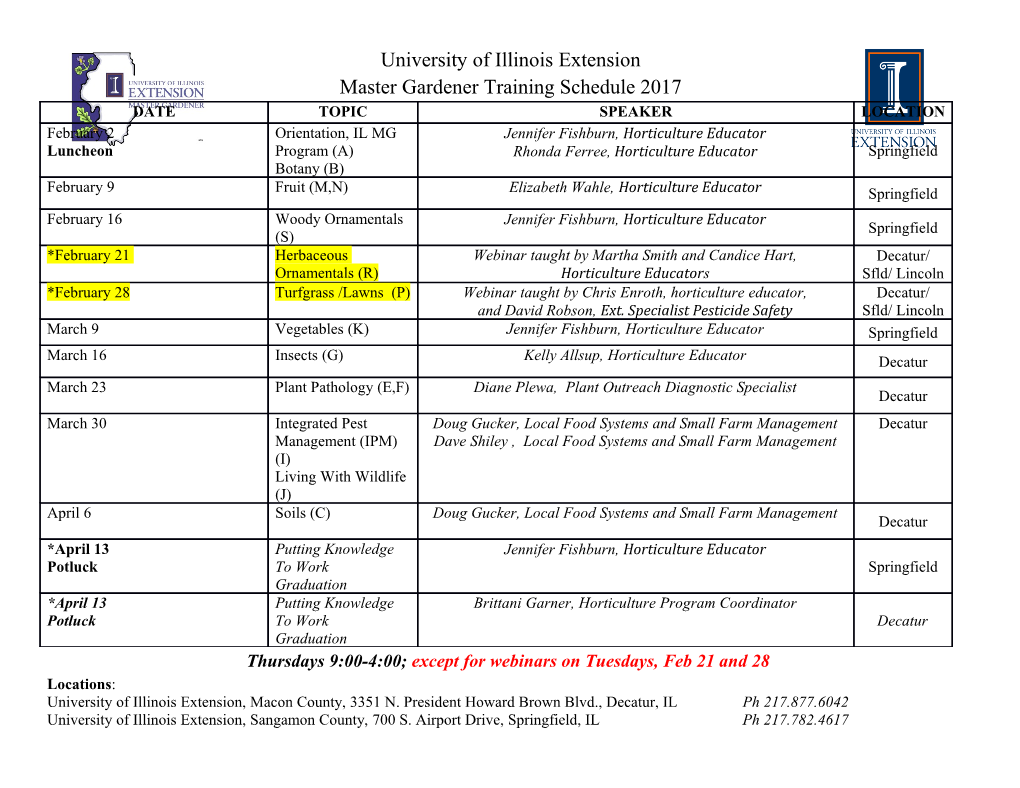
On the Sentence Embeddings from Pre-trained Language Models Bohan Liy;z∗, Hao Zhouy, Junxian Hez, Mingxuan Wangy, Yiming Yangz, Lei Liy yByteDance AI Lab zLanguage Technologies Institute, Carnegie Mellon University {zhouhao.nlp,wangmingxuan.89,lileilab}@bytedance.com {bohanl1,junxianh,yiming}@cs.cmu.edu Abstract 2019) – for example, they even underperform the GloVe (Pennington et al., 2014) embeddings which Pre-trained contextual representations like are not contextualized and trained with a much sim- BERT have achieved great success in natu- pler model. Such issues hinder applying BERT ral language processing. However, the sen- tence embeddings from the pre-trained lan- sentence embeddings directly to many real-world guage models without fine-tuning have been scenarios where collecting labeled data is highly- found to poorly capture semantic meaning of costing or even intractable. sentences. In this paper, we argue that the se- In this paper, we aim to answer two major ques- mantic information in the BERT embeddings tions: (1) why do the BERT-induced sentence em- is not fully exploited. We first reveal the the- beddings perform poorly to retrieve semantically oretical connection between the masked lan- similar sentences? Do they carry too little semantic guage model pre-training objective and the se- mantic similarity task theoretically, and then information, or just because the semantic meanings analyze the BERT sentence embeddings em- in these embeddings are not exploited properly? (2) pirically. We find that BERT always induces If the BERT embeddings capture enough semantic a non-smooth anisotropic semantic space of information that is hard to be directly utilized, how sentences, which harms its performance of can we make it easier without external supervision? semantic similarity. To address this issue, Towards this end, we first study the connection we propose to transform the anisotropic sen- between the BERT pretraining objective and the se- tence embedding distribution to a smooth and isotropic Gaussian distribution through nor- mantic similarity task. Our analysis reveals that the malizing flows that are learned with an un- sentence embeddings of BERT should be able to supervised objective. Experimental results intuitively reflect the semantic similarity between show that our proposed BERT-flow method ob- sentences, which contradicts with experimental ob- tains significant performance gains over the servations. Inspired by Gao et al.(2019) who find state-of-the-art sentence embeddings on a va- that the language modeling performance can be riety of semantic textual similarity tasks. The limited by the learned anisotropic word embedding code is available at https://github.com/ bohanli/BERT-flow. space where the word embeddings occupy a narrow cone, and Ethayarajh(2019) who find that BERT 1 Introduction word embeddings also suffer from anisotropy, we hypothesize that the sentence embeddings from Recently, pre-trained language models and its vari- BERT – as average of context embeddings from last ants (Radford et al., 2019; Devlin et al., 2019; Yang layers1 – may suffer from similar issues. Through et al., 2019; Liu et al., 2019) like BERT (Devlin empirical probing over the embeddings, we further et al., 2019) have been widely used as represen- observe that the BERT sentence embedding space tations of natural language. Despite their great is semantically non-smoothing and poorly defined success on many NLP tasks through fine-tuning, in some areas, which makes it hard to be used di- the sentence embeddings from BERT without fine- rectly through simple similarity metrics such as dot tuning are significantly inferior in terms of se- mantic textual similarity (Reimers and Gurevych, 1In this paper, we compute average of context embeddings from last one or two layers as our sentence embeddings since ∗ The work was done when BL was an intern at they are consistently better than the [CLS] vector as shown ByteDance. in (Reimers and Gurevych, 2019). product or cosine similarity. Reimers and Gurevych(2019) demonstrate that To address these issues, we propose to transform such BERT sentence embeddings lag behind the the BERT sentence embedding distribution into a state-of-the-art sentence embeddings in terms of smooth and isotropic Gaussian distribution through semantic similarity. On the STS-B dataset, BERT normalizing flows (Dinh et al., 2015), which is sentence embeddings are even less competitive to an invertible function parameterized by neural net- averaged GloVe (Pennington et al., 2014) embed- works. Concretely, we learn a flow-based genera- dings, which is a simple and non-contextualized tive model to maximize the likelihood of generating baseline proposed several years ago. Nevertheless, BERT sentence embeddings from a standard Gaus- this incompetence has not been well understood sian latent variable in a unsupervised fashion. Dur- yet in existing literature. ing training, only the flow network is optimized Note that as demonstrated by Reimers and while the BERT parameters remain unchanged. Gurevych(2019), averaging context embeddings The learned flow, an invertible mapping function consistently outperforms the [CLS] embedding. between the BERT sentence embedding and Gaus- Therefore, unless mentioned otherwise, we use av- sian latent variable, is then used to transform the erage of context embeddings as BERT sentence BERT sentence embedding to the Gaussian space. embeddings and do not distinguish them in the rest We name the proposed method as BERT-flow. of the paper. We perform extensive experiments on 7 stan- 2.1 The Connection between Semantic dard semantic textual similarity benchmarks with- Similarity and BERT Pre-training out using any downstream supervision. Our empir- We consider a sequence of tokens x = ical results demonstrate that the flow transforma- 1:T (x ; : : : ; x ). Language modeling (LM) factor- tion is able to consistently improve BERT by up 1 T izes the joint probability p(x ) in an autoregres- to 12.70 points with an average of 8.16 points in 1:T sive way, namely log p(x ) = PT log p(x jc ) terms of Spearman correlation between cosine em- 1:T t=1 t t where the context c = x . To capture bidirec- bedding similarity and human annotated similarity. t 1:t−1 tional context during pretraining, BERT proposes When combined with external supervision from a masked language modeling (MLM) objective, natural language inference tasks (Bowman et al., which instead factorizes the probability of noisy 2015; Williams et al., 2018), our method outper- reconstruction p(¯xjx^) = PT m p(x jc ), where forms the sentence-BERT embeddings (Reimers t=1 t t t x^ is a corrupted sequence, x¯ is the masked tokens, and Gurevych, 2019), leading to new state-of-the- m is equal to 1 when x is masked and 0 otherwise. art performance. In addition to semantic sim- t t The context c =x ^. ilarity tasks, we apply sentence embeddings to t Note that both LM and MLM can be reduced to a question-answer entailment task, QNLI (Wang modeling the conditional distribution of a token x et al., 2019), directly without task-specific super- given the context c, which is typically formulated vision, and demonstrate the superiority of our ap- with a softmax function as, proach. Moreover, our further analysis implies that BERT-induced similarity can excessively correlate > exp hc wx with lexical similarity compared to semantic sim- p(xjc) = P > : (1) 0 exp h w 0 ilarity, and our proposed flow-based method can x c x effectively remedy this problem. Here the context embedding hc is a function of c, which is usually heavily parameterized by a deep neural network (e.g., a Transformer (Vaswani et al., 2 Understanding the Sentence 2017)); The word embedding wx is a function of Embedding Space of BERT x, which is parameterized by an embedding lookup table. To encode a sentence into a fixed-length vector with The similarity between BERT sentence embed- BERT, it is a convention to either compute an aver- dings can be reduced to the similarity between age of context embeddings in the last few layers of T 2 BERT context embeddings hc hc0 . However, as BERT, or extract the BERT context embedding at the position of the [CLS] token. Note that there is 2This is because we approximate BERT sentence embed- dings with context embeddings, and compute their dot product no token masked when producing sentence embed- (or cosine similarity) as model-predicted sentence similarity. dings, which is different from pretraining. Dot product is equivalent to cosine similarity when the em- shown in Equation1, the pretraining of BERT does Higher-order context-context co-occurrence T not explicitly involve the computation of hc hc0 . could also be inferred and propagated during pre- Therefore, we can hardly derive a mathematical training. The update of a context embedding hc > formulation of what hc hc0 exactly represents. could affect another context embedding hc0 in the above way, and similarly hc0 can further affect an- Co-Occurrence Statistics as the Proxy for Se- other hc00 . Therefore, the context embeddings can mantic Similarity Instead of directly analyzing form an implicit interaction among themselves via T 0 > hc hc, we consider hc wx, the dot product between higher-order co-occurrence relations. a context embedding hc and a word embedding wx. According to Yang et al.(2018), in a well-trained 2.2 Anisotropic Embedding Space Induces > language model, hc wx can be approximately de- Poor Semantic Similarity composed as follows, As discussed in Section 2.1, the pretraining of BERT should have encouraged semantically mean- ingful context embeddings implicitly. Why BERT > ∗ hc wx ≈ log p (xjc) + λc (2) sentence embeddings without finetuning yield un- = PMI(x; c) + log p(x) + λc: (3) satisfactory performance? To investigate the underlying problem of the fail- p(x;c) ure, we use word embeddings as a surrogate be- where PMI(x; c) = log p(x)p(c) denotes the point- wise mutual information between x and c, log p(x) cause words and contexts share the same embed- ding space.
Details
-
File Typepdf
-
Upload Time-
-
Content LanguagesEnglish
-
Upload UserAnonymous/Not logged-in
-
File Pages12 Page
-
File Size-