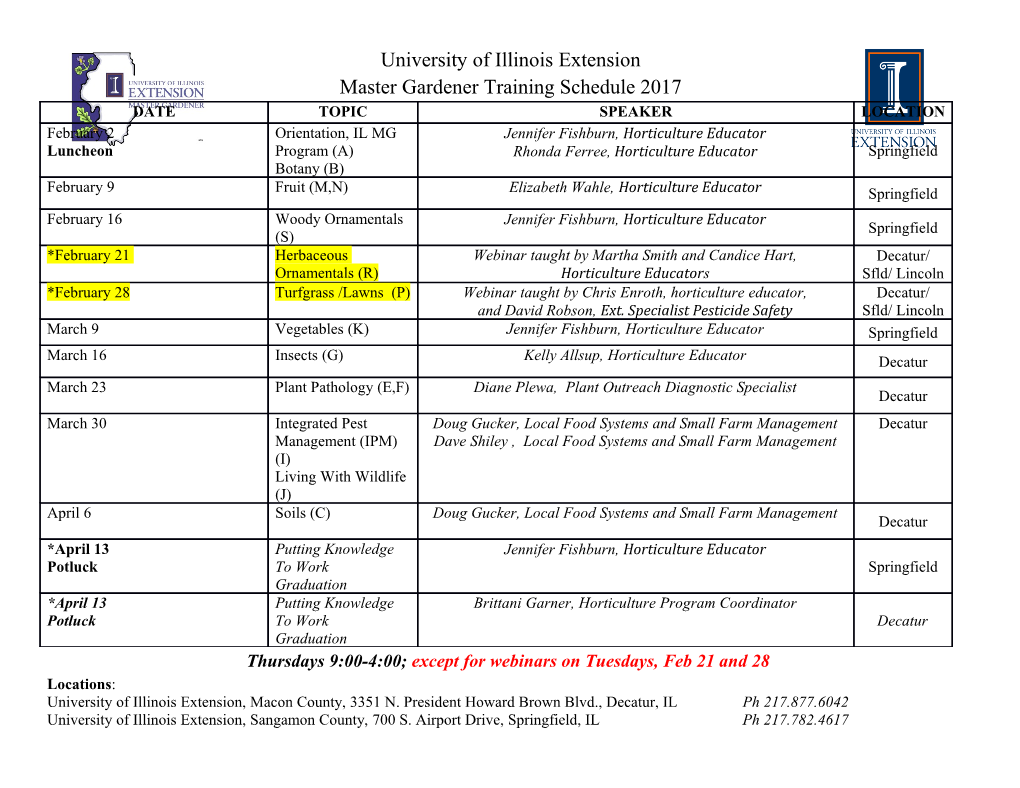
AUTOMATED PULMONARY ARTERY SEGMENTATION BY VESSEL TRACKING IN LOW-DOSE COMPUTED TOMOGRAPHY IMAGES A Thesis Presented to the Faculty of the Graduate School of Cornell University in Partial Fulfillment of the Requirements for the Degree of Master of Science by Jeremiah Anthony Wala January 2011 c 2011 Jeremiah Anthony Wala ALL RIGHTS RESERVED ABSTRACT Low-dose computed tomography (CT) imaging provides a method for obtain- ing accurate anatomical information without the full radiation exposure inherent in standard CT protocols, and is primarily used in lung cancer screening. Seg- mentation of the pulmonary arteries from low-dose chest CT images is a vital first step in improving computer-aided detection of frequently missed pulmonary nod- ules near major arteries. This thesis presents the first fully automated method for segmenting the main pulmonary arterial trees in low-dose CT images. The correlation between the arterial and airway trees was used to develop an automated pulmonary artery seed point detector. The main basal pulmonary arteries are identified by searching for candidate vessels near known airways, using a progressive morphological opening method. The arteries are tracked into the lungs by means of a cylindrical vessel tracker that iteratively fits model cylinders to the CT image. Vessel bifurcations are detected by measuring the rate of change of vessel radii. Subsequent vessels are segmented by initiating new cylinder trackers at bifurcation points. Quantitative analysis of both the number of arteries and veins segmented, as well as the error per vessel, was accomplished with a novel evaluation metric called the Sparse Surface (SS) metric. The SS metric was developed to capture the details of the true vessel surface while reducing the ground-truth marking burden on the human user. This metric is a unique new tool for ground truth marking and segmentation validation, with particular importance in problems with complex geometries. The segmentation method and SS metric were applied to a dataset of seven CT images, and achieved an overall sensitivity of 0.62 and specificity of 0.90 of all manually identified vessels. The average root mean square error between the vessel surface and the segmentation surface was 0.63 mm, or less than 1 voxel. Addition- ally, seed points were detected automatically for a majority (80%) of cases with labeled airways. This method is an important first step towards robust pulmonary artery segmentation and artery/vein separation in low-dose chest CT, and is the first fully automated method designed for accomplishing this task. BIOGRAPHICAL SKETCH Jeremiah Wala lived in Waseca, MN for 18 years before moving to Ithaca, NY in 2005 to attend Cornell University. In May 2009 he received a Bachelor of Science magna cum laude in Engineering Physics. He then continued at Cornell, pursuing a Master of Science degree in Applied Physics. While at Cornell, he has worked as a teaching assistant in the fields of nanotechnology, quantum and statistical physics, mathematics, and organic chemistry, and as a researcher in nanotechnology, radiobiology and medical imaging. In 2010, he began his medical studies at Harvard Medical School and the Massachusetts Institute of Technology, and is pursuing an M.D. degree. iii ACKNOWLEDGEMENTS First and foremost, I would like to thank my advisor, Prof. Anthony Reeves, for his assistance, guidance, and vast patience as I navigated with much trial and error the research and thesis writing process. Without his support, this work would not be possible. I also want to thank my fellow researchers in the VIA group, Artit Jirapatnakul, Alberto Biancardi, Sergei Fotin, Brad Keller and Jaesung Lee, for their endless knowledge and willingness to help me sort out the bugs. I would also like to thank my committee chair Prof. Joel Brock for his encour- agement as a teaching advisor and committee member. I am very grateful to both him and the Cornell Applied Physics department for their remarkable support of my goals. Finally, I would like to thank my parents, sister, and friends for their reassur- ance and encouragement throughout my time in college, graduate school, and life in general. iv TABLE OF CONTENTS Biographical Sketch . iii Acknowledgements . iv Table of Contents . .v List of Tables . vii List of Figures . viii 1 Introduction 1 1.1 Clinical Application . .2 1.2 Computed tomography . .3 1.2.1 X-ray physics . .3 1.2.2 CT scanner technology . .6 1.3 Pulmonary artery anatomy . .8 1.4 Issues in pulmonary artery segmentation . 11 1.5 Previous work . 13 1.5.1 General pulmonary vessel segmentation methods . 14 1.5.2 Pulmonary artery segmentation methods . 17 1.6 Outline . 19 2 Seed Point Detection 20 2.1 Method . 20 2.1.1 Anatomical model . 20 2.1.2 Region of interest construction . 23 2.1.3 Artery identification . 24 2.2 Training and Experiment Design . 29 2.3 Results . 30 2.4 Discussion . 31 3 Method for Tracking the Arterial Tree 34 3.1 Preliminary steps . 34 3.2 Cylinder matching metric . 35 3.3 Iterative vessel tracking . 37 3.4 Bifurcation detection . 40 4 Sparse Surface Evaluation Metric 46 4.1 Previously reported evaluation metrics . 46 4.2 Sparse surface metric . 48 4.3 Formal definition . 49 4.4 Comparison with dense markings . 52 v 5 Vessel Tracking Optimization 55 5.1 Dataset . 55 5.2 Performance evaluation with the SS metric . 56 5.3 Training and Optimization . 57 5.4 Experiment design . 59 6 Vessel Tracking Results and Discussion 60 6.1 Results . 60 6.2 Discussion . 62 7 Conclusion 66 7.1 Contributions . 66 7.2 Future Work . 67 Bibliography 69 vi LIST OF TABLES 1.1 Summary of previously reported methods for non-discriminantly segmenting the pulmonary vasculature and for discriminating ar- teries from veins. 15 2.1 Qualitative results for the basal artery identification method, tested on50cases................................ 30 3.1 Static and run-time optimized parameters of the vessel tracking method . 40 4.1 Summary of previously reported metrics for evaluating pulmonary vessel segmentations. 47 4.2 Statistical measurements used in the SS evaluation metric . 52 5.1 CT scanning parameters for the 50 cases in the low-dose data set. 55 5.2 Vessel classification for evaluating algorithm performance . 56 5.3 Parameter sets used in training on 3 documented CT images. 58 vii LIST OF FIGURES 1.1 A) Photelectric interaction and B) Compton scattering of an in- cident X-ray. These two processes account for the attenuation of X-rays in matter, providing information to the CT scanner about the radiodensity of the irradiated tissue. .5 1.2 Two common systems for X-ray generation in CT scanners. .7 1.3 The location of the pulmonary arteries in the mediastinum and the point where they emerge into the lung parenchyma. .8 1.4 Labelled airway tree demonstrating the bronchopulmonary nomen- clature used to describe the pulmonary arteries. 10 1.5 Segmentation issue caused by close proximity of arterial and venous trees . 12 1.6 Segmentation issue caused by proximity of airway wall to arterial tree . 13 1.7 Segmentation issue caused by vessel bifurcation. In this case, an adjacent vein has the appearance of stemming from a trifurcation of the parent artery. 14 2.1 Illustration of the relationships of the labelled airways to the pul- monary arteries . 21 2.2 Axial slice showing the location of the left and right basal arteries relative to the airway segmentation and the lung parenchyma. 22 2.3 Diagram of the airway ROI construction process and a single frame from the output. 24 2.4 Histogram of the ROI around the left basal airway. A threshold of -400 HU was applied to separate the peaks. 25 2.5 Process diagram for the identification of the main basal pulmonary arteries within the ROI . 26 2.6 Diagram of the progressive opening operation for separating the pulmonary artery from adjacent structures within the ROI . 27 2.7 Diagram of the seed point identification experiment . 30 2.8 Successful seed point detection. The result of the morphological opening is overlaid on the images. 31 2.9 Failed seed point detection. The morphological opening does not separate the artery from the mediastinum. Connected component analysis eliminates the morphological opening, as they are not vi- able artery candidates. The final result is that no seed point is detected. 32 3.1 Pre-processing of CT images reduces noise, eliminates airway walls, and converts to binary image. 35 3.2 Modeling of a blood vessel with discrete cylinders . 36 3.3 Iterative vessel tracking process diagram. 38 viii 3.4 Iterative fitting of the model cylinders . 40 3.5 Bifurcation detection using model cylinder radius change . 41 3.6 Process diagram for locating child vessels at bifurcation point. 42 3.7 Search space for locating the second child vessel at a bifurcation point. 43 3.8 False positive bifurcation detection and error correcting. A) The child vessel does not find any strong matches. B) The child vessel leaks onto the mediastinum. 44 4.1 Diagram illustrating the SS metric. The illustrated distances com- prise the set M............................. 51 4.2 Manual SS markings overlaid on the left main pulmonary artery and its two child branches . 52 4.3 Histograms of the truth-to-surface error applied to case 1 for a) sparse markings and b) dense markings. 53 5.1 Training results for the cylinder height, bifurcation detection, and incremental step size parameters. Each plot shows the effect of varying one parameter while retaining the other two at their opti- mal values. 58 5.2 Process diagrams vessel tracking experiment . 59 6.1 Sensitivity and specificity for the ten documented cases. 60 6.2 Results of the artery identification method for the LBPA and RBPA 61 6.3 Distribution of truth-to-surface distance errors for correctly identi- fied vessels using sparse markings across 10 cases.
Details
-
File Typepdf
-
Upload Time-
-
Content LanguagesEnglish
-
Upload UserAnonymous/Not logged-in
-
File Pages82 Page
-
File Size-