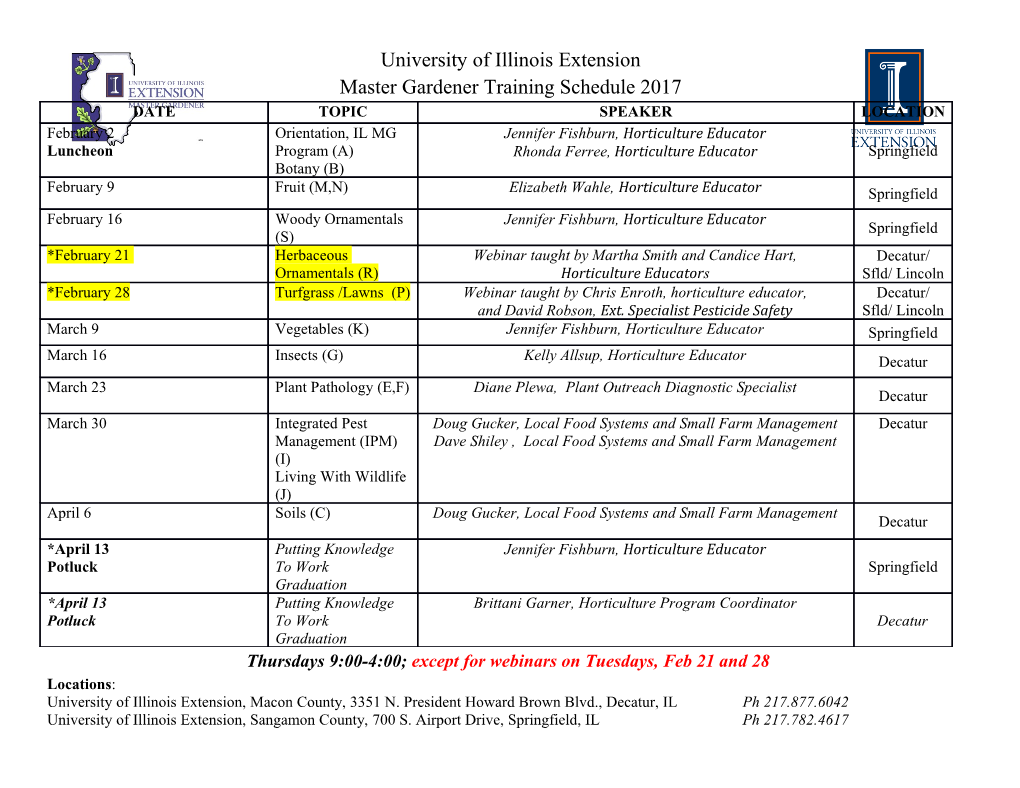
Chapter 2: Maximum Likelihood Estimation Advanced Econometrics - HEC Lausanne Christophe Hurlin University of Orléans December 9, 2013 Christophe Hurlin (University of Orléans) Advanced Econometrics - HEC Lausanne December9,2013 1/207 Section 1 Introduction Christophe Hurlin (University of Orléans) Advanced Econometrics - HEC Lausanne December9,2013 2/207 1. Introduction The Maximum Likelihood Estimation (MLE) is a method of estimating the parameters of a model. This estimation method is one of the most widely used. The method of maximum likelihood selects the set of values of the model parameters that maximizes the likelihood function. Intuitively, this maximizes the "agreement" of the selected model with the observed data. The Maximum-likelihood Estimation gives an uni…ed approach to estimation. Christophe Hurlin (University of Orléans) Advanced Econometrics - HEC Lausanne December9,2013 3/207 2. The Principle of Maximum Likelihood What are the main properties of the maximum likelihood estimator? I Is it asymptotically unbiased? I Is it asymptotically e¢ cient? Under which condition(s)? I Is it consistent? I What is the asymptotic distribution? How to apply the maximum likelihood principle to the multiple linear regression model, to the Probit/Logit Models etc. ? ... All of these questions are answered in this lecture... Christophe Hurlin (University of Orléans) Advanced Econometrics - HEC Lausanne December9,2013 4/207 1. Introduction The outline of this chapter is the following: Section 2: The principle of the maximum likelihood estimation Section 3: The likelihood function Section 4: Maximum likelihood estimator Section 5: Score, Hessian and Fisher information Section 6: Properties of maximum likelihood estimators Christophe Hurlin (University of Orléans) Advanced Econometrics - HEC Lausanne December9,2013 5/207 1. Introduction References Amemiya T. (1985), Advanced Econometrics. Harvard University Press. Greene W. (2007), Econometric Analysis, sixth edition, Pearson - Prentice Hil Pelgrin, F. (2010), Lecture notes Advanced Econometrics, HEC Lausanne (a special thank) Ruud P., (2000) An introduction to Classical Econometric Theory, Oxford University Press. Zivot, E. (2001), Maximum Likelihood Estimation, Lecture notes. Christophe Hurlin (University of Orléans) Advanced Econometrics - HEC Lausanne December9,2013 6/207 Section 2 The Principle of Maximum Likelihood Christophe Hurlin (University of Orléans) Advanced Econometrics - HEC Lausanne December9,2013 7/207 2. The Principle of Maximum Likelihood Objectives In this section, we present a simple example in order 1 To introduce the notations 2 To introduce the notion of likelihood and log-likelihood. 3 To introduce the concept of maximum likelihood estimator 4 To introduce the concept of maximum likelihood estimate Christophe Hurlin (University of Orléans) Advanced Econometrics - HEC Lausanne December9,2013 8/207 2. The Principle of Maximum Likelihood Example Suppose that X1,X2, ,XN are i.i.d. discrete random variables, such that Xi Pois (θ) with a pmf (probability mass function) de…ned as: exp ( θ) θxi Pr (Xi = xi ) = xi ! where θ is an unknown parameter to estimate. Christophe Hurlin (University of Orléans) Advanced Econometrics - HEC Lausanne December9,2013 9/207 2. The Principle of Maximum Likelihood Question: What is the probability of observing the particular sample x1, x2, .., xN , assuming that a Poisson distribution with as yet unknown parameterf θ generatedg the data? This probability is equal to Pr ((X1 = x1) ... (XN = xN )) \ \ Christophe Hurlin (University of Orléans) Advanced Econometrics - HEC Lausanne December9,2013 10/207 2. The Principle of Maximum Likelihood Since the variables Xi are i.i.d. this joint probability is equal to the product of the marginal probabilities N Pr ((X1 = x1) ... (XN = xN )) = ∏ Pr (Xi = xi ) \ \ i=1 Given the pmf of the Poisson distribution, we have: N exp ( θ) θxi Pr ((X1 = x1) ... (XN = xN )) = ∏ \ \ i=1 xi ! N x θ∑i=1 i = exp ( θN) N ∏ xi ! i=1 Christophe Hurlin (University of Orléans) Advanced Econometrics - HEC Lausanne December9,2013 11/207 2. The Principle of Maximum Likelihood De…nition This joint probability is a function of θ (the unknown parameter) and corresponds to the likelihood of the sample x1, .., xN denoted by f g LN (θ; x1.., xN ) = Pr ((X1 = x1) ... (XN = xN )) \ \ with N 1 ∑=1 xi LN (θ; x1.., xN ) = exp ( θN) θ N ∏ xi ! i=1 Christophe Hurlin (University of Orléans) Advanced Econometrics - HEC Lausanne December9,2013 12/207 2. The Principle of Maximum Likelihood Example Let us assume that for N = 10, we have a realization of the sample equal to 5, 0, 1, 1, 0, 3, 2, 3, 4, 1 , then: f g LN (θ; x1.., xN ) = Pr ((X1 = x1) ... (XN = xN )) \ \ e 10θθ20 L (θ; x .., x ) = N 1 N 207, 360 Christophe Hurlin (University of Orléans) Advanced Econometrics - HEC Lausanne December9,2013 13/207 2. The Principle of Maximum Likelihood Question: What value of θ would make this sample most probable? Christophe Hurlin (University of Orléans) Advanced Econometrics - HEC Lausanne December9,2013 14/207 2. The Principle of Maximum Likelihood This Figure plots the function LN (θ; x) for various values of θ. It has a single mode at θ = 2, which would be the maximum likelihood estimate, or MLE, of θ. •8 x 10 1.2 1 0.8 0.6 0.4 0.2 0 0 0.5 1 1.5 2 2.5 3 3.5 4 q Christophe Hurlin (University of Orléans) Advanced Econometrics - HEC Lausanne December9,2013 15/207 2. The Principle of Maximum Likelihood Christophe Hurlin (University of Orléans) Advanced Econometrics - HEC Lausanne December9,2013 16/207 2. The Principle of Maximum Likelihood Consider maximizing the likelihood function LN (θ; x1.., xN ) with respect to θ. Since the log function is monotonically increasing, we usually maximize ln LN (θ; x1.., xN ) instead. In this case: N N ln LN (θ; x1.., xN ) = θN + ln (θ) xi ln ∏ xi ! ∑ i=1 i=1 N ∂ ln LN (θ; x1.., xN ) 1 = N + ∑ xi ∂θ θ i=1 2 N ∂ ln LN (θ; x1.., xN ) 1 2 = 2 ∑ xi < 0 ∂θ θ i=1 Christophe Hurlin (University of Orléans) Advanced Econometrics - HEC Lausanne December9,2013 17/207 2. The Principle of Maximum Likelihood Under suitable regularity conditions, the maximum likelihood estimate (estimator) is de…ned as: θ = arg max ln LN (θ; x1.., xN ) θ R+ 2 b N ∂ ln LN (θ; x1.., xN ) 1 FOC : = N + xi = 0 ∂θ ∑ θ θ i=1 N b b θ = (1/N) ∑ xi () i=1 2 b N ∂ ln LN (θ; x1.., xN ) 1 SOC : = xi < 0 2 2 ∑ ∂θ θ θ i=1 θ is a maximum. b b Christopheb Hurlin (University of Orléans) Advanced Econometrics - HEC Lausanne December9,2013 18/207 2. The Principle of Maximum Likelihood The maximum likelihood estimate (realization) is: 1 N θ θ (x) = ∑ xi N i=1 b b Given the sample 5, 0, 1, 1, 0, 3, 2, 3, 4, 1 , we have θ (x) = 2. f g The maximum likelihood estimator (random variableb) is: 1 N θ = ∑ Xi N i=1 b Christophe Hurlin (University of Orléans) Advanced Econometrics - HEC Lausanne December9,2013 19/207 2. The Principle of Maximum Likelihood Continuous variables The reference to the probability of observing the given sample is not exact in a continuous distribution, since a particular sample has probability zero. Nonetheless, the principle is the same. The likelihood function then corresponds to the pdf associated to the joint distribution of (X1, X2, .., XN ) evaluated at the point (x1, x2, .., xN ) : LN (θ; x1.., xN ) = fX1,..,XN (x1, x2, .., xN ; θ) Christophe Hurlin (University of Orléans) Advanced Econometrics - HEC Lausanne December9,2013 20/207 2. The Principle of Maximum Likelihood Continuous variables If the random variables X1, X2, .., XN are i.i.d. then we have: f g N LN (θ; x1.., xN ) = ∏ fX (xi ; θ) i=1 where fX (xi ; θ) denotes the pdf of the marginal distribution of X (or Xi since all the variables have the same distribution). The values of the parameters that maximize LN (θ; x1.., xN ) or its log are the maximum likelihood estimates, denoted θ (x). b Christophe Hurlin (University of Orléans) Advanced Econometrics - HEC Lausanne December9,2013 21/207 Section 3 The Likelihood function De…nitions and Notations Christophe Hurlin (University of Orléans) Advanced Econometrics - HEC Lausanne December9,2013 22/207 3. The Likelihood Function Objectives 1 Introduce the notations for an estimation problem that deals with a marginal distribution or a conditional distribution (model). 2 De…ne the likelihood and the log-likelihood functions. 3 Introduce the concept of conditional log-likelihood 4 Propose various applications Christophe Hurlin (University of Orléans) Advanced Econometrics - HEC Lausanne December9,2013 23/207 3. The Likelihood Function Notations Let us consider a continuous random variable X , with a pdf denoted fX (x; θ) , for x R 2 | θ = (θ1..θK ) is a K 1 vector of unknown parameters. We assume that θ Θ RK . 2 Let us consider a sample X1, .., XN of i.i.d. random variables with the same arbitrary distributionf as X .g The realisation of X1, .., XN (the data set..) is denoted x1, .., xN or x for simplicity.f g f g Christophe Hurlin (University of Orléans) Advanced Econometrics - HEC Lausanne December9,2013 24/207 3. The Likelihood Function Example (Normal distribution) If X N m, σ2 then: 1 (z m)2 fX (z; θ) = exp 2 z R σp2π 2σ ! 8 2 with K = 2 and m θ = σ2 Christophe Hurlin (University of Orléans) Advanced Econometrics - HEC Lausanne December9,2013 25/207 3. The Likelihood Function De…nition (Likelihood Function) The likelihood function is de…ned to be: N + LN : Θ R R ! N (θ; x1, .., xn) LN (θ; x1, .., xn) = ∏ fX (xi ; θ) 7! i=1 Christophe Hurlin (University of Orléans) Advanced Econometrics - HEC Lausanne December9,2013 26/207 3. The Likelihood Function De…nition (Log-Likelihood Function) The log-likelihood function is de…ned to be: N `N : Θ R R ! N (θ; x1, .., xn) `N (θ; x1, .., xn) = ∑ ln fX (xi ; θ) 7! i=1 Christophe Hurlin (University of Orléans) Advanced Econometrics - HEC Lausanne December9,2013 27/207 3.
Details
-
File Typepdf
-
Upload Time-
-
Content LanguagesEnglish
-
Upload UserAnonymous/Not logged-in
-
File Pages207 Page
-
File Size-