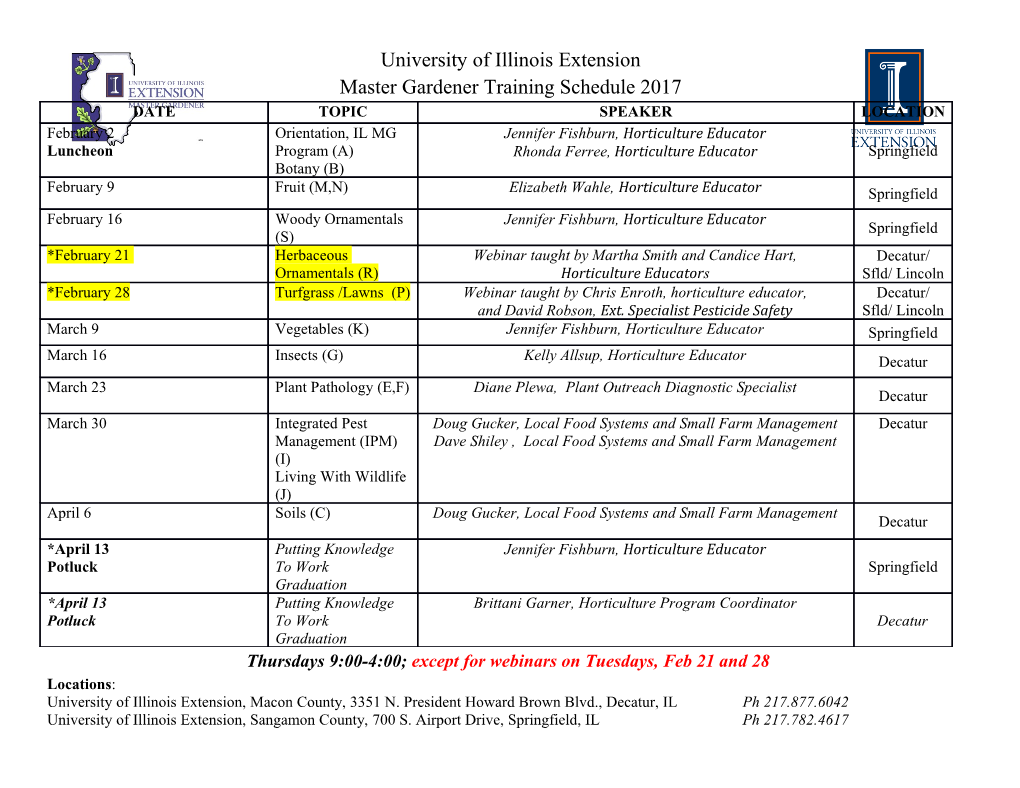
<p>Stat 462 April 5</p><p>1. Use the dataset prostatecancer.txt at www.stat.psu.edu/~rho/462data/. Dataset consists of n = 97 prostate cancer patients y = PSA_level, prostate specific antigen, a blood chemistry measurement affected by x1 x1 = CancerVol, cancer volume x2 = Capsular, a measure of the invasiveness of the cancer</p><p>A. Fit the model E(y) = 0 + 1 x1 + 2 x2 Use the Storage button, Store Deleted t residuals, Hi (leverages), and DFITS, and FITS</p><p>What is the estimated regression equation?</p><p>What is the value of MSE? </p><p>B. Do a dotplot of the Cook’s D values. Use Editor>Brush to help you identify any extreme values. What observation(s) have extreme Cook’s D values?</p><p>C. Explain what (in general) is measured by a Cook’s D value.</p><p>D. Do a dotplot of the DFITS. Identify any “extreme” points. (The book’s criterion for extreme is that absolute DFIT>1.) </p><p>E Explain what (in general) is measured by a DFFIT value.</p><p>F. Do a dotplot of the hi values. Identify any “extreme” points. The Minitab criterion for a large leverage is 3p/n. Use this as the definition of “extreme.” </p><p>G. Do a dotplot of the deleted residuals. Identify any “extreme” data points. </p><p>H. Graph PSA_level versus CancerVol. Identify any unusual points. </p><p>I. Graph PSA_level versus Capsular. Identify any unusual points. J. What is the predicted value for observation 97?</p><p>K. Delete observation 97 by replacing the y-value with an asterisk (by replacing the value of y with an asterisk). Recompute the regression equation. </p><p>What is the predicted value for observation 97? </p><p>What is the difference between this predicted value and the value found in the previous part? Note: This is the “unstandardized” version of a DFIT for observation 97.</p><p>L. In addition to observation 97, delete observations 95 and 96. Re-run the regression, and then repeat part A based on this new regression. Describe differences between the two sets of results. </p><p>3. Use the dataset party200.txt. We’ll use logistic regression to predict the probability that a student would say they have ever driven under the influence of alcohol based on X= how many days per month they say they drink at least two beers. </p><p>The underlying model of logistic regression for one X-variable is e01X p where p = probability of falling into a category of interest (saying yes to having driven 1 e01X under the influence.</p><p>Use Stat>Regression>Binary Logistic Regression. Enter “DrvDrnk” as “Response” enter “DaysBeer” in the Model box, click Storage and click to Store “Event Probabilities” (item is on right side of dialog box).</p><p>Then, use Graph>Plot to plot the stored event probabilities versus DaysBeer.</p><p>A. Based on this plot, estimate the probability of ever having driven under the influence for DaysBeer = 0, DaysBeer=10, and DaysBeer = 20</p><p>B. What are the estimated values of 0 and 1? (See output in session window.) </p><p>C. Use the equation given for the model to calculate predicted probabilities for DaysBeer = 0 and for DaysBeer=10. </p>
Details
-
File Typepdf
-
Upload Time-
-
Content LanguagesEnglish
-
Upload UserAnonymous/Not logged-in
-
File Pages2 Page
-
File Size-