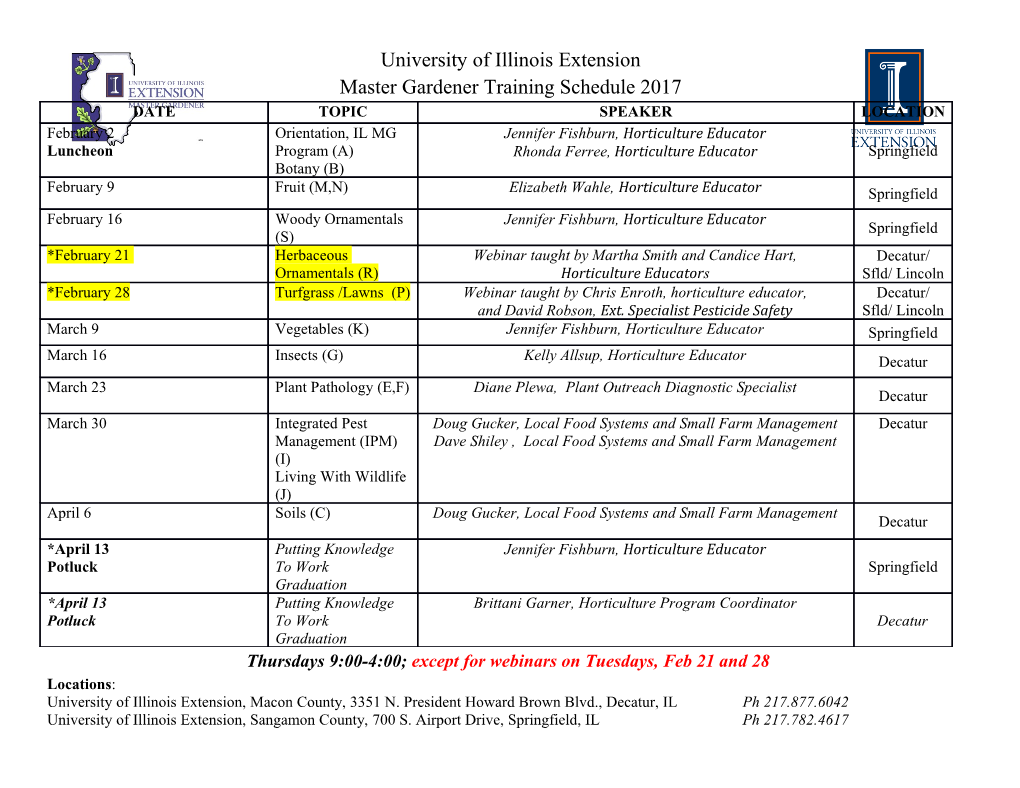
Distributed Data Stream Processing and Edge Computing: A Survey on Resource Elasticity and Future Directions Marcos Dias de Assuncao, Alexandre da Silva Veith, Rajkumar Buyya To cite this version: Marcos Dias de Assuncao, Alexandre da Silva Veith, Rajkumar Buyya. Distributed Data Stream Pro- cessing and Edge Computing: A Survey on Resource Elasticity and Future Directions. Journal of Net- work and Computer Applications (JNCA), Elsevier, 2018, 103, pp.1-17. 10.1016/j.jnca.2017.12.001. hal-01653842 HAL Id: hal-01653842 https://hal.inria.fr/hal-01653842 Submitted on 2 Dec 2017 HAL is a multi-disciplinary open access L’archive ouverte pluridisciplinaire HAL, est archive for the deposit and dissemination of sci- destinée au dépôt et à la diffusion de documents entific research documents, whether they are pub- scientifiques de niveau recherche, publiés ou non, lished or not. The documents may come from émanant des établissements d’enseignement et de teaching and research institutions in France or recherche français ou étrangers, des laboratoires abroad, or from public or private research centers. publics ou privés. Distributed Data Stream Processing and Edge Computing: A Survey on Resource Elasticity and Future Directions Marcos Dias de Assunc¸ao˜ a,∗, Alexandre da Silva Veitha, Rajkumar Buyyab aInria, LIP, ENS Lyon, France bThe University of Melbourne, Australia Abstract Under several emerging application scenarios, such as in smart cities, operational monitoring of large infrastructure, wearable assistance, and Internet of Things, continuous data streams must be processed under very short delays. Several solutions, including multiple software engines, have been developed for processing unbounded data streams in a scalable and efficient manner. More recently, architecture has been proposed to use edge computing for data stream processing. This paper surveys state of the art on stream processing engines and mechanisms for exploiting resource elasticity features of cloud computing in stream processing. Resource elasticity allows for an application or service to scale out/in according to fluctuating demands. Although such features have been extensively investigated for enterprise applications, stream processing poses challenges on achieving elastic systems that can make efficient resource management decisions based on current load. Elasticity becomes even more challenging in highly distributed environments comprising edge and cloud computing resources. This work examines some of these challenges and discusses solutions proposed in the literature to address them. Keywords: Big Data, Stream processing, Resource elasticity, Cloud computing 1. Introduction streams to detect patterns, identify failures [6], and gain insights. The increasing availability of sensors, mobile phones, Several stream processing frameworks and tools have and other devices has led to an explosion in the volume, been proposed for carrying out analytical tasks in a variety and velocity of data generated and that requires scalable and efficient manner. Many tools employ a analysis of some type. As society becomes more inter- dataflow approach where incoming data results in data connected, organisations are producing vast amounts of streams that are redirected through a directed graph data as result of instrumented business processes, mon- of operators placed on distributed hosts that execute itoring of user activity [1,2], wearable assistance [3], algebra-like operations or user-defined functions. Some website tracking, sensors, finance, accounting, large- frameworks, on the other hand, discretise incoming scale scientific experiments, among other reasons. This data streams by temporarily storing arriving data during data deluge is often termed as big data due to the chal- small time windows and then performing micro-batch lenges it poses to existing infrastructure regarding, for processing whereby triggering distributed computations instance, data transfer, storage, and processing [4]. on the previously stored data. The second approach A large part of this big data is most valuable when aims at improving the scalability and fault-tolerance of it is analysed quickly, as it is generated. Under sev- distributed stream processing tools by handling strag- eral emerging application scenarios, such as in smart gler tasks and faults more efficiently. cities, operational monitoring of large infrastructure, Also to improve scalability, many stream processing and Internet of Things (IoT) [5], continuous data frameworks have been deployed on clouds [7], aiming streams must be processed under very short delays. In to benefit from characteristics such as resource elastic- several domains, there is a need for processing data ity. Elasticity, when properly exploited, refers to the ability of a cloud to allow a service to allocate additional ∗Corresponding author: [email protected] resources or release idle capacity on demand to match Preprint submitted to Elsevier November 30, 2017 the application workload. Although efforts have been future directions on the topic and finally, Section8 con- made towards making stream-processing more elastic, cludes the paper. many issues remain unaddressed. There are challenges regarding the placement of stream processing tasks on 2. Background and Architecture available resources, identification of bottlenecks, and application adaptation. These challenges are exacer- This section describes background on stream- bated when services are part of a larger infrastructure processing systems for big-data. It first discusses how that comprises multiple execution models (e.g. lambda layered real-time architecture is often organised and architecture, workflows or resource-management bind- then presents a historical summary of how such systems ings for high-level programming abstractions [8,9]) or have evolved over time. hybrid environments comprising both cloud and edge computing resources [10, 11]. 2.1. Online Data Processing Architecture More recently, software frameworks [12, 13] and ar- Architecture for online1 data analysis is generally chitectures have been proposed for carrying out data multi-tiered systems that comprise many loosely cou- stream processing using constrained resources located pled components [15, 16, 17]. While the reasons for at the edge of the Internet. This scenario introduces structuring architecture in this way may vary, the main additional challenges regarding application scheduling, goals include improving maintainability, scalability, and resource elasticity, and programming models. This arti- availability. Figure1 provides an overview of com- cle surveys stream-processing solutions and approaches ponents often found in a stream-processing architec- for deploying data stream processing on cloud comput- ture. Although an actual system might not have all these ing and edge environments. By so doing, it makes the components, the goal here is to describe how a stream following contributions: processing architecture may look like and position the stream processing solutions discussed later. • It reviews multiple generations of data stream pro- The Data Sources (Figure1) that require timely pro- cessing frameworks, describing their architectural cessing and analysis include Web analytics, infrastruc- and execution models. ture operational monitoring, online advertising, social media, and IoT. Most Data Collection is performed by • It analyses and classifies existing work on exploit- tools that run close to where the data and that commu- ing elasticity to adapt resource allocation to match nicate the data via TCP/IP connections, UDP, or long- the demands of stream processing services. Previ- range communication [18]. Solutions such as JavaScript ous work has surveyed stream processing solutions Object Notation (JSON) are used as a data-interchange without a focus on how resource elasticity is ad- format. For more structured data, wire protocols such dressed [14]. The present work provides a more in- as Apache Thrift [19] and Protocol Buffers [20], can be depth analysis of existing solutions and discusses employed. Other messaging protocols have been pro- how they attempt to achieve resource elasticity. posed for IoT, some of which are based on HTTP [5]. • It discusses ongoing efforts on resource elasticity Most data collection activities are executed at the edges for data stream processing and their deployment on of a network, and some level of data aggregation is often edge computing environments, and outlines future performed via, for instance Message Queue Telemetry directions on the topic. Transport (MQTT), before data is passed through to be processed and analysed. The rest of this paper is organised as follows. Section An online data-processing architecture can comprise 2 provides background information on big-data ecosys- multiple tiers of collection and processing, with the con- tems and architecture for online data processing. Sec- nection between these tiers made on an ad-hoc basis. tion3 describes existing engines and other software so- To allow for more modular systems, and to enable each lutions for data stream processing whereas Section4 tier to grow at different paces and hence accommo- discusses managed cloud solutions for stream process- date changes, the connection is at times made by mes- ing. In Section5 we elaborate on how existing work sage brokers and queuing systems such as Apache Ac- tries to tackle aspects of resource elasticity for data tiveMQ [21], RabbitMQ [22] and Kestrel [23], publish- stream processing. Section6 discusses
Details
-
File Typepdf
-
Upload Time-
-
Content LanguagesEnglish
-
Upload UserAnonymous/Not logged-in
-
File Pages25 Page
-
File Size-