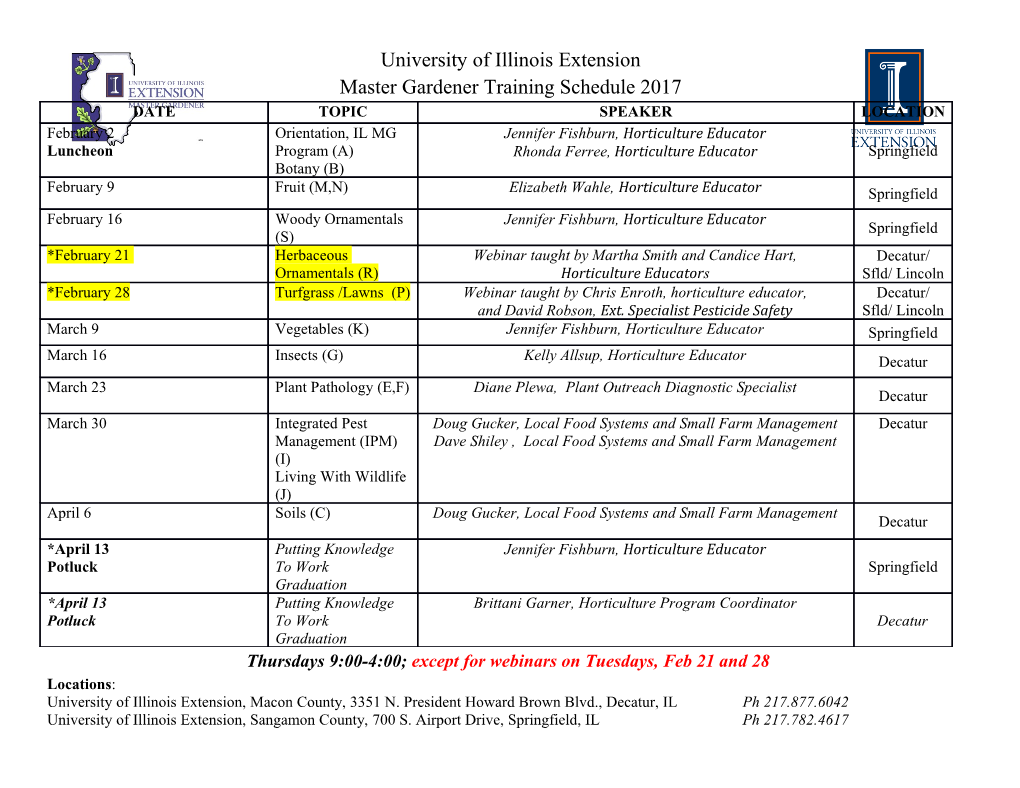
• Tues, Nov 30: Pairwise sequence alignment Gene Finding 1 Online FCE’s: Thru Dec 10 (global and local) • Thurs, Dec 2: Gene Finding 2, PS5 due • Tues, Dec 7: Multiple sequence alignment Project presentations 1 • Thurs, Dec 9 Substitution Database Project presentations 2 matrices searching Final papers due • Tues, Dec 14: global local BLAST DD: Extended office hours: 2:30pm – 5:30pm, MI 650 Sequence • Wed, Dec 15 statistics NS: office hours. DH 1321, noon – 2pm. • Friday Dec 17 Prokaryotic Gene Finding 8:30am Final Exam, Room: TBA Evolutionary tree reconstruction Eukaryotic Gene Finding What is a Gene? Prokaryotic Gene Finding Snyder and Gerstein, Science 2003 • Something that encodes a heritable trait • One gene, one enzyme • Identify Open Reading Frames (ORFs) • One gene,one polypeptide • Coding Statistics • One gene,one product (include RNA products) • Identify individual gene architecture features • “a complete chromosomal segment responsible for • Assemble an integrated gene description making a functional product” • Homology – coding region – regulatory region – expressed product – functional product Reading Frames Open Reading Frames An ORF is a contiguous set of codons, each specifying an A C G T A A C T G A C T A G G T G A A T amino acid (starting with ATG). ..C G T A A C T G A C T A G G T G A A.. ...G T A A C T G A C T A G G T G A A T . GGGAGCATGGTGCACCTGACTCCTGAGGTGACTTAGAC M V H L T P E V T Stop • Each grouping of the nucleotides into consecutive triplets constitutes a reading frame. All coding sequences are ORF's, but not all ORF's encode proteins • Three reading frames in the 5’->3’direction • Three in the reverse direction on the opposite strand. 1 Coding Statistics Prokaryotic Gene Finding Fickett and Tung,1992 Guigo and Fickett,1995 (Electronicreserves) • Identify Open Reading Frames (ORFs) • Codon usage • Coding Statistics – Determine codon (triplet) frequencies in known • Identify individual gene architecture features coding regions – Compare with codon frequencies in sliding • Assemble an integrated gene description window • Homology • Amino acid pair preference • CG content ccgcctggcgtcgcggtttgtttttcatctctcttcatctgca CodingStatistics CodingStatistics Fickett and Tung,1992 Fickett and Tung,1992 Guigo and Fickett,1995 Guigo and Fickett,1995 (Electronicreserves) (Electronicreserves) • Codon usage Species specific • Codon usage Species specific • Codon pair preference Species specific • Codon pair preference Species specific • Correlations in third base position • Amino acid usage Species specific • Amino acid usage • Amino acid pair preference • Amino acid pair preference • CG content • CG content Gly Val AlaVal Cys Phe Ser ccgcctggcgtcgcggtttgtttttcatctctcttcatctgca ccgcctggcgtcgcggtttgtttttcatctctcttcatctgca CodingStatistics CodingStatistics Fickett and Tung,1992 Fickett and Tung,1992 Guigo and Fickett,1995 Guigo and Fickett,1995 (Electronicreserves) (Electronic reserves) • Codon usage Species specific • Codon usage Species specific • Codon pair preference Species specific • Codon pair preference Species specific • Amino acid usage Species specific • Amino acid usage Species specific • Amino acid pair preference Species specific • Amino acid pair preference Species specific • Third position Any organism • Correlations in third base position –3rd base tends to be the same much more often • CG content than chance Gly Val AlaVal Cys Phe Ser Ser • CG content ccgcctggcgtcgcggtttgtttttcatctctcttcatctgca ccgcctggcgtcgcggtttgtttttcatctctcttcatctgca 2 Coding Statistics continued CodingStatistics Fickett and Tung,1992 Fickett and Tung,1992 Guigo and Fickett,1995 Guigo and Fickett,1995 (Electronicreserves) (Electronicreserves) CG content Species specific • Codon usage Species specific In E. coli: • Codon pair preference Species specific Coding regions are embedded in segments of uniform, Species specific 53% G+C, about 1000 bases long • Amino acid usage Non-coding regions are embedded in segments of • Amino acid pair preference Species specific uniform, 46% G+C, about 500 bases long • Third position Any organism aa, at, ta, tt occur more frequently than expected in coding regions • CG content Species specific tgccgcctggcgtcgcggtttctttttcatctctcttcatctg Look for variations in these measures in coding and non-coding regions acggcggaccgcagcgccaaagaaaaagtagagagaagtagacc (intergenic and intragenic). DNA PATTERNS IN THE E.coli lexA GENE Prokaryotic Gene Finding Promotor sequences PATTERN Repressor binding site 1 gaattcgataaatctctggtttattgtgcagtttatggttccaaaatcgccttttgctgt CTGNNNNNNNNNNCAG TTCCAA -35 TTGACA • Identify Open Reading Frames (ORFs) 61 atatactcacagcataactgtatatacacccagggggcggaatgaaagcgttaacggcca TATAAT, mRNA start GGAGG -10 TATACT mRNAstart+ +10GGGGG Ribosomal binding site • Coding Statistics 121 ggcaacaagaggtgtttgatctcatccgtgatcacatcagccagacaggtatgccgccga 181 cgcgtgcggaaatcgcgcagcgtttggggttccgttccccaaacgcggctgaagaacatc • Identify individual gene architecture features 241 tgaaggcgctggcacgcaaaggcgttattgaaattgtttccggcgcatcacgcgggattc 301 gtctgttgcaggaagaggaagaagggttgccgctggtaggtcgtgtggctgccggtgaac 361 cacttctggcgcaacagcatattgaaggtcattatcaggtcgatccttccttattcaagc • Assemble an integrated gene description ATG…TAA 421 cgaatgctgatttcctgctgcgcgtcagcgggatgtcgatgaaagatatcggcattatgg open reading frame 481 atggtgacttgctggcagtgcataaaactcaggatgtacgtaacggtcaggtcgttgtcg • Homology 541 cacgtattgatgacgaagttaccgttaagcgcctgaaaaaacagggcaataaagtcgaac 601 tgttgccagaaaatagcgagtttaaaccaattgtcgttgaccttcgtcagcagagcttca 661 ccattgaagggctggcggttggggttattcgcaacggcgactggctgtaacatatctctg 721 agaccgcgatgccgcctggcgtcgcggtttgtttttcatctctcttcatcaggcttgtct 781 gcatggcattcctcacttcatctgataaagcactctggcatctcgccttacccatgattt 841 tctccaatatcaccgttccgttgctgggactggtcgatacggcggtaattggtcatcttg 901 atagcccggtttatttgggcggcgtggcggttggcgcaacggcggaccagct Prokaryotic Gene Finding Homology • Identify Open Reading Frames (ORFs) • Coding Statistics • Identify individual gene architecture features • Assemble an integrated gene description • Homology Salzberg, Nature 2003 3 Prokaryotic Gene Finding Gene Finding Questions • Genome length: 0.5M bp – 10Mbp • Identify protein coding region • Coding density: ~90% • Identify Open Reading Frame • Long ORFs are usually real genes • Predict mRNA (including UTR’s) • Predict intron/exon structure Early approaches – Identify ORFs Eukaryotes only – Score windows with coding statistics • Regulatory signals – Identify gene structure elements • Protein sequence • Parse into a coherent gene model surrounded by intergenic DNA. An HMM that finds genes in E. coli Prokaryotic gene model Krogh et al,1995 (Electronic reserves) 5’ 3’ A A A observed frequencies for E. coli genes A A C … 61 triplet models Open Reading Frame Untranslated regions (UTRs) T T T Promoter region Ribosome binding site Termination sequence Start codon/Stop codon start codons stop codons Repressor site intergene model Codon models intergene model A C account for G T Example: TTT sequencing errors d0 d0 d0 A A A C C C G G G i i i T T T A A A i0 1 2 3 C C C G G G A A A T T T A A A C C C C C C G G G start codons Begin G G G End T T T T T T stop codons 4 Refinements Parameter estimation observed frequencies Krogh et al,1995 for E. coli genes coding region (Electronic reserves) • Data: 429 E. coli contigs model • Trained intergenic models with non-coding DNA • Transitions into coding model were observed codon overlap model frequencies in coding regions Training Test Contigs 300 129 Base pairs 1,271,528 324,684 long intergene model Genes 1007 251 start codons Av length 1008 1015 stop codons short Results Performance measures Perfect atg taa reality prediction • Exact locations of ~80% of known genes atg taa • Approximate locations of ~10% of known genes Almost perfect • About half of the false negatives were genes with atg reality unusual codon usage. prediction atg • Predicted genes: 286 About 150 were similar to known genes <10 Partly reality prediction >50% or >60 bp Outstanding Problems Outstanding Problems • Model cannot account for drift in CG content • Model cannot account for drift in CG content • Does not take position dependencies into • Does not take position dependencies into account account • Solution: A A A … – kth order Markov chain T T T – looks back k positions 5 First-order Markov chain Second-order Markov chain Example: transmembrane region model Example: transmembrane Transition matrix: region model Transition matrix: H L L H H L L H H L P[i, j] H H L P[i, j,k] L H: hydrophobic H: hydrophobic L: hydrophilic L: hydrophilic P(xt = i | xt−1 = j) P(xt = i | xt−1 = j, xt−2 = k) A second-order Markov chain can be expressed as a first order Markov chain with more states and Glimmer transitions Salzberg et al,1998 • Prokaryotic gene finder HH LH HL LL • Finds 98% of all genes in a bacterial genome HH LH HH • Genome independent – Uses all large, non-overlapping ORFs as training data LH • kth order Markov chain P(x = (ij) | x = ( jk)) HL LL HL t t−1 – (looks back k positions) • Higher order Markov models require more training LL data Pairwise sequence alignment (global and local) Multiple sequence alignment Substitution matrices Database searching global local BLAST Sequence statistics Prokaryotic Gene Finding Evolutionary tree reconstruction Eukaryotic Gene Finding 6.
Details
-
File Typepdf
-
Upload Time-
-
Content LanguagesEnglish
-
Upload UserAnonymous/Not logged-in
-
File Pages6 Page
-
File Size-