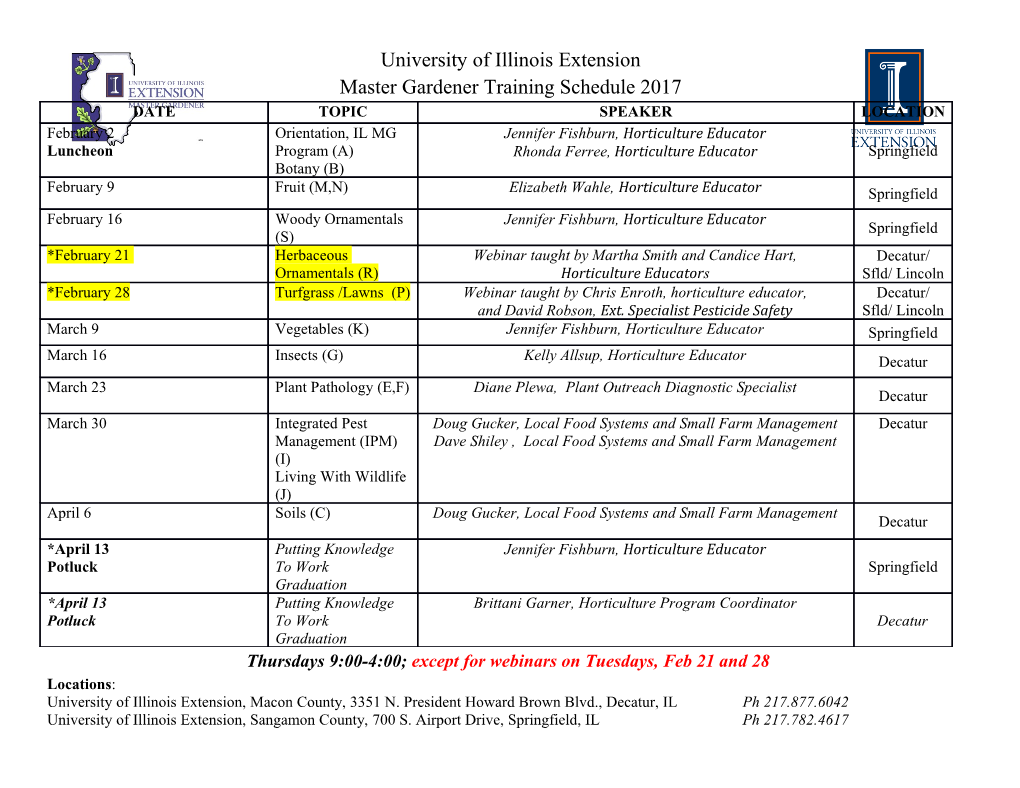
Analyzing Algorithms Goal: “Runs fast on typical real problem instances” Randomized Algorithms How do we evaluate this? Example: Binary search Given a sorted array, determine if the array contains the number 157? 2 3 Complexity " Measuring efficiency T! analysis n! Time ≈ # of instructions executed in a simple Problem size n programming language Best-case complexity: min # steps algorithm only simple operations (+,*,-,=,if,call,…) takes on any input of size n each operation takes one time step Average-case complexity: avg # steps algorithm each memory access takes one time step takes on inputs of size n Worst-case complexity: max # steps algorithm takes on any input of size n 4 5 1 Complexity The complexity of an algorithm associates a number Complexity T(n), the worst-case time the algorithm takes on problems of size n, with each problem size n. T(n)! Mathematically, T: N+ → R+ ! I.e., T is a function that maps positive integers (problem Time sizes) to positive real numbers (number of steps). Problem size ! 6 7 Simple Example Complexity Array of bits. The complexity of an algorithm associates a number I promise you that either they are all 1’s or # 0’s T(n), the worst-case time the algorithm takes on and # 1’s. problems of size n, with each problem size n. Give me a program that will tell me which it is. For randomized algorithms, look at worst-case value of E(T), where the Best case? expectation is taken over randomness in Worst case? algorithm. Neat idea: use randomization to reduce the worst case 8 9 2 Quicksort Analysis of Quicksort Worst case number of comparisons: n Sorting algorithm (assume for now all elements 2 distinct) ✓ ◆ How can we use randomization to improve running Given array of some length n time? If n = 0 or 1, halt Pick random element as a pivot each step Else pick element p of array as “pivot” Split array into subarrays <p, > p => Randomized algorithm Recursively sort elements < p Recursively sort elements > p 10 11 Analysis of Randomized Quicksort Analysis of Randomized Quicksort" Quicksort with random pivots Fix pair i,j. Compute E(Xij) Define A k indicator r.v. that is 1 if elt in [ei, ej] X = # of comparisons. first selected at kth level of tree X = Xij E(Xij)=Pr(Xij = 1) 1 i<j n X = Pr(X =1A )Pr(A ) What is condition for elements ith smallest and jth ij | k k 1 k n smallest to get directly compared? X 2 2 = Pr(Ak) = j i +1 Claim: fate determined first time an elt in [ei, ej] 1 k n j i +1 − X − picked. 2 12 Pr(X =1A )= 13 ij | k j i +1 − 3 Analysis of Randomized Quicksort E(X)= E(Xij) 1 i<j n X 2 = j i +1 1 i<n j>i X X − 1 1 1 2 + + ...+ 2 3 n i +1 1 i<n ✓ ◆ X − 2n ln(n)+O(n) 14 4 .
Details
-
File Typepdf
-
Upload Time-
-
Content LanguagesEnglish
-
Upload UserAnonymous/Not logged-in
-
File Pages4 Page
-
File Size-