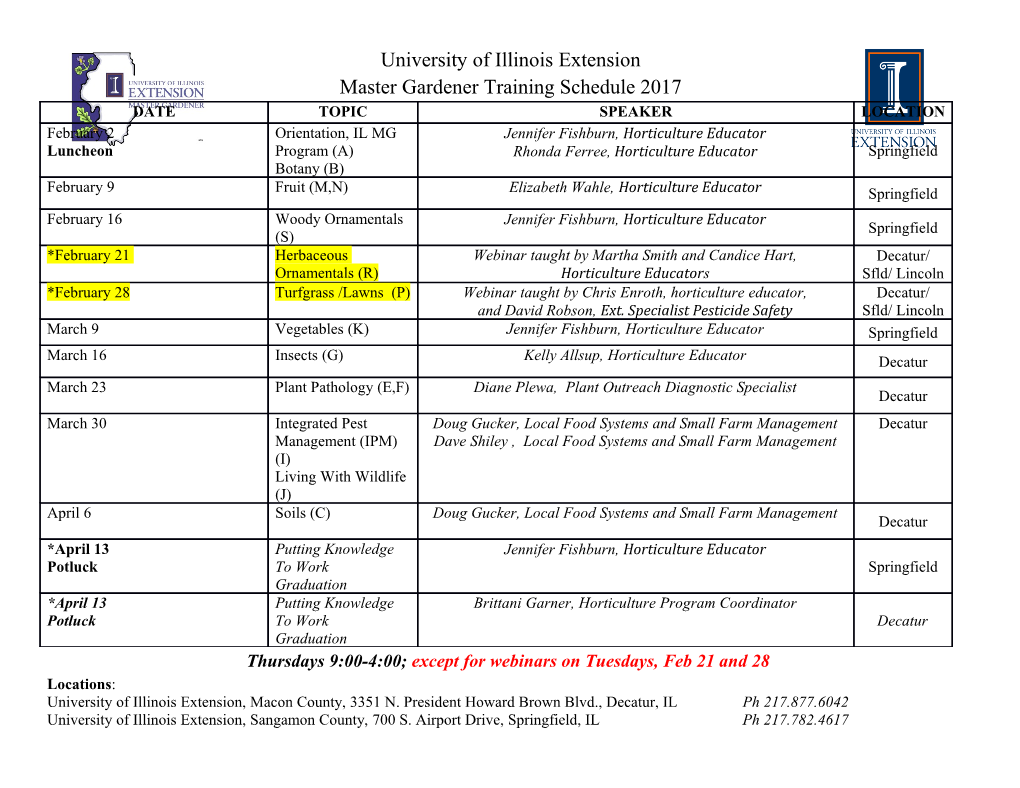
Wave-equation Rayleigh wave inversion using fundamental and higher modes Item Type Conference Paper Authors Zhang, Zhendong; Alkhalifah, Tariq Ali Citation Zhang Z, Alkhalifah T (2018) Wave-equation Rayleigh wave inversion using fundamental and higher modes. SEG Technical Program Expanded Abstracts 2018. Available: http:// dx.doi.org/10.1190/segam2018-2989655.1. Eprint version Publisher's Version/PDF DOI 10.1190/segam2018-2989655.1 Publisher Society of Exploration Geophysicists Journal SEG Technical Program Expanded Abstracts 2018 Rights Archived with thanks to SEG Technical Program Expanded Abstracts 2018 Download date 30/09/2021 13:45:49 Link to Item http://hdl.handle.net/10754/631151 Wave-equation Rayleigh wave inversion using fundamental and higher modes Zhen-dong Zhang∗ and Tariq Alkhalifah∗ ∗King Abdullah University of Science and Technology. SUMMARY as skeletonized inversion) aims to match the dispersion curves of Rayleigh waves instead of complex waveforms. It might en- Recorded surface waves often provide reasonable estimates of joy a more quasi-linear relationship between the model and the the Shear wave velocity in the near surface. However, these data and has a less bumpy misfit function than that correspond- estimates tend to be low in resolution considering that they ing to waveform inversion. However, the previously proposed depend on dispersion nature of the fundamental mode of sur- wave-equation inversion algorithms highly depend on the auto- face waves. We present a surface-wave inversion method that matic picking of the dispersion curves from the f −v spectrum. inverts for the S-wave velocity from the fundamental- and Although the automatic picking can only apply to predicted higher-modes of Rayleigh waves. The proposed method aims data, in which case the approach is stable, it inevitably ignores to maximize the similarity of the phase velocity ( f − v) spec- the higher-mode Rayleigh waves and therefore cannot handle trum of the surface waves with all-Rayleigh wave modes (if models with low-velocity layers (velocity reversal). The gen- they exist) in the inversion. The f − v spectrum is calcu- eration of higher modes is often attributed to the presence of lated using the linear Radon transform and by using a local low S-wave velocity layers (STOKOE II, 1994), and thus, such similarity-based objective function, we do not need to pick ve- low velocity (or velocity reversal) can not be recovered without locities in the spectrum plots. Thus, the best match between inverting such modes. Besides, higher modes penetrate deeper the predicted and observed data f − v spectrum provides the than the fundamental mode and can increase the resolution of optimal estimation of S-wave velocity. We derive the gradi- the estimated S-wave velocities (Xia et al., 2003). ent of the proposed objective function using the adjoint-state method and solve the optimization problem using the LBFGS In this abstract, we adapt the wave equation dispersion inver- method. Our method can invert for lateral velocity variations, sion to include the fundamental- and higher-modes Rayleigh include all-mode dispersions, and mitigate the local minimum waves. Instead of picking the dispersion curve, we use the problem in full waveform inversion with a reasonable compu- f − v spectrum as input data. A local-similarity based ob- tation cost. Results with synthetic and field data illustrate the jective function is introduced to measure the similarity of the benefits and limitations of this method. observed and predicted f − v spectrum. The f − v spectrum is calculated using a high-resolution linear Radon transform (Luo et al., 2008). This abstract is divided into four sections. After the introduction, we introduce a novel objective function INTRODUCTION and solve the optimization problem. In the third section, we first test the synthetic model with S-wave velocity reversal and Conventional surface wave inversion methods fall into three lateral variation, then apply the method to field data to ana- categories: 1) 1D inversion for a layered medium using semi- lyze the effectiveness and limitations of our method. The last analytical solutions to the elastic wave equation (Nazarian et al., section presents the summary of our work. 1983; Xia et al., 2004; Milana et al., 2014) or global optimiza- tion methods including genetic algorithms (Feng et al., 2005; Dong et al., 2014), 2) full waveform inversion (Groos et al., THEORY 2014; Solano et al., 2014), and 3) wave equation dispersion- curve based inversions (Zhang et al., 2015, 2016; Li et al., Objective functions intend to measure the mismatch between 2016; Lu et al., 2017). Semi-analytical solutions can be used to the predicted and the observed data. One of the most intuitive robustly and efficiently invert for a 1D S-wave velocity model, measurements is the L2 norm distance, which is given by but they are less accurate as the lateral variation in velocity is large in the subsurface. Global optimization methods can f(m) = jjd p(m) − dojj2; (1) be used in practice for a layered 1D model, but the compu- d p do tational cost is not acceptable for the 2D and 3D cases, and where f measures the differences; and are predicted and especially for strong lateral variations in S-wave velocity. In observed data, respectively. contrast, waveform inversion estimates the velocity model that The inverse problem is constrained by the first-order elastic minimizes the misfit between the predicted and recorded data. wave equation, which is given by However, the data-misfit function can be very sensitive to the accurate prediction of amplitudes, which is difficult to achieve with modeling methods that do not fully take into account the ¶Y T Downloaded 02/22/19 to 109.171.137.221. Redistribution subject SEG license or copyright; see Terms of Use at http://library.seg.org/ rI 0 0 E 3 − Y − f = 0; (2) viscoelastic and anisotropic nature of the Earth. Moreover, 0 C−1 ¶t E 0 a poor starting model will promote cycle-skipping and cause convergence to a local minimum (Virieux and Operto, 2009). where Y = (v1;v2;v3;s1;s2;s3;s4;s5;s6) is a vector con- taining three particle velocities and six stresses, C is the stiff- The wave-equation dispersion inversion method (also known ness matrix, E denotes space differentiation, and f is the source. © 2018 SEG 10.1190/segam2018-2989655.1 SEG International Exposition and 88th Annual Meeting Page 2511 a) b) Due to the oscillatory nature of seismic waves, the L2 norm objective function suffers from cycle skipping when the mis- matches between the predicted and observed data exceed a half cycle. A natural remedy to this problem is to compare two events within a predefined extension. Theoretically, the crit- ical value for cycle skipping in this approach is enlarged by the extension used. We propose a local-similarity based objec- tive function replacing the L2 norm based objective function, which is given by Figure 1: The actual vp (a) and r (b) models used for the syn- thetic examples. They’re also used as initial models but the 1 Z Z Z Z 2 f(m) = WjCp( f ;v)j · jCo( f + f 0;v)j d f 0d f drds; initial vp is equal to 90% of the true model. 2 s r f f 0 (3) a) b) where jCpj and jCo( f + f 0;v)j are normalized f − v spectrum of the predicted and observed data, respectively. f 0 denotes frequency extensions. W is a polynomial-type weighting func- tion, which satisfies the following boundary conditions: Wj± f 0 = 0 0 0;Wj0 = 1;W j± f 0 = 0;W j0 = 0. The f −v spectrum is calculated using a high-resolution linear Radon transform (Luo et al., 2008). After a temporal Fourier transform of the shot gather, the linear Radon transform can be Figure 2: The actual vs (a) and the estimated vs (b). Notice that calculated for each temporal frequency component f as: there are low S-wave velocity zones which cannot be captured by previous automatic picking approaches (usually only the Z xmax − i2p f x C( f ;v) = D( f ;x)e v dx; (4) fundamental mode can be picked automatically). xmin and its adjoint form is given by the inversion is 10% lower than its actual value in the syn- Z vmax i2p f x D( f ;x) = C( f ;v)e v dv: (5) thetic case. The P-wave velocity used for field data is a lin- vmin early increasing one. Initial S-wave velocities are both lin- early increasing in the synthetic and field examples. The sur- The adjoint form of the optimization problem can be calculated face waves are simulated by solving the elastic wave equation using the adjoint-state method (Plessix, 2006). The model is (equation 2) with a free-surface boundary condition. updated iteratively using the L-BFGS method, which is written as Synthetic S-wave velocity with reversals m = m − lH−1g; (6) 0 We first test our method on a model with velocity reversals where l is the step length calculated by the standard line- (low-velocity layers). The actual P-wave velocity and den- search method. H is the approximated Hessian matrix. sity are shown in Figure 1. There are 40 vertical sources and In summary, our proposed inversion approach includes the fol- 200 receivers evenly distributed on the surface. The maximum lowing steps: frequency used in the inversion is 30 Hz and the spatial sam- pling is 4 m. The actual S-wave velocity as shown in Figure 1. Temporal Fourier transform of the shot gathers. 2a is a layered one with velocity reversals, in which case the fundamental-mode based Rayleigh wave inversion fails.
Details
-
File Typepdf
-
Upload Time-
-
Content LanguagesEnglish
-
Upload UserAnonymous/Not logged-in
-
File Pages6 Page
-
File Size-