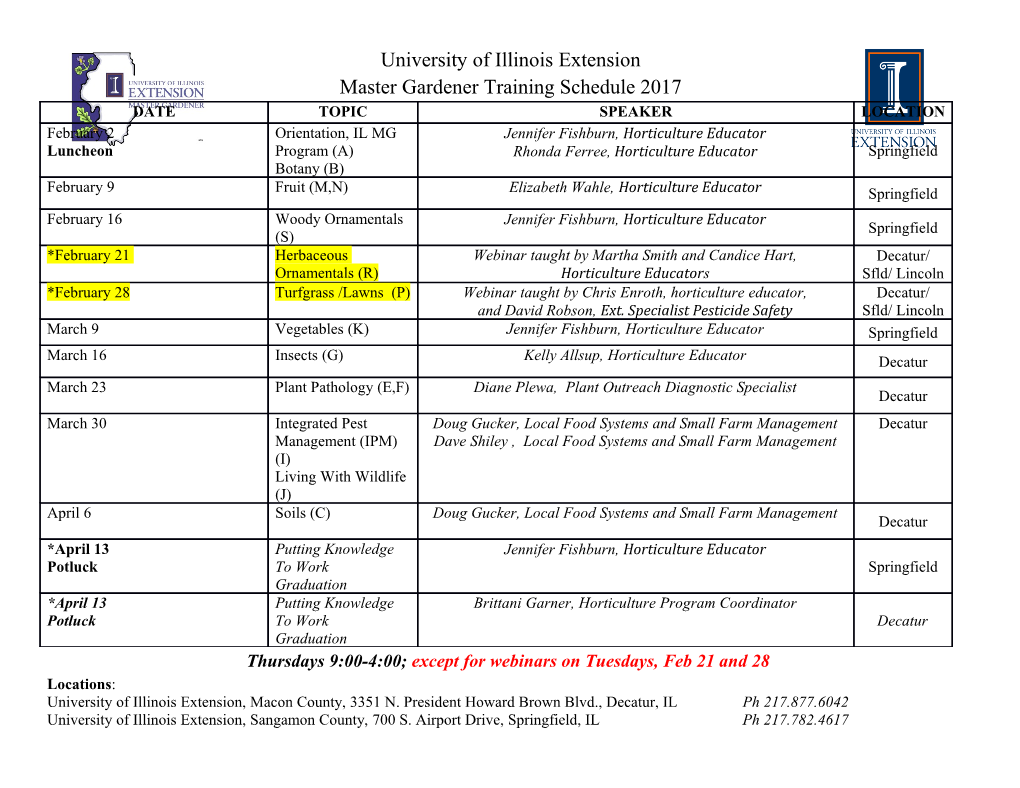
In silico Methods for Drug Repositioning and Drug-Drug Interaction Prediction Pathima Nusrath Hameed ORCID: 0000-0002-8118-9823 Submitted in total fulfilment of the requirements for the degree of Doctor of Philosophy Department of Mechanical Engineering THE UNIVERSITY OF MELBOURNE May 2018 Copyright © 2018 Pathima Nusrath Hameed All rights reserved. No part of the publication may be reproduced in any form by print, photoprint, microfilm or any other means without written permission from the author. Abstract Drug repositioning and drug-drug interaction (DDI) prediction are two fundamental ap- plications having a large impact on drug development and clinical care. Drug reposi- tioning aims to identify new uses for existing drugs. Moreover, understanding harmful DDIs is essential to enhance the effects of clinical care. Exploring both therapeutic uses and adverse effects of drugs or a pair of drugs have significant benefits in pharmacology. The use of computational methods to support drug repositioning and DDI prediction en- able improvements in the speed of drug development compared to in vivo and in vitro methods. This thesis investigates the consequences of employing a representative training sam- ple in achieving better performance for DDI classification. The Positive-Unlabeled Learn- ing method introduced in this thesis aims to employ representative positives as well as reliable negatives to train the binary classifier for inferring potential DDIs. Moreover, it explores the importance of a finer-grained similarity metric to represent the pairwise drug similarities. Drug repositioning can be approached by new indication detection. In this study, Anatomical Therapeutic Chemical (ATC) classification is used as the primary source to determine the indications/therapeutic uses of drugs for drug repositioning. This thesis presents a two-tiered clustering approach for obtaining pairwise drug similarity and het- erogeneous drug data integration which is employed for large-scale drug repositioning. Moreover, this thesis demonstrates subnetwork identification as a suitable approach for new indication detection for existing drugs. Subnetwork identification method iden- tifies a subgraph from a large drug similarity network, connecting a set of given drugs known as ‘terminals’. In this study, the ‘terminals’ are selected according to the ATC iii classification system; hence meaningful subnetworks are identified. The proposed sub- network identification method is employed to infer drug repositioning candidates for cardiovascular diseases and diseases related to the nervous system. New target detection for existing drugs is also beneficial for drug repositioning. This thesis proposes a useful computational method for target clustering which is extended to identify new drug-target relationships. It demonstrates the significance of integrating dimensionality reduction and outlier detection to overcome the limitations arising from the incomplete drug-target interaction data. The clinical significance and literature-based evidence illustrate the relevance of the proposed methods. The proposed methods can be employed in other similar applications where applicable. iv Declaration This is to certify that 1. the thesis comprises only my original work towards the PhD, 2. due acknowledgement has been made in the text to all other material used, 3. the thesis is less than 100,000 words in length, exclusive of tables, maps, bibliogra- phies and appendices. Pathima Nusrath Hameed, May 2018 v This page is intentionally left blank. Acknowledgements I am so grateful to many people who helped me in various ways throughout this journey. First and foremost, my sincere gratitude goes to my supervisors, Professor Saman Halgamuge and Professor Karin Verspoor for their tremendous guidance, feedback, and support throughout my doctoral studies. They spent their valuable time and devotion on supervision and guidance, without which the completion of this research would not have been possible. In many ways, they are great examples for me. Their keen insights, passion for research and life, patience in guiding students, bright ideas, encouragements, and diligence in every means are invaluable. I would also like to thank Karin again for the detailed comments and suggestions. I always feel lucky to have them both as my supervisors. My academic progress was kept on track by periodic observation from my committee chair, Professor James Bailey. I would like to express my thanks to him, for his friendly and constructive feedback and suggestions throughout my PhD career. I sincerely thank Dr. Snezana Kusljic and my colleague Yahui Sun for their collabora- tions. The outcomes of their collaborations have made important pieces of this thesis. I am grateful to The University of Melbourne, Data61, Victoria Research Lab, West Melbourne, Australia, and National ICT Australia for giving me the opportunity to pur- sue my PhD and for the financial support provided by means of postgraduate scholar- ships. I wish to thank all past and present members of the optimization and pattern recog- nition research group for their support, kindness, and friendship. Also, I express my thanks to all my lab mates and friends from The University of Melbourne. I found nice people, with different backgrounds, talking to each other with ease, humility and friend- vii ship. Chalini, thank you for supporting me in the process of university entrance. All staff members of the University of Ruhuna are remembered warmly. I appreciate the invaluable support from Dr. Tharaka Ilayperuma and Dr. MBF Mafasiya for signing my study leave bond agreement. I am indebted to Mr. T N Deen, my uncle and my first teacher who is still providing advice and guidance. Also, my primary, secondary and tertiary teachers are remembered with utmost respect. I would like to thank my cousins and relatives in my close-knit family for their love and blessings. Most importantly, my heartfelt thanks to my parents, my parents-in-law and my sis- ter who always supported me in every possible way with endless and unreserved love, encouragements, unmeasurable sacrifices and blessings. Safraz, you have been my strength and the source of all my happiness. To say thank you would be improper and impossible to list all the reasons why I should do it. I am so happy that you are my husband. I would have never started a PhD if not for your constant persuasion and belief in me. Finally, a big thank you to my lovely daughter. Your curious eyes and bright little smile made the last year of my PhD a joyful one. viii Preface This thesis includes two peer-reviewed journal articles in their published form: • Hameed, P. N., Verspoor, K., Kusljic, S., & Halgamuge, S. (2018). A two-tiered unsupervised clustering approach for drug repositioning through heterogeneous data integration. BMC Bioinformatics, 19(1), 129. • Hameed, P. N., Verspoor, K., Kusljic, S., & Halgamuge, S. (2017). Positive- Unlabeled Learning for inferring drug interactions based on heterogeneous at- tributes. BMC Bioinformatics, 18(1), 140. The two articles, Hameed et al. (2018) and Hameed et al. (2017) are presented in Chapter 3 and Chapter 4, respectively. In both articles, Pathima Nusrath Hameed formu- lated the research questions, developed specific methods and assessed their performance (substantially more than 50%) and is the lead-author. Snezana Kusljic provided the clin- ical relevance of the findings. The co-authors Saman Halgamuge and Karin Verspoor contributed in the supervision of work. Permission was provided by all co-authors to include these articles in their published form in this thesis. In addition to the above articles, content from another peer-reviewed published jour- nal article is included in Chapter 5 and Appendix A: • Sun, Y., Hameed, P. N., Verspoor, K., & Halgamuge, S. (2016). A physarum- inspired prize-collecting steiner tree approach to identify subnetworks for drug repositioning. BMC systems biology, 10(5), 128. In Sun et al. (2016), Pathima Nusrath Hameed is an equal first author. She formulated drug repositioning by Anatomical Therapeutic Chemical Classification as a suitable prob- lem to employ Prize-Collecting Steiner Tree (PCST) based subnetwork identification. She ix also contributed to design, asses and analysis of the experiment. Yahui Sun proposed a specific PCST algorithm and contributed to design and analysis of the experiment. This research was supported by a Melbourne International Research Scholarship, a Melbourne International Fee Remission Scholarship, and a NICTA scholarship of Na- tional ICT Australia, now Data61 since merging CSIRO’s Digital Productivity team. x To my husband for all his love, patience, sacrifices and encouragements... xi This page is intentionally left blank. Contents 1 Introduction 1 1.1 Motivation . .4 1.1.1 Heterogeneous data and pairwise drug similarities . .4 1.1.2 Drug-drug interaction prediction . .5 1.1.3 Drug repositioning . .6 1.1.4 Anatomical Therapeutic Chemical (ATC) classification . .7 1.2 Research focus and thesis outline . .8 1.3 Contributions of this thesis . 15 1.4 Related publications by the author . 16 2 Literature Review 19 2.1 Pharmacological data . 19 2.1.1 Importance of heterogeneous data integration . 24 2.2 Drug repositioning . 27 2.2.1 Network-based inferences . 27 2.2.2 Machine learning-based approaches . 30 2.2.3 Importance of clustering in pharmacological data network analysis 33 2.3 Drug-drug interaction prediction . 35 2.4 Summary . 37 3 Inferring pairwise drug interactions based on heterogeneous attributes 41 3.1
Details
-
File Typepdf
-
Upload Time-
-
Content LanguagesEnglish
-
Upload UserAnonymous/Not logged-in
-
File Pages204 Page
-
File Size-