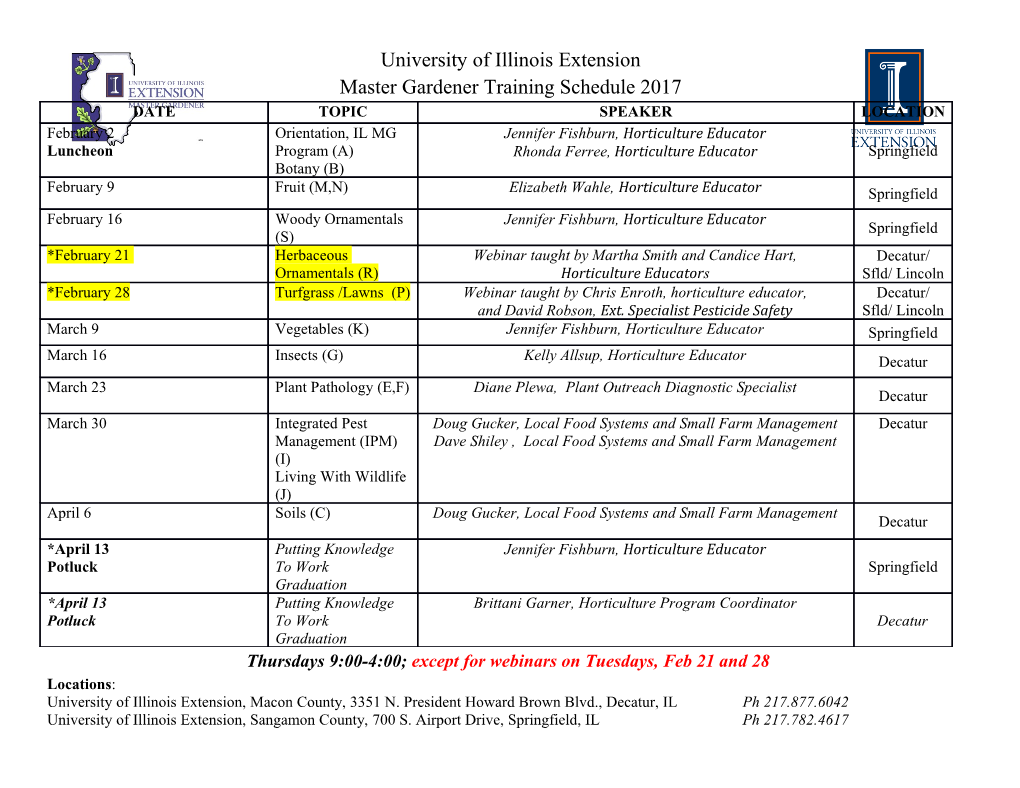
Supplementary Information Promoter hypomethylation of EpCAM-regulated bone morphogenetic protein genes in advanced endometrial cancer Ya-Ting Hsu, Fei Gu, Yi-Wen Huang, Joseph Liu, Jianhua Ruan, Rui-Lan Huang, Chiou-Miin Wang, Chun-Liang Chen, Rohit R. Jadhav, Hung-Cheng Lai, David G. Mutch, Paul J. Goodfellow, Ian M. Thompson, Nameer B. Kirma, and Tim Hui-Ming Huang Tables of contents Page Table of contents 2 Supplementary Methods 4 Supplementary Figure S1. Summarized sequencing reads and coverage of MBDCap-seq 8 Supplementary Figure S2. Reproducibility test of MBDCap-seq 10 Supplementary Figure S3. Validation of MBDCap-seq by MassARRAY analysis 11 Supplementary Figure S4. Distribution of differentially methylated regions (DMRs) in endometrial tumors relative to normal control 12 Supplementary Figure S5. Network analysis of differential methylation loci by using Steiner-tree analysis 13 Supplementary Figure S6. DNA methylation distribution in early and late stage of the TCGA endometrial cancer cohort 14 Supplementary Figure S7. Relative expression of BMP genes with EGF treatment in the presence or absence of PI3K/AKT and Raf (MAPK) inhibitors in endometrial cancer cells 15 Supplementary Figure S8. Induction of invasion by EGF in AN3CA and HEC1A cell lines 16 Supplementary Figure S9. Knockdown expression of BMP4 and BMP7 in RL95-2 cells 17 Supplementary Figure S10. Relative expression of BMPs and BMPRs in normal endometrial cell and endometrial cancer cell lines 18 Supplementary Figure S11. Microfluidics-based PCR analysis of EMT gene panel in RL95-2 cells with or without EGF treatment 19 Supplementary Figure S12. Knockdown expression of EpCAM by different shRNA sequences in RL95-2 cells 20 Supplementary Figure S13. Proposed model for EpICD mediated regulation of BMP genes by EGF 21 2 Supplementary Table 1. Characteristics of endometrial cancer patients 22 Supplemental Table 2. Summary of sequencing reads in endometrial tumors an normal controls 23 Supplementary Table 3. Differentially methylated promoter CpG islands in recurrent relative to non-recurrent tumors 26 Supplementary Table 4. List of primer sequences 55 Supplementary Table 5. List of siRNA and shRNA sequences 59 Supplementary References 60 3 Supplementary Methods MassARRAY analysis To quantify methylation levels of CpG islands in clinical samples, the high-throughput MassARRAY platform (Sequenom) was carried out as described previously (Huang et al. 2009). Briefly, DNA was bisulfite-converted and subjected to PCR and base-specific cleavage. The cleaved DNA was analyzed by MALDI-TOF mass spectrometry. Methylation data of HAAO, RXFP3, and miR-129-2 CpG islands were published previously (Huang et al. 2009, 2010). Definition of methylation types All known transcripts were divided into 1) promoter CpG island located within 2-kb up- or downstream of its TSS and 2) promoter non-CpG island located within 2-kb windows of its TSS. Other regions were defined as intragenic CpG island located after 2-kb downstream of a TSS and before 3’-UTR and intergenic CpG island located before >2-kb upstream of a TSS or after 3’-UTR. Bioinformatics analysis Sequence reads mapped to unique genome locations were normalized to the sum of unique reads and other reads (e.g., repeat sequences). Unique reads were collected after normalization and directionally extended to 200-bp. The methylation level of each bin size was calculated by accumulating the reads number if the read was located in the bin. Linear normalization method based on the unique mapped read number was used to normalize methylation enrichment: , , /^ 4 th Where , is the normalized read number of the i bin, and , is the uniquely mapped th read number of the i bin, is the total uniquely mapped reads number. “INT” function rounds the element (in the parenthesis) to the nearest integers towards minus infinity, “^” means the power operator. For two groups A and B, the sample number is for group A, and for group B. For a given region R (which includes m bin size, and start at the sth bin), the average methylation level is ∑ , , , ,,…, for group A or B (if is A or B). In equation 2, , means the average methylation level of group at region , , is the methylation levels of each sample of group at region . We then used paired t-test to compare if the methylation level of the region was significantly different between those two groups. Differentially methylated loci between endometrial carcinoma samples and normal controls and between recurrent and non-recurrent tumor groups were analyzed by the above algorithm. Network analysis of differential methylation loci by using Steiner-tree analysis Differentially methylated loci were mapped to a human protein-protein interaction (PPI) network (Wu G, Feng X, & Stein L. 2010) to identify a small number of connector genes that can link these loci into a singly connected sub-network. These connector genes were identified with the well-known Steiner-tree algorithm (Huang SS & Fraenkel E. 2009). Formally, the Steiner- tree for an edge-weighted graph G = (V, E, W) and a subset of vertices S ⊆V is a minimum- weight connected tree T, with vertices U⊆V and edges D⊆E that spans all vertices in S. Here the vertices in S are known as terminal vertices and UnS as Steiner vertices. For an un- weighted graph G, the problem then becomes to find the minimum number of vertices that can connect all the vertices in R through a tree in G. The Steiner tree problem is NP-hard and 5 therefore a polynomial-time 2-approximation shortest path heuristic algorithm of Steiner-tree problem was used (Huang SS & Fraenkel E. 2009). To de-noise and to increase the probability of covering all core members in the pathways and alternative functional links between pathways, a simple randomized algorithm was designed to obtain multiple Steiner trees with suboptimal quality (i.e., slightly more connector genes) (Huang SS & Fraenkel E. 2009). This procedure was repeated multiple times with different random weights, until the total number of unique Steiner vertices converges approximately. In this work, the rate of new coming Steiner vertices reduced significantly after 10 iterations. The Steiner vertices in the 10 Steiner trees were pooled to obtain connector genes, and a sub-network containing connectors and differentially methylated loci was extracted from the PPI network. The connectors were further analyzed for enriched KEGG pathways using the online functional annotation tool DAVID (Huang de W, Sherman BT, Lempicki RA. 2009). DNA methylation distribution and survival analysis of BMP genes in the TCGA cohort All TCGA cohort array and clinical data were downloaded from the TCGA website (http://www.cancergenome.nih.gov/) and the TCGA endometrial data snapshot website (http://23.23.224.116/ucec_tcga/). To compare DNA methylation levels in recurrent/progression group with non-recurrent group, we filtered patients who have clinical information provided with recurrent/progression status, survival and DNA methylation data as the final cohort for analysis. In this cohort, 39 patients showed primary tumors with recurrence/progression and 193 patients were non-recurrent. Recurrent rate is 16 % (39/232=0.16). We further filtered the DNA methylation data by only looking at probes located in the 2-kb region around transcription start site (TSS) with CpG island annotation or differential methylation regions identified in BMP2, BMP3, BMP4 and BMP7 in our own cohort. For progression free survival analysis, 15 percentile was used as the cutoff to dichotomize the DNA methylation level into high (n=197) and low methylation (n=35) in each of BMPs probe. The low methylation of BMP4 and BMP7 6 showed a significant association with poor survival (Both of P < 0.01) by Kaplan–Meier curve analysis. For the methylation distribution in disease stage, stage I and stage II were combined as early stage (n=153) and stage III and IV were designated as late stage (n=33). The methylation distributions of BMP genes were illustrated as representative figures showing one probe, corresponding to the same CpG site shown in Fig. 3A. lower panel. PI3K/AKT and Raf (MAPK) inhibitors treatment RL95-2 cells were grown in DMEM culture medium (Life Technologies, Grand Island, NY) supplemented with 10% fetal bovine serum (FBS) and 1% Penicillin/Streptomycin (Life Technologies) at 37oC. One day before the treatment, media was changed to DMEM supplemented with 10% charcoal stripped-heat inactivated (CSHI) FBS for treatments. PI3K/AKT inhibitor LY 294002 (4 uM, EMD Chemicals, Germany) and Raf (MAPK) inhibitor PLX-4720 (2uM, Selleck Chemicals, Houston, TX) were added with or without EGF (10 ng/mL, Peprotech, Rocky Hill, NJ) for 72 hours. RNA was then isolated using Direct-zolTM RNA MiniPrep according to the manufacturer’s manual (Zymo Research, Irvine, CA). 7 B Promoter region Whole genome (2-kb centered on TSS) (1-kb sliding window) (n=21,853) (n=3,022,656) No 1 No 1 coverage 2-4 coverage 2-4 ≥50 5-9 ≥50 Endometrial normal 5-9 25-49 25-49 10-24 10-24 No coverage 1 No 1 2-4 2-4 coverage ≥50 ≥50 Endometrial tumor 5-9 5-9 25-49 25-49 10-24 10-24 Supplementary Figure S1. Summarized sequencing reads coverage of MBDCap-seq. (A) Summarized sequencing reads in normal samples (n=10) and endometrial tumors (n=67). Each bar represents an individual sample. Blue and red bars indicate unique reads and repeat sequences, respectively. (B) Genomic coverage was quantified by the number of DNA methylation measurements that overlap with gene promoters and a 1-kb tiling of the genome. 8 Each read was extended to the mean length obtained during size selection. The number of measurements was equal to the number of unique sequencing reads that fall inside each region. Promoter regions were based on the human reference sequence (hg18; NCBI Build 36.1) and covered 1-kb upstream and 1-kb downstream of the annotated transcription start site.
Details
-
File Typepdf
-
Upload Time-
-
Content LanguagesEnglish
-
Upload UserAnonymous/Not logged-in
-
File Pages61 Page
-
File Size-