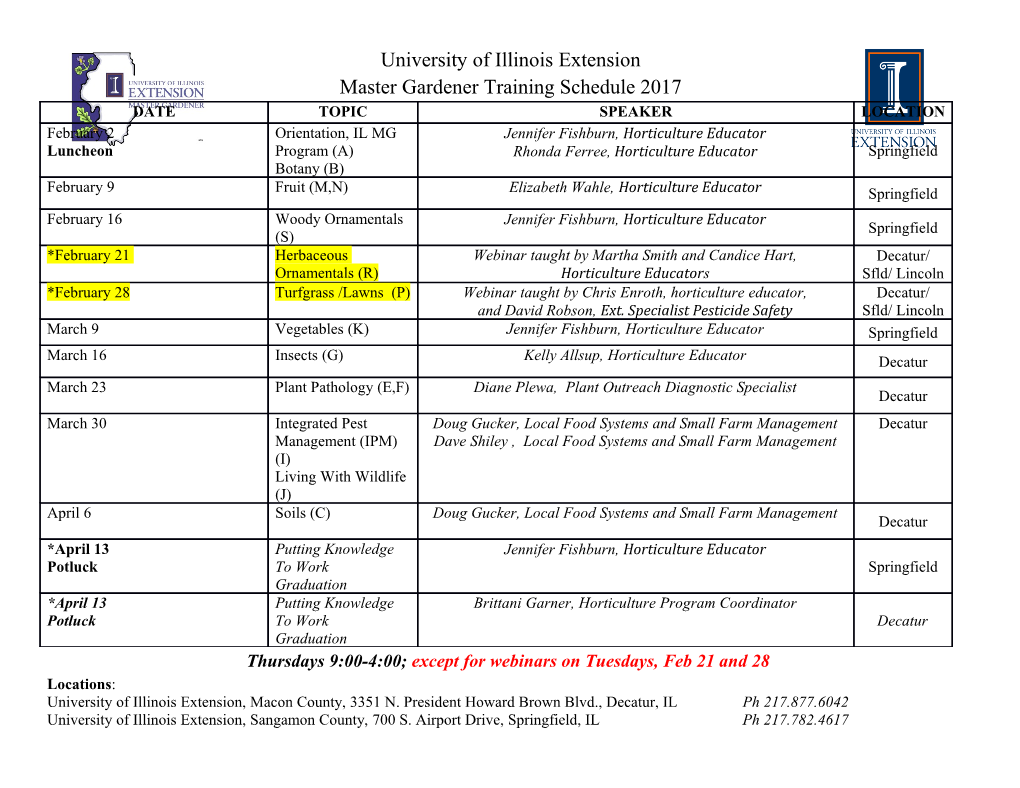
<p> 1</p><p>Haapala, Anu, Pietarinen, Janne, Rautopuro Juhani & Väisänen, Pertti</p><p>How to overcome stumbling blocks in learning applied statistics- The effect of concept mapping</p><p>Paper presented at the European Conference on Educational Research, University of Lisbon, 11-14 September 2002.</p><p>Introduction</p><p>As to statistics instruction in universities world wide, there is much research that suggests that compulsory statistics courses are challenging for teachers to teach and for students to learn, particularly in social sciences and education (Forte 1995; Schutz et al. 1998; Townsend et al. 1998; Yilmaz 1996). The academic setting of the present study is a Finnish Faculty of Education and the participants of the applied statistics course ‘Quantitative Research Methods in Education’ come mainly from teacher education programs. Students’ learning in this course will be tried to foster with the help of a research project that started in the spring term 2002 as an exploration using a survey and a concept map technique as research methods. In general, tertiary level statistics courses normally involve students from a variety of academic disciplines and levels of statistical and mathematical skills. Thus, due to the heterogeneity of participants, the course can become a forum for test, statistics and computer anxieties for some students, while others may not have any problems while taking the class. For many students, particularly in teacher education programs statistics is of no intrinsic interest but merely a hurdle to be overcome on the way to obtaining a master’s degree and thus leads them to put a minimum of effort to pass the course. Consequently, it is hard for learning to occur when emotions and attitudes in the lecture hall or computer lab are ranging from boredom for some students to frustration, anger, resistance and fear for others. (Cf, Gal & Ginsburg 1994.) In addition to attitudinal problems, we and many other statistics instructors have noted university students’ poor skills in statistics, even after they have taken one or more statistics courses. They may lack understanding of the connections among the important, functional concepts in the discipline, for example. Without understanding these concepts, students cannot effectively and efficiently engage in statistical reasoning and problem-solving required by their master’s theses, for example. According to Yilmaz (1996), there are many reasons why statistics is a difficult subject for non-specialists, not just from the viewpoint of the student but from the teacher as well. These include, for example, the abstract and complex nature of statistics concepts and the requirement for a student to has analytical and synthesising skills as well as some basic mathematical skills. These and other challenges involved in the teaching of statistics have resulted in a variety of studies designed to investigate factors that influence success – or failures respectively – in statistics classes. Previous studies have indicated that success is positively affected by students’ prior mathematics skills, prior statistics knowledge, and positive attitudes toward statistics as well as motivational strategies such as confidence about learning statistics and control of learning beliefs (Elmore, Lewis & Bay 1993; Green 1994; Schutz et al. 1998). In addition, typical for the high- performers in statistics are certain learning strategies such as self-monitoring of learning, 2 visualisation, relating the material to the real world with personal examples, and trying to keep mentally in touch with the class material (Schutz et al. 1998). Finally, others have looked at how, e.g., the gender of the student influences performance in statistics classes. These studies, however, have reported contradictory findings (see e.g. Schutz et al. 1998). On the opposite, there is much research on the factors that may cause learning problems in statistics classes. These are, for example, deficiencies in the mathematical background of students, prior beliefs, misconceptions and strongly-held but incorrect intuitions and pre-dispositions against statistics caused by, e.g., intuition or prior learning experiences in mathematics (Batanero et al.1994; Galagedera 1998; Garfield 1997; Konold 1995). In addition, problems in learning statistics can be associated with the students’ non-cognitive or socio-personal factors, such as negative emotions (e.g., fears), attitudes and beliefs and lack of motivation (Gal & Ginsburg 1994) as well as test anxiety (Benson 1989), anxiety about mathematics (Townsend et al. 1998; Zeidner 1991) or statistics anxiety (Birenbaum & Eylath 1994; Onwuegbuzie & Seaman 1995; Zeidner 1991), weak self-confidence in learning statistics and low self-efficacy (Forte 1995; Townsend et al. 1998). Thus, due to the problems in teaching and learning statistics, researchers and instructors have offered a variety of means as a solution to them, such as co-operative and collaborative forms of learning (Blalock 1987; Townsend et al. 1998; Townsend & Moore 1994) or ways of encouraging active learning (Garfield 1995; Moore 1997), efforts to make courses more applied and more practical with real data, cases and examples for solving real-life problems (Cobb 1992; Khamis1991; Nolan & Speed 1999; Royse & Rompf 1992; Watts & Carlson 1999) and the use of statistical software packages for computer-assisted instruction and computer simulations (Marasinghe & Meeker 1996; Moore 1997). In addition, different materials and aids, such as multimedia, videotapes and transparency sets (Moore 1993; Velleman & Moore 1996), the use of supportive teaching strategies to reduce anxiety (Schacht 1991) as well as games and humour (Friedman et al. 1999; Royse & Rompf 1992) has been recommended. Furthermore, some authors (e.g., Rubin 1992) recommend that only the faculty from the students’ main subject should teach statistics courses because they master the domain of the particular discipline and can adequately demonstrate the utility of research to students. Consequently, the interest in this piloting experiment was to find out what are the non- cognitive obstacles in learning applied statistics with non-specialists and whether we can overcome these obstacles through students’ engagement in active learning fostered by concept mapping, on the one hand, and what are the cognitive and non-cognitive factors that explain student’s learning outcomes and whether there are any differences between the concept-map group and the non- concept-map group in their learning experiences and outcomes as well, on the other hand. Concept mapping was selected as an experimental treatment due to promising findings in previous studies. According to Kinchin (2000), previous studies have suggested that the use of concept mapping helps students to gain a more unified understanding of a topic, organise their knowledge for more effective problem solving, and understand how to learn (i.e. to become more metacognitively aware). Furthermore, the promotion of meaningful learning resulting from concept mapping can act to reduce subject-based anxiety and overcome differential gender-related performance with respect to learning and achievement in a subject (Kinchin 2000). Thus, we hypothesise that the concept-mapping group has a better achievement in the course exam than the non-concept-mapping group. Furthermore, we believe that concept mapping is a good tool for measuring conceptual understanding that is usually considered one of several aspects of statistical knowledge (however, a detailed analysis of concept maps is under work and will not be reported here). By the term conceptual understanding Broers (2001) refers to the ability of students to tie their knowledge of statistical ideas and concepts into a network of interrelated propositions. Further convince of usefulness of concept mapping was offered by many studies that have shown this technique to be a valid and reliable measure of what a student understands (cf. Shavelson & Ruiz-Primo 2000). 3</p><p>Instead, traditional tests may measure only surface learning and learned routines and algorithms if not composed carefully. To conclude, in statistics instruction there are only a few reported studies that have used concept mapping (e.g., Roberts 1999; Schau & Mattern 1997) until now. Therefore, this study is an explorative endeavour where this technique as a tool for learning and measurement will be put under a scrutiny. </p><p>Theoretical Background</p><p>Concept Map as a Learning Tool</p><p>Much of the recent reform movement in education, especially in mathematics and science, has been based on the constructivist theory of learning. This theory explains the process of learning as actively constructing knowledge, which interacts with previous knowledge, beliefs, and intuitions. Therefore, we should encourage our students also in statistics classes (e.g., Moore & Cobb 1995) to be actively involved in their own learning and offer a learning environment that stimulates active learning and interaction between their prior knowledge, beliefs and the new knowledge to be learned, i.e., learning should be meaningful. Moreover, it is important to examine the relationship between beliefs and attitudes in statistics learning because research on mathematics (e.g., McLeod 1992) – given that many students identify statistics with mathematics – suggest that beliefs may be filters through which experiences and new knowledge are interpreted by learners. Meaningful learning and conceptual understanding may be encouraged by using concept maps as tools for learning (Roberts 1999; Shavelson et al. 1993). Concept maps – also used as an authentic assessment method – are believed to help students to assimilate well-integrated, strongly cohesive frameworks of interrelated concepts as a way of facilitating ‘real understanding’ of (natural) phenomena (Mintzes et al. 2001) or deep learning (Novak & Gowin 1984). Previous research suggest that concept-mapping is a highly flexible tool that can be adapted for use almost any group of learners in education, students and teachers from primary schools to universities, for example. A variety of student groups, e.g., low-achieving students have benefited from concept mapping technique (e.g., Guastello 2000). Concept map is based on Ausubel’s theory of meaningful learning. In this the learner is required to make a conscious effort to identify the key concepts in new information and relate them to concepts in her existing knowledge structure. Therefore, concept maps represent the organisation and structure of students’ ideas, the internal representation of knowledge, with emphasis on the relations or interconnectedness between ideas or units of information. Information is represented in a non-linear fashion in which concepts are linked in meaningful ways. (Oughton & Reed 2000; Pintrich, Marx & Boyle 1993; Williams 1998.) Williams (1998) has concluded from the literature of cognitive psychology that the more connections that exist among facts, ideas, and procedures, the better the understanding of an individual. In addition, individuals whose knowledge within a particular domain is interconnected and structured will activate large chunks of information when they perform an activity in that knowledge domain and highly integrated knowledge structure signals the transition from novice to expert performance. Williams conclude, however, that the degree to which concept maps describe a person’s actual mental representation is impossible to know. In practice, concept maps are graphical representations of an individual’s knowledge framework, and they consist of nodes and labelled lines. The nodes correspond to relevant concepts in a domain and the lines (links) express a relationship between a pair of concepts. The label on the line expresses how two concepts are related (Shavelson, Lang & Lewin 1993). The lines (visually 4 displayed as one- or two-headed arrows) should be labelled so that the meaning between the two concepts (nodes) is explicitly expressed. The combination of two concepts and a label line is referred to as a proposition, which is a unit of factual knowledge – or the smallest item of knowledge that can stand as an assertion (McNamarra 1994). Being individuals’ inner mental representations, such propositions are related to each other to form simple cognitive schemata, which can connect again to each other to form more complex knowledge structures. In concept maps, hierarchical structure and integration of concepts (cross-links between branches of concepts) are indices of an elaborated map (Novak, Gowin & Johansen 1983; Novak & Gowin 1984) and can reflect a more elaborated cognitive structure on the given sphere of knowledge of an individual. In previous studies using concept maps as tools of research, learning or assessment, there has also occurred disappointments and criticism in different disciplines (e.g., Hodson 1998; Parkes et al. 2000; Roberts 1999; Zimmaro et al. 1999). Part of this research seem to indicate that students do not fully implement concept maps but may wish to conceal some aspects of their understanding while part of research is incredulous of what it is that is measured by concept maps, i.e., conceptual understanding or bare propositional knowledge. Broers (2001), for example, argues that concept maps largely ignore the way that a student decomposes the statistical theory into propositions and they only focus on the big picture, and by doing so this technique pays scant attention to the way that students actually organise the statistical material. However, research on concept maps conducted in statistics (Roberts 1999; Schau & Mattern 1997) appears to show concept maps to be useful tools in instruction and assessment in statistics.</p><p>Statistics Anxiety</p><p>In this study we are also interested in to find out whether concept mapping is a learning tool that helps students to overcome their affective learning blocks, e.g., negative learning experiences in statistics. Statistics anxiety has been defined as an anxiety that occurs as a result of encountering statistics in any form and at any level (Onwuegbuzie et al. 1997). According to Onwuegbuzie and others (1997), it involves a complex array of emotional reactions that interfere with learning. Also, statistics anxiety can be considered to be situation-specific, inasmuch as the symptoms only emerge when learning or applying statistics. Previous research (see Onwuegbuzie 1999) have found the following factors to be statistically significant predictors of statistics anxiety: gender, mathematics self-concept, number of college mathematics courses completed, academic major, academic status, perceptions of previous success in mathematics courses, time elapsed since students’ last mathematics course, mathematics ability, mathematics preparation, calculator use, and expected grade. Additionally, learning modalities, level of perfectionism, students’ orientations toward social interdependence, level of academic locus of control, and study behaviours have been found to be related to statistics anxiety (Onwuegbuzie & Daley 1996, in Onwuegbuzie 1999). From our experience as teachers and also supported by previous research (see Townsend et al 1998), we can expect that many of these factors are unfavourable for the target group of our study, i.e., the majority are female with low mathematics self-concept and ability in mathematics, for example. Statistics anxiety has also been conceptualised as a multifaceted phenomenon (Cruise, Cash & Bolton 1985). Using factor analysis, Cruise and others (1985) identified six components of statistics anxiety: 1) worth of statistics, 2) interpretation anxiety, 3) test and class anxiety, 4) computational self-concept, 5) fear of asking help, and 6) fear of statistics teachers. Townsend and others (1998) examined the changes in mathematics self-concept and mathematics anxiety within students (n = 153) enrolled in the university level educational psychology course. The course emphasising co-operative learning activities included a laboratory component in social sciences statistics. Mathematics anxiety was defined according to Tobias (1995) as a person’s feelings of tension and anxiety that interfere with the manipulation of numbers 5 and the solving of mathematical problems in a wide variety of ordinary and academic situations. Although the results of the study showed that co-operative learning is effective in increasing the mathematics self-concept and there were positive changes in students’ confidence about doing statistics, their perceptions of anxiety in mathematics did not decrease over the course. This result, although consistent with previous research, re-affirms the fact that task of modifying deeply entrenched beliefs and attitudes is not an easy one, i.e. negative attitudes are highly resistant to change (cf, Birenbaum & Eylath 1994).</p><p>Research Problems </p><p>The research questions of the study have been defined as follows: 1. How do students feel about learning quantitative methods? a. Are there any differences in affective factors of learning quantitative methods and prior knowledge between, for example, gender, campus and degree program? </p><p>2. How are affective factors, prior knowledge and some background variables (e.g. maths school grade, elementary statistics course exam score) related into the learning results and learning experiences in different learning groups?</p><p>3. What is the relevance of using concept maps in learning quantitative methods? a. Are there any differences in learning results between the concept-map group and the non-concept-map group? b. Are there any differences in experiencing learning of quantitative methods between the concept-map group and the non-concept-map group? c. How are learning experiences related into the learning results in different learning groups?</p><p>Methods</p><p>The Subjects</p><p>There were 232 students enrolled in the course Quantitative Research Methods in Education 1 (2 cu) given in two campuses (Joensuu and Savonlinna) of the Faculty of Education, University of Joensuu in the spring term 2002. The teachers of the course were two of the authors of this paper (Haapala and Väisänen). This subject study level course was subsequent to the elementary statistics course and consisted of inferential statistics with the main stress on basic concepts and univariate statistical testing (nonparametric and parametric) as well as estimation of reliability of scales. Statistical analyses were based on the SPSS V10.0 printouts. Although the contents of the courses and contact teaching hours as well as the assignments of the course exams were very similar between the campuses they were not identical. All students enrolled in the course took part in the study (in Joensuu n = 83, and in Savonlinna n = 149). About one fifth (n = 51) of them came from classroom teacher education program, about one fifth (n = 50) from kindergarten teacher program and one tenth (n = 23) of them studied science of education as a major subject. The rest of them were mainly students to become teachers of special education (n = 20), home economics (n = 17), arts and crafts (n = 18) or study counsellors. 6</p><p>Experimental Design and Measurements</p><p>In the beginning of the course one third of the participants was randomly assigned (systematic sampling) to a concept mapping group (an experiment group) and thus two third of participants formed a control group. At the end of the course 56 students returned their concept maps. As an incentive these students were given extra points (bonus) for course exam. However, in order to avoid systematic error in data, these extras were excluded from the variable ‘course grade’. Participants of the control group in Savonlinna also wrote a learning log (not analysed here). In order to answer to the research questions, the course grades and the scores from the questionnaires were compared between the concept-map group and the non-concept-map group. Three measurements were conducted. In the beginning of the course the participants’ (1) prior knowledge and possible misconceptions were diagnosed using a test consisting of five assignments about very basics of statistics, i.e., probability (“the law of small numbers”), interpretation of crosstabulated data, reading of same data scaled with different ranges, correlation diagrams and inferring group differences on the basis of means and standard deviation with given sample sizes. At the second stage of the study, (2) the affective factors in learning (e.g., motivation, attitudes, self-efficacy, and anxiety about mathematics) were surveyed using a Likert-type questionnaire scaled 1–5, which included 35 statements. The scale to reflect attitudes, beliefs and feelings and to assess levels of anxiety related to doing statistics was partly proportional to research of Townsend et al. (1998) in mathematics. At the end of the course (3) students’ learning experiences, locus of control and effort to active learning were surveyed by using a Likert-type questionnaire scaled 1–5 (35 statements). In addition to this, there were some questions for self-rating of the learning outcomes and participation in the course (not reported here). Basic dimensions of the first questionnaire (affective factors in learning) were analysed with the help of factor analysis using principal axis factoring as an extraction method and direct oblim as a rotation method. A five factors solution was deemed to be the most interpretable. The five factors were labelled as F1 = Orientation of Avoidance, which might be defined to have four dimensions (negative emotions, negative attitudes, lack of motivation or external motivation, with Cronbach’s alpha coefficient .86), F2 = Course Specific Anxiety (alpha .77), F3 = Self Confidence in Learning Mathematics (alpha = .79), F4 = Mathematics Anxiety (alpha = .77) and F5 = Self-efficacy (course specific and general, alpha = .78). (See Appendix 1) Also the basic dimensions of the second (post course) questionnaire were analysed with the help of factor analysis using principal axis factoring as an extraction method and direct oblim as a rotation method. The five factors were labelled as F1 = Positive Learning Experiences (alpha = .88), F2 = Learning Efforts (alpha = .81), F3 = Test Anxiety (alpha = .73), F4 = Resorting to Other Students (alpha = .56) and F5 = Responsibility (alpha = . 63) In both analyses factor score coefficient variables were computed and used in further analyses. (See Appendix 2) </p><p>The differences between different groups were analysed by using t-tests (when two samples: campus, gender, group) and one-way analysis of variance (more than two samples: major subject). </p><p>Results</p><p>In the beginning of the course the prior knowledge (diagnostic test) and affective factors in learning quantitative methods were measured. There were no differences between the concept-map group and the non-concept-map group in the result of diagnostic test or in affective factors. In spite of that there were some statistically significant differences in affective factors when examining other variables (see Table 1). The students in Savonlinna try to avoid learning statistics, they feel more 7 statistics anxiety and less self-efficient than the students in Joensuu. Also female students were less self-efficient than male students. There was also statistically significant difference between the students in different degree programs, when kindergarten teachers seemed to avoid learning statistics, to feel more statistics anxiety and less self-efficient than the others.</p><p>Table 1. The differences between groups in diagnostic test and affective factors in the beginning of the course </p><p>Diagnostic Avoidance Statistics Mathematical Math Self-efficacy test anxiety self- anxiety confidence Campus Joensuu 5.16 (2.24)*) -0.40 (0.99) -0.51 (0.92) 0.02 (0.97) -0.19 (0.85) 0.41 (0.95) Savonlinna 5.74 (2.07) 0.19 (0.89) 0.24 (0.84) -0.01 (0.84) 0.09 (0.99) -0.19 (0.81) p = .055 p = .000 p = .000 p = .761 p = .061 p = .000</p><p>Gender Female 5.50 (2.10) -0.05 (0.96) 0.04 (0.94) -0.03 (0.88) -0.01 (0.98) -0.07 (0.91) Male 5.59 (2.66) 0.39 (0.94) -0.33 (0.78) 0.23 (0.84) 0.08 (0.69) 0.51 (0.66) p = .846 p = .037 p = .072 p = .181 p = .669 p = .004</p><p>Learning group Concept map 5.58 (2.20) -0.05 (0.95) 0.04 (0.99) 0.05 (0.93) -0.07 (0.95) -0.17 (0.85) Traditional 5.61 (2.11) 0.16 (0.88) -0.01 (0.85) -0.06 (0.77) 0.12 (1.01) 0.09 (0.97) p = .943 p = .200 p = .777 p = .692 p = .268 p = .468</p><p>Degree program Kindergarten Teacher 5.45 (2.16) 0.46 (0.90) 0.44 (0.83) -0.02 (0.78) 0.25 (0.98) -0.28 (0.79) Class teacher 5.90 (2.11) 0.14 (0.77) 0.13 (0.78) -0.05 (0.83) -0.06 (0.85) -0.06 (0.77) Domestic Science 5.83 (1.95) -0.22 (0.93) 0.05 (0.96) 0.07 (0.91) 0.05 (1.13) -0.20 (0.87) Education 4.67 (2.10) -0.72 (0.69) -0.38 (1.00) -0.41 (1.05) 0.10 (0.89) -0.10 (0.88) Special Education 6.95 (1.55) -0.23 (0.85) -0.56 (0.63) 0.13 (0.67) -0.48 (0.59) 0.50 (0.57) Other 4.48 (2.14) -0.29 (1.20) -0.60 (0.95) 0.19 (1.05) -0.20 (0.95) 0.65 (1.13) p = .000 p = .000 p = .000 p = .376 p = .106 p = .000 *) Mean (Standard deviation)</p><p>In the end of the course, the students were asked their learning experiences, locus of control and effort to active learning. There was only slight difference between learning groups in sense of responsibility, when students in concept map group seemed to be more responsible of their studies and more ready to relate their learning into prior knowledge than the others in traditional group. Also there were some statistically significant differences in other factors and total exam score when examining the different groups (See Table 2). There were most statistically significant differences between two campuses, when students in Joensuu seemed having more positive learning experiences and taking more responsibility of their studies than students in Savonlinna, which is related to fact that kindergarten teachers, who studied in Savonlinna, had least positive learning experiences and least responsibility of their studies. They also did not succeed in the final exam so well than the others. Students in Joensuu seemed feeling less test anxiety and seemed to succeed better in the final exam than students in Savonlinna. On the other hand the students in Savonlinna seemed to resort to other students more than students in Joensuu. The only statistically significant difference between gender was that male students seemed to have less learning effort than female student. There were also many statistically significant 8</p><p> differences between students in different degree program in addition the differences between kindergarten teachers and the others discussed already above. The students, who are studying science of education as a major subject felt least test anxiety and they did not resort to other students that much than the others.</p><p>Table 2. The differences between groups in learning experiences, locus of control, effort to active learning and total exam scores in the end of the course </p><p>Positive learning Learning Test anxiety Resorting to Responsibility Exam total experiences efforts other students Campus Joensuu 0.57 (0.86) *) 0.11 (0.94) -0.29 (0.82) -0.62 (0.60) 0.43 (0.68) 19.11 (6.24) Savonlinna -0.33 (0.89) -0.02 (0.89) 0.15 (0.89) 0.37 (0.81) -0.27 (0.85) 15.01 (6.49) p = .000 p = .356 p = .002 p = .000 p = .000 p = .000</p><p>Gender Female -0.05 (0.97) 0.10 (0.89) 0.03 (0.89) 0.05 (0.88) -0.02 (0.87) 16.77 (6.72) Male 0.19 (0.86) -0.66 (0.77) -0.26 (0.91) -0.07 (0.88) -0.17 (0.73) 14.30 (6.01) p = .336 p = .001 p = .200 p = .610 p = .498 p = .171</p><p>Learning group Concept map 0.03 (0.96) -0.05 (0.94) -0.04 (0.92) 0.06 (0.91) 0.08 (0.87) 16.58 (6.66) Traditional -0.09 (0.94) 0.01 (0.93) 0.01 (0.88) -0.02 (0.85) -0.20 (0.85) 16.99 (6.74) p = .433 p = .908 p = .351 p = .884 p = .050 p = .704</p><p>Degree program Kindergarten teacher -0.48 (0.77) -0.13 (0.80) 0.22 (0.86) 0.39 (0.83) -0.49 (0.86) 12.83 (6.39) Class teacher 0.01 (0.98) -0.08 (0.87) -0.09 (0.89) 0.48 (0.81) -0.13 (0.84) 16.51 (6.68) Domestic science -0.44 (0.99) 0.24 (0.99) 0.39 (0.87) 0.13 (0.80) -0.09 (0.84) 16.24 (5.93) Education 0.47 (0.78) -0.05 (0.91) -0.49 (0.75) -0.87 (0.60) 0.44 (0.60) 19.65 (6.01) Special education 0.08 (0.75) -0.07 (1.06) -0.27 (0.85) -0.39 (0.54) 0.17 (0.63) 17.31 (6.45) Other 0.64 (0.78) 0.11 (1.06) -0.08 (0.95) 0.49 (0.76) 0.49 (0.86) 19.16 (6.28) p = .000 p = .551 p = .005 p = .000 p = .000 p = .000 *) Mean (Standard deviation)</p><p>The students’ achievements in final exam were predicted firstly by using the information from the pre-course measurements, i.e., diagnostic test, maths school grade, elementary statistics course exam score and affective factors in the beginning of the course. Second, the exams score was predicted by using the factors created based on the results of students’ learning experiences, locus of control and effort to active learning measured at the end of the course. According to the data analysis it seemed that in the beginning of the course in the whole data elementary statistics course exam score, maths school grade and mathematical self-confidence predict positively total exam score. Statistics anxiety explained it negatively. When examining the differences between the concept-map group and the non-concept-map group, it seemed that total exam score was predicted mostly by diagnostic test score and statistic anxiety in traditional group, but in the concept map group statistically significant explanatory variables were elementary statistics course exam score, mathematical self-confidence and maths school grade. (See Table 3)</p><p>Table 3. The results of the regression analysis (stepwise selection)</p><p>Dependent variable: Total exam score 9</p><p>Predictors Regression Standard p-value coefficient error Whole data (F = 9.93, p = .000) (R-square = .222) Elementary course exam score 1.715 .814 .037 Maths school grade .995 .466 .035 Mathematical self-confidence 1.441 .623 .022 Statistics anxiety -1.283 .577 .028 Traditional group (F = 10.79, p = .000) (R-square = 0.179) Diagnostic test score .858 .283 .003 Statistics anxiety -1.735 .639 .008 Concept map group (F = 8.48, p = .000) (R-square = 0.401) Elementary course exam score 3.275 1.258 .013 Mathematical self-confidence 2.604 1.150 .029 Maths school grade 1.552 .715 .036</p><p>The exams score predicted by using the factors created based on the post course questionnaire, it seemed that both in the whole data and in two learning groups positive learning experiences and learning effort were the most explanatory variables in predicting total exam score. In concept map group also test anxiety predicted negatively total exam score. (See Table 4)</p><p>Table 4. The results of the regression analysis (stepwise selection)</p><p>Dependent variable: Total exam score</p><p>Predictors Regression Standard p-value coefficient error Whole data (F = 35.63, p = .000) (R-square = .284) Positive learning experiences 2.989 .446 .000 Learning efforts 1.901 .457 .000 Traditional group (F = 22.66, p = .000) (R-square = 0.510) Positive learning experiences 2.861 .538 .000 Learning efforts 1.774 .550 .000 Concept map group (F = 11.51, p = .000) (R-square = 0.651) Positive learning experiences 2.992 1.258 .001 Learning efforts 2.712 1.150 .002 Test anxiety -1.822 .715 .049 10</p><p>Conclusions</p><p>Contradictory to our hypotheses it seemed that there were no differences either in cognitive learning outcomes or in affective factors of learning between the concept-mapping group and non-concept- mapping group. In spite of different learning groups there are some other factors like prior knowledge (maths school grade, elementary statistics course exam score and diagnostic test score), mathematical self-confidence and statistics anxiety, which predict the learning outcomes. Also significant factors are positive learning experiences and effort to active in spite of learning group and methods used. The concept-mapping group seemed to be slightly more responsible in learning than the others, however it did not result in better achievements in final exam. According to former research (Guastello 2000), the better performance of the mapping group in studies could be explained by students’ active engagement in learning. It is possible that active participation associated with the concept-mapping technique simply serves to keep students focused on their task so that they assimilate more information. In this study it seems that student did not invest that much time and efforts on working with the concept map task. Also feedback from the teaching assistants with the course (Parkes et al. 2000) and other research conducted on the maps themselves (Zimmaro et al. 1999) seems to indicate that students do not fully implement concept maps. In their study that aimed at examining the effects of concept maps on the task-related variance components in performance assessments, Parkes and others (2000) concluded that the implications of concept- mapping are not for reliability alone but there are validity implications as well. In spite of fact, that the concept maps students constructed, were not analysed for this study, they were given a brief survey, which showed that some of the maps were quite poor and some students expressed confusion about how the maps should help them. The relatively weak effect that the concept maps apparently had on the cognitive structure of the students is due to two factors. First, students at least in Joensuu, were not very well used to use concept maps as a learning tool and they were not very well trained for it. Second, the maps could have been better embedded in the instruction. Students were not reminded often about what the maps should do for them. They were not helped to use them or encouraged to make them a part of their studying for the course. The students, however, mentioned quite many propositions in their concept maps. Anyhow the propositions do not constitute direct evidence that the students have mastered conceptual understanding of the course material. As research has indicated (Schau & Mattern 1997), there is a difference between propositional knowledge and conceptual understanding, and what we have established in this study may be the existence of propositional knowledge rather than conceptual understanding. Hodson (1998) notes that some students may wish to conceal some aspects of their understanding and so their maps would not provide ‘total insight’ to the student’s perspective. In addition, it is clear from observations of changes in students’ maps that such structures are in a continual state of flux in an active learner. According to Williams (1998) the degree to which concept maps describe a person’s actual mental representation is impossible to know. However, evidence from Williams’ study as a whole did suggest subtle differences between the concept-map group and non-concept-map group. The analysis also provided information about students’ understanding that is not readily gained from the traditional pen-and-paper tests. Concept maps, therefore, provide important information about conceptual understanding and can play a useful role in the statistics researchers’ repertoire of tools. In the forthcoming study this perspective will be examined more specifically.</p><p>References 11</p><p>Ayersman, D.J. 1995. Effects of knowledge representation format and hypermedia instruction on metacognitive accur- acy. Computers in Human Behavior 11 (3/4), 533–556. Batanero, C., Godino, J., Green, D. & Holmes, P. 1994. Errors and difficulties in understanding elementary statistical concepts. International Journal of Mathematical Education in Science and Technology 25 (4), 527–547. Benson, J. 1989. Structural components of statistical test anxiety in adults: An exploratory model. The Journal of Experimental Education 57, 247–261. Birenbaum, M. & Eylath, S. 1994. Who is afraid of statistics? Correlates of statistics anxiety among students in educational sciences. Educational Research 36, 93–98. Blalock, H. M. Jr. 1987. Some general goals in teaching statistics. Teaching Sociology 15, 164–172. Broers, N. 2001. Analyzing propositions underlying the theory of statistics. Journal of Statistics Education 9 (3). http://www.amstat.org/publications/jse/v9n3/broers.html. Cobb, G. 1992. Teaching statistics. In L. A. Steen (Ed.) Heeding the call for change: Suggestions for curricular action. Washington, DC: Mathematical Association of America. Cruise, R., Cash, R. & Bolton, D. 1985. Development and validiation of an instrument to measure statistical anxiety. Paper presented at the annual meeting of the Statistical Education Section. Proceedings of the American Statistical Assiciation, Washington, DC. Elmore, P. B., Lewis, E. L. & Bay, M. L. 1993. Statistics achievement: A function of attitudes and related experience. Paper presented at the annual meeting of the American Educational Research Association, Atlanta, GA. Forte, J. 1995. Teaching statistics without sadistics. Journal of Social work Education 31 (2), 204–218. Friedaman. H. H., Halpern, M. & Salb, D. 1999. Teaching statistics using humorous anecdotes. Mathematics Teacher 92 (4), 305–308. Gal, I. & Ginsburg, L. 1994. The role of beliefs and attitudes in learning statistics: Towards an assessment framework. Journal of Statistics Education (electronic journal) 2 (2). Garfield, J. 1994. Beyond testing and grading: Using assessment to improve student learning. Journal of Statistics Education (electronic journal) 2 (1). Garfield, J. 1995. How students learn statistics. International Statistics Review 63, 25–34. Garfield, J. 1997. Discussion about the paper of S.D. Moore “New pedagogy and new content”. International Statistical Review 65 (2), 137–141. Garfield, J. B., and Gal, I. 1999. Assessment and statistics education: Current challenges and directions. International Statistical Review 67, 1–12. Green, K. E. 1994. Affective components of attitude and statistics instruction. Paper presented at the Annual Meeting of the American Educational Research Association, New Orleans, LA. Guastello, E. F. 2000. Concept mapping effects on science content comprehension of low-achieving inner-city seventh graders. Remedial & Special Education 21 (6), 356–364. Hodson, D. 1998. Teaching and learning science: Towards a personalized approach. Buckingham. UK: Open University Press. Khamis, H. 1991. Manual computations – A tool for reinforcing concepts and techniques. The American Statistician 45 (4), 294–299. Kinchin, I. M. From ‘ecologist’ to ‘conceptual ecologist’: The utility of the conceptual ecology analogy for teachers of biology. Journal of Biological Education 34 (4), 178–183. Konold, C. 1995. Issues in Assessing Conceptual Understanding in Probability and Statistics. Journal of Statistics Education 3 (1). www.amstat.org/publications/jse/v3n1/konold.html. Marasinghe, M. G. Meeker, W. Q. 1996. Using graphics and simulation to teach statistical concepts. American Statistician 50 (4), 342–351. McLeod, D. B. 1992. Research on affect in mathematics education: A reconceptualization. In D. A. Grouws (Ed.) Handbook of Research on Mathematics Teaching and Learning. New York: Macmillan, 575–596. McNamarra, T. P. 1994. Knowledge representation. In R. J. Sternberg (Ed.) Thinking and problem solving. San Diego;: Academic Press. Mintzes, J. J., Wandersee, J. H. & Novak, J. D. 2001. Assessing understanding in biology. Journal of Biological Education. 35 (3), 118–124. Moore, D. S. 1993. The place of video in new styles of teaching and learning statistics. The American Statistician 47 (2), 172–176. Moore, D. S. 1997. New pedagogy and new content: The case of statistics. International Statistical Review 65 (2), 123– 165. Moore, D. S. & Cobb, G. W. 1995. Statistics education fin de siecle. American Statistician 49 (3), 250–260. Nolan, D. & Speed, T. P. 1999. Teaching statistics theory trough applications. American Statistician 53 (4), 370–375. Novak, J. D. & Gowin, D. B. 1984. Learning how to learn. New York: Cambridge University Press. Novak, J. D., Gowin, D. B. & Johansen, G. T. 1983. The use of concept mapping and knowledge via mapping with junior high school science students. Science Education 67 (5), 625–645. 12</p><p>Oughton, J. & Reed, W. 2000. The effect of hypermedia knowledge and learning style on student-centered concept maps about hypermedia. Journal of Research on Computing in Education 32 (3), 366–384. Onwuegbuzie, A. J. & Seaman, M. 1995. The effect of time and anxiety on statistics achievement. Journal of Experimental Psychology 63, 115–124. Onwuegbuzie A. J. 1999. Statistics anxiety among African American graduate students: An affective filter? Journal of Black Psychology 25 (2), 189–209. Onwuegbuzie, A. J., DaRos, D. & Ryan, D. 1997. The components of statistics anxiety: A phenomenological study. Focus on Learning Problems in mathematics 19 (4), 11–35. Parkes, J., Zimmaro, D. M., Zappe, S. M. & Suen, H. K. 2000. Reducing task-related variance in performance assessment using concept maps. Educational Research and Evaluation 6 (4), 357–378. Pintrich, P. R., Marx, R. W. & Boyle, R. A. 1993. Beyond cold conceptual change: The role of motivational beliefs and classroom contextual factors in the process conceptual change. Review of Educational Research 63, 167–199. Roberts, L. 1999. Using concept maps to measure statistical understanding. International Journal of Mathematical Education in Science & Technology 30 (5) 707–717. Royse, D. & Rompf, E. L. 1992. Math anxiety: A comparison of social work and non-social work students. Journal of Social Work Education 28, 270–277. Rubin, A. 192. Education for research utilization in BSW programs. In A. J. Grasso & I. Epstein (Eds.) Research utilization in the social services. New York: Haworth Press, 369–392. Schau, C. & Mattern, N. 1997. Assessing students' connected understanding of statistical relationships. In I. Gal and J. B. Garfield (Eds.) The Assessment Challenge in Statistics Education. Amsterdam: IOS Press, 91–104. Schacht, S. 1991. What does Opus know about statistics? The cartoon technique for reducing student anxiety. In L. R. Gaydosh (Ed.) Syllabi and instructional material for social statistics. Washington, DC: American Sociological Association Teaching Resources Center, 55–57. Schutz, P. A., Drogosz, L. M., White, V. E. & Distefano, C. 1998. Prior knowledge, attitude, and strategy use in an introduction to statistics course. Learning and Individual Differences 10 (4), 291–307. Shavelson, R., Lang, H. & Lewin, B. 1993. On concept maps as potential “authentic” assessments in science. (ERIC Document Reproduction Service No. ED 367691. Shavelson & Ruiz-Primo 2000). Shavelson, R. & Ruiz-Primo, M. 2000 On the psychometrics of assessing science understanding. In J. J. Mintzes, J. H. Wandersee & J. D: Novak (Eds.) Assessing science understanding: A human constructivist view. San Diego, CA: Academic Press. Tobias, S. 1995. Overcoming math anxiety. New York: W.W. Norton & Co Inc. Townsend, M., Moore, D., Tuck, B. & Wilton, K. 1998. Self-concept and anxiety in university students studying social science statistics within a co-operative learning structure. Educational Psychology 18 (1), 41–53. Townsend, M. & Moore, D. 1994. Cooperative group versus individual evaluations of instruction in professional in- service training. Australian Journal of Education 38, 139–147. Velleman, P. F. & More, D. S. 1996. Multimedia for teaching statistics: Promises and pitfalls. The American Statistician 50 (3), 217–225. Watts, M. & Carlson, W. L. 1999. A case method for teaching statistics. Journal of Economic Education 30 (1), 52–58. Williams, C.G. 1998. Using concept maps to assess conceptual knowledge function. Journal of Research Mathematics Education. 29 (4), 414–421. Yilmaz, M. R. 1996. The Challenge of teaching statistics to non-specialists. Journal of Statistics Education [Online], 4 (1) www.amstat.org/publications/jse/v4n1/yilmaz.html. Zeidner, M. 1991. Statistics and mathematics anxiety in social science students – some interesting parallels. British Journal of Educational Psychology 61, 319–328. Zimmaro, D. M., Zappe, S. M., Parkes, J. T. & Suen, H. K. 1999. Validation of cooncept maps as a representation of structural knowledge. Paper presented at the Annual Meeting of the American Educational Research Association, Montreal, Quebec, Canada. 13</p><p>Appendix 1. The solutions of principal axis factoring (oblim rotation) based on the questionnaire used in the beginning of the course (structure matrix)</p><p>Avoidance Statistics Mathematical Maths Self- anxiety self-confidence anxiety efficacy The course is ”de rigueur” ,797 ,477 I really want to learn quantitative methods -,793 I think I’ll need quantitative methods in the future -,740 I’m frustrated to quantitative methods and I lack inner motivation to study them ,718 I’m motivated and I’m going to do my best during the course -,716 ,485 I just want to pass the course ,704 ,458 I really want to learn to understand quantitative methods -,703 I’m feeling positive for the course -,692 -,543 ,475 I’m interested in quantitative methods -,666 I try to get a good grade -,589 -,467 It is boring to study quantitative methods ,576 ,390 -,477 The requirements at quantitative methods courses should be lower ,532 ,489 ,422 There are too much quantitative methods in our degree ,522 I’m going to revise my prior knowledge of statistics -,449 There are too many strange concepts and terminology in the teaching of quantitative methods ,444 I think the course will be difficult for me ,788 I feel anxiety and uncertainty how to reach the course aims ,781 ,433 -,506 I’ve heard that the course is difficult and I feel anxiety ,747 I fear I don’t understand the content of the course ,684 ,523 -,529 I’ll resort on my more talented fellow student to pass the course ,440 </p><p>Logical thinking in mathematics and statistics is easy for me ,622 -,560 The course in a possibility for me to show what I learnt ,612 Mathematics is an insuperable barrier for me ,438 ,902 -,407 I’m nervous and uncertain with mathematics ,868 I like mathematics and I don’t have any troubles in learning it ,551 -,638 ,445 Only mathematically talented students are able to pass the course ,428 ,423 ,456 -,435 well I’m able to work individually at the course -,362 -,433 I trust on myself as a learner -,493 -,520 ,744 I trust in my skills to find solutions to course assignments -,435 -,471 ,652 Passing the course depends rather on your attitudes than your skills ,618 It’s only up to me how well I’m doing in this course ,543 I’ll give up easily -,540 I don’t panic if I don’t find the solution immediately ,519 I’m afraid of using computers for assignments already in advance -,367</p><p>14</p><p>Appendix 2. The results of principal axis factoring (oblim rotation) – based on the questionnaire used in the end of the course (structure matrix)</p><p>Positive Learning Test Resorting to Responsibility learning efforts anxiety other experiences students My attitude about the course is more positive than before ,746 ,433 I trust on my understanding of quantitative methods more ,697 ,308 The course was easier than I expected ,669 I learnt easier and better than I expected ,669 The course made me feel low and inferiority -,650 I lost the feeling of controlling myself as a learner during the -,641 course The course enhanced my self-confidence and self-awareness ,626 I felt successful learning experiences and joy of learning ,622 ,366 I think I'm capable and I have enough knowledge to pass the course well ,557 The course was stressful -,551 ,371 The teacher could have made things easier for me to understand -,550 ,393 It's enough for me to pass the course -,519 ,310 -,461 The course was a difficult as I expected -,458 The course was more positive than I expected ,442 I don't have to push hard to pass the course ,437 I didn't spend very much time for this course -,756 -,365 I used a lot of time to utilise different learning styles to understand the idea of statistics ,711 ,363 When I met difficulties, I really tried to solve the problems ,693 ,337 I worked hard to learn the course contents ,687 ,516 I tried to avoid preparing myself for the exam as far as possible -,610 If I didn't understand something, it was because I didn't work hard enough -,568 I something remained unclear to me in the lecture, I clarified it ,553 later I gave up with difficult subjects and concentrated on easier ones -,496 To understand the contents I asked question from myself ,492 I don't panic in an exam, even if it felt difficult -,778 I use to panic in exams ,763 I tried to learn by rote and make rules to learn better ,352 I tried to co-operate with my fellow students at the course ,683 If I didn't understand, I asked help from my fellow students ,359 ,651 I think I won't need what I learnt in the future -,334 ,364 ,385 I'd like to apply what I learnt ,458 ,650 The course was more useful than I expected ,565 ,574 If I don't pass the exam, I'll try harder ,565 I tried to accommodate the new knowledge from this course to my prior knowledge ,450 Passing the course is only on my responsibility ,346 </p>
Details
-
File Typepdf
-
Upload Time-
-
Content LanguagesEnglish
-
Upload UserAnonymous/Not logged-in
-
File Pages14 Page
-
File Size-