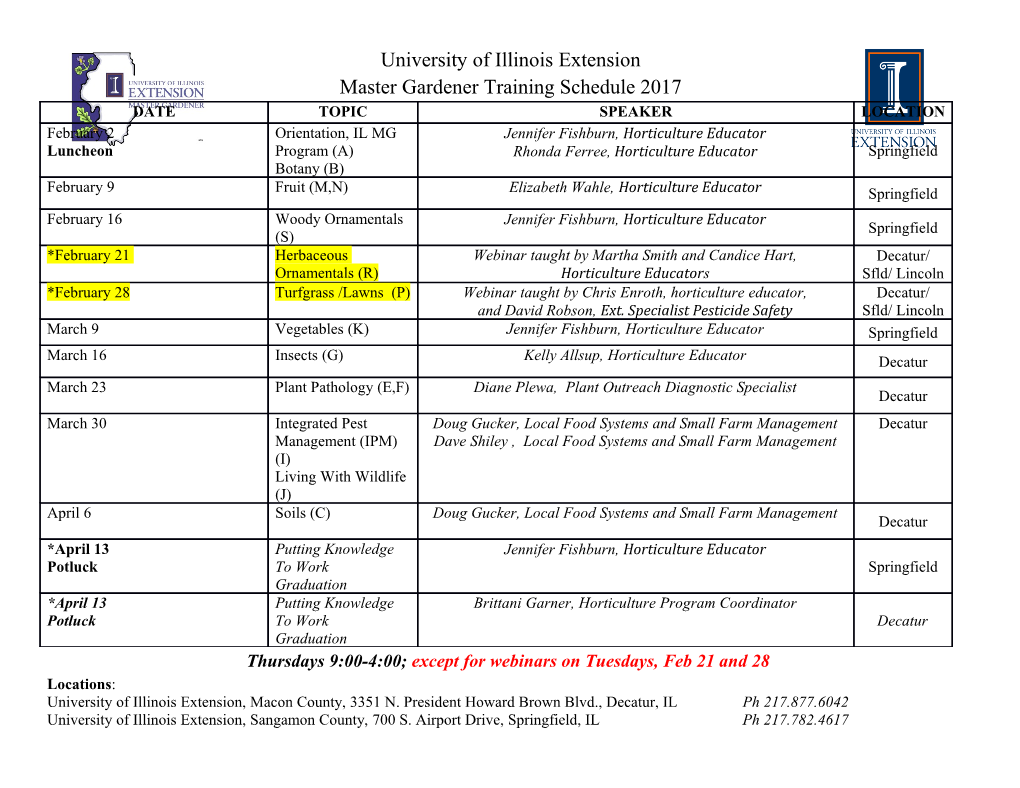
An Unsupervised Method for Discovering Lexical Variations in Roman Urdu Informal Text Abdul Rafae, Abdul Qayyum, Muhammad Moeenuddin, ‡ ‡ Asim Karim, Hassan Sajjad and Faisal Kamiran, Qatar Computing Research† Institute, Hamad‡ Bin Khalifa University Lahore† University of Management Sciences, Information Technology University ‡ Abstract tasks such as Urdu word segmentation (Durrani and Hussain, 2010), part of speech tagging (Saj- We present an unsupervised method to jad and Schmid, 2009), spell checking (Naseem find lexical variations in Roman Urdu and Hussain, 2007), machine translation (Durrani informal text. Our method includes a et al., 2010), etc. phonetic algorithm UrduPhone, a feature- In this paper, we propose an unsupervised based similarity function, and a clustering feature-based method that tackles above men- algorithm Lex-C. UrduPhone encodes ro- tioned challenges in discovering lexical variations man Urdu strings to their phonetic equiv- in Roman Urdu. We exploit phonetic and string alent representations. This produces an similarity based features and incorporate contex- initial grouping of different spelling vari- tual features via top-k previous and next words’ ations of a word. The similarity function features. For phonetic information, we develop an incorporates word features and their con- encoding scheme for Roman Urdu, UrduPhone, text. Lex-C is a variant of k-medoids clus- motivated from Soundex. Compared to other tering algorithm that group lexical varia- available phonetic-based schemes that are mostly tions. It incorporates a similarity thresh- limited to English sounds only, UrduPhone maps old to balance the number of clusters and Roman Urdu homophones effectively. Unlike pre- their maximum similarity. We test our sys- vious work on short text normalization (see Sec- tem on two datasets of SMS and blogs and tion 2), we do not have information about stan- show an f-measure gain of up to 12% from dard word forms in the dataset. The problem be- baseline systems. comes more challenging as every word in the cor- pus is a candidate of every other word. We present 1 Introduction a variant of the k-medoids clustering algorithm Urdu is the national language of Pakistan and one that forms clusters in which every word has at of the official languages of India. It is written in least a specified minimum similarity with the clus- Perso-Arabic script. However in social media and ter’s centroidal word. We conduct experiments on short text messages (SMS), a large proportion of two Roman Urdu datasets: an SMS dataset and Urdu speakers use roman script (i.e., the English a blog dataset and evaluate performance using a alphabet) for writing, called Roman Urdu. gold standard. Our method shows an f-measure Roman Urdu lacks standard lexicon and usu- gain of up to 12% compared to baseline methods. ally many spelling variations exist for a given The dataset and code are made available to the re- word, e.g., the word zindagi [life] is also written as search community. zindagee, zindagy, zaindagee and zndagi. Specifi- 2 Previous Work cally, the following normalization issues arise: (1) differently spelled words (see example above), (2) Normalization of short text messages and tweets identically spelled words that are lexically differ- has been in focus (Sproat et al., 2001; Wei et al., ent (e.g., bahar can be used for both [outside] 2011; Clark and Araki, 2011; Roy et al., 2013; and [spring], and (3) spellings that match words Chrupala, 2014; Kaufmann and Kalita, 2010; in English (e.g, had [limit] for the English word Sidarenka et al., 2013; Ling et al., 2013; Desai ‘had’). These inconsistencies cause a problem of and Narvekar, 2015; Pinto et al., 2012). However, data sparsity in basic natural language processing most of the work is limited to English or to other 823 Proceedings of the 2015 Conference on Empirical Methods in Natural Language Processing, pages 823–828, Lisbon, Portugal, 17-21 September 2015. c 2015 Association for Computational Linguistics. resource-rich languages. In this paper, we focus on which in turn serves as grouping words of similar Roman Urdu, an under-resourced language, that sounds (lexical variations) to one code. However, does not have any gold standard corpus with stan- most of the schemes are designed for English and dard word forms. Therefore, we are restricted to European languages and are limited when apply to the task of finding lexical variations in informal other family of languages like Urdu. text. This is a rather more challenging problem In this work, we propose a phonetic encoding since in this case every word is a possible varia- scheme, UrduPhone, tailored for Roman Urdu. tion of every other word in the corpus. The scheme is derived from the Soundex algo- Researchers have used phonetic, string, and rithm. It groups consonants on the basis of com- contextual knowledge to find lexical variations in mon homophones in Urdu and English. It is differ- informal text.1 Pinto et al. (2012; Han et al. ent from Soundex in two particular ways:3 Firstly, (2012; Zhang et al. (2015) used phonetic-based UrduPhone generates encoding of length six com- methods to find lexical variations. Han et al. pared to length four in Soundex. This enables (2012) also used word similarity and word con- UrduPhone to avoid mapping different forms of text to enhance performance. Wang and Ng (2013) a root word to same code. For example, musku- used normalization operations e.g., missing word rana [smiling] and mshuraht [smile] encode to recovery and punctuation correction to improve one form MSKR in Soundex but in UrduPhone, normalization process. Irvine et al. (2012) used they have different encoding which are MSKRN, manually prepared training data to build an au- MSKRHT respectively. Secondly, we introduce tomatic normalization system. Contractor et al. consonant groups which are mapped differently in (2010) used string edit distance to find candidate Soundex. We do this by analyzing Urdu alpha- lexical variations. Yang and Eisenstein (2013) bets that map to a single roman form e.g. words used an unsupervised approach with log linear samar [reward], sabar [patience] and saib [apple], model and sequential Monte Carlo approximation. all start with different Urdu alphabets that have We propose an unsupervised method to find lex- identical roman representation: s. In UrduPhone, ical variations. It uses string edit distance like we map all such cases to a single form.4 Contractor et al. (2010), Sound-based encoding like Pinto et al. (2012) and context like Han et al. 3.2 Similarity Function (2012) combined in a discriminative framework. The similarity between two words wi and wj is However, in contrast, it does not use any corpus of computed by the following similarity function: standard word forms to find lexical variations. F (f) (f) f=1 α σij S(wi, wj) = × F (f) 3 Our Method P f=1 α P The lexical variations of a lexical entry usually Here, α(f) > 0 is the weight given to feature f, have high phonetic, string-based, and contextual (f) σ [0, 1] is the similarity contribution made by similarity. We integrate a phonetic-based encod- ij ∈ feature f, and F is the total number of features. ing scheme, UrduPhone, a feature-based similarity In the absence of additional information, and as function, and a clustering algorithm, Lex-C. used in the experiments in this work, all weights 3.1 UrduPhone can be taken equal to one. The similarity function returns a value in the interval [0, 1] with larger Several sound-based encoding schemes for words values signifying higher similarity. have been proposed in literature such as Soundex We use two types of features in our method: (Knuth, 1973; Hall and Dowling, 1980), NYSIIS word features and contextual features. Word fea- (Taft, 1970), Metaphone (Philips, 1990), Caver- tures can be based on phonetics and/or string sim- phone (Wang, 2009) and Double Metaphone.2 ilarity. The phonetic similarity between words wi These schemes encode words based on their sound and wj is 1 (i.e., σij = 1) if both words have the 1Spell correction is also considered as a variant of text same UrduPhone ID or encoding; otherwise, their normalization (Damerau, 1964; Tahira, 2004; Fossati and Di Eugenio, 2007). Here, we limit ourselves to the previous 3Due to limited space, we limit the description of Urdu- work on short text normalization. Phone to its comparison with Soundex. 2http://en.wikipedia.org/wiki/ 4A complete table of UrduPhone mappings is provided in Metaphone the supplementary material. 824 k similarity is zero. The string similarity between satisfied (i.e., S(wi, wc ) < t) then instead of as- words wi and wj is defined as follows: signing word wi to cluster k, it starts a new cluster. These two steps are repeated until convergence. lcs(w , w ) σ = i j ij min[len(w ), len(w )] edist(w , w ) i j × i j 4 Experimental Evaluation Here, lcs(wi, wj) is the length of the longest com- We empirically evaluate UrduPhone and our com- mon subsequence in words wi and wj and len(wi) plete method involving Lex-C separately on two is the length of word wi. edist(wi, wj) returns the real-world datasets. Performance is reported with edit distance between words except when the edit B-Cubed precision, recall, and f-measure (Bagga distance is 0, in which case it returns 1. and Baldwin, 1998; Hassan et al., 2015) on a gold Contextual features include top-k frequently oc- standard dataset. These performance measures are curring previous and next words’ features. Let based on element-wise comparisons between pre- i i i j j j a1, a2, . , a5 and a1, a2, . , a5 be the word IDs dicted and actual clusters that are then aggregated for the top-5 frequently occurring words preceding over all elements in the clustering.
Details
-
File Typepdf
-
Upload Time-
-
Content LanguagesEnglish
-
Upload UserAnonymous/Not logged-in
-
File Pages6 Page
-
File Size-