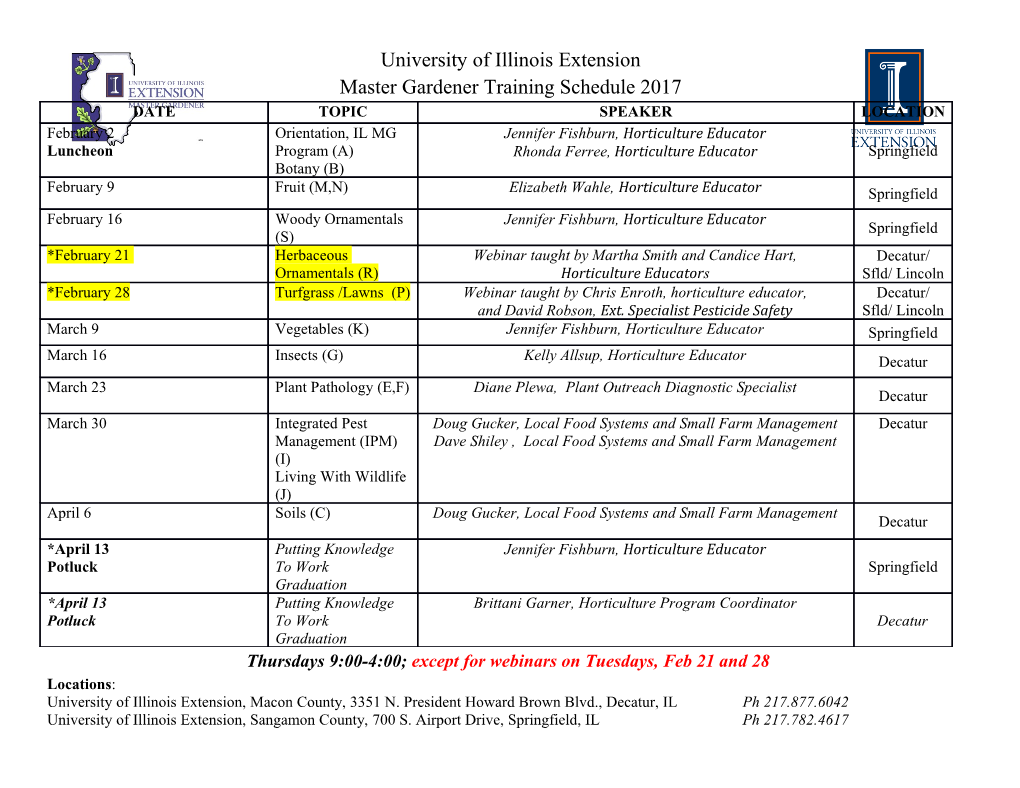
Physics 116A Fall 2019 Rotation Matrices in two, three and many dimensions 1. Proper and improper rotation matrices in n dimensions A matrix is a representation of a linear transformation, which can be viewed as a machine that consumes a vector and spits out another vector. A rotation is a trans- formation with the property that the vector consumed by the machine and the vector spit out by the machine have the same length. That is, physically rotating a vector by an angle θ leaves the length of the vector unchanged. As a matrix equation, if R is a rotation matrix and ~v is a vector, then w~ = R~v , where w~ = ~v . (1) k k k k In eq. (1), the length of the vector ~v is denoted by ~v . Note that if ~v is a vector in k k an n-dimensional space, then in terms of the components of ~v = (v1, v2,...,vn) and w~ =(w1,w2,...,wn), eq. (1) is equivalent to n wi = Rijvj , (2) j=1 X where Rij are the matrix elements of the rotation matrix R. In addition, the length of the vector ~v is given by the n-dimensional generalization of the Pythagorean theorem, 2 2 2 ~v = v1 + v2 + ... + v , (3) k k n q with a similar formula for the length of w~ . Let us now ask the following question: what is the most general form of the matrix R such that the lengths of the vectors ~v and w~ are equal, as specified in eq. (1)? That is, what is the most general form of R in eq. (2) such that n n wiwi = vivi , (4) i=1 i=1 X X which is the condition that must be satisfied if ~v = w~ in light of eq. (3). To answer this question, we insert eq. (2) into the leftk handk sidek k of eq. (3). Here, we must be careful with indices and write: n n wiwi = Rijvj Rikvk . (5) j=1 k=1 X X The second time we write wi as a sum on the right hand side of eq. (5), one must use a different summation index (e.g., k), since the index j has already been used as a 1 summation index in the first sum. The best way to see this is to take a simple example, say with i = 1 and n = 2. Then eq. (5) yields 2 w1 =(R11v1 + R12v2)(R11v1 + R12v2) . (6) It would be wrong to write n wiwi = RijvjRijvj , WRONG!, j=1 X 2 2 2 2 2 which in our simple example with i = 1 and n = 2 would yield w1 = R11v1 + R12v2, thereby missing the cross terms that appear when multiplying out the two factors on the right hand side of eq. (6). Since the sums in eq. (5) consist of a finite number of terms, one can move the summation signs to the left and reorder the terms being multiplied to obtain, n n wiwi = RijRikvjvk . (7) j=1 k=1 X X Next, we sum over the free index i on both sides of eq. (7) and use eq. (4) to obtain, n n n n n wiwi = RijRikvjvk = vivi . (8) i=1 i=1 j=1 k=1 i=1 X X X X X One can now use the following trick. Recalling the definition of the Kronecker delta symbol, 1 , if j = k, δjk = (9) 0 , if j = k, ( 6 it follows that the right hand side of eq. (8) can be rewritten as n n n vivi = δjkvjvk . (10) i=1 j=1 k=1 X X X As this is one of the crucial steps in the analysis, you should convince yourself that eq. (10) is an identity (e.g., by examining the case of n = 2 and writing out the sums explicitly). One can now insert eq. (10) back into eq. (8) to obtain, n n n n n RijRikvjvk = δjkvjvk . (11) i=1 j=1 k=1 j=1 k=1 X X X X X Interchanging the order of summation, n n n n n RijRikvjvk = δjkvjvk . (12) j=1 k=1 i=1 j=1 k=1 X X X X X 2 Moving the right hand side above over to the left hand side of eq. (12) yields, n n n RijRik δjk vjvk =0 . (13) − j=1 k=1 " i=1 ! # X X X Let us denote the expression inside the square brackets as, n Ajk RijRik δjk , (14) ≡ i=1 ! − X in which case, eq. (13) is equivalent to n n Ajkvjvk =0 . (15) j=1 k=1 X X In the above analysis, I never specified a specific value for the vj. In other words, if one were to rotate any vector ~v, the end result would still be eq. (15). This observation implies that eq. (15) must be true for any choice of the vj. Consequently, it must be 1 true that Ajk = 0 for all j, k =1, 2,...,n. Inserting Ajk = 0 into the left hand side of eq. (14) yields out final result, n RijRik = δjk . (16) i=1 X To interpret eq. (16), I remind you that the jk element of the product of two n n × matrices is given by the formula [cf. eq. (9.3) in Chapter 3, p. 138 of Boas], n (BC)jk = BjiCik . (17) i=1 X Inserting B = RT and C = R in eq. (17) and using eq. (16) then yields the matrix equation RTR = I. In obtaining this result, we noted that the matrix elements of the identity matrix are Iij = δij, and the transpose of a matrix interchanges the rows and T columns, so that (R )ji = Rij. In conclusion, eq. (16) in matrix form is equivalent to the statement that RTR = I . (18) A matrix R that satisfies eq. (18) is called an orthogonal matrix. Multiplying both sides of eq. (18) on the right by R−1 and using RR−1 = I yields, RT = R−1 . (19) 1 For example, choose ~v = (1, 0, 0,..., 0) and insert this choice in eq. (15) to obtain A11 = 0. Now keep on choosing vectors with different components, and eventually you will see that Ajk = 0 for all j, k =1, 2,...,n. 3 which can also be used as the definition of an orthogonal matrix. Finally, multiplying both sides of eq. (19) on the left by R and again using RR−1 = I yields, RRT = I . (20) Hence, orthogonal matrices are matrices that satisfy R−1 = RT, or equivalently satisfy, RTR = RRT = I . (21) We conclude that rotation matrices must be real orthogonal matrices. Next, consider the following question. Can any real n n orthogonal matrix be identified as some rotation matrix in n-dimensional space× [which is the converse of statement following eq. (21)]? To gain some insight, let us take the determinant of both sides of eq. (18). Recall that det I = 1 and that for any n n matrices B and C, we have det(BC) = (det B)(det C). Finally, for any matrix B,× we have det(BT) = det B. Making use of these properties, eq. (18) yields, det(RTR) = (detRT)(detR) = (det R)2 = det I =1 . (22) It then follows that2 det R = 1 . (23) ± That is, some real orthogonal matrices have determinant equal to 1, whereas other real orthogonal matrices have determinant equal to 1. In these notes, I shall explore the explicit forms for rotation matrices in 2 and 3 dimensions.− Extending those results to n dimensions, one can show that any real n n orthogonal matrix with determinant equal to 1 can be identified as some rotation matrix× in n dimensions. A real orthogonal matrix with determinant equal to 1 can be identified as a product of a rotation and − a reflection. The above observation leads to the following nomenclature. A real orthogonal matrix R with det R = 1 provides a matrix representation of a proper rotation. The most general rotation matrix R represents a counterclockwise rotation by an angle θ about a fixed axis that is parallel to the unit vector nˆ.3 The rotation matrix operates on vectors to produce rotated vectors, while the coordinate axes are held fixed. In typical parlance, a rotation refers to a proper rotation. Thus, in the following sections of these notes we will often omit the adjective proper when referring to a proper rotation. A real orthogonal matrix R with det R = 1 provides a matrix representation of an improper rotation.4 To perform an improper− rotation requires mirrors. That is, the most general improper rotation matrix is a product of a proper rotation by an angle θ about some axis nˆ and a mirror reflection through a plane that passes through the origin and is perpendicular to nˆ. 2Note that eq. (23) implies that detR = 0. This result implies that the inverse of a rotation matrix, R−1 always exist. We implicitly used this6 fact to go from eq. (18) to eq. (19), so this step is now justified. 3The corresponding inverse matrix R−1 represents a clockwise rotation by an angle θ about a fixed axis that is parallel to the unit vector nˆ. With this observation, you can quickly check that RR−1 = I, as expected. 4To distinguish matrices representing improper rotations from those of proper rotations, we shall employ the notation R to denote an improper rotation matrix. 4 Finally, it is instructive to consider the following question: how many real indepen- dent parameters (which we shall denote by N below) describe a real orthogonal n n × matrix? To answer this question, we start with eq.
Details
-
File Typepdf
-
Upload Time-
-
Content LanguagesEnglish
-
Upload UserAnonymous/Not logged-in
-
File Pages17 Page
-
File Size-