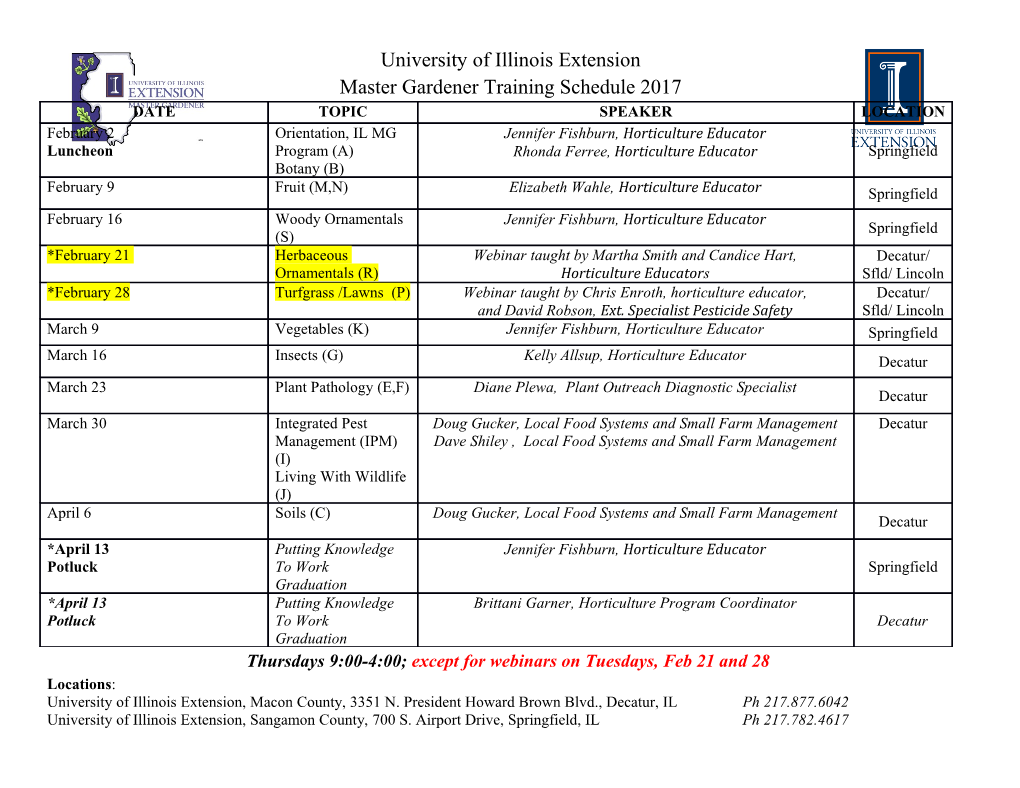
LEVERAGING PERFORMANCE OF 3D FINITE DIFFERENCE SCHEMES IN LARGE SCIENTIFIC COMPUTING SIMULATIONS by Raúl de la Cruz Martínez Advisors: José María Cela Mauricio Araya Polo DISSERTATION Submitted in partial fulfillment of the requirements for the PhD Degree issued by the Department of Computer Architecture Universitat Politècnica de Catalunya Barcelona, Spain October 2015 Abstract Gone are the days when engineers and scientists conducted most of their experiments empir- ically. During these decades, actual tests were carried out in order to assess the robustness and reliability of forthcoming product designs and prove theoretical models. With the ad- vent of the computational era, scientific computing has definetely become a feasible solution compared with empiricial methods, in terms of effort, cost and reliability. The deployment of powerful supercomputers, with thousands of computing nodes, have additionally promoted the extent and use of scientific computing. Large and massively parallel computational re- sources have reduced the simulation execution times and have improved their numerical results due to the refinement of the sampled domain. Several numerical methods coexist for solving the Partial Differential Equations (PDEs) governing the physical phenomena to simulate. Methods such as the Finite Element (FE) and the Finite Volume (FV) schemes are specially well suited for dealing with problems where unstructured meshes are frequent owing to the complex domain to simulate. Unfortunately, this flexibility and versatility are not bestowed for free. These schemes entail higher memory latencies due to the handling of sparse matrices which involve irregular data accesses, there- fore increasing the execution time. Conversely, the Finite Difference (FD) scheme has shown to be an efficient solution for specific problems where the structured meshes suit the problem domain requirements. Many scientific areas use this scheme for solving their PDEs due to its higher performance compared to the former schemes. This thesis focuses on improving FD schemes to leverage the performance of large sci- entific computing simulations. Different techniques are proposed such as the Semi-stencil, a novel algorithm that increases the FLOP/Byte ratio for medium- and high-order stencils oper- ators by reducing the accesses and endorsing data reuse. The algorithm is orthogonal and can be combined with techniques such as spatial- or time-blocking, adding further improvement to the final results. New trends on Symmetric Multi-Processing (SMP) systems —where tens of cores are repli- cated on the same die— pose new challenges due to the exacerbation of the memory wall problem. The computational capability increases exponentially whereas the system band- width only grows linearly. In order to alleviate this issue, our research is focused on different strategies to reduce pressure on the cache hierarchy, particularly when different threads are sharing resources due to Simultaneous Multi-Threading (SMT) capabilities. Architectures with high level of parallelism also require efficient work-load balance to map computational blocks to the spawned threads. Several domain decomposition schedulers for work-load bal- ance are introduced ensuring quasi-optimal results without jeopardizing the overall perfor- mance. We combine these schedulers with spatial-blocking and auto-tuning techniques con- ducted at run-time, exploring the parametric space and reducing misses in last level cache. As alternative to brute-force methods used in auto-tuning, where a huge parametric space must be traversed to find a suboptimal candidate, performance models are a feasible solution. Performance models can predict the performance on different architectures, selecting subop- timal parameters almost instantly. In this thesis, we devise a flexible and extensible perfor- mance model for stencils. The proposed model is capable of supporting multi- and many-core architectures including complex features such as hardware prefetchers, SMT context and al- gorithmic optimizations (spatial-blocking and Semi-stencil). Our model can be used not only to forecast the execution time, but also to make decisions about the best algorithmic parame- ters. Moreover,it can be included in run-time optimizers to decide the best SMT configuration based on the execution environment. Some industries rely heavily on FD-based techniques for their codes, which strongly moti- vates the ongoing research of leveraging the performance. Nevertheless, many cumbersome aspects arising in industry are still scarcely considered in academia research. In this regard, we have collaborated in the implementation of a FD framework which covers the most im- portant features that an HPC industrial application must include. Some of the node-level optimization techniques devised in this thesis have been included into the framework in or- der to contribute in the overall application performance. We show results for a couple of strategic applications in industry: an atmospheric transport model that simulates the dis- persal of volcanic ash and a seismic imaging model used in Oil & Gas industry to identify hydrocarbon-rich reservoirs. To my little Inés, my resilient wife Mónica and my dear family Contents 1 Preface 1 1.1 MotivationofthisThesis . .... 1 1.2 ThesisContributions . .. .. .. .. .. .. .. .. .. .. .. ... 2 1.2.1 ThesisLimitations . .. .. .. .. .. .. .. .. .. .. .. 3 1.3 ThesisOutline................................... 3 1.4 ListofPublications .............................. ... 4 1.5 Acknowledgements................................ 6 2 Introduction 9 2.1 NumericalMethods................................ 9 2.2 FiniteDifferenceMethod. 11 2.3 ImplicitandExplicitMethods . ..... 14 2.4 Summary ...................................... 18 3 Experimental Setup 19 3.1 ArchitectureOverview . 19 3.1.1 IntelXeonX5570(Nehalem-EP) . 19 3.1.2 IBMPOWER6................................ 20 3.1.3 IBMBlueGene/P.............................. 21 3.1.4 AMDOpteron(Barcelona). 22 3.1.5 IBMCell/B.E................................. 22 3.1.6 IBMPOWER7................................ 23 3.1.7 Intel Xeon E5-2670 (Sandy Bridge-EP) . 23 3.1.8 IntelXeonPhi(MIC)............................ 24 3.2 ParallelProgrammingModels . 26 3.3 Programming Languages and Compilers . ..... 27 3.4 PerformanceMeasurement. 28 3.5 STREAM2...................................... 29 3.6 Prefetchers ...................................... 31 3.7 TheRooflineModel................................. 33 3.8 TheStencilProbeMicro-benchmark . ...... 35 3.9 Summary ...................................... 36 i Contents ii 4 Optimizing Stencil Computations 37 4.1 TheStencilProblem ............................... 38 4.2 StateoftheArt ................................... 40 4.2.1 SpaceBlocking ............................... 41 4.2.2 TimeBlocking ............................... 42 4.2.3 PipelineOptimizations . 43 4.3 TheSemi-stencilAlgorithm . 45 4.3.1 Forward and Backward Updates...................... 46 4.3.2 Floating-Point Operations to Data Cache Access Ratio .......... 47 4.3.3 Head, Body and Tail computations..................... 48 4.3.4 OrthogonalAlgorithm . 48 4.4 Experiments..................................... 55 4.4.1 DataCacheAccesses ............................ 55 4.4.2 OperationalIntensity. 57 4.4.3 Performance Evaluation and Analysis . ..... 60 4.4.4 SMPPerformance.............................. 70 4.5 Summary ...................................... 73 5 SMT, Multi-core and Auto-tuning Optimizations 75 5.1 StateoftheArt ................................... 76 5.2 Simultaneous Multithreading Awareness . ........ 78 5.3 Multi-coreandMany-coreImprovements . ....... 81 5.4 Auto-tuningImprovements . 87 5.5 ExperimentalResults . 88 5.6 Summary ...................................... 94 6 Performance Modeling of Stencil Computations 97 6.1 PerformanceModelingOverview . 98 6.2 StateoftheArt ................................... 99 6.3 Multi-LevelCachePerformanceModel . 101 6.3.1 BaseModel ................................. 102 6.3.2 Cache Miss Cases and Rules . 104 6.3.3 Cache Interference Phenomena: II×JJEffect. .. .. .. .. .. .. 106 6.3.4 AdditionalTimeOverheads . 107 6.4 From Single-core to Multi-core and Many-core . ......... 108 6.5 ModelingthePrefetchingEffect . 109 6.5.1 HardwarePrefetching . 109 6.5.2 SoftwarePrefetching. 111 6.6 Optimizations................................... 112 6.6.1 SpatialBlocking .............................. 112 Contents iii 6.6.2 Semi-stencilAlgorithm. 114 6.7 ExperimentalResults . 114 6.7.1 PreliminaryModelResults . 115 6.7.2 AdvancedModelResults . 119 6.8 Summary ......................................126 7 Case Studies 127 7.1 Oil&GasIndustry ................................. 127 7.1.1 RTMOverview ............................... 129 7.1.2 Semi-stencil Implementation in Cell/B.E. ........ 132 7.2 WARISFramework ................................. 136 7.2.1 SystemArchitecture . 137 7.2.2 OptimizationModule. 143 7.3 Atmospheric Transport Modeling - Ash Dispersal . .......... 144 7.3.1 WARIS-Transport Specialization . 145 7.3.2 Volcanic Ash Dispersal Results . 146 7.4 Summary ......................................153 8 Conclusions and Future Work 155 8.1 OptimizingStencilComputations . 155 8.2 SMT, Multi-core and Auto-tuning . 156 8.3 Performance Modeling of Stencil Computations . ......... 158 8.4 CaseStudies..................................... 159 A Numerical Equations 161 A.1 HeatEquation.................................... 161 A.2 WaveEquation ..................................
Details
-
File Typepdf
-
Upload Time-
-
Content LanguagesEnglish
-
Upload UserAnonymous/Not logged-in
-
File Pages191 Page
-
File Size-