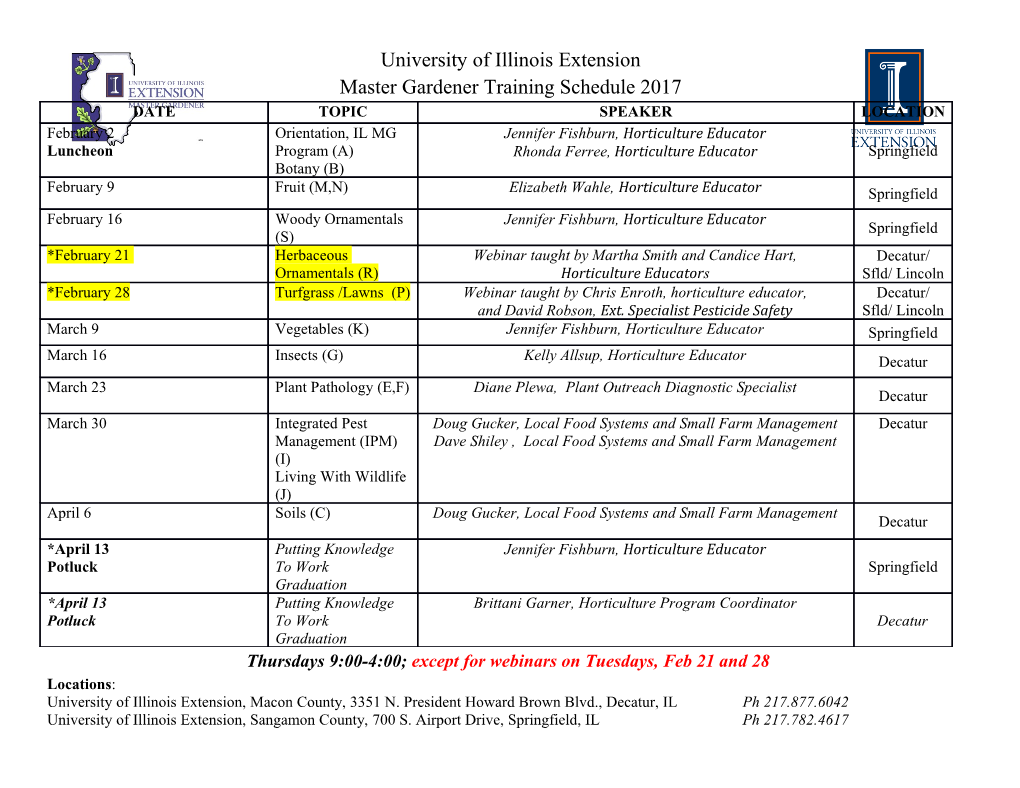
DOCTORAL T H E SIS Lara Lorna Jiménez Decentralized Location-aware Orchestration of Containerized Microservice Applications of Containerized Orchestration Microservice Lara Lorna Jiménez Decentralized Location-aware Department of Computer Science and Electrical Engineering Division of Computer Science ISSN 1402-1544 Decentralized Location-aware ISBN 978-91-7790-617-9 (print) ISBN 978-91-7790-618-6 (pdf) Orchestration of Containerized Luleå University of Technology 2020 Microservice Applications Enabling Distributed Intelligence at the Edge Lara Lorna Jiménez Pervasive and Mobile Computing Decentralized Location-aware Orchestration of Containerized Microservice Applications Enabling Distributed Intelligence at the Edge Lara Lorna Jiménez Luleå University of Technology Department of of Computer Science and Electrical Engineering Division of Computer Science Printed by Luleå University of Technology, Graphic Production 2020 ISSN 1402-1544 ISBN 978-91-7790-617-9 (print) ISBN 978-91-7790-618-6 (pdf) Luleå 2020 www.ltu.se Decentralized Location-aware Orchestration of Containerized Microservice Applications Enabling Distributed Intelligence at the Edge Lara Lorna Jim´enez Dept. of Computer Science and Electrical Engineering Lule˚aUniversity of Technology Sweden Supervisors: Olov Schel´enand K˚areSynnes ii To my parents iii iv Abstract Services that operate on public, private, or hybrid clouds, should always be available and reachable to their end-users or clients. However, a shift in the demand for current and future services has led to new requirements on network infrastructure, service orches- tration, and Quality-of-Service (QoS). Services related to, for example, online-gaming, video-streaming, smart cities, smart homes, connected cars, or other Internet-of-Things (IoT) powered use cases are data-intensive and often have real-time and locality require- ments. These have pushed for a new computing paradigm, Edge computing, based on moving some intelligence from the cloud to the edge of the network to minimize latency and data transfer. This situation has set new challenges for cloud providers, telecommu- nications operators, and content providers. This thesis addresses two issues in this problem area that call for distinct approaches and solutions. Both issues share the common objectives of improving energy-efficiency and mitigating network congestion by minimizing data transfer to boost service perfor- mance, particularly concerning latency, a prevalent QoS metric. The first issue is related to the demand for a highly scalable orchestrator that can manage a geographically distributed infrastructure to deploy services efficiently at clouds, edges, or a combination of these. We present an orchestrator using process containers as the virtualization technology for efficient infrastructure deployment in the cloud and at the edge. The work focuses on a Proof-of-Concept design and analysis of a scalable and resilient decentralized orchestrator for containerized applications, and a scalable monitoring solution for containerized processes. The proposed orchestrator deals with the complexity of managing a geographically dispersed and heterogeneous infrastructure to efficiently deploy and manage applications that operate across different geographical locations | thus facilitating the pursuit of bringing some of the intelligence from the cloud to the edge, in a way that is transparent to the applications. The results show this orchestrator's ability to scale to 20 000 nodes and to deploy 30 000 applications in parallel. The resource search algorithm employed and the impact of location awareness on the orchestrator's deployment capabilities were also analyzed and deemed favorable. The second issue is related to enabling fast real-time predictions and minimizing data transfer for data-intensive scenarios by deploying machine learning models at devices to decrease the need for the processing of data by upper tiers and to decrease predic- tion latency. Many IoT or edge devices are typically resource-scarce, such as FPGAs, ASICs, or low-level microcontrollers. Limited devices make running well-known machine learning algorithms that are either too complex or too resource-consuming unfeasible. Consequently, we explore developing innovative supervised machine learning algorithms v to efficiently run in settings demanding low power and resource consumption, and real- time responses. The classifiers proposed are computationally inexpensive, suitable for parallel processing, and have a small memory footprint. Therefore, they are a viable choice for pervasive systems with one or a combination of these limitations, as they facil- itate increasing battery life and achieving reduced predictive latency. An implementation of one of the developed classifiers deployed to an off-the-shelf FPGA resulted in a pre- dictive throughput of 57.1 million classifications per second, or one classification every 17.485 ns. vi Contents Part I 1 Chapter 1 { Introduction 3 1.1 Motivation and Problem Formulation . .3 1.2 Methodology . .5 1.3 Thesis Outline . .6 Chapter 2 { Research Context 9 2.1 Virtualization . .9 2.2 Recent Computing Paradigms . 11 2.3 Enabling Applications in the Cloud-Edge Continuum . 14 Chapter 3 { Scalable Orchestration and Monitoring of Containers 15 3.1 A Decentralized Container Orchestration Platform . 15 3.1.1 Context Awareness: Location and Latency . 17 3.1.2 Node Identifiers and Discovery . 18 3.1.3 Management of Deployment Objects . 18 3.1.4 Replication and Scaling . 19 3.1.5 Service Discovery and Load Balancing . 20 3.1.6 Scheduling . 21 3.1.7 Multi-tenancy . 23 3.1.8 Distributed Consensus . 23 3.1.9 Other functionalities . 25 3.2 Scalable Monitoring of Container-based Infrastructures . 26 3.3 Use cases . 27 Chapter 4 { Classifiers for resource-constrained devices 31 4.1 Computationally Inexpensive Classifiers for edge and IoT devices . 31 4.2 Use Cases . 34 Chapter 5 { Contributions 35 5.1 Paper A . 35 5.2 Paper B . 36 5.3 Paper C . 36 5.4 Paper D . 37 5.5 Paper E . 38 5.6 Paper F . 39 vii Chapter 6 { Conclusions and Future Work 41 6.1 Conclusions . 41 6.2 Future Work . 44 Part II 53 Paper A 55 1 Introduction . 57 2 Related Work . 59 3 System Architecture . 60 3.1 CoMA: Container Monitoring Agent . 60 3.2 Complementary Components . 62 4 Evaluation . 63 4.1 Validity of CPU and Memory Measurements . 63 4.2 Validity of Block I/O Measurements . 71 5 Discussion . 71 6 Conclusion and Future Work . 72 Paper B 77 1 Introduction . 79 2 DOCMA: an overview . 81 2.1 Applications . 81 2.2 Routing . 82 2.3 Roles . 82 2.4 The DOCMA protocol . 83 3 Deploying an Application . 84 4 Orchestrating an Application . 85 5 Related Work . 86 6 Results and Discussion . 87 7 Conclusions and Future work . 90 Paper C 93 1 Introduction . 95 2 HYDRA System Design . 98 2.1 ID-based Identifier Design . 98 2.2 Node Discovery . 98 2.3 Application Management . 99 2.4 Search for Resources . 99 2.5 Distributed Consensus . 100 3 Location-aware Nodes . 100 4 Applications . 102 5 Roles for Decentralized Orchestration . 102 5.1 The Entry Role . 102 5.2 The Controller Roles: Root and Leaf . 102 viii 5.3 The Service Host Role . 103 5.4 The Roles in Concert . 103 6 Location-aware Application Management . 104 6.1 Type 1 Application Control: Flat . 104 6.2 Type 2 Application Control: Layered . 104 6.3 Application Root ID and Leaf IDs . 106 7 Application Deployment . 107 8 Location-aware Search for Resources . 108 8.1 Random ID . 110 8.2 Maximized XOR Distance between Queried IDs . 110 9 Replication of Services . 110 10 Failure Recovery . 111 10.1 Service Replicas . 112 10.2 Controllers . 112 11 Related Work . 112 12 Experimental Design . 114 13 Experimental Results and Discussion . 120 13.1 Network Scalability . 120 13.2 Application Deployment Scalability . 121 13.3 Performance of the Random Search Algorithm . 123 13.4 Location-aware Deployment . 126 13.5 Location-aware Deployment - One Region Network Partitioned . 127 13.6 HYDRA Design Considerations . 129 14 Conclusions and Future Work . 129 Paper D 135 1 Introduction . 137 1.1 The McCulloch-Pitts neuron model . 138 1.2 Cellular Automata . 138 1.3 Research questions . 139 2 Related work . 140 3 The CAMP algorithm . 141 4 Training of the classifier . 142 4.1 Chromosome encoding . 143 4.2 Fitness calculation . 143 4.3 Selection of individuals . ..
Details
-
File Typepdf
-
Upload Time-
-
Content LanguagesEnglish
-
Upload UserAnonymous/Not logged-in
-
File Pages199 Page
-
File Size-