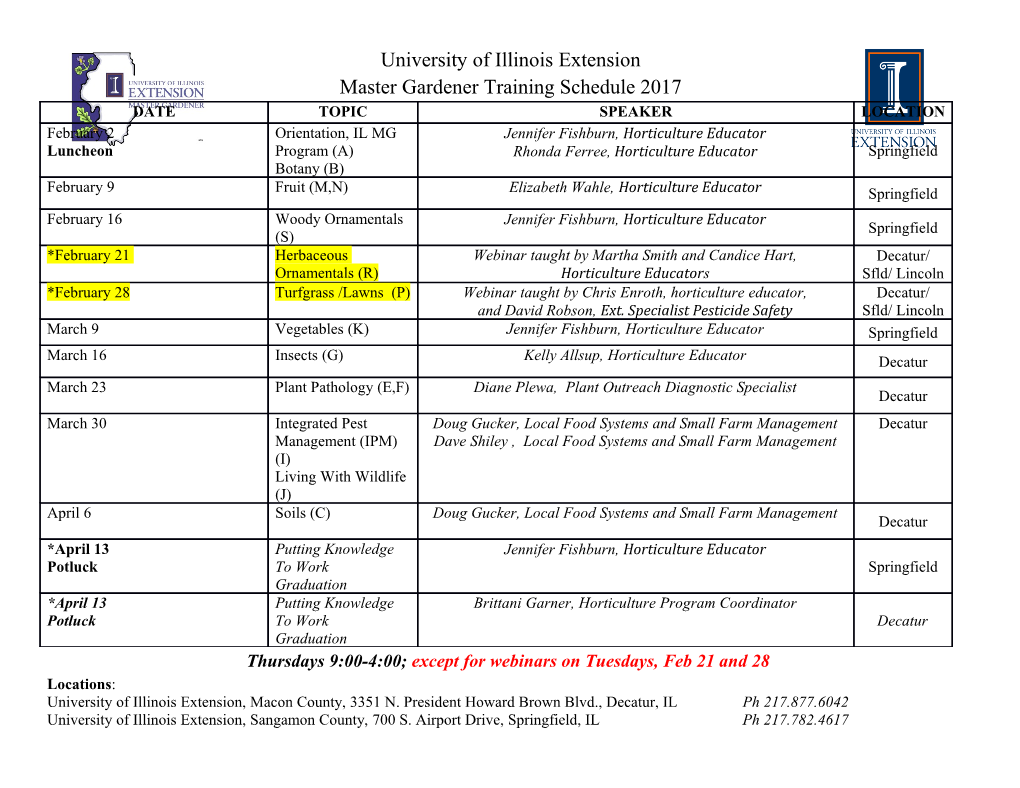
Inteligencia Artificial. Revista Iberoamericana de Inteligencia Artificial ISSN: 1137-3601 [email protected] Asociación Española para la Inteligencia Artificial España Antiqueira, Lucas; Salgueiro Pardo, Thiago Alexandre; das Graças Volpe Nunes, Maria; Oliveira Jr., Osvaldo N. Some issues on complex networks for author characterization Inteligencia Artificial. Revista Iberoamericana de Inteligencia Artificial, vol. 11, núm. 36, 2007, pp. 51- 58 Asociación Española para la Inteligencia Artificial Valencia, España Available in: http://www.redalyc.org/articulo.oa?id=92503608 How to cite Complete issue Scientific Information System More information about this article Network of Scientific Journals from Latin America, the Caribbean, Spain and Portugal Journal's homepage in redalyc.org Non-profit academic project, developed under the open access initiative AAAAAAAAAAAAAAAAAAAAAAAA´ AAAAAAAAAAAAAAAAAAAAAAAA´ ART´ICULO Some issues on complex networks for author characterization Lucas Antiqueira1,3, Thiago Alexandre Salgueiro Pardo1,3, Maria das Gra¸casVolpe Nunes1,3 and Osvaldo N. Oliveira Jr.2,3 1Instituto de Ciˆencias Matem´aticas e de Computa¸c˜ao(ICMC), Universidade de S˜aoPaulo (USP) – S˜ao Carlos, SP, Brasil 2Instituto de F´ısica de S˜aoCarlos (IFSC), Universidade de S˜aoPaulo (USP) – S˜ao Carlos, SP, Brasil 3N´ucleoInterinstitucional de Ling¨u´ıstica Computacional (NILC), www.nilc.icmc.usp.br [email protected], [email protected], [email protected], [email protected] Abstract This paper presents a modeling technique of texts as complex networks and the investigation of the correlation between the properties of such networks and author characteristics. In an experiment with several books from eight authors, we show that the networks produced for each author tend to have specific features, which indicates that complex networks can capture author characteristics and, therefore, could be used for the traditional task of authorship identification. Keywords: Authorship Attribution, Complex Networks. 1 Introduction We focus in this work on graph representation of texts, using measures usually employed in the studies of complex networks. Differently from Recent trends in Natural Language Processing regular networks, the dynamics and topology of a (NLP) have indicated that graphs are a powerful complex network follow non-trivial organization modeling and problem-solving technique. Graph principles [17, 5]. Complex networks concepts theory, a branch of mathematics, has been stud- have been applied to a number of world phe- ied for a long time, providing elegant and sim- nomena, e.g., internet, social networks and flight ple solutions to several problems. Recently, the routes, which includes NLP and linguistics prob- field of complex networks, an intersection be- lems - as we briefly introduce in the next section. tween graph theory and statistical mechanics, has motivated renewed interest in modeling systems We tackled the problem of authorship characteri- as graphs. As evidence, graphs have been used zation using a network of words and a set of net- in various applications, including text summa- work measures. As known, authors present dif- rization [10, 14], information retrieval and related ferent styles of writing, resulting in diverse infor- tasks [19, 13, 18], and sentiment analysis in texts mation flows and text structures. We therefore [20]. Inteligencia Artificial, Revista Iberoamericana de Inteligencia Artificial. Vol. 11, No 36 (2007), pp. 51-58. ISSN: 1137-3601. c AEPIA (http://www.aepia.org/revista) 52 Inteligencia Artificial Vol. 11, No 36, 2007 look for correlations between the texts of a same ferent from the random networks, because their author and the measures extracted from the cor- distributions of connections are not uniform; in- responding networks. In this paper we show an stead, a scale-free network has few nodes with a experiment made with several books of 8 authors high number of connections (called hubs), which from diverse text genres, including fiction, science coexist with a much higher number of nodes and poetry. We show that different measures that have a small number of connections. Scale- vary considerably between some authors, which free networks are explained by two related con- encourages the use of complex networks in the cepts, (i) growth, which determines that a net- task of authorship identification. work is continually incorporating new nodes, and (ii) preferential attachment, which defines that In the next section we introduce complex net- new nodes prefer to establish connections with works, its main concepts and the measures used already well connected nodes. in this work. The methodology we follow and the obtained results are presented in Section 3 and 4, Erd¨os and R´enyi’s random graph theory is a respectively. Some conclusions are presented in good example of an early study on complex net- Section 5. works, but such networks only started to receive an enormous attention from the scientific com- munity in the recent years, after the publication of the Watts-Strogatz and Barab´asi-Albert pa- 2 Complex Networks and pers. The modern reserch on complex networks Language typically incorporates concepts from mechanical statistics, and aims to characterize networks in terms of their structure and dynamics. A series Complex networks have received enormous atten- of network models and measurements have been tion recently, but their origins can be traced back applied or created in recent network research [8], to the first study on graph theory, i.e., the Leon- forming a profusion of tools available to almost hard Euler solution to the K¨onigsberg bridges every network study. In our present paper, we use problem in 1736 [3]. The concept of nodes linked a set of complex network measures (introduced in by edges (used by Euler) provides a general math- the next paragraphs) in order to face the problem ematical way to describe discrete elements and of authorship characterization. their inter-relationships. Erd¨os and R´enyi, two centuries later, created a way to explain the for- The tendency of the network nodes to form local mation of real networks, which is called random interconnected groups is quantified by a measure graph theory. They published a series of eight referred to as clustering coefficient. For comput- papers, started in 1959 [9], that established for ing this coefficient for a node i in a directed net- decades a random view of our world, an egalitar- work, consider S as the set of nodes that have an ian world in which almost all nodes have a similar input edge from i, |S| as the number of nodes in number of connections. S, and B the number of edges among the nodes in S. Equation (1) computes the clustering coef- Alternatives to the random graph theory were ficient of a node i: later developed, which motivated a whole new interest in the study of networks, showing that many real networks are not random. Small-world B Clustering Coefficient (i) = . (1) networks were introduced more recently (e.g., |S|(|S| − 1) Milgram [15] and Watts and Strogatz [24]), which differs from random networks in the sense that in such networks it is possible to reach every node If |S| = 0 or |S| = 1, the coefficient is set to through a relatively small number of other nodes. 0. The clustering coefficient of a network is the In other words, a small-world network has a small average coefficient of its complete set of nodes. mean shortest path length (it also increases slowly - logarithmically - as the network grows). More- We have created another measure, based on the over, these networks have a high clustering coef- dynamical connectivity of the network growth. ficient (defined later in this section), which is a This type of measure is typical in complex net- tendency to form local groups of interconnected work studies, since it considers the evolution of a nodes. Barab´asi and Albert [4], introduced an- network. It is given by the number of connected other special class of networks, named scale-free components as edges are progressively incorpo- networks. These networks are also markedly dif- rated or have their associated weights increased Inteligencia Artificial Vol. 11, No 36, 2007 53 in the network1,2. The obtained dynamics is then other words, since each edge associates two nodes compared to a hypothetical uniform variation of n1 and n2, it is possible to create a set of bidi- the number of components (Figure 1), and the mensional points that represents the edges. Each extent to which the real dynamics departs from edge is then denoted by the coordinates (degree 4 the hypothetical one is quantified by a measure of n1, degree of n2) . The degree correlation is called components dynamics deviation. This de- the Pearson correlation coefficient applied to this viation is obtained for a network as follows: set of points. PE |f(k) − g(k)| Deviation = k=1 , (2) Complex networks have been applied to several NE NLP and linguistics tasks. Sigman and Cec- chi [23] modeled WordNet semantic relations be- where f(k) is the function that determines the tween words into a network of word meanings, number of components for k considered edge where the presence of highly polysemic words led modifications (insertions or weight changes) and to a small-world network. Another semantic net- g(k) is the function that determines the linear work, which was derived from a thesaurus, was variation of components (see Figure 1). N is the analysed by Motter et al. [16]. This network number of different words in the text and E is the was found to be scale-free. In fact, this model total number of edges modifications. is very common in linguistic networks, since it also conforms to (i) the word co-occurrence net- work, which models the sequence of words in a text [11], (ii) the word association network, where related concepts are linked [7], and (iii) the syn- tactic dependency network, which models syntac- tic relationships between words [12].
Details
-
File Typepdf
-
Upload Time-
-
Content LanguagesEnglish
-
Upload UserAnonymous/Not logged-in
-
File Pages9 Page
-
File Size-