories; the members of the cohort can then be assigned to different categories of exposure.</p><p>In prospective studies for infectious diseases, other characteristics must be observed for the identification of exposure. The presence, duration and intensity of exposure to an infectious agent depends on the source of infection and the route of transmission of the agent. When the source of infection and the transmission period are well defined and of only one type, the division into exposed and unexposed groups can be a simple matter. </p><p>When there are many sources of exposure or different routes of transmission, however, classification of individuals as exposed and unexposed can become difficult. In studies to establish the nature of exposure and the routes of transmission for infectious diseases, the normal practice is to use the mean time between exposure and emergence of the disease among exposed persons. Studies of infections from food poisoning are good examples: if high rates of illness occur among individuals who have eaten a particular food in a specified period of time and place, the source of infection and route of transmission can be identified.</p><p>100 Cohort studies</p><p>Exposures to risk factors can change in the course of a study: (1) participants can change what they are doing; (2) women can change their means of contraception; and (3) sexually active individuals can modify their habits in terms of protection from HIV, etc. Participation in a study can itself induce members of a cohort to change their exposure in reaction to information received; participants in a nutrition study, having been questioned about their food habits, may change those habits. When this happens, the researcher has no control; the participants are free to choose, and in many cases the changes they make are desirable. It is important, however, to record all changes as they occur in the course of the study, the reasons why they have occurred, and the different periods of exposure, so that they may be considered in the analysis. In some cases these changes can be prompted by causes extraneous to the study, for example, a change in an environmental factor can appreciably alter the degree of exposure.</p><p>In nonconcurrent cohort studies, when the exposure has happened many years before the beginning of a study, the available information may be insufficient for classification by level and duration, and in many cases only one means of classification of exposed and unexposed can be used. Without this information it is impossible to evaluate whether there is or is not a dose-response relationship between exposure and a disease. The use of exposure information collected solely from already available sources is subject to several limitations; in the great majority of cases the data were collected for other purposes than those assigned to the study.</p><p>Evaluation of outcome</p><p>In concurrent studies, information for the identification of an infection, disease or death is collected at intervals during the course of the study in clinical examinations, laboratory tests, interviews, questionnaires, reviews of medical histories and death certificates. The length of the interval depends primarily on the disease under study. It is also important to obtain information on other characteristics of the study groups, such as age, sex, occupation and other factors of interest so that other variables may be identified that could be related to the disease or the relevant exposure.</p><p>In many studies the outcome is death from a specific disease. Their limitations are those inherent in the use of death certificates as sources of data: the quality of the information entered on them, errors in the classification of the primary cause of death, errors of coding, etc. In addition, these studies are confined to the information found on death certificates.</p><p>Determination of incidence is particularly dependent on the disease under study and the sources of information used. Apart from the methods employed, the procedures for the identification of diseases must be the same for the exposed and unexposed subjects. To avoid bias, the clinical or laboratory diagnosis of the disease must be made, whenever possible, by professionals that have no knowledge of the participant’s exposure group.</p><p>When medical histories are used to determine the occurrence of diseases, special care must be exercised as the information taken from different hospitals and clinics may not be standardized, the diagnostic criteria used may vary from one facility to another, and some histories may be more complete than others.</p><p>101 Cohort studies</p><p>Exposed persons may seek more medical care than those who have not been exposed, which may result in overestimation of the occurrence of the disease and give rise to a false (spurious) association.</p><p>A case definition should be established based on the most sensitive and specific tests, particularly for diseases that have a high percentage of subclinical or asymptomatic forms.</p><p>In diseases of low case-fatality, death must not be chosen as an outcome: for endemic parasitic diseases clinical signs and symptoms and laboratory tests are better as outcomes.</p><p>Problems with nonconcurrent cohort studies are similar to those discussed above in relation to exposure. However, if the losses in the exposed and unexposed groups are the same, the relationship between the rates of the outcome will be valid even though the rates calculated may under- or overestimate those outcomes.</p><p>ANALYSIS OF RESULTS</p><p>Measurements of association between risk factors and disease</p><p>As discussed above, in a cohort study the data collected refer to (1) information on exposure of the participants to a given risk factor, and (2) the development in them of a specific disease in consequence of that exposure. Incidence (or death) rates are then calculated for (1) the group of participants exposed and (2) the group of participants not exposed to the risk factor under study. The purpose is to determine whether the rate of incidence among those exposed a/(a+b) is greater than the rate of incidence among those not exposed c/(c+d) (Table 1); if it is found to be greater, it is accepted that there is an association between the risk factor studied and subsequent development of the disease.</p><p>Table 1. Structure of cohort studies</p><p>Exposure or Will develop disease Total characteristic Yes No Present (exposed) a b a + b Absent (not exposed) c d c + d</p><p>The next step is to determine the “force” of this association by calculating the Relative Risk (RR), defined as “the ratio of the incidence rate of the disease in the exposed group to the incidence rate of the disease in the unexposed group ”</p><p>Incidence in exposed group a/(a + b) RR = = Incidence in unexposed group c/(c + d)</p><p>102 Cohort studies</p><p>An RR of 1(one) indicates that there is no association between the risk factor and the disease; as the RR increases, the “force” of the association increases too. Hence the magnitude of the RR reflects the force of the association between the risk factor and the disease. Formulas for calculation of the confidence interval of the RR are available to test its statistical significance. This procedure is indicated for studies in which the duration of monitoring is uniform and constant for all participants in the studied.</p><p>Some cohort studies, however, whether prospective or retrospective, require observations for variably long periods of time, and some participants may be lost to follow-up (refusers, defaulters, etc.) or die at different times, in which case the participants may have different time of observation. In other studies the participants can be recruited or join the study on different dates (during a pre-established period of time) and if the study is ended on a preset date they will have been under observation for different periods of time. In these situations the results must be analyzed by using persons/time of observation as denominator for calculation of the incidence and death rates, or by using life table methods or survival analysis to calculate the cumulative incidence or mortality.</p><p>Persons/time - Persons/time of observation is used as denominator to estimate incidence rates in cohort studies, mainly when the existence of various characteristics (age, sex, ethnic group, etc.) and different durations of monitoring make the calculations needed to construct a life table difficult or even impossible. It takes into consideration both the number of persons being observed and the duration of the observation periods for each participant in the study. Rates are expressed as number of outcomes per persons/time of observation. The principal limitation on the use of persons/time is the premise that the risk of the occurrence of one event per unit time is constant during the observation period, but this assumption is acceptable in most studies.</p><p>Survival analysis - Two different points are considered in a survival model: the time to an event and whether the event occurred. One of the assumptions made by survival analysis is that if the participants were observed long enough, all would experience the event of interest. In these sense, when constructing a survival curve we observe a decrease on the number of participants over time. This method must be used when the conditions requiring the use of persons/time cannot be satisfied. Survival analysis (life tables) is regarded by many as the method of choice for the analysis of cohort studies, in this type of analysis the time interval has a fixed length. It can be used to calculate the probability of an event over a specified period of time and the RR can be computed as the ratio of those probabilities. Another type of survival analysis is the Kaplan-Meier analysis, also called product limit method. In this method the exact survival time for each participant of the study is considered. When stratified Kaplan-Meier curves are presented the log-rank test is used to identify statistical differences.</p><p>The RR calculated by any of the methods discussed above is known as the unadjusted RR; it does not take account of a possible effect from other confounding variables. A confounding variable is an external variable or secondary factor that confounds the association between the risk factor (exposure) and the disease by over- or underestimating the value of the RR found (Figure 3).</p><p>103 Cohort studies</p><p>Figure 3. Confounding variable</p><p>Cause Owing to confounding variable Exposure Exposure</p><p>Observed Observed Confounding association association variable</p><p>Disease Disease</p><p>For a variable to be confounding, it must meet three criteria:</p><p>(1) it must be associated with the exposure of interest (2) it must be a risk factor for the disease (independent of the exposure of interest), and (3) it must not constitute a link between the exposure and the disease.</p><p>Stratification - When the design of a study does not adjust for the confounding variable in the design (by matching groups on confounding variables), the analysis makes use of methods to control its effect. The adjustment may be made by stratifying the data for the possible confounding variables in many 2x2 tables for calculation of the RR in the strata and the adjusted RR (Mantel-Haenszel technique), which is then compared to the unadjusted RR. A statistically significant difference indicates the presence of the confounding variable. </p><p>The example that follows is a hypothetical cohort study for pulmonary tuberculosis among M. tuberculosis infected (exposure) individuals with and without HIV infection (confounder).</p><p>Principal risk factor (exposure): M.tuberculosis infection</p><p>Disease (outcome): Pulmonary tuberculosis Confounding variable: HIV infection (1) Unadjusted RR: </p><p>Pulmonary tuberculosis Total M.tuberculosis infection Yes No Present 39 701 740 Absent 27 1,244 1,271</p><p>RR = 39/740 = 2.48 104 Cohort studies</p><p>27/1,271</p><p>Unadjusted RR = 2.48 95% confidence interval = 1.53-4.02</p><p>(2) Determine whether HIV infection (a possible confounding variable) is associated with the disease and exposure: a) Association with Pulmonary tuberculosis?</p><p>Pulmonary tuberculosis Total</p><p>HIV infection Yes No Yes 23 180 203 No 43 1,765 1,808</p><p>23/203 RR = = 4,76 95% confidence interval = 2.93-7.74 43/1,808 b) Association with M.tuberculosis infection?</p><p>M.tuberculosis infection Total</p><p>HIV infection Yes No Yes 124 79 203 No 616 1,192 1,808</p><p>124/203 RR = = 1.79 95% confidence interval = 1.58-2.04 616/1,808 (3) Adjustment for confounding variable: HIV infection a) First stratum = HIV positive</p><p>Pulmonary tuberculosis Total M.tuberculosis infection Yes No Present 17 107 124 Absent 6 73 79 RR = 17/124 = 1.81 95% confidence interval = 0.74-4.38</p><p>105 Cohort studies</p><p>6/79</p><p> b) Second stratum = HIV negative </p><p>Pulmonary tuberculosis Total M.tuberculosis infection Yes No Present 22 594 616 Absent 21 1,171 1,192</p><p>22/616 RR = = 2.03 95% confidence interval = 1.12-3.66 21/1,192</p><p>(4) Calculation of adjusted RR - The RR adjusted by the Mantel-Haenszel method is a weighted mean of the values of the strata. The formula for calculating it can be found in advanced epidemiology textbooks. This estimate may be calculated directly with the EpiInfo program.</p><p>Adjusted RR = 1.95 95% confidence interval = 1.19-3.19</p><p>(5) Interpretation: The value of the unadjusted RR was 2.48, and after adjustment (and removal of the effect of the confounding variable), went down to 1.95. In this study, therefore, HIV infection is a confounding variable: it overestimated the value of RR.</p><p>Multivariate model such as Cox’s proportional hazard can be used to adjust simultaneously for the effects of several confounding factors. The Cox proportional hazard model is the most commonly used multivariate approach to analyze survival data in medical research. Many of the basic concepts related to logistic regression are applied to Cox proportional hazards modeling. The proportional hazard is the basic assumption for the Cox proportional hazards model, where the hazard ratio (the equivalent to relative risk or Odds ratio) must be constant over time. Another important requirement is to have an adequate sample size, since when multivariate models are fitted to a small dataset the results are not reliable. Proportional hazards model assumes that observations are independent of one another. In other words, these models cannot incorporate the same outcome occurring more than once in the same person.</p><p>106 Cohort studies</p><p>ADVANTAGES AND LIMITATIONS</p><p>Advantages</p><p>1) A cohort study allows the estimation of the risk of developing a specified outcome in individuals exposed to a specific risk factor, compared with persons not exposed to the same risk factor.</p><p>2) When criteria and procedures for conducting a study are established beforehand, the data on exposure and disease can be of good quality, with less possibility of introducing bias when information is being obtained.</p><p>3) Relationships between the risk factor of interest and other outcomes (diseases) can be investigated; including degrees of severity. In the design stage it is important to define the outcomes to be evaluated. In contrast, in case-control studies only one disease is selected.</p><p>4) Depending on the characteristics and the disease under study, information can be obtained on participants whose exposure to the risk factor have changed.</p><p>5) A cohort study does not pose the ethical problems involved in deciding to expose participants to risk factors or treatment, as intervention studies do.</p><p>6) In contrast with case-control studies, in the cohort study the selection of controls is a relatively simple matter.</p><p>107 Cohort studies</p><p>Limitations</p><p>1) In addition of high cost, the cohort study is more difficult to conduct, especially in studies of long duration. Administrative changes and financing difficulties can compromise its completion.</p><p>2) Since a cohort study requires that the entire sample undergo periodic examination, the fact of participating in a study can influence the behavior of the exposed and the unexposed subject relative to the risk factor of interest, and consequently the development of the outcome.</p><p>3) It is inefficient for the study of rare diseases, as cohort studies require large samples to permit the calculation of significant relative risks.</p><p>4) Losses during follow-up may be large, especially in studies of long duration.</p><p>5) Absence of information on exposure and on morbidity on retrospective cohort studies.</p><p>6) Changes of exposure category can lead to errors of classification.</p><p>7) Extrinsic or confounding variables can mask a possible association between the exposure factor and disease, and so over- or underestimate the results.</p><p>108 Cohort studies</p><p>CHECKLIST FOR THE DESIGN OF A COHORT STUDY</p><p>. Define clearly the question to be answered by the study </p><p>. state the hypothesis to be tested and objectives </p><p>. Explain the criteria for exposed and unexposed subjects . laboratory and clinical tests and information to be collected . past information</p><p>. Define reference population to be sampled . source of participants: health services data, population groups, industrial plants, etc. . for retrospective cohorts, determine the existence of unexposed groups that are appropriate for comparison purposes</p><p>. Calculate the sample size</p><p>. define exposed/unexposed ratio . establish the level of significance and power . state the minimum value of the relative risk to be detected . estimate the frequency of the event in the general population (unexposed) . analyse the logistics and time required to recruit participants . estimate the follow-up time considering the endpoint of the study . Design data collection instruments . establish data to be collected and scales for measurement of the variables . design data forms and pretest them . prepare instruction manuals with information and definitions . Define the diagnostic methods . define endpoints . define how the laboratory methods and clinical tests are to be interpreted . explain the intervals at which the tests are to be performed during follow- up . Spell out the criteria for study eligibility and any relevant ethical issues</p><p>. age group, sex, and biological and physical conditions . procedure for recruitment of participants . procedures for obtaining informed consent . strategies for ensuring confidentiality of the information collected . medical care of participants who present with event during follow-up</p><p>109 Cohort studies</p><p>CHECKLIST FOR THE DESIGN OF A COHORT STUDY</p><p>. Describe the follow-up procedure</p><p>. length of follow-up . manner and intervals of contact with participants . strategies for minimizing losses during follow-up</p><p>. Describe the plan of analysis . comparisons to be made . calculate the incidence of the outcome and relative risk . describe the methods to be used</p><p>ADDITIONAL READING</p><p>BEAGLEHOLE, R., BONITA, R. & KJELLSTORM, T. Basic Epidemiology. World Health Organization, Geneva, 1993.</p><p>BRESLOW, N.E. & DAY, N.E. Statistical methods in cancer research. Switzerland, International Agency for Research on Cancer, Lyon, V.1I - The Design and Analysis of Cohort Studies. IARC Scientific Publications No. 82, 1987.</p><p>GORDIS, L. Epidemiology. Philadelphia, Editora W.B. Saunders Company, 2004. </p><p>KHAN, H.A. & CHRISTOPHER, T.S. Statistical Methods in Epidemiology. New York, Editora Oxford University Press, 1986.</p><p>KELSEY, J.L., THOMPSON, W.D. & EVANS, A.S. Methods in Observational Epidemiology. New York, Editora Oxford University Press, 1986.</p><p>LAST, J.M. A Dictionary of Epidemiology. 3 ed., New York, Editora Oxford University Press, 1995.</p><p>LILIENFELD, D.E. & STOLLEY, P. Foundations of Epidemiology. 3 ed. New York, Editora Oxford University Press, 1994. </p><p>110 Cohort studies</p><p>EXERCISES</p><p>Files: ViewGhanabcl ViewGhanafcl ViewGhanapre ViewGhanapos ViewGhanasmc ViewGhanasm ViewIsfahan ViewNeonatalD View Staphylo</p><p>Exercise 1</p><p>Impact of permetrim impregnated bednets on child mortality in Kasena- Nankana district, Ghana: a randomized controlled trial. Binka et al (1996).</p><p>A community-based randomized, controlled trial of permethrin impregnated bednets was carried out in a rural area of northern Ghana to assess the impact on children mortality in an area of intense transmission of malaria and no tradition of bednet use. The district was divided into 96 geographical areas and 48 randomly selected household clusters were provided with permethrin impregnated bednets. A longitudinal demographic surveillance system was used to record births, deaths and migrations to evaluate compliance and to measure child mortality between July 1993 and June 1995. **Before starting the exercise route out the results to a HTML file named “Results Ghana”. ROUTEOUT 'Results Ghana ' [Figure 1] [Figure 1 – Route out results]</p><p>2- Define the folder to save the HTM file</p><p>4- Mark this box if you 3- Write the file name w ant to replace an existing file</p><p>1- Click on RouteOut</p><p>111 Cohort studies</p><p>Question 1. Sample Size The trial was designed to have a 90% power to detect a minimum 30% reduction in all cause mortality associated with impregnated bednets use. There were about 13,000 children enrolled in each of the two groups during a 2-year follow-up. Assuming an expected overall mortality rate of 2.3 deaths/100 child-year, was the number of participants enrolled adequate?</p><p>Note 1: Run EPITABLE Select SAMPLE/ SAMPLE SIZE / TWO PROPORTIONS Return to Analysis</p><p>Question 2. Mortality rates Mortality data are provided for a pre- and pos-intervention periods. Calculate mortality rates by age group for each period. Calculate relative risks and 95% CI for child mortality associated with bednet use for the post- intervention period. What is your conclusion on bednet use? What is the estimated impact in all cause mortality rate in the post intervention period?</p><p>Note 2: [Start by drawing a table with row and columns labels - Commands for Table 1] READ ‘C:\EPIGUIDE\EpiGuide.mdb':viewGHANABCL [Figure 2] Calculate years of follow-up by group (treated or not), total denominators for pre-intervention period MEANS FOLLYR BEDNET TABLES=(-) [Figure 3] [NOTE THE RESULTS OF THE TOTAL COLUMN] [for sum of follow-up by study group and age group] SELECT BEDNET = 1 [Figure 4] MEANS FOLLYR AGEGR TABLES=(-) [NOTE THE RESULTS OF THE TOTAL COLUMN] SELECT [Figure 5] SELECT BEDNET = 2 MEANS FOLLYR AGEGR TABLES=(-) [NOTE THE RESULTS OF THE TOTAL COLUMN] SELECT</p><p>[Figure 2 – Read command]</p><p>112 Cohort studies</p><p>1- Click on Read from the Analysis Commands tree</p><p>3 – Identify the data file you w ill use in the exercise</p><p>2 – Change to the desired project: EPIGUIDE.MDB</p><p>4 – Click Ok</p><p>[Figure 3 – Means command] 2- Choose the variable to 3- Choose the variable to apply the means command use for com parison</p><p>1- Click 4- Click Means Settings</p><p>5 – Uncheck the Show Tables in Output box</p><p>[Figure 4 – Select command] 113 Cohort studies</p><p>1- Click Select 3- Define the selection criteria</p><p>2- Choose the 4- Click OK w hen variable(s) to build the finished selection criteria [Figure 5 – Cancel Select]</p><p>1- Click Cancel Select</p><p>2 - Click OK to cancel current selection criteria</p><p>114 Cohort studies</p><p>Note 2- continue… READ ‘C:\ EPIGUIDE \EpiGuide.mdb':viewGHANAFCL Sum of follow-up years by group treated or not, total denominators for post-intervention period MEANS FOLLYR BEDNET TABLES=(-) for sum of follow-up by group and age group SELECT BEDNET = 1 MEANS FOLLYR AGEGR TABLES=(-) [NOTE THE RESULTS OF THE TOTAL COLUN] SELECT SELECT BEDNET = 2 MEANS FOLLYR AGEGR TABLES=(-) [NOTE THE RESULTS OF THE TOTAL COLUN] SELECT Calculating the numerators for baseline (pre intervention) READ ‘C:\ EPIGUIDE \EpiGuide.mdb':viewGHANAPRE For the total line: TABLES OUTCOME BEDNET [Figure 6] For each age group DEFINE AGEEXIT [standard] [Figure 7] ASSIGN AGEEXIT = TRUNC(AGEMN+FOLLMN) [Figure 8] DEFINE AGEGR [standard] IF AGEEXIT >= 6 AND AGEEXIT < 12 THEN ASSIGN AGEGR = 6 END [Figure 9] IF AGEEXIT >= 12 AND AGEEXIT < 24 THEN ASSIGN AGEGR = 12 END IF AGEEXIT >= 24 AND AGEEXIT < 36 THEN ASSIGN AGEGR = 24 END IF AGEEXIT >= 36 AND AGEEXIT < 48 THEN ASSIGN AGEGR = 36 END IF AGEEXIT >= 48 THEN ASSIGN AGEGR = 48 END Display the final outcome in each age group according to treated or not treated bednet TABLES OUTCOME AGEGR STRATAVAR=BEDNET [Figure 10]</p><p>115 Cohort studies</p><p>[Figure 6 – Tables command]</p><p>3- Choose the 2- Choose the Outcome v ariable Exposure Variable</p><p>4 – Click Ok</p><p>1- Click on Tables</p><p>[Figure 7 – Define New variable] 2 – Type the new variable name 1- Click Define to create a new variable</p><p>[Figure 8 - Assign values] 3 - Choose f rom the Available Variables to 2 - Choose the construct the variable to receiv e the expression new v alues </p><p>5 – Click OK 4 - Revise the 1 – Click Assign Assign Expression 116 Cohort studies</p><p>[Figure 9 – IF command]</p><p>3</p><p>2 1 4</p><p>10</p><p>6</p><p>8</p><p>7</p><p>5 9</p><p>1 – Click IF to establish conditions for the new variable 2 – Choose the variable to build the condition 3 – Create the condition(s) to assign the values for the new variable 4 – Click THEN to access the THEN Block 5 – Click ASSIGN 6 – Choose the variable to receive the new values 7 – Choose from the Available variables to construct the expression 8 – Revise the assign expression 9 – Click ADD to return to the IF window 10 – Click OK when finished</p><p>117 Cohort studies</p><p>[Figure 10 – Tables with stratification]</p><p>2- Choose the 3- Choose the 4- Choose the Exposure Variable Outcome variable Variable to Stratify by</p><p>5 – Click Ok</p><p>1- Click on Tables</p><p>Note 2- continue… Calculate numerators for post-intervention READ ‘C:\ EPIGUIDE \EpiGuide.mdb':viewGHANAPOS For the total line: TABLES OUTCOME BEDNET For each age group DEFINE AGEEXIT [standard] ASSIGN AGEEXIT = TRUNC(AGEMN+FOLLMN) DEFINE AGEGR [standard] IF AGEEXIT >= 6 AND AGEEXIT < 12 THEN ASSIGN AGEGR = 6 END IF AGEEXIT >= 12 AND AGEEXIT < 24 THEN ASSIGN AGEGR = 12 END IF AGEEXIT >= 24 AND AGEEXIT < 36 THEN ASSIGN AGEGR = 24 END IF AGEEXIT >= 36 AND AGEEXIT < 48 THEN ASSIGN AGEGR = 36 END IF AGEEXIT >= 48 THEN ASSIGN AGEGR = 48 END</p><p>118 Cohort studies</p><p>Display the final outcome in each age group according to treated or not treated bednet TABLES OUTCOME AGEGR STRATAVAR= BEDNET</p><p>To calculate the RR and the 95% CIs Run EPITABLE – select STUDY, then select COHORT/CROSS SECTIONAL, then select INCIDENCE DENSITY [Use the results obtained] Press F10 to leave EPITABLE Return to ANALYSIS</p><p>For Open Epi: Access Open Epi from the EPIGUIDE CD or go to www.openepi.com.Click click on COMPARE TWO RATES on the Person time folder. Click Enter New Data. Enter the values on the Open Epi Input Table window. Click calculate. Note the results. [Figure 11] Return to ANALYSIS</p><p>Question 3. Cause specific mortality rate Calculate cause specific (malaria, acute respiratory infection gastro-enteritis, accidents, other known causes and indeterminate) mortality rates for the overall study population in the post-intervention period. How did the intervention affect the malaria specific mortality rate? Compare this results with that of all cause mortality in post-intervention group obtained in question 2.</p><p>Note 3: Commands for table 2 – Cause specific mortality rate Denominators READ ‘C:\ EPIGUIDE \EpiGuide.mdb':viewGHANASMC MEANS FOLLYR BEDNET TABLES=(-) [NOTE THE RESULTS OF THE TOTAL COLUN]</p><p>Numerators READ ‘C:\ EPIGUIDE \EpiGuide.mdb':viewGHANASM</p><p>TABLES BEDNET MAL TABLES BEDNET ARI TABLES BEDNET GASTR TABLES BEDNET ACCIDENT TABLES BEDNET OTHER TABLES BEDNET UNDETMIS</p><p>To calculate the RR and the 95% Cis Run EPITABLE – select STUDY, then select COHORT/CROSS SECTIONAL, then select INCIDENCE DENSITY 119 Cohort studies</p><p>[Use the results obtained] Press F10 to leave EPITABLE Return to ANALYSIS</p><p>For Open Epi: Access Open Epi from the EPIGUIDE CD or go to www.openepi.com.Click click on COMPARE TWO RATES on the Person time folder. Click Enter New Data. Enter the values on the Open Epi Input Table window. Click calculate. Note the results. Return to ANALYSIS</p><p>120 Cohort studies</p><p>Exercise 2</p><p>Safety and efficacy of killed L. major vaccine plus BCG against zoonotic cutaneous leishmaniasis in Iran. Momeni et al., 1998. </p><p>A randomized double-blind controlled trial was conducted in Isfahan, Iran, to evaluate the safety and protective efficacy of a single dose of an autoclaved-killed L. major promastigotes vaccine (ALM), mixed with BCG against zoonotic cutaneous leishmaniasis (CL) vs. BCG as control group. Volunteers were examined on days 1, 7, 30 and 80 after vaccination to assess the presence of systemic and local side effects. On day-80 and one year post vaccination a leishmanin skin test (LST) was performed. The incidence of CL was assessed by passive surveillance and by five active follow-up visits; at 6,8,10,18 and 24 months after vaccination. The file ViewIsfahan part of the EPIGUIDE.MDB project contains records of the 2310 participants, a list of variables and codes is appended.</p><p>**Before starting the exercise route out the results to a HTML file named “Results ISFAHAN”. ROUTEOUT 'Results ISFAHAN '</p><p>Question 1. Sample size The study was designed to detect a 50% reduction in incidence of cutaneous leishmaniasis (CL), at 5% significance level and power of 80%. Assuming a CL annual incidence of 5% and a dropout rate of 20%/year, do you consider the number of participants enrolled as sufficient?</p><p>Note 1: Open the file: READ 'C:\ EPIGUIDE \EpiGuide.mdb':viewISFAHAN</p><p>Run EPITABLE to calculate the sample size Select SAMPLE, then SAMPLE SIZE, and TWO PROPORTIONS Press F10 to leave EPITABLE Return to ANALYSIS </p><p>[Select only the primary cases (4 secondary cases are included in the data base)] [Before proceeding assign 8 (Not applicable) to the records where LEISHX has missing data.] SET MISSING=(+) IF LEISHX = (.) THEN ASSIGN LEISHX = 8 END SET MISSING=(-) [Create a new data file: ISFAHAN2. Use the WRITE Replace command]</p><p>WRITE REPLACE "Epi2000" 'C:\EPIGUIDE\EpiGuide.mdb':isfahan2 * </p><p>[Open the new data table. Use option “Show all”</p><p>121 Cohort studies</p><p>READ 'C:\ EPIGUIDE \EpiGuide.mdb':ISFAHAN2 SELECT LEISHX <> 2 FREQ GROUP [Figure 12]</p><p>[Figure 12 – Frequencies command] 2 – Choose the variable(s)</p><p>1- Click Frequenci es</p><p>3 - Click OK w hen finished</p><p>Question 2. Randomization Compare the two groups with regard to baseline characteristics: mean age (AGE), sex (SEX) and the PPD response (RESPPD). To compare the PPD response intensity create a new variable (PPDGR) and allocate the participants into groups of 0, 1-4, 5-9 and >=10mm diameter of response. Are the two groups comparable?</p><p>Note 2: MEANS AGE GROUP TABLES=(-)</p><p>TABLES SEX GROUP [Create a new variable “PPDGR” (PPD result groups)] DEFINE PPDGR [standard] IF RESPPD = 0 then ASSIGN PPDGR = 0 END IF RESPPD >= 1 AND RESPPD < 5 THEN ASSIGN PPDGR =1 END IF RESPPD >= 5 AND RESPPD < 10 THEN ASSIGN PPDGR = 2 END IF RESPPD >= 10 THEN ASSIGN PPDGR = 3 END TABLES PPDGR GROUP</p><p>122 Cohort studies</p><p>Question 3. Adverse reactions Compare the side effects observed at 1, 7, 30 and 80 days after vaccination in the two groups. Compare frequency of pain (PAIN1, PAIN7, PAIN30 and PAIN80); induration (INDUR1, INDUR7, INDUR30 and INDUR80); ulcer (ULCER1, ULCER7, ULCER30 and ULCER80); itching (ITCH1, ITCH7, ITCH30 and ITCH80); and lymphadenopathy (LYMPH1, LYMPH7, LYMPH30 and LYMPH80). Recode the variables as 0=no side effects or 1=side-effects of any grade. Is there any difference in adverse reaction frequency between vaccine and BCG control group?</p><p>Note 3: [Continue to work with primary cases]</p><p>DEFINE PAIN1R [standard] RECODE PAIN1 TO PAIN1R 0=0 1-3 = 1 END DEFINE PAIN7R [standard] RECODE PAIN7 TO PAIN7R 0=0 1-3 = 1 END DEFINE PAIN30R RECODE PAIN30 TO PAIN30R 0=0 1-2 = 1 END DEFINE PAIN80R RECODE PAIN80 TO PAIN80R 0=0 1-2 = 1 END TABLES PAIN1R GROUP TABLES PAIN7R GROUP TABLES PAIN30R GROUP TABLES PAIN80R GROUP</p><p>DEFINE INDUR1R [standard] RECODE INDUR1 TO INDUR1R 0=0 1-3 = 1 END DEFINE INDUR7R [standard] RECODE INDUR7 TO INDUR7R 0=0 1-3 = 1 END</p><p>123 Cohort studies</p><p>DEFINE INDUR30R [standard] RECODE INDUR30 TO INDUR30R 0=0 1-3 = 1 END DEFINE INDUR80R [standard] RECODE INDUR80 TO INDUR80R 0=0 1-3 = 1 END TABLES INDUR1R GROUP TABLES INDUR7R GROUP TABLES INDUR30R GROUP TABLES INDUR80R GROUP</p><p>DEFINE ULCER1R [standard] RECODE ULCER1 TO ULCER1R 0=0 1- 2 = 1 END DEFINE ULCER7R [standard] RECODE ULCER7 TO ULCER7R 0=0 1-3 = 1 END DEFINE ULCER30R [standard] RECODE ULCER30 TO ULCER30R 0=0 1-3 = 1 END DEFINE ULCER80R [standard] RECODE ULCER80 TO ULCER80R 0=0 1-2 = 1 END TABLES ULCER1R GROUP TABLES ULCER7R GROUP TABLES ULCER30R GROUP TABLES ULCER80R GROUP</p><p>DEFINE ITCH1R [standard] RECODE ITCH1 TO ITCH1R 0=0 1-3 = 1 END DEFINE ITCH7R [standard] RECODE ITCH7 TO ITCH7R 0=0 1-3 = 1 END</p><p>DEFINE ITCH30R [standard] 124 Cohort studies</p><p>RECODE ITCH30 TO ITCH30R 0=0 1-2 = 1 END DEFINE ITCH80R [standard] RECODE ITCH80 TO ITCH80R 0=0 1-2 = 1 END TABLES ITCH1R GROUP TABLES ITCH7R GROUP TABLES ITCH30R GROUP TABLES ITCH80R GROUP</p><p>DEFINE LYMPH80R [standard] RECODE LYMPH80 TO LYMPH80R 0=0 1-2 = 1 END</p><p>TABLES LYMPH1 GROUP TABLES LYMPH7 GROUP TABLES LYMPH30 GROUP TABLES LYMPH80R GROUP SELECT [to disable selection]</p><p>Question 4. Skin test response Compare the frequencies and means LST response at day 80 (LST80) and 1 year (LST1Y) in the two groups. Group LST responses into new variables (LST80GR and LST1YGR) in the following categories: 0, 1-4, 5-9, >=10. Group LST responses (day 80 and 1 year) into a binary variables (LST80GR2, LST1YGR2) for LST <5 or >=5. Note that for a proper evaluation of 1 year response, incident cases during the first year should be excluded (YEAR<>1).</p><p>Note 4: [Create new variable “LST80GR”] DEFINE LST80GR [standard] IF LST80 = 0 THEN ASSIGN LST80GR = 0 END</p><p>IF LST80 > 0 AND LST80 < 5 THEN ASSIGN LST80GR = 1 END IF LST80 >= 5 AND LST80 < 10 THEN ASSIGN LST80GR = 2 END</p><p>125 Cohort studies</p><p>IF LST80 >= 10 THEN ASSIGN LST80GR = 3 END TABLES LST80GR GROUP [Create new variable “LST80GR2”] DEFINE LST80GR2 [standard] IF LST80 >= 5 THEN ASSIGN LST80GR2 = 1 END IF LST80 >= 0 AND LST80 < 5 THEN ASSIGN LST80GR2 = 2 END TABLES LST80GR2 GROUP MEANS LST80 GROUP TABLES=(-) To analyse LST response after 1 year exclude incident cases occurring during the first year as the LST results are likely to be altered by the disease SELECT YDIAG <> 1 [Create new variable “LST1YGR”] DEFINE LST1YGR [standard] IF LST1Y = 0 THEN ASSIGN LST1YGR = 0 END IF LST1Y > 0 AND LST1Y < 5 THEN ASSIGN LST1YGR = 1 END IF LST1Y >= 5 AND LST1Y < 10 THEN ASSIGN LST1YGR = 2 END IF LST1Y >= 10 THEN ASSIGN LST1YGR = 3 END TABLES LST1YGR GROUP</p><p>[Create new variable “LST1YGR2”] DEFINE LST1YGR2 [standard] IF LST1Y >= 5 THEN ASSIGN LST1YGR2 = 1 END IF LST1Y >= 0 AND LST1Y < 5 THEN ASSIGN LST1YGR2 = 2 END TABLES LST1YGR2 GROUP MEANS LST1Y GROUP TABLES=(-) SELECT [to disable selection]</p><p>126 Cohort studies</p><p>Question 5. Incidence – Vaccine efficacy Compare the overall cumulative incidence of CL over the two years of follow-up in each group. Calculate the overall protective effect of ALM+BCG as compared to BCG alone.</p><p>Note 5: TABLES GROUP LEISH [Note the results]</p><p>Run EPITABLE to calculate the vaccine efficacy Select STUDY, then VACCINE EFFICACY, and COHORT STUDY</p><p>Press F10 to leave EPITABLE Return to ANALYSIS</p><p>Question 6. Stratified analysis (by LST response) Using the binary variables LST80GR2 and LST1YGR2 calculate CL incidence on the first and second year of follow-up (YDIAG) for each group. Compare incidence rates according to PPD response and year of incidence. Create new variable: (YDIAG1) to distinguish first-year incident cases.</p><p>Note 6: [Select the ALM+BCG group] SELECT GROUP = 1 [Create new variable “YDIAG1”] DEFINE YDIAG1 [standard] RECODE YDIAG TO YDIAG1 1=1 2-3=3 END TABLES LST80GR2 YDIAG1 SELECT [to disable selection]</p><p>[Select the BCG group] SELECT GROUP = 2 TABLES LST80GR2 YDIAG1 SELECT [to disable selection]</p><p>[Exclude the incident cases of the first year and select the ALM+BCG group] SELECT YDIAG <> 1 AND GROUP = 1 TABLES LST1YGR2 YDIAG SELECT [to disable selection]</p><p>[Exclude the incident cases of the first year and select the BCG group]</p><p>127 Cohort studies</p><p>SELECT YDIAG <> 1 AND GROUP = 2 TABLES LST1YGR2 YDIAG SELECT [to disable selection] EXIT [to leave Analysis]</p><p>128 Cohort studies</p><p>Exercise 3</p><p>A live-birth cohort study was carried out in Goiania, Central Brazil, from November 1999 to October 2000. Linked birth and infant death certificates were used to ascertain the cohort of live born infants. An additional active surveillance system of neonatal based mortality was implemented. Exposure variables were collected from birth and death certificates. The objective of the study was to identify potential prognostic factors for neonatal mortality among newborns referred to intensive care units. Details on the methodology can be found in: Weirich, 2005. The file ViewNeontalD part of the EPIGUIDE.MDB project contains records of the 875 newborns; a list of variables and codes is appended.</p><p>**Before starting the exercise route out the results to a HTML file named “Results Neonatald”. ROUTEOUT 'Results neonatald '</p><p>Question 1. According to the data provided what was the mortality rate and respective 95% CI for newborns admitted to the NICU?</p><p>Note 1: READ 'C:\ EPIGUIDE \EpiGuide.mdb':viewNeontalD FREQ DEATH</p><p>Question 2. What was the proportion of deaths occurring before 24 hours of admission to the NICUs? Among the neonatal deaths what was the proportion of infants weighting at least 2,500 grams? What was the age distribution (age group <20, 20-34, >34) of the mothers of those infants?</p><p>Note 2: SELECT DEATH = (+) FREQ DAYS FREQ WEIGHT</p><p>[To calculate the mother’s age distribution, create a new variable AGEMGR. Consider <20, 20-34, >34 years of age.] DEFINE AGEMGR RECODE AGEM TO AGEMGR 14 – 19 = 1 20 – 34 = 2 35 – 42 = 3 END [Figure 13]</p><p>FREQ AGEMGR</p><p>SELECT [to cancel selection]</p><p>[Figure 13 – Recode command] 129 Cohort studies</p><p>3 - Choose source 4 – Choose variable destination variable (new )</p><p>1 – Define the 7 – Click OK new variable</p><p>2 – Click Recode 5 – Type old values 6 – Type the new or range of values values. Press enter to go to the next line</p><p>Question 3. Taking into account all admissions to the NICUs describe the mother’s characteristics according to: number of pre natal visits, type of delivery and type of health insurance.</p><p>Note 3: FREQ PNVISITS FREQ DELIVERY FREQ HEALTHI</p><p>Question 4. Among the low birth weight infants (<2500) how many were preterm (born before 37 weeks of gestation)?</p><p>Note 4: SELECT WEIGHT < 2500 FREQ GESTAGE SELECT</p><p>Question 5. Calculate (in univariate analysis) the hazard ratios for neonatal 130 Cohort studies</p><p> mortality according to the follow prognostic factors: Type of health insurance, Marital satus, Mother age (group), multifetal pregnancy, number of prenatal visits, gestational age, type of delivery, neonatal sex, neonatal birth weight (>2,500; 1,500-2,499; <1,499) and 5 minutes apgar score (7-10, 4-6, 0-3) Which of these prognostic factors are statistically associated with neonatal mortality?</p><p>Note 5: [Sum 1 to the variable DAYS creating a new variable DAYS_R to calculate the hazard ratios]</p><p>DEFINE DAYS_R ASSIGN DAYS_R = DAYS + 1</p><p>COXPH DAYS_R = (HEALTHI) * death ( (+) ) TIMEUNIT="Days" DIALOG GRAPHTYPE="None" [Figure 14]</p><p>COXPH DAYS_R = (MSTATUS) * death ( (+) ) TIMEUNIT="Days" DIALOG GRAPHTYPE="None"</p><p>DEFINE AGEMGR RECODE AGEM TO AGEMGR 14 - 19 = 1 20 - 34 = 2 35 - 42 = 3 END</p><p>COXPH DAYS_R = (AGEMGR) * death ( (+) ) TIMEUNIT="Days" DIALOG GRAPHTYPE="None"</p><p>COXPH DAYS_R = (MFETAL) * death ( (+) ) TIMEUNIT="Days" DIALOG GRAPHTYPE="None"</p><p>COXPH DAYS_R = (PNVISITS) * death ( (+) ) TIMEUNIT="Days" DIALOG GRAPHTYPE="None"</p><p>COXPH DAYS_R = (GESTAGE) * death ( (+) ) TIMEUNIT="Days" DIALOG GRAPHTYPE="None"</p><p>COXPH DAYS_R = (DELIVERY) * death ( (+) ) TIMEUNIT="Days" DIALOG GRAPHTYPE="None"</p><p>COXPH DAYS_R = (SEX) * death ( (+) ) TIMEUNIT="Days" DIALOG GRAPHTYPE="None"</p><p>[Create a new variable WEIGHTGR. Consider: >=2,500g; 1,500g-2,499g; <1,499g with code 1, 2 and 3 respectively] 131 Cohort studies</p><p>DEFINE WEIGHTGR RECODE WEIGHT TO WEIGHTGR 2500 – 5700 = 1 1500 – 2499 = 2 510 -1499 = 3 END</p><p>COXPH DAYS_R = (WEIGHTGR) * death ( (+) ) TIMEUNIT="Days" DIALOG GRAPHTYPE="None"</p><p>[Create a new variable APGAR5GR. Consider Apgar groups of: 7-10, 4-6, 0-3 with code 1, 2 and 3 respectively)</p><p>DEFINE APGAR5GR RECODE APGAR5 TO APGAR5GR 7 - 10 = 1 4- 6 = 2 0 – 3 = 3 END</p><p>COXPH DAYS_R = (APGAR5GR) * death ( (+) ) TIMEUNIT="Days" DIALOG GRAPHTYPE="None"</p><p>[Figure 14 – Cox Proportional hazards] 2- Choose 2- Choose Censored Value for Variable Uncensored</p><p>1- Click Cox Proportional</p><p>3 – Choose Tim e 5- Choose variables to 4 – Choose Tim e Variable compose the model. Unit Create Dum m y variables 6- Choose NONE for if necessary Graph Options</p><p>Question 6. To identify the factors independently associated with neonatal 132 Cohort studies</p><p> mortality conduct a multivariate analysis (Cox proportional hazard) including in the model the following variables: Type of health insurance, multifetal pregnancy, number of prenatal visits, gestational age, type of delivery, neonatal birth weight (>2,500; 1,500-2,499; <1,499) and apgar (7-10, 4-6, 0-3) .</p><p>Note 6: COXPH DAYS_R = (WEIGHTGR) (HEALTHI) (MFETAL) (PNVISITS) (GESTAGE) (DELIVERY) (APGAR5GR) * death ( (+) ) TIMEUNIT="Days" DIALOG GRAPH=WEIGHTGR2 GRAPHTYPE="None"</p><p>133 Cohort studies</p><p>Exercise 4</p><p>The aim of this study was to evaluate the incidence of bloodstream infection due to Staphylococcus aureus and the risk factors for mortality. The design was a two-year retrospective cohort of patients more than one year of age with clinically significant and microbiologically documented bloodstream infection due to S. aureus between January 2000 and December 2001 in a tertiary teaching hospital in Midwest Brazil. Details of the methodology can be found in: Guilarde et al., 2006. The data file ViewStapylo from the Epiguide.MDB project contains records of the 111 participants; a list of variables and codes is appended.</p><p>**Before starting the exercise route out the results to a HTML file named “Results Staphylo”. ROUTEOUT 'Results Staphylo'</p><p>Question 1. Among the S. aureus isolates what was the percentage of meticillin resistance and its respective 95% confidence interval?</p><p>Note 1: READ 'C:\ EPIGUIDE \EpiGuide.mdb':viewNeontalD FREQ MARSA</p><p>Question 2. Describe the overall data for this study regarding the following characteristics: percentage of hospital acquired infection; overall mortality rate and mortality rate due to S. aureus bacteraemia; percentage of patients diagnosed with primary bacteraemia.</p><p>Note 2: FREQ HOSPINF FREQ DEATH FREQ DEATHB FREQ BACP</p><p>Question 3. Construct a table to describe the characteristics of the patients with meticillin resistant Staphylococus aureus (MRSA) and meticillin sensitive S. aureus (MSSA) bacteraemia. Consider the following data: sex, age (<60; >= 60), use of immunosuppressant therapy, length of stay of central venous catheter CVC (<=10; >10).</p><p>Note 3: TABLES SEX MRSA TABLES AGEGR MRSA TABLES IMMUNOT MRSA TABLES CVCSTAY MRSA</p><p>Question 4. Calculate the potential prognostic death factors (hazard ratios and 95% CI) associated with S. aureus bacteraemia in univariate analysis, present the results in a table. Include the following variables: sex, age group, hospital acquired infection, 134 Cohort studies</p><p> susceptibility to meticillin, clinical status, and adequacy of the initial antimicrobial therapy. Which variables were significantly associated with death?</p><p>Note 4: [To calculate the hazard ratios add 1 to the variable DAYS creating a new variable DAYS_R] DEFINE DAYS_R ASSIGN DAYS_R = DAYS + 1</p><p>COXPH days_r = (SEX) * DEATHB ( (+) ) TIMEUNIT="Days" DIALOG GRAPHTYPE="None"</p><p>COXPH days_r = (AGEGR) * DEATHB ( (+) ) TIMEUNIT="Days" DIALOG GRAPHTYPE="None"</p><p>COXPH days_r = (HOSPINF) * DEATHB ( (+) ) TIMEUNIT="Days" DIALOG GRAPHTYPE="None"</p><p>COXPH days_r = (MRSA) * DEATHB ( (+) ) TIMEUNIT="Days" DIALOG GRAPHTYPE="None"</p><p>COXPH days_r = (CLINICAL) * DEATHB ( (+) ) TIMEUNIT="Days" DIALOG GRAPHTYPE="None"</p><p>COXPH days_r = (ANTIMICROB) * DEATHB ( (+) ) TIMEUNIT="Days" DIALOG GRAPHTYPE="None"</p><p>COXPH days_r = (IMMUNOT) * DEATHB ( (+) ) TIMEUNIT="Days" DIALOG GRAPHTYPE="None"</p><p>Question 5. Apply the Kaplan-Meir Survival method to build a survival curve to compare patients with MRSA and MSSA bacteraemia. Is the difference statistically significant?</p><p>Note 5: KMSURVIVAL DAYS_R = MRSA * DEATHB ( (+) ) TIMEUNIT="Days" GRAPHTYPE="Survival Probability" [Figure 15]</p><p>135 Cohort studies</p><p>[Figure 15 – Kaplan-Meier Survival]</p><p>2- Choose Censored 3- Choose Value for Variable Uncensored</p><p>1- Click Kaplan- Meier Survival</p><p>4- Choose Time Variable 5- Choose Time Unit</p><p>6- Choose Group 7- Select Graph Variable Type</p><p>Question 6. Conduct a multivariate analysis applying the Cox proportional Hazards method to adjust for potential confounders. Include the variables that were statistically significant in the univariate model (clinical severity, adequacy of initial antimicrobial therapy and meticillin susceptibility) and variables considered to be potential confounders (sex, age). After adjustment which variables showed to be independently associated with fatal outcome?</p><p>Note 6: COXPH DAYS_R = (AGEGR) ANTIMICROB CLINICAL MRSA (SEX) * DEATHB ( (+) ) TIMEUNIT="Days" DIALOG GRAPHTYPE="None"</p><p>136 Cohort studies</p><p>REFERENCES</p><p>BINKA F.N., KUBAJE A, ADJUIK M. WILLIAMS L.A., LENGELER C. MAUDE G.H., ARMAH G.E., KAJIHARA B., ADIAMAH J.H. & SMITH P.G. Impact of permethrin impregnated bednets on child mortality in Kassena-Nankana district, Ghana: a randomized controlled trial. Tropical Medicine and International Health, 1(2): 147-154, 1996.</p><p>MOMENI A.Z., JALAYER T., EMAMJOMEH M., KHAMESIPOUR A., ZICKER F., GHASSEMI R.L., DOWLATI Y., SHARIFI I., AMINJAVAHERI M., SHARIFI A., ALIMOHAMMADIAN H.M., HASHEMI-FESHARKI R., NASSERI K., GODAL T., SMITH P.G. & MODABBER F. A randomised, double blind, controlled trial of a killed L. major vaccine plus BCG against zoonotic cutaneous leishmaniasis in Iran. Vaccine. 17:466-472, 1998.</p><p>WEIRICH CF, ANDRADE A.L.S.S., TURCHI M.D., SILVA S.A., MORAIS-NETO O.L., MINAMISAVA R, MARQUES S.M. Neonatal mortality in intensive care units of Central Brazil. Rev. Saúde Pública, 39(5): 775-81, 2005.</p><p>GUILARDE A.O.,TURCHI M.D., MARTELLI C.M.T. & PRIMO M.G.B. Staphylococcus aureus bacteraemia: incidence, risk factors and predictors for death in a Brazilian teaching hospital.. Journal of Hospital Infection, 63:330-6, 2006.</p><p>For Analysis:</p><p>DEAN AG, ARNER TG, SUNKI GG, FRIEDMAN R, LANTINGA M, SANGAM S, ZUBIETA JC, SULLIVAN KM, BRENDEL KA, GAO Z, FONTAINE N, SHU M, FULLER G. Epi Info™ a database and statistics program for public health professionals. Centers for Disease Control and Prevention, Atlanta, Georgia, USA, 2002. http://www.cdc.gov/epiinfo/downloads.htm</p><p>DEAN A.G., DEAN J.A., COULOMBIER D. et al. Epi Info™, Version 6.04, a word processing, database, and statistics program for public health on IBM-compatible microcomputers. http://www.cdc.gov/epiinfo/Epi6/ei6.htm</p><p>DEAN, A., SULLIVAN, K, & SOE, M.M. OpenEpi - Open Source Epidemiologic Statistics for Public Health. http://www.openepi.com</p><p>137 Cohort studies</p><p>DATA FILE DICTIONARY</p><p>Project: EPIGUIDE.MDB File: ViewGhanabcl</p><p>Variable Description Code Description of code BNC Identification of the cluster 1-96 1 Treated BEDNET Group allocation 2 Not treated 6 6-11 months 12 12-23 months AGEGR Age group in months 24 24-35 months 36 36-47 months 48 48-59 months FOLLYR Child/years follow-up</p><p>Project: EPIGUIDE.MDB File: ViewGhanafcl Variable Description Code Description of code BNC Identification of the cluster 1-96 1 Treated BEDNET Group allocation 2 Not treated 6 6-11 months 12 12-23 months AGEGR Age group in months 24 24-35 months 36 36-47 months 48 48-59 months FOLLYR Child/years follow-up</p><p>Project: EPIGUIDE.MDB File: ViewGhanapre</p><p>Variable Description Code Description of code ID Identification of the participant 1 Treated BEDNET Group allocation 2 Not treated FOLLMN Total months of follow-up AGEMN Age in months at the trial entry Outcome at the end of follow-up 0 Alive OUTCOME period 1 Dead</p><p>138 Cohort studies</p><p>Project: EPIGUIDE.MDB File: Viewghanapos</p><p>Variable Description Code Description of code ID Identification of the participant 1 Treated BEDNET Group allocation 2 Not treated FOLLMN Total months of follow-up AGEMN Age in months at the trial entry Outcome at the end of follow-up 0 Alive OUTCOME period 1 Dead</p><p>Project: EPIGUIDE.MDB File: Viewghanasmc</p><p>Variable Description Code Description of code</p><p>1 Treated BEDNET Group allocation 2 Not treated FOLLYR Child/years follow-up</p><p>Project: EPIGUIDE.MDB File: ViewGhanasm Variable Description Code Description of code</p><p>ID Identification of the participant 1 Treated BEDNET Group allocation 2 Not treated 0 No MAL Death from malaria 1 Yes Death from acute respiratory 0 No ARI infection 1 Yes 0 No GASTR Death from gastro-enteritis 1 Yes 0 No ACCIDENT Death from accident 1 Yes 0 No OTHER Other known cause of death 1 Yes Undetermined / missing cause of 0 No UNDETMIS death 1 Yes</p><p>139 Cohort studies</p><p>Project: EPIGUIDE.MDB Dataset: ViewIsfahan</p><p>Variable Description Code Description of code F Female SEX Sex M Male AGE Age in completed years 5-72 RESPPD PPD reactivity 0-15.0 0 Absence 1 Mild PAIN1 Local pain at day 1 2 Moderate 3 Severe 0 Absence 1 Mild INDUR1 Induration at day 1 2 Moderate 3 Severe 0 Absence ULCER1 Ulcer at day 1 1 Mild 2 Moderate 0 Absence 1 Mild ITCH1 Itching at day 1 2 Moderate 3 Severe 0 Absence LYMPH1 Lymphadenopathy at day 1 1 Mild 0 Absence 1 Mild PAIN7 Local pain at day 7 2 Moderate 3 Severe 0 Absence 1 Mild INDUR7 Induration at day 7 2 Moderate 3 Severe 0 Absence 1 Mild ULCER7 Ulcer at day 7 2 Moderate 3 Severe 0 Absence 1 Mild ITCH7 Itching at day 7 2 Moderate 3 Severe 0 Absence LYMPH7 Lymphadenopathy at day 7 1 Mild</p><p>140 Cohort studies</p><p>0 Absence PAIN30 Local pain at day 30 1 Mild 2 Moderate 0 Absence 1 Mild INDUR30 Induration at day 30 2 Moderate 3 Severe 0 Absence 1 Mild ULCER30 Ulcer at day 30 2 Moderate 3 Severe 0 Absence ITCH30 Itching at day 30 1 Mild 2 Moderate 0 Absence LYMPH30 Lymphadenopathy at day 30 1 Mild 0 Absence PAIN80 Local pain at day 80 1 Mild 2 Moderate 0 Absence 1 Mild INDUR80 Induration at day 80 2 Moderate 3 Severe 0 Absence ULCER80 Ulcer at day 80 1 Mild 2 Moderate 0 Absence ITCH80 Itching at day 80 1 Mild 2 Moderate 0 Absence LYMPH80 Lymphadenopathy at day 80 1 Mild 2 Moderate LST80 Leishmanin skin test at day 80 0.0-25.0 LST1Y Leishmanin skin test at 1 year 0.0-25.0 1 Yes LEISH Case identification 2 No 1 Yes LEISHX Primary case? 2 No 1 First year YDIAG Year of diagnosis 2 Second year 3 No case 1 ALM+BCG GROUP Vaccine allocation group 2 BCG</p><p>141 Cohort studies</p><p>Project: Epiguide.MDB File: ViewNEONATALD</p><p>Variable Description Code Description of code</p><p>ID Identification number</p><p>AGEM Mother’s age (years) 1 No MFETAL Multifetal pregnancy 2 Yes 1 >=7 2 4-6 PNVISITS Number of prenatal visits 3 1-3 4 None 1 Cesarean section DELIVERY Type of delivery 2 Vaginal 1 Term (>=37 weeks) GESTAGE Gestational age 2 Preterm (<37 weeks) 1 Married MSTATUS Marital Status 2 Not married 1 Private HEALTHI Type of health insurance 2 Public Health System 1 Female SEX Neonatal sex 2 Male WEIGHT Birth weight (grams) 1 7-10 APGAR5 5 minutes Apgar score 2 4-6 3 0-3 Yes DEATH Death No DAYS Time of death (days)</p><p>142 Cohort studies</p><p>Project: Epiguide.MDB File: ViewStaphylo</p><p>Variables Description Codes Codes description</p><p>ID Identification number 1 Female SEX Sex 2 Male 1 < 60 AGEGR Age group (years) 2 > = 60 Yes HOSPINF Hospital acquired infection No Yes BACP Primary bacteraemia No Meticillin-resistant Staphylococcus Yes MRSA aureus No Yes IMMUNOT Immunosuppressant therapy No 1 <= 10 days CVCSTAY Length of CVC stay 2 > 10 days ANTIMICR 1 Adequate Initial antimicrobial therapy OB 2 Inadequate DAYS Time in days until death 1 Fever/Sepsis CLINICAL Clinical status 2 Severe sepsis / Shock Yes DEATH Death No Yes DEATHB Death due to S. aureus bacteraemia No</p><p>143</p>
Details
-
File Typepdf
-
Upload Time-
-
Content LanguagesEnglish
-
Upload UserAnonymous/Not logged-in
-
File Pages50 Page
-
File Size-