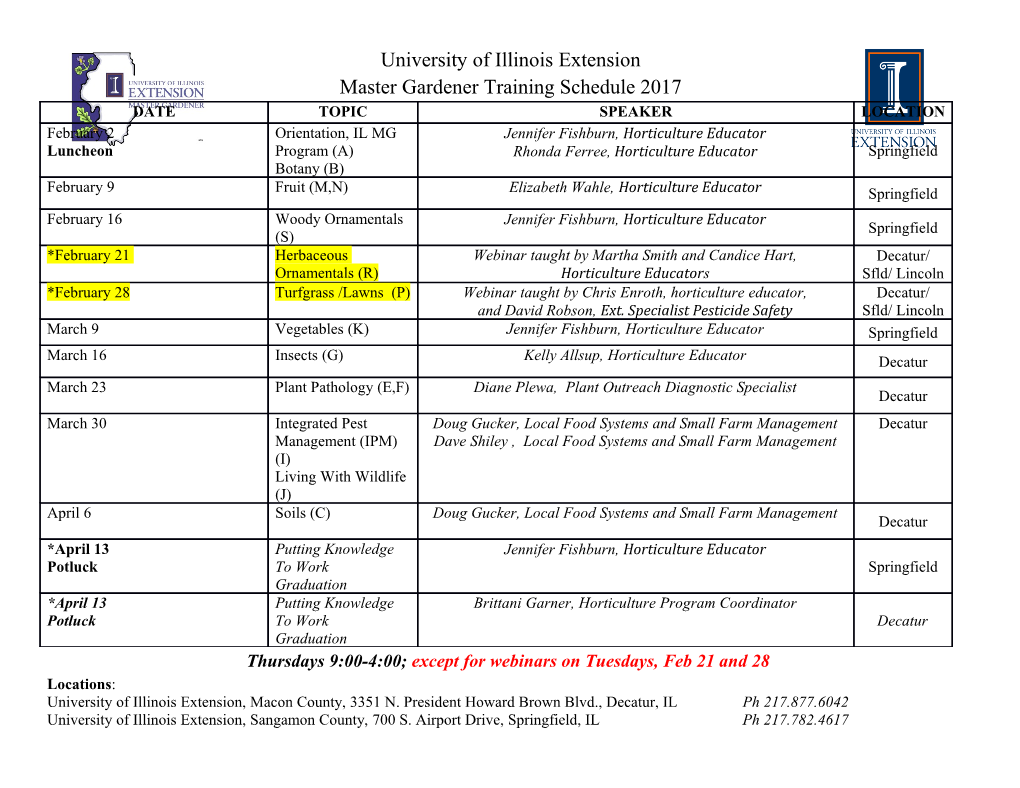
<p> AP Statistics - Chapter 3 Summary Sheet</p><p>CSSO: Use this acronym when being asked to comment on the appearance of or to describe any distribution of single variable data. It stands for Center – Shape – Spread – Outliers.</p><p>FSDD: Use this acronym when being asked to comment on the appearance of or to describe any scatterplot of bivariate data. It stands for Form – Strength – Direction – Deviations.</p><p>When asked to explain the meaning of – or to interpret:</p><p>Slope ( b ) of the LSRL: There is “ON AVERAGE” a (numerical slope value increase/decrease) in the ( Response variable named with the correct units) per a one unit increase in the (Explanatory Variable named with correct units).</p><p>Correlation Coefficient r: There is a (very strong , strong, moderate, weak, very weak) (positive/negative) linear relationship between (state the name of the response variable in the context of the problem) and (state the name of the explanatory variable in the context of the problem) .</p><p>Coefficient of determination r 2: (Percentage arrived at on calculator) % of the VARIATION in the (state the name of the response variable in the context of the problem) can be explained by a linear relationship to the (state the name of the explanatory variable in the context of the problem).</p><p>(or)</p><p>(Percentage arrived at on calculator) % of the VARIATION in the (state the name of the response variable in the context of the problem) can be explained by the LSRL of (state the name of the response variable in the context of the problem) on (state the name of the explanatory variable in the context of the problem)</p><p>Facts on Correlation: 1. Correlation makes no distinction regarding which you call x and which you call y. Switch the axes, and the r – value remains the same. 2. Correlation requires Quantitative Variable only. (Ex: There is no such thing as a correlation between race and crime; or gender and IQ.) 3. Because r (based on standardized z-values) is itself, unit-less … changes units of measure will not alter the r value. (Ex: height in inches vs. age in months, r =.963. Repeat same data with height in cm vs. age in days, and the r still will - .963) 4. Positive r indicates a positive association (and positive sloped LSRL); Negative r indicates a negative association (and a negatively slope LSRL). 5. 1 r 1; Where r = 1 is a perfectly linear positive relationship; and r = -1 is a perfectly linear negative relationship. An r = 0 indicates the weakest linear association between variables. AS you move away from 0 to either extreme, this indicates a stronger relationship. 6. Correlation is ONLY applicable to measuring the strength of a LINEAR relationship. 7. Similar to the mean and standard deviation, r is very much so influenced by individual extreme values (regression outliers and influential points). That is to say … r is a “non-resistant measure. Facts on the LSRL: 1. The distinction between the explanatory and response variable is essential, and therefore, will make a huge difference if their roles are switched. Unlike with r, when you switch the x and y roles, a new result in the form of a new LSRL will be formed. 2. There is a close relationship between the SLOPE of the LSRL and the correlation r. Specifically,</p><p> sy b r . This also means that the higher r is, and then changes in x will be more noticeable in their sx impact on yˆ . When r is very low (and weak), then the impact is not as drastic. This is the low r, will in turn make a less steeped line (approaching horizontal), and when even big changes in x do NOT produce big responses in yˆ . 3. The LSRL always contains the point X ,Y . So the LSRL then can be described solely in terms of the </p><p> sy descriptive statistics X ,sx ,Y,sy ,r. Again form Fact 2:b r ; and also a Y bX . sx 4. The square of the correlation r, i.e. r 2 (coefficient of determination) is the fraction (or percentage) of the variation on the values of y that is explained by the LSRL of y on x. 5. The final determination as to the validity and overall “correctness” and strength of a LSRL model rests upon a visual examination of the RESIDUAL PLOT. Even with exceptionally high values of r close to 1 or -1, the patterns that might appear in the residual plot will over-ride any r – value. (See pp. 170-171; p. 800 (good plots for good LSRL), and p. 801 (for 2 ways the residuals can show a bad LSRL).</p><p>What is left for you??? Do random problems in 3.1, 3.2 and 3.3 Can you read and interpret, and sift through extraneous information on computer generated reports (ex: see p. 156 figure 3.12; p. 189 problem # 3.76; p. 787 problems 14.2 and 14.3; p. 795 problem # 14.10 and 14.11 1.1 summary: pp.134 -135; 1.2 summary: pp.146 – 147; 1.3 summary: p.176; Chapter review summary: pp. 181- 183 Review problems; pp. 183 – 190 Practice Test A and B Examine solutions to the quizzes that you took – Solutions are posted in Class.</p>
Details
-
File Typepdf
-
Upload Time-
-
Content LanguagesEnglish
-
Upload UserAnonymous/Not logged-in
-
File Pages2 Page
-
File Size-