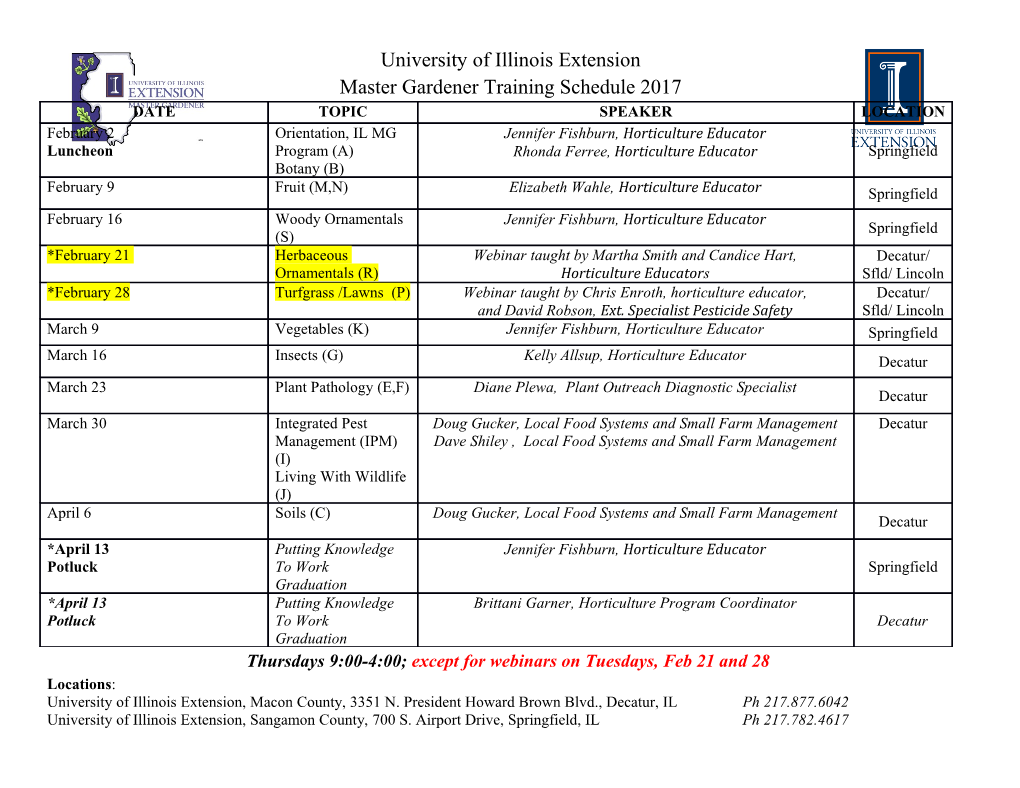
Problem Description MML GLM Ridge Regression Results/Examples Minimum Message Length Ridge Regression for Generalized Linear Models Daniel F. Schmidt and Enes Makalic Centre for Biostatistics and Epidemiology The University of Melbourne 26th Australasian Joint Conference on Artificial Intelligence Dunedin, New Zealand 2013 Problem Description MML GLM Ridge Regression Results/Examples Outline 1 Problem Description Generalized Linear Models GLM Ridge Regression 2 MML GLM Ridge Regression Minimum Message Length Inference Message Lengths of GLMs 3 Results/Examples Parameter Estimation Experiments Example: Dog Bite Data Conclusion Problem Description Generalized Linear Models MML GLM Ridge Regression GLM Ridge Regression Results/Examples Outline 1 Problem Description Generalized Linear Models GLM Ridge Regression 2 MML GLM Ridge Regression Minimum Message Length Inference Message Lengths of GLMs 3 Results/Examples Parameter Estimation Experiments Example: Dog Bite Data Conclusion Problem Description Generalized Linear Models MML GLM Ridge Regression GLM Ridge Regression Results/Examples Generalized Linear Models (GLMs) (1) We have A vector of targets, y ∈ Rn 0 0 0 n×p A matrix of features, X = (x¯1,..., x¯n) ∈ R ⇒ Usual problem is to use X to predict y If targets are reals, linear-normal model is a standard choice yi ∼ N(ηi, τ) where ηi = x¯iβ + α, is the linear predictor, and β ∈ Rp are the coefficients α ∈ R is the intercept Problem Description Generalized Linear Models MML GLM Ridge Regression GLM Ridge Regression Results/Examples Generalized Linear Models (GLMs) (1) We have A vector of targets, y ∈ Rn 0 0 0 n×p A matrix of features, X = (x¯1,..., x¯n) ∈ R ⇒ Usual problem is to use X to predict y If targets are reals, linear-normal model is a standard choice yi ∼ N(ηi, τ) where ηi = x¯iβ + α, is the linear predictor, and β ∈ Rp are the coefficients α ∈ R is the intercept Problem Description Generalized Linear Models MML GLM Ridge Regression GLM Ridge Regression Results/Examples Generalized Linear Models (GLMs) (2) What if the targets are not continuous variables? Binary data (classification problems) Integers, counts ⇒ We can use generalized linear models (GLMs) GLM framework assumes target distribution satisfies E[yi|µi] = µi var [yi|µi, φ] = φ v(µi) −1 where µi = f (ηi), f(·) is called a link function, v(·) is the variance function, and φ is a dispersion parameter. Problem Description Generalized Linear Models MML GLM Ridge Regression GLM Ridge Regression Results/Examples Generalized Linear Models (GLMs) (2) What if the targets are not continuous variables? Binary data (classification problems) Integers, counts ⇒ We can use generalized linear models (GLMs) GLM framework assumes target distribution satisfies E[yi|µi] = µi var [yi|µi, φ] = φ v(µi) −1 where µi = f (ηi), f(·) is called a link function, v(·) is the variance function, and φ is a dispersion parameter. Problem Description Generalized Linear Models MML GLM Ridge Regression GLM Ridge Regression Results/Examples Estimating the model (1) Let p(yi|β, α, φ) denote the probability distribution for yi In general β, α, φ are unknown ⇒ Must be estimated from the data y One powerful estimation procedure is ridge regression ( n ) n o X βˆ, α,ˆ φˆ = arg min − log p(yi|β, α, φ) α∈R,φ∈R+,β∈S(c) i=1 where p 0 S(c) = β ∈ R : β Σβ ≤ c Hyperparameter c controls the amount of regularisation ⇒ As c → ∞, we recover maximum likelihood Problem Description Generalized Linear Models MML GLM Ridge Regression GLM Ridge Regression Results/Examples Estimating the model (1) Let p(yi|β, α, φ) denote the probability distribution for yi In general β, α, φ are unknown ⇒ Must be estimated from the data y One powerful estimation procedure is ridge regression ( n ) n o X βˆ, α,ˆ φˆ = arg min − log p(yi|β, α, φ) α∈R,φ∈R+,β∈S(c) i=1 where p 0 S(c) = β ∈ R : β Σβ ≤ c Hyperparameter c controls the amount of regularisation ⇒ As c → ∞, we recover maximum likelihood Problem Description Generalized Linear Models MML GLM Ridge Regression GLM Ridge Regression Results/Examples Estimating the model (2) Ridge regression requires that c also be estimated We therefore need to estimate β, α, φ and c Further, we would like to determine which of the p features are associated with the target We will use minimum message length to solve these problems Problem Description Minimum Message Length Inference MML GLM Ridge Regression Message Lengths of GLMs Results/Examples Outline 1 Problem Description Generalized Linear Models GLM Ridge Regression 2 MML GLM Ridge Regression Minimum Message Length Inference Message Lengths of GLMs 3 Results/Examples Parameter Estimation Experiments Example: Dog Bite Data Conclusion Problem Description Minimum Message Length Inference MML GLM Ridge Regression Message Lengths of GLMs Results/Examples Minimum Message Length (1) Practical implementation of theory of inductive inference inspired by Kolmogorov complexity Model that yields the briefest encoding of data in a hypothetical message is optimal The message is composed of two-parts assertion, statement describing a particular model θ ∈ Θ ⊂ Rk detail, encoding of the data y using the assertion model θ Bayesian procedure, requires prior distributions Problem Description Minimum Message Length Inference MML GLM Ridge Regression Message Lengths of GLMs Results/Examples Minimum Message Length (2) The total length of the two-part message, I(θ, y), is sum of the lengths of the assertion and the detail I(θ, y) = I(θ) + I(y|θ) MML advocates choosing model θ that minimises the codelength of the hypothetical two-part message In this paper we used the Wallace–Freeman approximation to the exact message length Problem Description Minimum Message Length Inference MML GLM Ridge Regression Message Lengths of GLMs Results/Examples Message Lengths of GLMs (1) Exploit Bayesian interpretation of ridge regression Need to specify prior distributions π(β, α|φ, λ) = π(β|φ, λ)π(α|φ) We choose k 0 λ 2 1 λβ Σβ π(β|φ, λ) = · |Σ| 2 · exp − 2πφ 2φ 1 π(α|φ) ∝ √ φ where λ is a regularisation hyperparameter Conditioning on φ decouples (β, α) and φ Problem Description Minimum Message Length Inference MML GLM Ridge Regression Message Lengths of GLMs Results/Examples Message Lengths of GLMs (2) Applying Wallace–Freeman formula, plus a suitable “correction” arising because of the choice of priors (details in paper) yields a message length I(y, β, α, φ|λ; X) Estimate β, α and φ by minimising the message length n ˆ ˆ o βλ, αˆλ, φλ = arg min {I(y, β, α, φ|λ; X)} β,α,φ Use modified iteratively reweighted least-squares algorithm ⇒ Decoupling between φ and (β, α) simplifies procedure Problem Description Minimum Message Length Inference MML GLM Ridge Regression Message Lengths of GLMs Results/Examples Message Lengths of GLMs (2) Applying Wallace–Freeman formula, plus a suitable “correction” arising because of the choice of priors (details in paper) yields a message length I(y, β, α, φ|λ; X) Estimate β, α and φ by minimising the message length n ˆ ˆ o βλ, αˆλ, φλ = arg min {I(y, β, α, φ|λ; X)} β,α,φ Use modified iteratively reweighted least-squares algorithm ⇒ Decoupling between φ and (β, α) simplifies procedure Problem Description Minimum Message Length Inference MML GLM Ridge Regression Message Lengths of GLMs Results/Examples Message Lengths of GLMs (3) Including λ in message length yields a new message length 1 I(y, β, α, φ, λ; X) = I(y, β, α, φ|λ; X) + log n 2 Regularisation parameter can be estimated by minimising message length ˆ n ˆ ˆ o λ = arg min I(y, βλ, αˆλ, φλ, λ; X) λ∈R+ Problem Description Minimum Message Length Inference MML GLM Ridge Regression Message Lengths of GLMs Results/Examples Message Lengths of GLMs (4) Finally we can select which features are associated with y Let γ ⊂ {1, . , p} denote a list of columns of X Let Xγ denote the submatrix indexed by γ Let π(γ) be a prior for γ Estimate the “best” subset by minimising the message length: n ˆ ˆ ˆ o γˆ = arg min I(y, βλˆ, αˆλˆ, φλˆ, λ; Xγ) − log π(γ) γ ⇒ MML estimates all parameters using a single criterion Problem Description Parameter Estimation Experiments MML GLM Ridge Regression Example: Dog Bite Data Results/Examples Conclusion Outline 1 Problem Description Generalized Linear Models GLM Ridge Regression 2 MML GLM Ridge Regression Minimum Message Length Inference Message Lengths of GLMs 3 Results/Examples Parameter Estimation Experiments Example: Dog Bite Data Conclusion Problem Description Parameter Estimation Experiments MML GLM Ridge Regression Example: Dog Bite Data Results/Examples Conclusion Parameter Estimation (1) Parameter estimation experiment Compared MML ridge estimates against Maximum likelihood Corrected Akaike information criterion (AICc) ridge estimates Experimental parameters Distributions: Normal, Binomial, Poisson Sample sizes, n = {25, 50, 100, 250} Number of features, p = 10 Feature Toeplitz correlations, ρ = {0.1, 0.5, 0.9} True coefficients, βi ∼ N(0, 1) Kullback–Leibler divergence used as loss function Problem Description Parameter Estimation Experiments MML GLM Ridge Regression Example: Dog Bite Data Results/Examples Conclusion Parameter Estimation (1) Parameter estimation experiment Compared MML ridge estimates against Maximum likelihood Corrected Akaike information criterion (AICc) ridge estimates Experimental parameters Distributions: Normal, Binomial, Poisson Sample sizes, n = {25, 50, 100, 250} Number of features, p = 10 Feature Toeplitz correlations, ρ = {0.1, 0.5, 0.9} True coefficients, βi ∼ N(0, 1) Kullback–Leibler divergence used as loss function Problem Description Parameter Estimation
Details
-
File Typepdf
-
Upload Time-
-
Content LanguagesEnglish
-
Upload UserAnonymous/Not logged-in
-
File Pages28 Page
-
File Size-