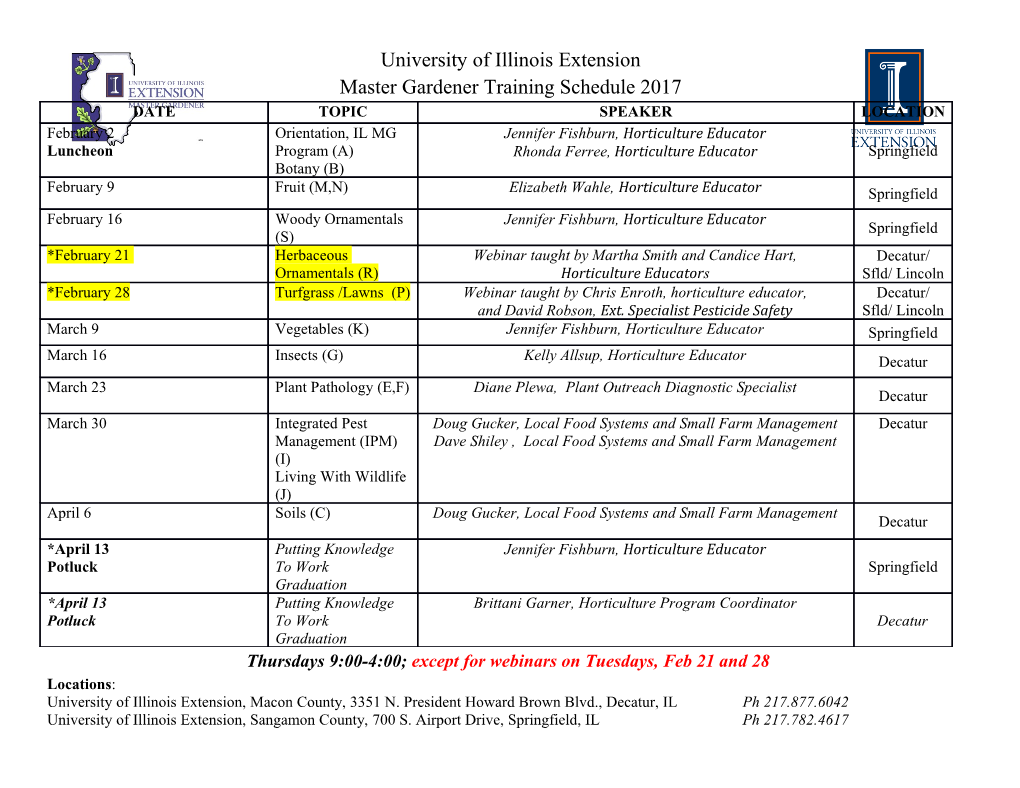
The Matrix Cookbook Kaare Brandt Petersen Michael Syskind Pedersen Version: February 16, 2006 What is this? These pages are a collection of facts (identities, approxima- tions, inequalities, relations, ...) about matrices and matters relating to them. It is collected in this form for the convenience of anyone who wants a quick desktop reference . Disclaimer: The identities, approximations and relations presented here were obviously not invented but collected, borrowed and copied from a large amount of sources. These sources include similar but shorter notes found on the internet and appendices in books - see the references for a full list. Errors: Very likely there are errors, typos, and mistakes for which we apolo- gize and would be grateful to receive corrections at [email protected]. Its ongoing: The project of keeping a large repository of relations involving matrices is naturally ongoing and the version will be apparent from the date in the header. Suggestions: Your suggestion for additional content or elaboration of some topics is most welcome at [email protected]. Keywords: Matrix algebra, matrix relations, matrix identities, derivative of determinant, derivative of inverse matrix, differentiate a matrix. Acknowledgements: We would like to thank the following for contribu- tions and suggestions: Christian Rishøj, Douglas L. Theobald, Esben Hoegh- Rasmussen, Lars Christiansen, and Vasile Sima. We would also like thank The Oticon Foundation for funding our PhD studies. 1 CONTENTS CONTENTS Contents 1 Basics 5 1.1 Trace and Determinants . 5 1.2 The Special Case 2x2 . 5 2 Derivatives 7 2.1 Derivatives of a Determinant . 7 2.2 Derivatives of an Inverse . 8 2.3 Derivatives of Matrices, Vectors and Scalar Forms . 9 2.4 Derivatives of Traces . 11 2.5 Derivatives of Structured Matrices . 12 3 Inverses 15 3.1 Basic . 15 3.2 Exact Relations . 16 3.3 Implication on Inverses . 17 3.4 Approximations . 17 3.5 Generalized Inverse . 17 3.6 Pseudo Inverse . 17 4 Complex Matrices 19 4.1 Complex Derivatives . 19 5 Decompositions 22 5.1 Eigenvalues and Eigenvectors . 22 5.2 Singular Value Decomposition . 22 5.3 Triangular Decomposition . 24 6 Statistics and Probability 25 6.1 Definition of Moments . 25 6.2 Expectation of Linear Combinations . 26 6.3 Weighted Scalar Variable . 27 7 Gaussians 28 7.1 Basics . 28 7.2 Moments . 30 7.3 Miscellaneous . 32 7.4 Mixture of Gaussians . 33 8 Special Matrices 34 8.1 Units, Permutation and Shift . 34 8.2 The Singleentry Matrix . 35 8.3 Symmetric and Antisymmetric . 37 8.4 Vandermonde Matrices . 37 8.5 Toeplitz Matrices . 38 8.6 The DFT Matrix . 39 Petersen & Pedersen, The Matrix Cookbook, Version: February 16, 2006, Page 2 CONTENTS CONTENTS 8.7 Positive Definite and Semi-definite Matrices . 40 8.8 Block matrices . 41 9 Functions and Operators 43 9.1 Functions and Series . 43 9.2 Kronecker and Vec Operator . 44 9.3 Solutions to Systems of Equations . 45 9.4 Matrix Norms . 47 9.5 Rank . 48 9.6 Integral Involving Dirac Delta Functions . 48 9.7 Miscellaneous . 49 A One-dimensional Results 50 A.1 Gaussian . 50 A.2 One Dimensional Mixture of Gaussians . 51 B Proofs and Details 53 B.1 Misc Proofs . 53 Petersen & Pedersen, The Matrix Cookbook, Version: February 16, 2006, Page 3 CONTENTS CONTENTS Notation and Nomenclature A Matrix Aij Matrix indexed for some purpose Ai Matrix indexed for some purpose Aij Matrix indexed for some purpose An Matrix indexed for some purpose or The n.th power of a square matrix A−1 The inverse matrix of the matrix A A+ The pseudo inverse matrix of the matrix A (see Sec. 3.6) A1=2 The square root of a matrix (if unique), not elementwise (A)ij The (i; j).th entry of the matrix A Aij The (i; j).th entry of the matrix A [A]ij The ij-submatrix, i.e. A with i.th row and j.th column deleted a Vector ai Vector indexed for some purpose ai The i.th element of the vector a a Scalar <z Real part of a scalar <z Real part of a vector <Z Real part of a matrix =z Imaginary part of a scalar =z Imaginary part of a vector =Z Imaginary part of a matrix det(A) Determinant of A Tr(A) Trace of the matrix A diag(A) Diagonal matrix of the matrix A, i.e. (diag(A))ij = δijAij vec(A) The vector-version of the matrix A (see Sec. 9.2.2) jjAjj Matrix norm (subscript if any denotes what norm) AT Transposed matrix A∗ Complex conjugated matrix AH Transposed and complex conjugated matrix (Hermitian) A ◦ B Hadamard (elementwise) product A ⊗ B Kronecker product 0 The null matrix. Zero in all entries. I The identity matrix Jij The single-entry matrix, 1 at (i; j) and zero elsewhere Σ A positive definite matrix Λ A diagonal matrix Petersen & Pedersen, The Matrix Cookbook, Version: February 16, 2006, Page 4 1 BASICS 1 Basics (AB)−1 = B−1A−1 (ABC:::)−1 = :::C−1B−1A−1 (AT )−1 = (A−1)T (A + B)T = AT + BT (AB)T = BT AT (ABC:::)T = :::CT BT AT (AH )−1 = (A−1)H (A + B)H = AH + BH (AB)H = BH AH (ABC:::)H = :::CH BH AH 1.1 Trace and Determinants P Tr(A) = Aii Pi Tr(A) = iλi; λi = eig(A) Tr(A) = Tr(AT ) Tr(AB) = Tr(BA) Tr(A + B) = Tr(A) + Tr(B) Tr(ABC) = Tr(BCA) = Tr(CAB) Q det(A) = iλi λi = eig(A) det(AB) = det(A) det(B) det(A−1) = 1= det(A) det(I + uvT ) = 1 + uT v 1.2 The Special Case 2x2 Consider the matrix A A A A = 11 12 A21 A22 Determinant and trace det(A) = A11A22 − A12A21 Tr(A) = A11 + A22 Eigenvalues λ2 − λ · Tr(A) + det(A) = 0 Petersen & Pedersen, The Matrix Cookbook, Version: February 16, 2006, Page 5 1.2 The Special Case 2x2 1 BASICS p p Tr(A) + Tr(A)2 − 4 det(A) Tr(A) − Tr(A)2 − 4 det(A) λ = λ = 1 2 2 2 λ1 + λ2 = Tr(A) λ1λ2 = det(A) Eigenvectors A12 A12 v1 / v2 / λ1 − A11 λ2 − A11 Inverse 1 A −A A−1 = 22 12 det(A) −A21 A11 Petersen & Pedersen, The Matrix Cookbook, Version: February 16, 2006, Page 6 2 DERIVATIVES 2 Derivatives This section is covering differentiation of a number of expressions with respect to a matrix X. Note that it is always assumed that X has no special structure, i.e. that the elements of X are independent (e.g. not symmetric, Toeplitz, positive definite). See section 2.5 for differentiation of structured matrices. The basic assumptions can be written in a formula as @Xkl = δikδlj @Xij that is for e.g. vector forms, @x @x @x @x @x @x = i = = i @y i @y @y i @yi @y ij @yj The following rules are general and very useful when deriving the differential of an expression ([13]): @A = 0 (A is a constant) (1) @(αX) = α@X (2) @(X + Y) = @X + @Y (3) @(Tr(X)) = Tr(@X) (4) @(XY) = (@X)Y + X(@Y) (5) @(X ◦ Y) = (@X) ◦ Y + X ◦ (@Y) (6) @(X ⊗ Y) = (@X) ⊗ Y + X ⊗ (@Y) (7) @(X−1) = −X−1(@X)X−1 (8) @(det(X)) = det(X)Tr(X−1@X) (9) @(ln(det(X))) = Tr(X−1@X) (10) @XT = (@X)T (11) @XH = (@X)H (12) 2.1 Derivatives of a Determinant 2.1.1 General form @ det(Y) @Y = det(Y)Tr Y−1 @x @x 2.1.2 Linear forms @ det(X) = det(X)(X−1)T @X @ det(AXB) = det(AXB)(X−1)T = det(AXB)(XT )−1 @X Petersen & Pedersen, The Matrix Cookbook, Version: February 16, 2006, Page 7 2.2 Derivatives of an Inverse 2 DERIVATIVES 2.1.3 Square forms If X is square and invertible, then @ det(XT AX) = 2 det(XT AX)X−T @X If X is not square but A is symmetric, then @ det(XT AX) = 2 det(XT AX)AX(XT AX)−1 @X If X is not square and A is not symmetric, then @ det(XT AX) = det(XT AX)(AX(XT AX)−1 + AT X(XT AT X)−1) (13) @X 2.1.4 Other nonlinear forms Some special cases are (See [8, 7]) @ ln det(XT X)j = 2(X+)T @X @ ln det(XT X) = −2XT @X+ @ ln j det(X)j = (X−1)T = (XT )−1 @X @ det(Xk) = k det(Xk)X−T @X 2.2 Derivatives of an Inverse From [19] we have the basic identity @Y−1 @Y = −Y−1 Y−1 @x @x from which it follows −1 @(X )kl −1 −1 = −(X )ki(X )jl @Xij @aT X−1b = −X−T abT X−T @X @ det(X−1) = − det(X−1)(X−1)T @X @Tr(AX−1B) = −(X−1BAX−1)T @X Petersen & Pedersen, The Matrix Cookbook, Version: February 16, 2006, Page 8 2.3 Derivatives of Matrices, Vectors and Scalar Forms 2 DERIVATIVES 2.3 Derivatives of Matrices, Vectors and Scalar Forms 2.3.1 First Order @xT a @aT x = = a @x @x @aT Xb = abT @X @aT XT b = baT @X @aT Xa @aT XT a = = aaT @X @X @X = Jij @Xij @(XA)ij mn = δim(A)nj = (J A)ij @Xmn T @(X A)ij nm = δin(A)mj = (J A)ij @Xmn 2.3.2 Second Order @ X X XklXmn = 2 Xkl @Xij klmn kl @bT XT Xc = X(bcT + cbT ) @X @(Bx + b)T C(Dx + d) = BT C(Dx + d) + DT CT (Bx + b) @x T @(X BX)kl T = δlj(X B)ki + δkj(BX)il @Xij T @(X BX) T ij ji ij = X BJ + J BX (J )kl = δikδjl @Xij See Sec 8.2 for useful properties of the Single-entry matrix Jij @xT Bx = (B + BT )x @x @bT XT DXc = DT XbcT + DXcbT @X @ (Xb + c)T D(Xb + c) = (D + DT )(Xb + c)bT @X Petersen & Pedersen, The Matrix Cookbook, Version: February 16, 2006, Page 9 2.3 Derivatives of Matrices, Vectors and Scalar Forms 2 DERIVATIVES Assume W is symmetric, then @ (x − As)T W(x − As) = −2AT W(x − As) @s @ (x − s)T W(x − s) = −2W(x − s) @s @ (x − As)T W(x − As) = 2W(x − As) @x @ (x − As)T W(x − As) = −2W(x − As)sT @A 2.3.3 Higher order and non-linear @ nX−1 aT Xnb = (Xr)T abT (Xn−1−r)T (14) @X r=0 @ nX−1 h aT (Xn)T Xnb = Xn−1−rabT (Xn)T Xr @X r=0 i +(Xr)T XnabT (Xn−1−r)T (15) See B.1.1 for a proof.
Details
-
File Typepdf
-
Upload Time-
-
Content LanguagesEnglish
-
Upload UserAnonymous/Not logged-in
-
File Pages56 Page
-
File Size-