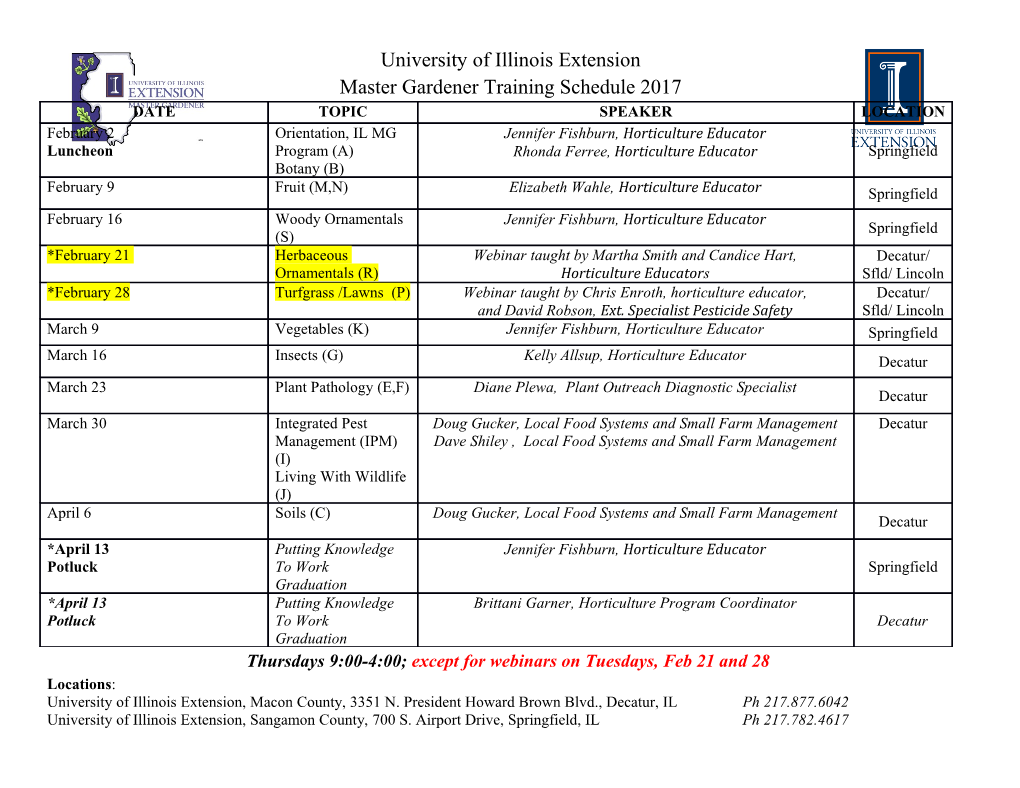
A Service of Leibniz-Informationszentrum econstor Wirtschaft Leibniz Information Centre Make Your Publications Visible. zbw for Economics Berg, Tim Oliver; Henzel, Steffen Working Paper Point and Density Forecasts for the Euro Area Using Bayesian VARs CESifo Working Paper, No. 4711 Provided in Cooperation with: Ifo Institute – Leibniz Institute for Economic Research at the University of Munich Suggested Citation: Berg, Tim Oliver; Henzel, Steffen (2014) : Point and Density Forecasts for the Euro Area Using Bayesian VARs, CESifo Working Paper, No. 4711, Center for Economic Studies and ifo Institute (CESifo), Munich This Version is available at: http://hdl.handle.net/10419/96866 Standard-Nutzungsbedingungen: Terms of use: Die Dokumente auf EconStor dürfen zu eigenen wissenschaftlichen Documents in EconStor may be saved and copied for your Zwecken und zum Privatgebrauch gespeichert und kopiert werden. personal and scholarly purposes. Sie dürfen die Dokumente nicht für öffentliche oder kommerzielle You are not to copy documents for public or commercial Zwecke vervielfältigen, öffentlich ausstellen, öffentlich zugänglich purposes, to exhibit the documents publicly, to make them machen, vertreiben oder anderweitig nutzen. publicly available on the internet, or to distribute or otherwise use the documents in public. Sofern die Verfasser die Dokumente unter Open-Content-Lizenzen (insbesondere CC-Lizenzen) zur Verfügung gestellt haben sollten, If the documents have been made available under an Open gelten abweichend von diesen Nutzungsbedingungen die in der dort Content Licence (especially Creative Commons Licences), you genannten Lizenz gewährten Nutzungsrechte. may exercise further usage rights as specified in the indicated licence. www.econstor.eu Point and Density Forecasts for the Euro Area Using Bayesian VARs Tim O. Berg Steffen R. Henzel CESIFO WORKING PAPER NO. 4711 CATEGORY 12: EMPIRICAL AND THEORETICAL METHODS MARCH 2014 An electronic version of the paper may be downloaded • from the SSRN website: www.SSRN.com • from the RePEc website: www.RePEc.org • from the CESifo website: www.CESifoT -group.org/wp T CESifo Working Paper No. 4711 Point and Density Forecasts for the Euro Area Using Bayesian VARs Abstract Recent articles suggest that a Bayesian vector autoregression (BVAR) with shrinkage is a good forecast device even when the number of variables is large. In this paper we evaluate different variants of the BVAR with respect to their forecast accuracy for euro area real GDP growth and HICP inflation. We consider BVAR averaging, Bayesian factor augmented VARs (BFAVARs), and large BVARs, which differ in the way information is condensed and shrinkage is implemented. We find that: (a) large BVARs produce accurate point forecasts but show a poor performance when the entire density is considered; (b) BVAR averaging shows the opposite pattern; (c) BFAVARs perform well under both evaluation criteria; (d) choosing the degree of shrinkage optimally does not improve forecast accuracy; (e) all variants except the large BVAR tend to be well calibrated for inflation but poorly calibrated for real GDP growth; (f) these findings are robust to several features of the forecast experiment. JEL-Code: C110, C520, C530, E370. Keywords: Bayesian vector autoregression, forecasting, model validation, large cross-section, euro area. Tim O. Berg Steffen R. Henzel Ifo Institute – Leibniz Institute for Ifo Institute – Leibniz Institute for Economic Research at the Economic Research at the University of Munich University of Munich Poschingerstr. 5 Poschingerstr. 5 Germany – 81679 Munich Germany – 81679 Munich [email protected] [email protected] February 28, 2014 We thank Peter Zorn, and participants of the 1stWorkshop on High Dimensional Time Series in Macroeconomics and Finance 2013 (Vienna), the 67th European Meeting of the Econometric Society 2013 (Gothenburg), the Annual Meeting of the German Economic Association 2013 (Düsseldorf), and the Ifo Macro Seminar (Munich) for their helpful comments and suggestions. 1 Introduction When forecasting economic outcomes, a large set of indicators is wishful to avoid model mis- specification. However, forecasting models with large cross-sections are often subject to over- parameterization leading to unstable parameter estimates and inaccurate forecasts. In vector autoregressions (VARs) the number of parameters may easily exceed the number of observa- tions which makes classical estimation infeasible in a data-rich environment. Traditionally, fac- tor models have been used to handle large cross-sections and achieve dimension reduction.1 In a seminal article Ba´nbura, Giannone, and Reichlin (2010) argue, however, that VARs can fore- cast better even when the number of variables is large. They propose Bayesian methods and impose additional information in form of a Minnesota-type prior to shrink the overparameter- ized VAR towards a parsimonious random walk (see also Carriero, Kapetanios, and Marcellino, 2009; Giannone, Lenza, and Primiceri, 2012; D‘Agostino, Gambetti, and Giannone, 2013; Koop, 2013; Carriero, Clark, and Marcellino, 2014). There exist, however, many possible ways to implement a Bayesian VAR (BVAR) for fore- casting. In this paper we build on the results in Ba´nbura et al. (2010) and evaluate different variants of the BVAR which differ in the way information is condensed. In particular, we con- sider BVAR averaging, Bayesian factor augmented VARs (BFAVARs), as well as large BVARs. We also include the random walk variant and the autoregressive (AR) model as benchmarks. Moreover, we consider different specification choices which may also affect the performance of each variant. Along with the aggregation weights of the BVAR averaging and the number of factors of the BFAVAR, there are several ways to choose the degree of shrinkage. We compare the three predominant approaches of the related literature. First, we follow Ba´nbura et al. (2010) and select the shrinkage hyperparameter such that the average in-sample fit for our target vari- ables is the same across variants during a training sample period. Second, we calibrate the hy- perparameter by maximizing the marginal likelihood in each period as in Carriero et al. (2014). Finally, we follow Giannone et al. (2012) and estimate the tightness parameter by modelling it in a hierarchical fashion. Our study thus evaluates all major specification choices discussed in the prevalent literature. To our best knowledge, no one else has yet compared all these ap- proaches within the same setup. The competing approaches are evaluated according to their out-of-sample forecast performance one step and four steps ahead. Specifically, we forecast the quarterly change in the euro area harmonized index of consumer prices (HICP) and the real 1The idea in this literature is that the information contained in a large number of indicator variables can be summarized by a rather small number of factors that are added to the variables of interest (see, e.g., Stock and Watson, 2002, 2005, 2006, 2011; Forni, Hallin, Lippi, and Reichlin, 2003, among others). 1 gross domestic product (GDP). A feature of the analyses in Ba´nbura et al. (2010) and related studies is that the authors com- pare forecast models of fairly different size. For instance Ba´nbura et al. (2010) consider systems with 3, 7, 20, and 131 variables. The BVARs hence produce forecasts conditional on different information sets, making it difficult to identify whether the relative performance of the variants is result of an efficient use of information or a richer information set. While these authors fo- cus on the potential benefits of the latter, we aim at revealing possible differences among the competing approaches with respect to the former. For each variant of the BVAR we ensure that forecasts are produced conditional on all the information that is contained in our dataset. In order to evaluate whether adding indicator variables is useful at all, we compare all variants to both the random walk variant and a univariate AR model. Few studies exist so far which evaluate BVAR forecasts for aggregate euro area data (see, e.g., Giannone, Lenza, Momferatou, and Onorante, 2010, for a rare exception).2 Our dataset comprises up to 44 quarterly macroeconomic and financial indicators for the euro area span- ning the years 1975 to 2011. While applications for the U.S. often build on datasets containing more than one hundred variables, we believe that such large cross-sections are typically not available for most countries. This assumption should at least be true when the time series di- mension is required to be large as well. Thus, it is not clear whether conclusions drawn from the specific case of the U.S. translate to other forecast situations. In our view, we consider a set of indicators that most forecasters would probably label a typical dataset. Moreover, we empha- size at this point that the size of our cross-section is also appropriate with respect to all variants we consider. Even for BFAVARs it has been shown that about 40 series are sufficient to yield satisfactory forecast accuracy (see Bai and Ng, 2002; Boivin and Ng, 2006). On the other hand, it has been documented that large BVARs achieve a good forecast performance with about 20-25 variables (see, e.g., Ba´nbura et al., 2010; Giannone et al., 2012; Koop, 2013). Our baseline results are thus derived from a subset of 22 variables which is similar to that considered in the related literature whereas all 44 variables are considered in a robustness check. While discriminating among BVARs based on the accuracy of their point forecasts is ap- propriate if the loss function of the forecaster depends solely on the forecast error, such a pro- ceeding neglects the uncertainty surrounding the forecasts. Policymakers nowadays closely monitor the uncertainty that is associated with business cycle and inflation developments. The density forecasts of the Bank of England‘s Monetary Policy Committee and the Sveriges Riksbank are prominent examples (see, e.g., Mitchell and Hall, 2005; Boero, Smith, and Wallis, 2Results for Germany are provided by Pirschel and Wolters (2014). 2 2011; Knüppel and Schultefrankenfeld, 2012, among others).
Details
-
File Typepdf
-
Upload Time-
-
Content LanguagesEnglish
-
Upload UserAnonymous/Not logged-in
-
File Pages47 Page
-
File Size-