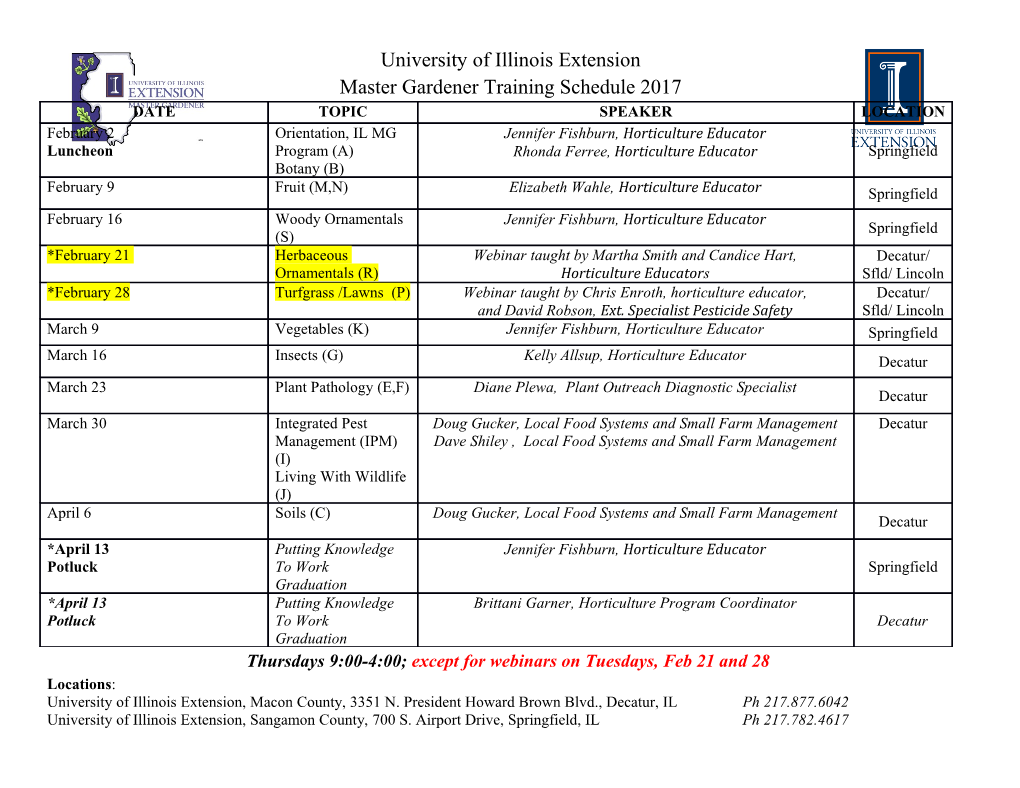
<p> ARABIAN PENINSULA SAND STORMS REGIONALISATION AND TRENDS ANALYSIS</p><p>1 Arabian Peninsula wet season dust storm distribution: regionalisation 2 and trends analysis (1983-2013) 3 4 Meshari Alobaidi a*, Mansour Almazroui a, Abdulwahab Mashat a, Philip Douglas Jones a,b 5 6 a Center of Excellence for Climate Change Research/Department of Meteorology, King Abdulaziz University, Jeddah, Saudi Arabia 7 b Climatic Research Unit, School of Environmental Sciences, University of East Anglia, Norwich, UK. 8 9 *Corresponding Author: E-mail Address: [email protected] 10 11 12 Abstract 13 14 15 A dust storm climatic regionalisation of the Arabian Peninsula (AP) that aims to categorise the region into 16 several cohesive sub regions is conducted. This approach was carried out by grouping together similar wet 17 season climatological features of Dust Storm Activity (DSA). A common period of 30 years (1983-2013) for 38 18 meteorological weather stations across the AP was utilised. The mathematical, statistical and subjective 19 methods that are employed in the process of regionalisation resulted in dividing the region into three sub 20 regions. Each of the sub regions North, North Western and Central (NWC), North East (NE) and South, West 21 and Coastal (SWC) shows distinct features of its own. Subsequently, the temporal trend of the representative 22 station (Key Station) for each sub region is examined. It is found that only the Northeastern part of the region 23 has a significant positive trend over the last 30 years. However, this positive increase in DSA is not associated 24 with an increase in wind speed. This study assists future research in exploring the relationship between large 25 scale forcing and the AP sub regional DSA. As well, this could enhance regional DSA forecast and future 26 projections. 27 28 KEYWORDS Arabian Peninsula; Wet Season; Dust Storms; Regionalisation; Trends Analysis 29 30 1. Introduction 31 32 The Arabian Peninsula is located in the south west of Asia and to the north east of Africa. It is surrounded 33 by the Arabian Gulf to the east, Arabian Sea to the south and the Red Sea to the west. The total area of the 34 peninsula is approximately 3,000,000 square kilometres. Desert is the most prominent feature in the peninsula, 35 however, mountain ranges are the prominent feature in the southwestern corner. Due to the location of the 36 peninsula, which is bounded mostly from all directions by local and distant dust sources, most populated areas 37 in the region are severely affected by dust storms (Prospero et al., 2002). Thus, any change in dust storm 38 frequency could lead to significant impacts on the economy, environment, ecosystem, human beings and the 39 global radiation budget (Natsagdorj et al., 2003). 40 The visibility could be severely reduced during an outbreak of dust storms. Kutiel and Furman (2003) have 41 categorised dust activities based on the reduction in horizontal visibilities: the term raising/blowing dust is used 42 when the horizontal visibility is less than 11 km, a dust storm is desiganted when the horizontal visibility is less 43 than 1 km and a severe dust storm when the horizontal visibility is less than 200 m. 44 The mechanisms that favour the generation of dust storms in the AP vary with season. In the wet season 45 (October - May) dust storms are caused by prefrontal and postfrontal winds that are associated with eastward 46 travelling depressions from the Mediterranean Sea. However, in summer, the persistent northerly and north 47 westerly winds induced by the Indian monsoon low pressure system are the main cause (Prospero et al., 2002; 48 Taghavi and Asadi, 2008; Hamidi et al., 2012; Alharbi et al., 2014). 49 Due to the lack of AP climate understanding, the AP has become an area of interest for many researchers. 50 Many climate studies are conducted for the region for different climatic fields and one of the most interesting 51 fields is forecasting dust storm activity. However, to do so, the characteristics and behaviour of DSA needs to be 52 well understood. In an early attempt, Middleton (1986) has regionalised the Middle East area based on the 53 season that has the highest dust activity. However, the data used was short in duration (1950 – 1960) and 54 covered varying lengths between stations. Later, Kutiel and Furman (2003) have spatially delimited the region 55 based on the temporal behaviour of dust activity. The data used here was monthly time series for 21 years record 56 (1973 – 1993), constructed based on the reduction in horizontal visibility to below 11 km. Notaro et al. (2013) 57 have utilised eight years (2005 – 2012) of daily deep blue Aerosol Optical Depth (AOD) measurements at 550 58 nm to regionalize dust activity across Saudi Arabia, based on the source regoins. They applied cluster analysis to 59 backward trajectories, which led to the identification of the primary dust sources for each station/area within 1 M. ALOBAIDI et al.</p><p>60 Saudi Arabia. Yu et al. (2013) found a reasonable consistency between dust surface observations, remotely 61 sensed AOD obtained from Multiangle Imaging SpectroRadiometer (MISR) and Moderate Resolution Imaging 62 Spectroradiometer (MODIS) as well as surface measurements of AOD measured from the Aerosol Robotic 63 Network (AERONET). This was achieved by utilising 10 year record of data for remotely sensed and surface 64 measured AOD to examine the seasonal cycle of dust activity across Saudi Arabia. Yu et al. (2015) also used 65 empirical orthogonal function (EOF) to study the spatial mode of dust variability across Saudi Arabia to identify 66 the climatic controls on Saudi Arabian dust activities. As well, they have identified a positive trend in dust 67 activity across the Arabian Peninsula since 2000. 68 69 Climate regionalization is a useful technique which enables researchers to discriminate climate variables of 70 a region into sub regions based on similar long-term seasonal variability (Kutzbach, 1967; Richman and Lamb, 71 1985, 1987; Ogallo, 1989; Eklundh and Pilesjö, 1990; White et al., 1991; Bunkers et al., 1996; Cahalan et al., 72 1996). Therefore, dividing the spatial continuum of a dust storm climatology into a manageable number of 73 quasi-homogeneous areas makes the process of temporal trend analysis and correlations with climatic large- 74 scale forcings more convenient (Comrie & Glenn 1998). 75 Such techniques could be automated (objective) or manual (subjective). The automated approaches involve 76 the application of mathematical and statistical tools (respectively, Principal Components Analysis and 77 Correlations). On the other hand, manual approaches involve some form of human intervention that has to be 78 conducted within the process of regionalization. No method is totally objective, as the subjective choice of the 79 number of components retained has to be made. Additionally, other decisions made are likely based on prior 80 knowledge of the region (Virmani 1980, Yarnal 1993; Almazroui et al. 2014a and 2015). 81 In this research, Principal Components Analysis (PCA) and correlations of surface observations of dust 82 storms are used to identify the patterns within DSA that have similar spatial characteristics during the wet 83 season. As well as the application of regionalization, subjective methods are also utilised. Thereafter, the 84 temporal trends of the resulted dust storm sub-regional patterns are investigated. This study offers new insights 85 into dust storm patterns and their behaviour during the wet season in the region. 86 This research differs from the analysis of Kutiel and Furman (2003) and Yu et al (2015) in four ways: (a) 87 dust storm time series for each surface station are constructed based on horizontal visibility reduction to below 1 88 km, while considering the minimum wind speed needed to lift dust for each respective area, (b) the application 89 of objective and subjective climate regionalization, (c) analysis is implemented on a common period that spans 90 the 30 years (1983 – 2013) and (d) DSA characteristics and behaviour are only examined during the wet season. 91 92 93 2. Data 94 95 Dust storms are phenomena that cannot be directly measured like other meteorological variables such as 96 precipitation, pressure and temperature. Their occurrence can be observed or in remote areas can be remotely 97 sensed or extrapolated from other climatic variables like visibility and wind speed. 98 Satellite data could provide valuable information about the occurrence of dust storms, especially with 99 advanced remote sensing instruments like the Ozone Monitoring Instrument. Yet, these new instruments only 100 provide a short record of data and thus will not fulfil the objective of this study. However, Yu et al. (2013) 101 found a reasonable consistency between remotely sensed AOD and surface dust observations. Hence, remotely 102 sensed AOD was subjected to PCA to validate the reliability of this study before final regionalisation output. 103 Monthly remote sensed AOD data gridded with a 0.25° spatial resolution is used in this study. These data were 104 obtained from the National Aeronautics and Space Administration (NASA). The AOD data set is the level 2 105 AOD at 558 nm measured by MISR instrument (2000 – 2013). This AOD data set is quality assured and the 106 cloud contaminated pixels have been removed. However, not all AOD gridded pixels that cover the AP are 107 subjected to PCA; only the pixels values within ±0.25° of the location of each station are used (Yu et al., 2013). 108 Thus, only 38 of monthly AOD time series are used, where each covers the location of the selected stations. 109 This is because the results from performing PCA on 38 indices will definitely differ from the results obtained 110 from performing PCA on all 360 indices (MISR could cover the AP with at least 360 grid points), and this will 111 not assist in the validation process of the final results of this study. 112 DSA phenomena do not occur at some locations every day or every month. Yet during the course of a 113 season, almost all stations encounter this phenomenon. Therefore, it is more valuable and meaningful to analyse 114 DSA patterns on seasonal time scales. 115 The data used in this study were collected from 38 meteorological weather stations which comprise a record 116 of hourly observations of wind speed and visibility (figure 1 and table 1). However, the number of stations 117 involved is relatively low when considering the overall area of the AP. In addition to this, the Empty Quarter 118 desert (EQ) has no meteorological weather station. This is because this part of the region is not populated by</p><p>2 ARABIAN PENINSULA SAND STORMS REGIONALISATION AND TRENDS ANALYSIS</p><p>119 human beings and is very far away from the nearest populated area. Consequently, no data record is available 120 for this area (figure 1). 121 To meet the recommended period for long-term climate assessments, a record of 30-year is selected for all 122 stations (WMO, 2011). A common period (1983-2013) has been used to avoid the bias that could be caused by 123 inter-annual and decadal fluctuations when using different periods between stations (Almazroui et al., 2014a). 124 As well, this assists in capturing the possible influence of major synoptic circulation pattern on the resulted dust 125 storm sub-regions in follow up studies. 126 This study has adopted a strict role in station selection at earlier stages, only stations that have at least 75% 127 data presence are selected. However, due to the low station density in the AP and for better dust storm spatial 128 analysis, stations that have more than 50% of complete data were included. This resulted in having 85% of 129 selected stations having more than 80% of data presence and 15% having between 52% and 72.5% of data 130 presence (table 1). 131 Currently many data sources are available for researchers to retrieve climate data. However, 132 NOAA/National Climatic Data Center (NCDC) strictly applies rigorous quality checking procedures to their 133 archived global data (WMO, 2008). This is considered a precautionary procedure to avoid any possible data 134 issues that could occur at local meteorological offices and to assure the quality of the archived data. Therefore, 135 NCDC climate data are the optimal choice for this research. 136 Normally climate data providess the user with a report that summarizes the daily weather (WMO, 1988). 137 However, due to the lack of awareness in the AP region of the importance of climate data before 2001, the daily 138 summary reports that normally provide the researcher with the occurrence of any special weather phenomenon 139 like haze, dust and fog are mostly missing and inaccurate for most of the stations. 140 To overcome this major obstacle a 30-year period of daily dust storm activity for each surface station was 141 constructed. In order to achieve this, we adopted the Kutiel and Furman (2003) definition of a dust storm and 142 severe dust storm, which is a reduction in horizontal visibility to below 1 km. As well, we adopted Clements et 143 al. (1963) definition for the minimum wind speed needed to suspend dust in the air based on different soil types. 144 The AP regional soil type is comprehensively studied by Goudie and Middleton (2001), Washington et al. 145 (2003) and UCAR/COMET (2010) (see their Appendix A for the soil type and wind threshold needed to 146 suspend dust for each location). Therefore, a dusty day is considered when a visibility of lower than 1 km occurs 147 with the minimum wind speed required to suspend dust for that respective location at least once during the day. 148 149 150 151 3. Methodology 152 153 Several studies have indicated that dust activities across the AP have increased with time. Chin et al. (2014) 154 revealed a slight increase in AOD across the Middle East since 1980 and they attributed this increase to the 155 increase in surface wind speeds. As well, Notaro et al. (2015) found positive trends in northern of AP dust 156 activity since 2000, which was attributed to the persistent drought across the Fertile Crescent. In addition to this, 157 Yu et al. (2015) found a positive trend in dust activity since 2000 over the northern of the AP. However, this 158 increase in dust activity is believed to be part of a distinct decadal variability in Saudi Arabian dust activity. To 159 enhance the understanding of the behaviour of DSA in the Peninsula, this research willinvestigate and analyse 160 longer-term trends of DSA across the AP. 161 To produce dust storm time series for the wet season, monthly total numbers of dusty days (defined above) 162 are summed for each month at each station. After monthly time series for each station are constructed, wet 163 season time series are created by summing all dusty days between wet season months from October to May for 164 each year. Hence, each station has a single value for the wet season each year, which gives us a total of 30 165 values for each station. By doing this, the effect of seasonality is minimised. This is because the behaviour and 166 causes of dust storms in the AP in the wet season are completely different from the dry season (Prospero et al., 167 2002; Taghavi and Asadi, 2008; Hamidi et al., 2012; Alharbi et al., 2014) (all stations wet season DSA time 168 series are in Appendix A). Then, a matrix of all stations wet season DSA activity is constructed, which is then 169 utilised in the regionalisation process. We refer to this overall activity as an index. 170 . 171 172 3.1. Wet season DSA distribution (Climatology) 173 174 Climate is recognised as the average, total or variability of a certain variable over a period of time. The WMO 175 considers the period of 30 years to be the minimum period needed to define the climatology of a variable. To 176 define a wet season climatology for DSA across the AP, the total number of dusty days for each station is 177 calculated and plotted spatially. Boyles and Raman (2003) postulated that when stations are unevenly spaced in 3 M. ALOBAIDI et al.</p><p>178 a domain, Kriging interpolation technique would be more appropriate to be used for spatial analysis of a 179 variable. Thus, this technique is applied in this research for DSA spatial analysis as the stations in the selected 180 domain are unevenly spaced. This is undertaken mainly to help with the mapping of the results across the AP. 181 3.2. Climate Regionalization based on Dust storms frequencies 182 183 PCA is a technique used to reduce a large dataset into a manageable number of components (Jollife, 184 1993; Tabachnick and Fidell, 2007; Almazroui et al. 2015). This assists in identifying spatially cohesive 185 dust storm regions based on seasonal pattern similarity and variability (figure 2 for PCA steps flow chart). 186 There are two types of PCA, T-Mode and S-Mode. The T-Mode PCA is used to correlate between 187 different fields, on the other hand, S-Mode PCA is used to correlate between different temporal series. The 188 data matrix is constructed with multiple stations over time, so S-mode PCA is applied in the current 189 analysis (Comrie and Glenn, 1998). This study performs the PCA using a covariance matrix, as it is 190 conducted on a single variable that has one scale (Fung, 1995). In addition to this, PCA performed using a 191 correlation matrix generates standardized data; hence distinguishing stations with relatively low dust storm 192 frequency from others would likely be unachievable and chances of having a group that represents such 193 stations would be low. We want to be able to distinguish regions with high and low levels of DSA as well 194 as common interannual variability. 195 Several steps are conducted prior to finalizing the dust storm sub-regions. The first step is determining 196 the number of retained Principal Components (PCs). There are many methods to determine the number of 197 PCs to retain and selecting the proper process is crucial. This is because extracting too few components 198 might result in missing valuable common variance and extracting too many components might results in 199 having undesirable error variance (Jolliffe, 1993; Costello and Osborne, 2005; Tabachnick and Fidell, 200 2007). As a result, the appropriate number of sub regions will not be accurate. 201 The first method for extracting the number of PCs to retain are Kaiser’s Criterion and Jolliffe’s 202 Criterion, both being considered rules of thumb to determine the correct number of PCs. Both criteria 203 suggest retaining components that have eigenvalues of 1.0 and 0.7 respectively (Kaiser, 1960; Jolliffe, 204 1886). However, both criteria could substantially overestimate the number of PCs extracted (Costello and 205 Osborne, 2005; Fidell, 2009). Another approach is extracting PCs from a variance explained threshold, one 206 can keep components that account for 90% or to a lesser extent 70% of variance (Jolliffe, 2002; Stevens, 207 2002; Almazroui et al. 2014a). The fourth approach is the mean eigenvalue rule, this rule utilizes 208 components that score more than or equal to the mean eigenvalue. This method is regarded to be strict as it 209 potentially underestimates the actual number of PCs that have to be retained. The Scree curve plot test is 210 another approach used to extract PCs. The elbow of the curve (point of inflexion) is the determinant for the 211 number PCs to retain, all components that are above the point of inflexion are retained. However, this 212 method is only reliable when the sample size is not less than 200 (Cattell, 1978; Gorsuch, 1983; Wilks, 213 2006). . Parallel Analysis (PA) is considered as the most accurate approach in extracting the proper number 214 of PCs (Fabrigar et al., 1999; Hayton et al, 2004; Henson and Roberts, 2006). This technique generates 215 eigenvalues from an artificial data set. Then, the original and artificial eigenvalues are compared with each 216 other, for example eigenvalue artificial of PC6 artificial compared with eigenvalue original of PC6 original and so on. 217 Only PCs that have eigenvalues greater than or equal to the artificial eigenvalues are retained (Hayton et al, 218 2004; Watkins, 2008). In this research, all above methods are implemented to guarantee the proper 219 extraction of PCs. 220 While extracting the appropriate number of PCs, it is essential to remove all stations that have weak 221 loading scores (< 0.5). Retaining such stations canlead to having unstable structure at the final PCA result. 222 This is achieved by running PCA (Unrotated) on all stations, and stations that are not loaded on to any of 223 the major components with sufficient large component loadings ( in this study) are removed (Henson and 224 Roberts, 2006). These stations are considered unclassified. Thus, the final number of retained PCs is 225 determined only on stations that have sufficient component loadings. Thereafter, stations that loaded 226 significantly are assigned to a PC according to its highest loading score.. Hence, each station is only 227 assigned to one PC. 228 Then, rotation is performed to attain the best simple structure, which assists in having each station 229 significantly loaded on only one component (Gorsuch, 1983; Comery and Lee, 1992). As well, this 230 procedure supports the identification of key stations within each sub region. 231 Two major rotational techniques are available for this study, Orthogonal and Oblique (Tabachnick and 232 Fidell, 2007). Orthogonal rotations (Quartimax, Varimax and Equamax) are when components will not to 233 be correlated with each other (perpendicular to each other or rotated 90 from each other) (Rummel, 1970; 234 Decoster, 1998). Quartimax rotation is used to minimize the number of components required to explain the 235 variable in use (Gorsuch, 1983). Varimax rotation means that each component has a small number of large 236 loadings and a large number of small loadings and this assists in the process of interpretation if the results 237 due to the high values of explained variance (Yong and Pearce, 2013). Equamax rotation is a combination 4 ARABIAN PENINSULA SAND STORMS REGIONALISATION AND TRENDS ANALYSIS</p><p>238 of Quartimax and Varimax (Tabachnick and Fidell, 2007). In contrast, Oblique rotations (Direct Oblimin 239 and Promax) are when components can be correlated with each other (not rotated 90 from each other). 240 Direct Oblimin simplifies the structure and the mathematics of the outputs, hence, is easier to interpret. In 241 Promax rotation, the simple structure is achieved by raising the loadings to the power of four and this 242 increases the correlations between components (Gorsuch, 1983; Yong and Pearce, 2013). It is 243 recommended by White et al. (1991) that the use of Oblique rotations for climate regionalization, as it 244 produced stable results when compared with orthogonal types. 245 This study implements all types of rotations mentioned above and then follows the recommendations of 246 Tabachnick and Fidell (2007)on selecting the best type of rotation. This is achieved by analysing the 247 resulting component correlation matrix (for each of the Oblique rotations) and component transformation 248 matrix (for each of the orthogonal rotations). 249 After applying rotation on the extracted PCs, the highest positive scores within each component is 250 determined using a key station for that component. This assists in having a single cohesive region with one 251 clear centre (e.g. Wigley et al., 1984; Almazroui et al. 2014a). As well, key stations could be used as 252 representatives for their respective sub regions and correlated with global climate forcing series. 253 Thereafter, key stations are correlated with all unclassified stations and the results of the correlations 254 assists in assigning unclassified stations to their appropriate sub region. However, if any of the unclassified 255 stations fails to correlate significantly with any of the key stations, these stations will be assigned to sub 256 groups subjectively. This is achieved by locating such stations within the same sub region of the closest 257 regionalised station. This process is influenced by the geographical location and prior knowledge of the 258 region. 259 The outcomes of regionalisation should present similarity between stations DSA patterns within each 260 sub region, meanwhile, the arithmetic averages of each sub region should not be similar. Therefore, for 261 evaluating the outcomes of the regionalization process, stations DSA frequencies are plotted against each 262 other for each sub region. This assists in determining the pattern consistency between stations within each 263 sub region, and thus, the outcomes of regionalisation. As well, the arithmetic averages for each sub region 264 are plotted against each other, assisting in and determining the dissimilarity between sub regions. After 265 finalising the final sub regions based on surface observations, the same steps will be conducted on the AOD 266 data obtained from MISR. This step is to validate the results of the findings of this research. 267 268 269 3.3. Trend analysis 270 271 There are several trend analysis techniques that could be applied for this study, such as linear trend 272 analysis and complex trends analysis. Complex trend analysis techniques are not considered suitable for this 273 research. This is because such techniques could be applied differently between different locations (Boyles 274 and Raman, 2003). The equations used in complex trend analysis techniques are allowed to change from 275 one station to another to obtain the best fit between the curve and data. For example, when two stations 276 have a slope value of 0.5, it does not mean that both slopes are equal in the magnitude, as both have been 277 calculated from different best-fit equations. Even more, each equation is modified for its particular time 278 series (different formulas for different time series). Hence, stations cannot be compared between each other 279 for spatial analysis, as the slope magnitude scale is uneven between different locations. In contrast, linear 280 trends use a common formula for all locations/stations, thus, the slope magnitude scale is the same for all 281 locations/stations (Boyles and Raman, 2003). 282 In this study, to detect any changes in DSA and wind speeds that might have occurred for each sub 283 region, slopes of linear trends are fit to the standardised time series (fitting a straight line using the least 284 square method). Trend analysis is performed for several time windows; the entire 30 years, the first and 285 second halves of the time series (1983 – 1998 and 1999 – 2013 respectively) and division into three periods 286 where each part covers 10 years (from 1983 to 1992, 1993 to 2002 and 2002 to 2013). 287 Linear trends are considered the best choice available to compare changes between different stations 288 across a region over a common period (Boyles and Raman, 2003). Consequently, this study only 289 implements this simple statistical method on DSA time series of the key stations (which are considered 290 representative of each sub regions). 291 292 4. Results and discussion 293 294 4.1. Regional Dust Strom Climatology 295</p><p>5 M. ALOBAIDI et al.</p><p>296 The entire region is effected by dust storms as shown in figure 3. However, the spatial distribution 297 varies significantly over the domain with the northern and north eastern parts of AP having the highest 298 frequency of occurrence during the wet season. However, the frequency of events gradually decreases 299 towards the western and southern coastal areas where dust storms activities are considerably lower. This is 300 because both areas are bounded by mountain ranges to the east and north respectively. These mountain 301 ranges act as a barrier that isolates both coastal areas from the main DSA areas of AP (Washington et al., 302 2003). Most of the dust storms that strike the western coasts of the AP come across the Red Sea from Africa 303 (Taghavi and Asadi, 2008; Notaro et al., 2013). 304 The topographic features of the area play a crucial role in the evolvement of this spatial distribution. 305 This is because the Arabian plateau is flat in the northeast and east and higher elsewhere. In addition to this, 306 the existence of mountain ranges east of the Arabian Gulf force winds to be funneled over the northeastern 307 and eastern parts of the region from a northwesterly direction most of the year (Prospero et al., 2002). Dust 308 from the erodible alluvial plain of the Tigris and Euphrates basins, which are major dust sources for the 309 region is transported to the northeastern and eastern parts of the AP. Thus, a higher frequency of DSA is 310 expected over those parts of the region (Prospero et al., 2002; Washington et al., 2003; Hamidi et al., 2013). 311</p><p>312 4.2. Climate regionalization 313 314 Unrotated PCA has been applied to the wet season dust storm indices covariance matrix. In order to 315 obtain the appropriate number of PCs that corresponds with the recommended percentage of the total 316 variance explained, the Scree plot is examined The initial results suggest that PCs between PC4 and PC8 317 overlap and this gives an indication to the possibility of retaining only four PCs (not shown). 318 Kaiser’s criterion and Jolliffe’s criterion are then applied to confirm the results of the Scree test. In this 319 analysis both methods severely overestimated the number of PCs to retain. Both respectively suggested 27 320 and 28, particularly considering that the maximum number is 38, so both criteria are rejected 321 While applying PA following recommendations of Watkins (2008), it is observed that PA as well over 322 estimated the true number of PCs. The PA 1000 simulations suggested retaining 19 PCs; hence, this method 323 was rejected. 324 On the other hand, the mean eigenvalue score analysis indicates only four components are greater than 325 the mean eigenvalue. Hence, this method concurs with result of the Scree test. Therefore, four PCs are 326 retained and considered to be the preliminary number of retained PCs. The total variance explained for the 327 four selected components is 70.4% and this considered to be an acceptable percentage. 328 While examining the stability of each of the extracted PCs, it was found that the fourth PC was 329 unstable and weak as it has less than three stations that are substantially loaded onto it. As a result, PC4 is 330 removed following the recommendations of Tabachnick and Fidell (2007). Consequently, the extracted 331 number of PCs is three and these explain 70.4% of the total variance. PC1 contributes 48.6%, PC2 332 contributes 13.7% and PC3 contributes 8.1%. The resulting number of stations that are used in the final 333 PCA run is 20; the remainder of stations that unclassified. Stations that are significant loaded to a PC are 334 assigned according to their highest loading score (see table 2). 335 Subsequently, rotations are applied to the extracted four PCs and on the final pool of stations (20). 336 Scores obtained from all methods of rotation were spatially plotted to investigate any significant 337 differences. It was found that the spatial distributions of loading patterns for each rotation method are very 338 similar to each other for all PCs. Hence, all plots are almost the same for each PC for all rotation methods 339 (not shown). Therefore, the application of any rotation method does not significantly alter the results. 340 However, for optimizing the application of rotated PCA, Tabachnick and Fidell (2007) 341 recommendations on the selection of the appropriate rotation method are considered. It is found that 342 Promax rotation provided the simplest structure for interpretation, and thus, it is the suitable rotation 343 method for this analysis. After the application of Promax rotation, the total explained variance barely 344 changed and stations loaded on the same components as shown earlier in table 2. The Promax rotated 345 loading patterns for the resulting three PCs are shown in figure 4 witheach of the three PCs having its core 346 centred in a different location. The resulting scores indicate that the key stations for each PC (sub region) 347 are Rafha (PC1), Alhasa (PC2) and Jeddah (PC3). Thus, our PCA-based preliminary regionalization is 348 established and each key station is considered to represent its own sub region (figure 5). 349 In order to assign unclassified stations, the correlation coefficients between key stations and all 350 unclassified stations are examined (see correlation coefficients Table Appendix A). It is found that the 351 unclassified stations significantly correlated (at 95% confidence interval) with at least one key station 352 except Abha, Damascus, Dubai and Gizan (Station ID 38, 16, 24 and 32).</p><p>6 ARABIAN PENINSULA SAND STORMS REGIONALISATION AND TRENDS ANALYSIS</p><p>353 Albaha, Altaif are significantly correlated with all key stations. However, both had their highest 354 correlations with Jeddah (PC3), so they are placed with PC3. As well, Almadinah, Alwejh, Khamis 355 Mushait, Makkah, Ras Alkhamah, Salalah, Seiyon and Sharjah are placed with PC3 due to their significant 356 correlations with the key station Jeddah (PC3). Amman is placed with PC1 due to its significant correlation 357 with key station Rafha (PC1). Cairo has significantly correlated with key station Alhasa (PC1), however, 358 due to its geographical location it is subjectively placed with PC1. Because of their geographical locations, 359 Sanaa and Sur are placed subjectively with PC3 in spite of their significant correlations with key station 360 Rafha (PC1). The four stations that failed to correlate significantly with any group are assigned 361 subjectively as follows; Abha, Dubai and Gizan are located in PC3 sub region due to their geographical 362 location. Damascus is subjectively placed in PC1 sub region due to its geographical location. 363 After assigning all 38 stations mathematically (PCA), statistically (Correlations) or subjectively 364 (regional knowledge), the final dust storm climatic sub regions are shown in (figure 6) and have been given 365 new names according to their geographical location. . PC1 is North, North Western and Central of AP 366 (NWC), PC2 is North East (NE), and PC3 is South, West and Coastal areas of AP (SWC). 367 Subsequently, the outcome of regionalization is examined by comparing between the three arithmetic 368 averages of the sub regions. As well, the main DSA characteristics for each sub region are depicted from 369 this comparison (Almazroui et al., 2014a) (figure 7 and table 3). It is found between October and January, 370 the DSA behaviour for all sub regions are not significantly different from each other. However, the SWC 371 sub region starts to be substantially different and lower in DSA than the arithmetic average, and for NE and 372 NWC after January. Meanwhile, NE and NWC sub regions have similar DSA characteristics throughout the 373 season. Except in April where the NE sub region is significantly different and lower from (NWC) sub 374 region and the arithmetic average. Contrary, in April the NWC sub region is significantly greater than the 375 arithmetic average. 376 The results of the regionalisation based on the AOD data obtained from MISR show very similar 377 distribution patterns to the findings of this study (see Appendix B for distribution pattern plots and 378 correlations between MISR AOD & observations at each location). Therefore, the constructed 30 years 379 DSA indices and the results of regionalisation based on surface observations are robust as they are 380 consistent with the findings of an independent data set (MISR AOD data). 381 382 383 4.3. Regional trend analysis 384 385 The trend analysis results reveal that DSA for the NWC sub region has not significantly changed 386 during the 30 years. However, DSA in the NE sub region has significantly increased over the past 30 years 387 (+0.0028 at 95% confidence interval). However, the wind speed has significantly decreased for the NE sub 388 region during the same period. For the SWC sub region a significant decrease in DSA is observed (-0.0022 389 at 95% confidence interval). However, no significant wind speed trends are observed for this sub region 390 during the 30 years period. Hence, significant changes in DSA in these two sub regions (NE and SWC) 391 could not be linked to changes in wind speeds between 1983 and 2013. 392 During the first 15 years of the time series, DSA significantly decreased in the SWC sub region (- 393 0.0094 at 95% confidence interval), but there were no other significant trends observed in the other sub 394 regions. In contrast, for this period, winds speed has significantly increased in the SWC sub region (+0.006 395 at 95% confidence interval). Thus, the DSA decrease can not be associated to changes in wind speeds. 396 DSA in the other two sub regions (NWC and NE) has significantly increased during the second 15 397 years period (respectively, +0.006 and +0.011 at 95% confidence interval), butwind speed trends reveal that 398 only wind speeds in the NE sub region have significantly increased (+0.0156 at 95% confidence interval). 399 Thus, the increase in DSA for this sub region could possibly be attributed to the increase in wind speeds. 400 On the other hand, the DSA trend in the NWC sub region cannot be linked to increases in winds speeds. 401 The only DSA significant trend observed during the first 10 years (1983 -1992) is an increase in the 402 NWC sub region (+0.012 at 95% confidence interval). Yet, this increase could not be attributed to increases 403 in wind speeds as no wind speed significant trend is observed for this period. 404 For all sub regions, no DSA significant trends were found during the second 10 years period (1993 – 405 2002). Trends analysis for the last 10 years period (2003 – 2012) shows that DSA has only significantly 406 increased in the NE sub region (+0.013 at 95% confidence interval). As well, wind speed trends analysis 407 reveals a significant increase in wind speed for this period (+0.0096 at 95% confidence interval). 408 Consequently, the DSA increase in this sub region could possibly be associated with increases in wind 409 speeds (see Appendix A for DSA and wind speeds linear slopes analysis). 410 4.4. Discussion 411 7 M. ALOBAIDI et al.</p><p>412 The DSA seasonal fluctuations, shown in figures 7 display the characteristics based on averaged 413 monthly DSA events for each sub region. The NWC sub region which covers the largest area of the AP is 414 characterised by a steady increase in DSA as the wet season progresses, until it reaches its maximum in 415 April then starts to decline in May. This is consistent with the finding of Yu et al. (2013). The maximum 416 DSA is 41 events (95% confidence interval of 2.9, 8.4), which is significantly greater than the regional 417 average for the NE and SWCsub regions, respectively by (25%, 55% and 80%) for the same month. The 418 SWC sub region has similar characteristics to the regional average; however, their magnitudes are 419 significantly distinct from each other in April. Meanwhile, the characteristics of the NWC sub region are 420 similar to the NE sub region, except in April. On the other hand, the NWC sub region is distinctly different 421 in magnitude and characteristics between (February and May) from the SWC sub region. 422 With respect to the differences between this study and Kutiel and Furman (2003) in the dust activity 423 selection criteria and the time of the season, Kutiel and Furman (2003) have identified this region to have 424 distinct characteristics from the remainder of their domain (named region “b” in their paper). In addition to 425 this, Yu et al. (2015) have found that this part of the region has distinct characteristics from the rest of their 426 domain. 427 The NE ub region covers the north eastern area of the region, this sub region has two peaks that occur 428 in March and May (respectively, 29 and 31 events, 95% confidence interval of 2.2, 5.9). Notaro et al. 429 (2013) postulated that dust activities in the north eastern part of Saudi Arabia (Alhasa station) peak in early 430 spring (March) and in the middle of the summer (June). This is observed for this sub region, DSA starts to 431 increase in May after the substantial reduction to 18 events in April (95% confidence interval of 2.2, 5.9), 432 which is 35% lesser than its March month value. Generally, the NE sub region has similar characteristics to 433 the regional average and the NWC sub region, except in April where it is substantially lower by 41% and 434 55% than the regional average and the NWC sub region respectively. 435 Both Kutiel and Furman (2003) and Yu et al. (2015) find that the north eastern and southwestern parts 436 of the region have the same characteristics. Thus, both are considered to be in one sub region. This conflicts 437 with our findings This could be due to the regionalisation technique adopted in this study, or from the 438 unique observation time series utilised in this study that assisted in delimiting this large part of the region 439 into two distinct sub regions. In addition to this, DSA in the north eastern part of the AP is substantially 440 different from the southwestern part (figure 3). Therefore, the separation between them that is implied by 441 the regionalisation process adopted in this research is robust. 442 The SWC sub region has the quietest DSA in the AP, as it is distinctly lower in magnitude than the rest 443 of the sub regions and the regional average. The DSA begins to be substantially different form the 444 remainder of the sub regions after January. Notaro et al. (2013) found that the peak month during our wet 445 season for this part of the region is February or Mach. As shown in figure 7, the maxima for the SWC sub 446 region occurs in March (12 events, 95% confidence interval of 0.01, 2.1), which is consistent with Notaro et 447 al. (2013). 448 Overall, all sub regions show a seasonal signal that corresponds well with the climatology of the 449 region. After December the polar jet shifts southward, which enhances the contrast in temperature between 450 northern and southern airmasses over the AP (Taghavi and Asadi, 2008). Consequently, the atmospheric 451 conditions become unstable for most of the season and AP becomes prone to the passage of frontal systems. 452 This instability continues and enhances through the wet season until May (Wilkerson, 1991 and Bartlett, 453 2004). Thus, DSA would be higher at the end of the wet season and this is consistent for all sub regions as 454 shown in figure 7. 455 The trend analysis finding of this study oppose the findings of Chin et al. (2014), which associated the 456 increase in dust activity in the Middle East to the increase in regional wind speeds. This is because the 457 significant changes in regional DSA are mostly not associated with changes in winds speeds. This is based 458 on trend analysis; where only two out of the seven significant changes in DSA (which counts for 28.5%) 459 could be linked to changes in wind speeds. In addition to this, several significant changes in wind speed in 460 all sub regions have not lead to significant changes in DSA. As well, on two occasions the increase in DSA 461 concurs with significant decreases in wind speeds. Therefore, this study might support either or both of the 462 findings of Notaro et al. (2015) and Yu et al. (2015). 463 The trend analysis of this study agrees with the finding of Notaro et al. (2015) and Yu et al (2015), 464 where both postulated a significant increase in dust activity in the northern and north eastern parts of the 465 region after 2000. DSA in the NE and NWC sub regions has significantly increased in the last 15 years of 466 the time series (1999 – 2013). 467 468 5. Conclusions 469 470 This study has determined climatic sub regions for the AP based on wet season variability of dust storms. The 471 regionalisation procedures conducted in this study employed mathematical, statistical and subjective methods. A 8 ARABIAN PENINSULA SAND STORMS REGIONALISATION AND TRENDS ANALYSIS</p><p>472 common period of 30 years of data for 38 stations was used to distinguish between dust storm sub regions of the 473 AP. Three sub regions were determined for the AP, the characteristics of the sub regions beginning to become 474 distinct from each other after February. As well, all sub regions reflected the seasonal signal of the regional 475 climate like depression passages that are strongly associated with regional DSA. In addition to this, the output of 476 the regionalisation process was verified with an independent data set (MISR AOD data). Both showed similar 477 regional pattern distribution. This indicates that the constructed DSA indices utilized in this study could be used 478 as representatives for historical regional dust events analysis. In addition to this, the validation process showed 479 the reliability of the remotely sensed AOD data, which could be used in the future when they provide a 30 year 480 data record.. 481 Subsequently, temporal trends for time series of key stations within each sub region are analysed. Only the 482 NE sub region showed significant positive DSA trend for the 30 year time series. However, this significant 483 trend is found not to be associated with changes in wind speeds. Some studies suggested that positive trends in 484 DSA could possibly be attributed to decadal variability in dust activity (Shao et al., 2013 and Yu et al., 2015), 485 while Notaro et al. (2015) attributed the significant trend in DSA to persistent drought across the Fertile 486 Crescent. However, another factor should be considered, which is mentioned in the Early Warning and 487 Assessment Technical Report of The United Nations Environment Program (UNEP Demise of an Ecosystem, 488 2001). The report reveals that the land use change in northern Iraq, southern Syria and southern Turkey, and the 489 construction of dams has severely affected the Mesopotamian Marshlands. This has led to significant lowering 490 of the river levels, which led to an abundance of alluvial deposits ready to be suspended by air. 491 Some limitations of this study should be accounted for. First, The small number of the available stations 492 that had a minimum of 30 years of data record, resulted in having a lower DSA spatial resolution of region. If all 493 the meteorological weather stations in the AP provided 30 years data records (450 stations are in the AP), the 494 DSA spatial resolution would have been significantly higher and provided more valuable information to this 495 study. In addition to this, the Empty Quarter desert has no stations that met the requirements for this study. As 496 well, this area is considered as a major dust/dust source that could significantly alter the results. However, this 497 issue should be resolved in the coming 15 years, as the remotely sensed AOD will provide a minimum data of 498 30 years. Second, a longer record of data would have provided a better knowledge of the regional DSA patterns 499 variations with time. 500 501 502 Acknowledgments The authors would like to acknowledge the Center of Excellence for Climate Change 503 Research (CECCR) and the Deanship of Graduate Studies of King Abdulaziz University for supporting this 504 work. The Met Offices are acknowledged for providing dust storms data and MODIS data are collected from its 505 website. Computation for the work described in this paper was performed using Aziz Supercomputer at King 506 Abdulaziz University’s High Performance Computing Center, Jeddah, Saudi Arabia. 507 508 509 References 510 511 Alharbi, B.H., Maghrabi, A., and Tapper, N., 2014: The March 2009 dust event in Saudi Arabia 512 precursor and supportive environment. Bull. Amer. Meteor. Soc., 94, 515–528. 513 Available online at [ doi: http://dx.doi.org/10.1175/BAMS-D-11-00118.1]. 514 515 Almazroui M, Ramzah Dambul, Islam MN, Jones PD., 2014a . Principal components-based regionalization of 516 the Saudi Arabian. climate. Int. J. Climatol. 35: 2555–2573 (2015) Available online at DOI: 10.1002/joc.4139 517 518 Almazroui, M., Awad, A., Islam, MN., Al-Khalaf, A., 2014b. A climatological study: wet season cyclone tracks 519 in the East Mediterranean region. Theoretical and Applied Climatology April 2015, Volume 120, Issue 1, pp 520 351-365. 521 522 Almazroui M, Ramzah Dambul, Islam MN, Jones PD., 2015. Atmospheric circulation patterns in the arab 523 region and its relationships with Saudi Arabian surface climate: A preliminary assessment. Atmospheric 524 research 161-162 (2015) 26-51. 525 526 Ammar, K., El-Metwally, M., Almazroui, M., and Abdel Wahab, M., 2013. A climatological analysis of 527 Saharan cyclones. Clim Dyn (2014) 43:483–501 [ DOI 10.1007/s00382-013-2025-0 ] 528</p><p>9 M. ALOBAIDI et al.</p><p>529 Bartlett, K.S., 2004: Dust Storm Forecasting For Al Udeid Airbase, Qatar: An Empirical Analysis. M.S. thesis, 530 Department Of The Air Force, Air University, 102 pp [ Available on line at 531 http://www.dtic.mil/dtic/tr/fulltext/u2/a422645.pdf ]. 532 533 Boyles, R.P. and Raman, S., 2003: Analysis of climate trends in North Carolina (1949 – 1998). Environment 534 International 29 (2003) 263 – 275. 535 Bunkers MJ, Miller JR, DeGaetano AT (1996) Definition of climate regions in the northern plains using an 536 objective cluster modification technique. J Clim 9:130–146 537 Cahalan RF, Wharton LE, Wu ML (1996) Empirical orthogonal functions of monthly precipitation and 538 temperature over the United States and homogeneous stochastic models. J Geophys Res 101(D21):26309–26318 539 Cattell, R.B. (1973). Factor analysis. Westport, CT: Greenwood Press. 540 Chin, M. et al. (2014), Multi-decadal aerosol variations from 1980-2009: a perspective from observations and a 541 global model, Atmos. Chem. Phys., 14, 3657-3690. 542 Costello, A.B., & Osborne, J.W. (2005). Best practices in exploratory factor analysis: Four recommendations for 543 getting the most from your analysis. Practical Assessment, Research and Evaluation, 10(7), 1-9. </p><p>544 Comrie, A. C. and Glenn. E. C., (1998) Principal components-based regionalization of precipitation regimes 545 across the southwest United States and northern Mexico, with an application to monsoon precipitation 546 variability CLIMATE RESEARCH 10: 201–215, 1998 547 Comrey, L.A. & Lee, H.B. (1992). A first course in factor analysis (2nd ed.). Hillside, NJ: Lawrence Erlbaum 548 Associates. 549 DeCoster, J. (1998). Overview of factor analysis. Retrieved March 22, 2012 from http://www.stat-help 550 .com/notes.html 551 Eklundh L, Pilesjö P (1990) Regionalization and spatial esti- mation of Ethiopian mean annual rainfall. Int J 552 Climatol 10:473 – 494. 553 Fabrigar, L. R., Wegener, D. T., MacCallum, R. C., & Strahan, E. J. (1999). Evaluating the use of exploratory 554 factor analysis in psychological research. Psychological Methods, 4, 272-299. 555 Field, A. (2009). Discovering Statistics Using SPSS: Introducing Statistical Method (3rd ed.). Thousand Oaks, 556 CA: Sage Publications. 557 Fung, D. K. (1995). Sensitivity analysis in factor analysis: Difference between using covariance and correlation 558 matrices. Psychometrika, 60 (4), 607-617. doi: 10.1007/BF02294330 559 Goodale, C.L., Aber, J.D., and Ollinger, S.V., 1998: Mapping monthly precipitation, temperature and solar 560 radiation for Ireland with polynomial regression and digital elevation model. Clim. Res 1998;10:35-49. 561 Gorsuch, R.L. (1983). Factor analysis (2nd ed.). Hillsdale, NJ: Lawrence Erlbaum Associates. 562 Goudie, A. S., & Middleton. N. J., 2001: Saharan dust storms:Nature and consequences. Earth-Science Rev. 56: 563 179–204.</p><p>564 Gullu, G., G. Dogan, G., Tuncel, 2005: Source Regions of Dust Transported to the EasternMediteranean 565 Proceedings of the Third International Symposium on Air Quality Management at Urban,Regional and Global 566 Scales. 26-30 September 2005, Istanbul – Turkey 567 Hamidi M., Kavianpour M.R., and Shao Y., 2014: Synoptic analysis of Dust Storms in the Middle East. Asia- 568 pacific J. Atmos. Sci., 49(3), 279-286. 569 Hayton, J. C., Allen, D. G., & Scarpello, V. (2004). Factor retention decisions in exploratory factor analysis: A 570 tutorial on parallel analysis. Organizational Research Methods, 7, 191-205. 571 Henson RK, Robert JK. 2006. Use of exploratory factor analysis in published research: common errors and 572 some comment on improved practice. Educ. Psychol. Meas. 66: 393–416. 573 574 IPCC, 2013. Summary for Policymakers. In: Climate Change 2013: The Physical Science Basis. Contribution of 575 Working Group I to the Fifth Assessment Report of the Intergovernmental Panel on Climate Change [Stocker, 576 T.F., et al. (eds.)]. Cambridge University Press, 3-29. PDF (9.2 MB) 577</p><p>10 ARABIAN PENINSULA SAND STORMS REGIONALISATION AND TRENDS ANALYSIS</p><p>578 Jolliffe IT. 1993. Principal component analysis: a beginner’s guide-II. Pitfalls, myths and extensions. Weather 579 48: 246–253. 580 581 Jolliffe IT. 2002. Principal Component Analysis, 2nd ed. Springer-Verlag: New York, NY. 582 583 Jolliffe I. 2005. Principal component analysis. In Encyclopedia of Statistics in Behavioral Science, Vol. 3. John 584 Wiley & Sons. Ltd.: Chichester, UK, 1580–1584, doi: 10.1002/0470013192.bsa501. 585 Kaiser, H. F. (1960). The application of electronic computers to factor analysis. Educational and Psychological 586 Measurement, 20, 141-151. doi: 10.1177/001316446002000116 587 Kutzbach JE (1967) Empirical eigenvectors of sea-level sur- face temperature and precipitation complexes over 588 North America. J Appl Meteorol 6:791–802 589 Ming, L., Douglas, L., Westphal, A., Walker, T., Holt, K., Richardson, and Steven, D., 2007, COAMPS Real- 590 Time Dust Storm Forecasting during Operation Iraqi Freedom. Wea. Forecasting, 22, 192-206 [doi: 591 http://dx.doi.org/10.1175/WAF971.1] 592 593 Natsagdorj, L., Jugder, D., and Chung, Y.S., 2003: Analysis of dust storms observed in Mongolia during 1937– 594 1999. Atmospheric Environment 37 (2003) 1401–1411. 595 Nissen, K.M., Leckebusch, G.C., Pinto, J.G., Renggli, D., Ulbrich, S., and Ulbrich, U., 2010: Cyclones causing 596 wind storms in the Mediterranean: characteristics, trends and links to large-scale patterns, 2010, Nat. Hazards 597 Earth Syst. Sci., 10, 1379–1391, 2010. 598</p><p>599 Notaro, M., Alkolibi, F., Fadda, E., and Bakhrjy, F., 2013: Trajectory analysis of Saudi Arabian dust storms 600 Journal Of Geophysical Research: Atmospheres, 118, 6028–6043, [Doi:10.1002/Jgrd.50346,2014]. 601 602 Notaro, M., Yu, Y., Kalashnikova, O., 2015: Regime shift in Arabian dust activity, triggered by persistent 603 Fertile Crescent drought, Journal of Geophysical Research-Atmosphere, 120 (19), 10229-10249. 604 605 Ogallo LJ (1989) The spatial and temporal patterns of the east African seasonal rainfall derived from principal 606 compo- nent analysis. Int J Climatol 9:145–167 607 Prospero, J.M., Ginoux, P., Torres, O., Sharon E. Nicholson, S.E., and Gill, T.E., 2002: Environmental 608 characteristics of Global Sources of Atmospheric Soil Dust identified with the Nimbus 7 Total Ozone Mapping 609 Spectrometer (TOMS) Absorbing Aerosol Product. Reviews of Geophysics, 40, 1 / February 2002. 610 Richman M, Lamb PJ (1985) Climatic pattern analysis of 3- and 7-day summer rainfall in the central United 611 States: some methodological considerations and a regionaliza- tion. J Clim Appl Meteorol 24:1325–1343 612 Richman M, Lamb PJ (1987) Pattern analysis of growing sea- son precipitation in southern Canada. Atmos 613 Ocean 25(2): 137 – 158 614 Rummel, R.J. (1970). Applied factor analysis. Evanston, IL: Northwestern University Press. 615 Shao, Y., Klose, M., Wyrwoll, K-H., 2013: Recent global dust trend and connections to climate forcing, Journal 616 of Geophysical Research-Atmosphere, 118, 11107-11118. 617 Stevens, J. P. (2002). Applied multivariate statistics for the social sciences (4th ed.). Hillsdale, NS: Erlbaum. 618 Tabachnick, B. G., & Fidell, L. S. (2007). Using multivariate statistics (5th ed.) Upper Saddle River, NJ: Pearson 619 Allyn & Bacon. 620 Taghavi, F., and Asadi, A., 2008: cited 2014 (only found on EUMETSAT website): The Persian Gulf 12th April 621 2007 dust storm : Observation and model analysis [ available on line at https://www.eumetsat.int/cs/idcplg? 622 IdcService=GET_FILE&dDocName=pdf_conf_p_s5_25_taghavi_p&allowInterrupt=1&noSaveAs=1&Revision 623 SelectionMethod=LatestReleased ]. 624 UCAR/COMET. 2010. Forecasting Dust Storms v2. [http://www.meted. ucar.edu/mesoprim/dust]. 625 United Nations Environment Program (UNEP), 2001: Early Warning and Assessment Technical Report: 626 Demise of an Ecosystem available on line: 627 [http://eoimages.gsfc.nasa.gov/images/imagerecords/1000/1716/meso2.pdf] 628</p><p>11 M. ALOBAIDI et al.</p><p>629 Virmani SM. 1980. Need, relevance, and objectives of the consultants meeting on climate classification. In 630 Proceeding of Climatic Classification: A Consultants’ Meeting, 14–16 April 1980, Interna- tional Crops 631 Research Institute for the Semi-Arid Tropics, Patancheru, Andhra Pradesh, India, xvii–xx. 632 633 Washington, R., Todd, M., Middleton, N.J., Goudie, A.S., 2003. Dust-storm source areas determined by the 634 Total Ozone Monitoring Spectrometer and Surface Observations, Annuals of the Association of American 635 Geographers, 93(2), 297-313. 636 White D, Richman M, Yarnal B (1991) Climate regionalization and rotation of principal components. Int J 637 Climatol 11: 1–25 638 Wilkerson, W. D., 1991: Dust and dust forecasting in Iraq and adjoining countries. USAF Environmental 639 Technical Applications Center, 72 pp. 640 Wilks DS. 2006. Statistical Methods in the Atmospheric Sciences. Aca- demic Press: London. 641 642 Wigley TML, Lough LM, Jones PD. 1984. Spatial patterns of precipita- tion in England and Wales and a 643 revised, homogeneous England and Wales precipitation series. J. Climatol. 4: 1–25. 644 (WMO, 1988) General Meteorological Standards and Recommended Practices 645 http://www.wmo.int/pages/prog/hwrp/publications/technical_regulations/WMO_N49_TechnReg_Vol1.pdf 646 647 (WMO, 2008) Guidelines on Quality Control Procedures for Data from Automatic Weather Stations 648 https://www.wmo.int/pages/prog/www/OSY/Meetings/ET-AWS3/Doc4(1).pdf 649 Yarnal B. 1993. Synoptic Climatology in Environmental Analysis. Belhaven Press: London. 650 Yong, A. G. & Pearce, S. (2013). A Beginner’s Guide to Factor Analysis: Focusing on Exploratory Factor 651 Analysis. Tutorials in Quantitative Methods for Psychology 2013, Vol. 9(2), p. 79-94. 652 Yu, Y., Notaro, M., Liu, Z., Kalashnikova, O., Alkolibi, F., Fadda, E., Bakhrjy, F., 2013: Assessing temporal 653 and spatial variations in atmospheric dust over Saudi Arabia through satellite, radiometric, and station data, 654 Journal of Geophysical Research-Atmosphere, 118, 13253-13264. 655 656 Yu, Y., Notaro, M., Liu, Z., Wang, F., Alkolibi, F., Fadda, E., Bakhrjy, F., 2015: Climatic controls on the 657 interannual to decadal variability in Saudi Arabian dust activity: toward the development of a seasonal dust 658 prediction model, Journal of Geophysical Research-Atmosphere, 120 (5), 1739-1758. 659</p><p>12</p>
Details
-
File Typepdf
-
Upload Time-
-
Content LanguagesEnglish
-
Upload UserAnonymous/Not logged-in
-
File Pages12 Page
-
File Size-