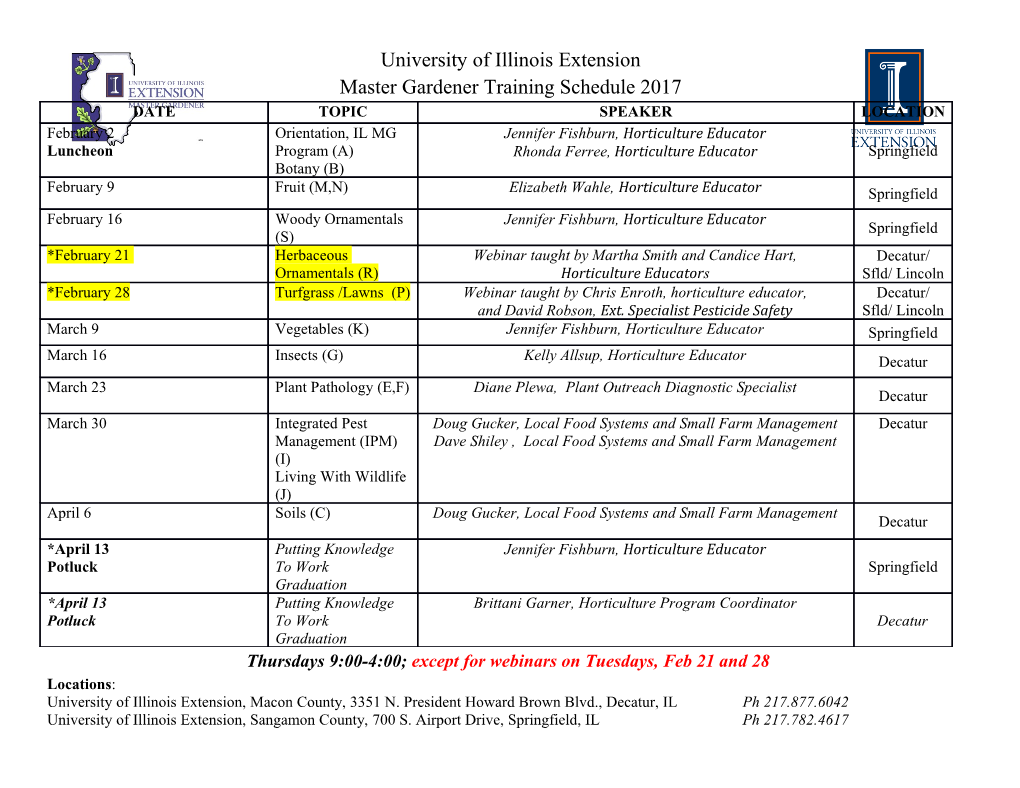
4. Comparison of Two (K) Samples K=2 Problem: compare the survival distributions between two groups. Ex: comparing treatments on patients with a particular disease. 푍: Treatment indicator, i.e. 푍 = 1 for treatment 1 (new treatment); 푍 = 0 for treatment 0 (standard treatment or placebo) Null Hypothesis: H0: no treatment (group) difference H0: 푆0 푡 = 푆1 푡 , for 푡 ≥ 0 H0: 휆0 푡 = 휆1 푡 , for 푡 ≥ 0 Alternative Hypothesis: Ha: the survival time for one treatment is stochastically larger or smaller than the survival time for the other treatment. Ha: 푆1 푡 ≥ 푆0 푡 , for 푡 ≥ 0 with strict inequality for some 푡 (one-sided) Ha: either 푆1 푡 ≥ 푆0 푡 , or 푆0 푡 ≥ 푆1 푡 , for 푡 ≥ 0 with strict inequality for some 푡 Solution: In biomedical applications, it has become common practice to use nonparametric tests; that is, using test statistics whose distribution under the null hypothesis does not depend on specific parametric assumptions on the shape of the probability distribution. With censored survival data, the class of weighted logrank tests are mostly used, with the logrank test being the most commonly used. Notations A sample of triplets 푋푖, Δ푖, 푍푖 , 푖 = 1, 2, … , 푛, where 1 푛푒푤 푡푟푒푎푡푚푒푛푡 푋푖 = min(푇푖, 퐶푖) Δ푖 = 퐼 푇푖 ≤ 퐶푖 푍 = ቊ 푖 0 푠푡푎푛푑푎푟푑 푇푟푒푎푡푚푒푛푡 푇푖 = latent failure time; 퐶푖 = latent censoring time Also, define, 푛 푛1 = number of individuals in group 1 푛푗 = 퐼(푍푗 = 푗) , 푗 = 0, 1 푛0 = number of individuals in group 0 푖=1 푛 = 푛0 + 푛1 푛 푌1(푥) = number of individuals at risk at time 푥 from trt 1 = σ푖=1 퐼(푋푖 ≥ 푥, 푍푖 = 1) 푛 푌0(푥) = number of individuals at risk at time 푥 from trt 0 = σ푖=1 퐼(푋푖 ≥ 푥, 푍푖 = 0) 푌(푥) = 푌0(푥) + 푌1(푥) 푛 푑푁1(푥) = # of deaths observed at time 푥 from trt 1 = σ푖=1 퐼(푋푖 = 푥, Δ푖 = 1, 푍푖 = 1) 푛 푑푁0(푥) = # of deaths observed at time 푥 from trt 0 = σ푖=1 퐼(푋푖 = 푥, Δ푖 = 1, 푍푖 = 0) 푛 푑푁 푥 = 푑푁0 푥 + 푑푁1 푥 = σ푖=1 퐼(푋푖 = 푥, Δ푖 = 1) Note: 푑푁 푥 actually correspond to the observed number of deaths in time window 푥, 푥 + Δ푥 for some partition of the time axis into intervals of length Δ푥. If the partition is sufficiently fine then thinking of the number of deaths occurring exactly at 푥 or in 푥, 푥 + Δ푥 makes little difference, and in the limit makes no difference at all. Weighted logrank Test Statistic 푈(푤) 푇 푤 = 푠푒 푈 푤 Where, 푌 푥 × 푑푁(푥) 푈 푤 = 푤 푥 푑푁 푥 − 1 1 푌(푥) 푥 푠푒 푈 푤 will be given later. The null hypothesis of treatment equality will be rejected if 푇 푤 is sufficiently different from zero. Note: 1. At any time 푥 for which there is no observed death 푌 푥 ×푑푁 푥 푑푁 푥 − 1 = 0. 1 푌 푥 This means that the sum above is only over distinct failure times. 2. A weighted sum over the distinct failure times of observed number of deaths from treatment 1 minus the expected number of deaths from treatment 1 if the null hypothesis were true. 3. When 푤 푥 = 1, logrank test statistic Motivation Take a slice of time 푥, 푥 + Δ푥 : The following 2 × 2 table can be formulated: Under H0: 푑푁1 푥 |푌1 푥 , 푌 푥 , 푑푁 푥 ~퐻푦푝푒푟푔푒표푚푒푡푟푖푐 푌1 푥 , 푑푁 푥 , 푌 푥 푌 푥 푑푁(푥) So, 퐸 푑푁 푥 |푌 푥 , 푌 푥 , 푑푁 푥 = 1 1 1 푌(푥) 푌 푥 ×푑푁(푥) 푑푁 푥 − 1 is the observed number of deaths minus expected number of 1 푌(푥) deaths due to treatment 1. Hence, 푌 푥 ×푑푁(푥) • if H is true, sum of 푑푁 푥 − 1 over 푥 is expected to be near zero. 0 1 푌(푥) • If the hazard rate for treatment 1 were lower than that for treatment 0 consistently 푌 푥 ×푑푁 푥 over 푥, then on average, we expect 푑푁 푥 − 1 to be negative. 1 푌 푥 • If the hazard rate for treatment 1 were higher than that for treatment 0 consistently 푌 푥 ×푑푁 푥 over 푥, then on average, we expect 푑푁 푥 − 1 to be positive. 1 푌 푥 Specifically, the weighted logrank test statistic is given by 푌 푥 × 푑푁(푥) σ 푤 푥 푑푁 푥 − 1 푥 1 푌(푥) 푇 푤 = 푌 푥 푌 푥 푑푁 푥 [푌 푥 − 푑푁(푥)] 1/2 σ 푤2 푥 1 0 푥 푌2 푥 푌 푥 − 1 a Under H0: T(w) ~ N(0, 1) Therefore, a level 훼 test (two-sided) will reject H0: 푆0 푡 = 푆1 푡 , when 푇 푤 ≥ 푧훼/2 Remarks: 푌 푥 ×푑푁(푥) σ 푑푁 푥 − 1 푥 1 푌(푥) 1. Logrank test stat. = 1/2 σ 푌1 푥 푌0 푥 푑푁 푥 [푌 푥 −푑푁(푥)] 푥 푌2 푥 푌 푥 −1 2. The statistic in the numerator is a weighted sum of observed minus the expected over the 푘 2 × 2 tables, where 푘 is the number of distinct failure times. 3. The weight function 푤 푥 can be used to emphasize differences in the hazard rates over time according to their relative values. For example, if the weight early in time is larger and later becomes smaller, then such test statistic would emphasize early differences in the survival curves. 4. If the weights 푤 푥 are stochastic (functions of data), then they need to be a function of the censoring and survival information prior to time 푥. 5. 푤 푥 = 1: Logrank test 6. 푤 푥 = 푌(푥): Gehan′s generalization of wilcoxon test 7. 푤 푥 = 퐾푀(푥): Peto−Prentice′s generalization of wilcoxon test Note: Since both 푌(푥) and 퐾푀(푥) are non-increasing functions of 푥, both Gehan′s and Peto−Prentice′s tests emphasize the difference early in the survival curves. A Heuristic Proof Define a set of random variables: 퐹 푥 = 푑푁0 푢 , 푑푁1 푢 , 푌1 푢 , 푌0 푢 , 푤1 푢 , 푤0 푢 , 푑푁 푥 for all grid points 푢 < 푥 Assume H0 is true. Knowing 퐹 푥 would imply (with respect to the 2 × 2 table) that: We know 푌1 푥 , 푌0 푥 (i.e., the number at risk at time 푥 from either treatment group), and, in addition, we know 푑푁 푥 (i.e., the number of deaths – total from both treatment groups – occurring in 푥, 푥 + Δ푥 ). The only thing we don't know is 푑푁1 푥 . Conditional on 퐹 푥 , we have a 2 × 2 table, which under the null hypothesis follows independence, and we have the knowledge of the marginal counts of the table (i.e., the marginal count are fixed conditional on 퐹 푥 ). Therefore, the conditional distribution of one of the counts, say, 푑푁1 푥 , in the cell of the table, given 퐹 푥 follows a hypergeometric distribution. 푑푁(푥) 푌 푥 −푑푁(푥) 푐 푌 푥 −푐 푃 푑푁 푥 = 푐|푌 푥 , 푌 푥 , 푑푁 푥 = 1 1 1 푌(푥) 푌 푥 푌 푥 푑푁(푥) 1 퐸 푑푁 푥 |퐹 푥 = 1 1 푌(푥) 푌 푥 푌 푥 푑푁 푥 [푌 푥 − 푑푁(푥)] 푉푎푟 푑푁 푥 |퐹 푥 = 1 0 1 푌2 푥 푌 푥 − 1 The numerator of the weighted logrank test statistic is: 푌 푥 × 푑푁(푥) 푈 푤 = 푤 푥 푑푁 푥 − 1 1 푌(푥) 푥 Notice that under H0 : 푌 푥 × 푑푁(푥) 퐸 푈 푤 = 퐸 푤 푥 푑푁 푥 − 1 1 푌(푥) 푥 푌 푥 × 푑푁(푥) = 퐸 퐸 푤 푥 푑푁 푥 − 1 퐹(푥) 1 푌(푥) 푥 푌 푥 × 푑푁(푥) = 퐸 푤 푥 퐸 푑푁 푥 퐹(푥) − 1 = 0 1 푌(푥) 푥 Next, we will find an unbiased estimator for the variance of 푈 푤 . Let 푌 푥 × 푑푁(푥) 퐴 푥 = 푤 푥 푑푁 푥 − 1 . 1 푌(푥) Then, 푉푎푟 푈 푤 = 푉푎푟 퐴(푥) = 푉푎푟 퐴 푥 + 퐶표푣 퐴 푥 , 퐴 푦 . 푥 푥 푥≠푦 Notice that we already show: 퐸 퐴 푥 = 퐸 퐴 푦 = 0. WOLG, suppose y < 푥, then, 퐶표푣 퐴 푥 , 퐴 푦 = 퐸 퐴 푥 ∗ 퐴(푦) = 퐸 퐸 퐴 푥 ∗ 퐴(푦) 퐹(푥) = 퐸 퐴 푦 퐸 퐴(푥) 퐹(푥) = 0 Now, 푉푎푟 푈 푤 = 푉푎푟 퐴 푥 = 퐸 퐴2 푥 = 퐸 퐸 퐴2 푥 퐹(푥) 푥 푥 푥 푌 푥 × 푑푁(푥) 2 = 퐸 퐸 푤2 푥 푑푁 푥 − 1 퐹(푥) 1 푌(푥) 푥 2 2 = 퐸 푤 푥 퐸 푑푁1 푥 − 퐸 푑푁1 푥 퐹(푥) 푥 2 = 퐸 푤 푥 푉푎푟 푑푁1 푥 퐹(푥) 푥 푌 푥 푌 푥 푑푁 푥 [푌 푥 − 푑푁(푥)] = 퐸 푤2 푥 1 0 푌2 푥 푌 푥 − 1 푥 This means: 푌1 푥 푌0 푥 푑푁 푥 [푌 푥 − 푑푁(푥)] 푤2 푥 푉푎푟 푈 푤 푌2 푥 푌 푥 − 1 is an unbiased estimator for . 푥 Recapping: Under H0 : 푆0 푡 = 푆1 푡 1. The Statistics 푈 푤 = σ푥 퐴(푥) has expectation equal to zero, i.e. E 푈 푤 = 0. 2. 푈 푤 = σ푥 퐴(푥) is made up of a sum of conditionally uncorrelated terms each with mean zero. By the central limit theory for such martingale structures, U(w) properly normalized will be approximately a standard normal random variable. That is: 푈(푤) a 푇 푤 = N(0, 1) 푠푒 푈 푤 ~ 3. An unbiased estimate of the variance of 푈 푤 was given by 푌 푥 푌 푥 푑푁 푥 [푌 푥 − 푑푁(푥)] 푤2 푥 1 0 푌2 푥 푌 푥 − 1 푥 Therefore, 푌 푥 × 푑푁(푥) σ 푤 푥 푑푁 푥 − 1 푈(푤) 푥 1 푌(푥) a 푇 푤 = N(0, 1) 푠푒 푈 푤 푌 푥 푌 푥 푑푁 푥 [푌 푥 − 푑푁(푥)] 1/2 ~ σ 푤2 푥 1 0 푥 푌2 푥 푌 푥 − 1 # An Example The data give the survival times for 25 myelomatosis patients randomized to two treatments (1 or 2): dur status trt renal 8 1 1 1 180 1 2 0 … 1296 1 2 0 dur is the patient's survival or censored time, status is the censoring indicator, trt is the treatment indicator, renal is the indicator of impaired renal function (0 = normal; 1 =impaired).
Details
-
File Typepdf
-
Upload Time-
-
Content LanguagesEnglish
-
Upload UserAnonymous/Not logged-in
-
File Pages32 Page
-
File Size-