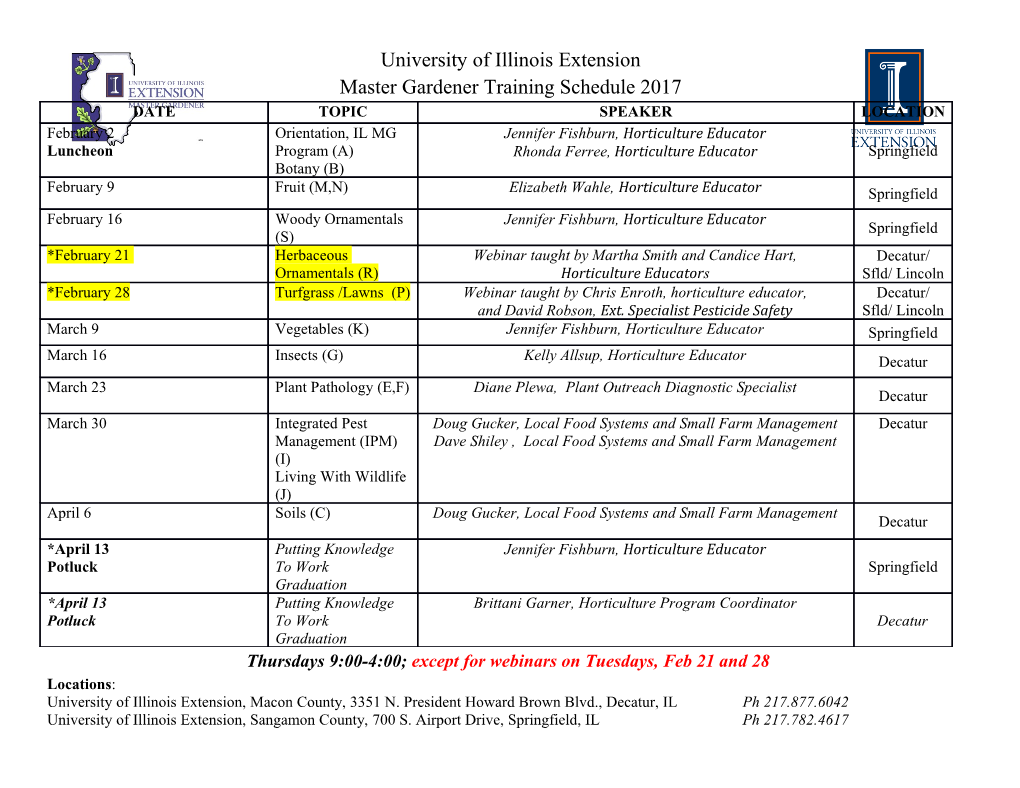
bioRxiv preprint doi: https://doi.org/10.1101/2021.06.03.446950; this version posted June 3, 2021. The copyright holder for this preprint (which was not certified by peer review) is the author/funder, who has granted bioRxiv a license to display the preprint in perpetuity. It is made available under aCC-BY-NC-ND 4.0 International license. Discovery, Article A fast, general synteny detection engine Joseph B. Ahrens1*, Kristen J. Wade1 and David D. Pollock1* 1Department of Biochemistry and Molecular Genetics, University of Colorado Denver, Anschutz Medical Campus, Denver, Colorado, USA * Corresponding authors Joseph B. Ahrens E-mail: [email protected] David D. Pollock E-mail: [email protected] 1 bioRxiv preprint doi: https://doi.org/10.1101/2021.06.03.446950; this version posted June 3, 2021. The copyright holder for this preprint (which was not certified by peer review) is the author/funder, who has granted bioRxiv a license to display the preprint in perpetuity. It is made available under aCC-BY-NC-ND 4.0 International license. Abstract The increasingly widespread availability of genomic data has created a growing need for fast, sensitive and scalable comparative analysis methods. A key aspect of comparative genomic analysis is the study of synteny, co-localized gene clusters shared among genomes due to descent from common ancestors. Synteny can provide unique insight into the origin, function, and evolution of genome architectures, but methods to identify syntenic patterns in genomic datasets are often inflexible and slow, and use diverse definitions of what counts as likely synteny. Moreover, the reliable identification of putatively syntenic regions (i.e., whether they are truly indicative of homology) with different lengths and signal to noise ratios can be difficult to quantify. Here, we present Mology, a fast, flexible, alignment-free, nonparametric method to detect regions of syntenic elements among genomes or other datasets. The core algorithm operates on consecutive, rank-ordered elements, which could be genes, operons, motifs, sequence fragments, or any other orderable element. It is agnostic to the physical distance between distinct elements and also to directionality and order within syntenic regions, although such considerations can be addressed post hoc. We describe the underlying statistical theory behind our analysis method, and employ a Monte Carlo approach to estimate the false positive rate and positive predictive values for putative syntenic regions. We also evaluate how varying amounts of noise affect recovery of true syntenic regions among Saccharomycetaceae yeast genomes with up to ~100 million years of divergence. We discuss different strategies for recursive application of our method on syntenic regions with sparser signal than considered here, as well as the general applicability of the core algorithm. Introduction When biological objects have shared ancestry, they are said to be homologous. DNA and protein sequence homology can be inferred based on alignment and evaluation of sequence similarity, but over evolutionary time the accumulation of insertions, deletions, and mutations make accurate alignments infeasible. The structural arrangement of sequences along genomes may be retained longer, and can provide independent information about homology and the evolution of genomes. One useful structural evolutionary signal is conserved clustering of genes among divergent genomes, called synteny. The term ‘synteny’ was originally used to denote the presence of multiple genetic loci on the same chromosome (Renwick 1971; Nadeau 1989), but now often refers to a common localization or ordering of identifiable genetic elements among genomes that is associated with homology. Given this relationship between shared ancestry and organization, synteny provides an excellent framework through which to understand genome evolution (Hurst et al. 2004; Oliver and Misteli 2005; Splinter et al. 2006; Batada and Hurst 2007; Michalak 2008; Xu et al. 2009). However, the biological underpinnings driving selection for genome architecture are quite complex and can inhibit the detection of meaningful syntenic signal. Factors that affect the evolution of synteny include effective population size (Lunter et al. 2006; Molina and van Nimwegen 2008; Koonin and Wolf 2010; Weber and Hurst 2011), large paralogous gene families (Fischer et al. 2001; Innan and Kondrashov 2010; Sugino and Innan 2012), genomic scope in terms of macro vs. micro synteny (Putnam et al. 2007; Putnam et al. 2008; Srivastava et al. 2008), presence of structural elements such as nucleosomes and chromatin looping (Oliver and Misteli 2005; Splinter et al. 2006; Batada and Hurst 2007; Spitz and Duboule 2 bioRxiv preprint doi: https://doi.org/10.1101/2021.06.03.446950; this version posted June 3, 2021. The copyright holder for this preprint (which was not certified by peer review) is the author/funder, who has granted bioRxiv a license to display the preprint in perpetuity. It is made available under aCC-BY-NC-ND 4.0 International license. 2008; Tena et al. 2011; Bagadia et al. 2016), genome rearrangement events (Fischer et al. 2006; Denoeud et al. 2010), intergene distance (Poyatos and Hurst 2007), and variable regulatory constraints acting on adjacent genes (Hurst et al., 2002; Kensche et al., 2008; Wang et al., 2011, Woolfeet al., 2005; Royo et al., 2011; Tena et al., 2011; Irimia et al., 2012). The biological factors that affect evolution of synteny impact how it should be detected and evaluated. In particular, it is useful to compare synteny detection to sequence alignment, which has the same aim to use similarity to infer homology, but differs in its assumptions of how the underlying elements are structured and how they change over time. In sequence alignment, the number of character states is limited to 4 for nucleotide sequences and 20 for amino acid sequences, while synteny analysis may involve tens of thousands of different genes. Furthermore, the states of nucleotides and amino acids are relatively easy to identify, whereas the gene categories used in synteny analysis may be uncertain. Because of the limited number of states, nucleotide and protein sequences tend to exhibit frequent homoplasy (independent evolution to the same state along different lineages), while recurrent evolution to the same gene category is rare. In contrast, gene order and clustering may be more malleable than nucleotide and amino acid rearrangement within sequences. Because of this, most sequence alignment algorithms assume collinearity (that the ordering of sites in homologous sequences is conserved), while for synteny analysis the collinearity assumption may sometimes be unrealistic (Fischer et al. 2006; Denoeud et al. 2010). Given the markedly different assumptions one can make when comparing large genomic regions, as opposed to sequences within individual genes, there is growing enthusiasm for improved synteny detection methods that scale well for genomic data (Armstrong et al. 2019). The characterization of synteny is confounded by a multitude of synteny concepts in the literature, many of which are incongruent with one another, pertain to synteny occurring at vastly different scales, and entail different analytical methods. Durand and Sankoff (2003) defined “gene clusters” as sets of genetic loci that are all contained within a short interval on a chromosome. Raghupathy and Durand (2009) then showed that expected frequencies of gene clusters in genomic datasets can be approximated—assuming that the genes are randomly ordered—if the copy numbers of all genes are equal (that is, that each gene occurs exactly 2, 3, 4, etc. times within the dataset). However, calculating the expected number of gene clusters in the general case appears to be computationally intractable (Durand and Sankoff 2003; Raghupathy and Durand 2009). Luc et al. (2002) described an algorithm to identify what they term “gene teams” (or “δ-teams”) which differ from the above gene clusters in that adjacent genes must be within a certain sequence distance (δ), while the entire team may be arbitrarily large. He and Goldwasser (2005) extended this algorithm by removing the constraint that each gene can only occur once on a chromosome, referring to the more general patterns as “homology teams”. Gene teams and homology teams are notably similar to the “max-gap clusters” from pairwise genomic datasets described by Hoberman et al. (2005), who also discuss methods for calculating the expected number of such clusters, assuming a pairwise genomic dataset (where each gene has exactly one matching predicted homolog). The gene clusters described by Durand and Sankoff pertain to synteny on a small scale (i.e., “microsynteny”). In contrast, the homology teams of He and Goldwassser (2005) may characterize synteny at larger scales, as they may contain any number of genes. However, the relative gene ordering among putatively syntenic homology teams may be completely different, and to our knowledge, there 3 bioRxiv preprint doi: https://doi.org/10.1101/2021.06.03.446950; this version posted June 3, 2021. The copyright holder for this preprint (which was not certified by peer review) is the author/funder, who has granted bioRxiv a license to display the preprint in perpetuity. It is made available under aCC-BY-NC-ND 4.0 International license. are no easy methods for calculating expected numbers of gene teams, homology teams or max-gap clusters in the general case (Hoberman et al. 2005; Raghupathy and Durand 2009).
Details
-
File Typepdf
-
Upload Time-
-
Content LanguagesEnglish
-
Upload UserAnonymous/Not logged-in
-
File Pages24 Page
-
File Size-