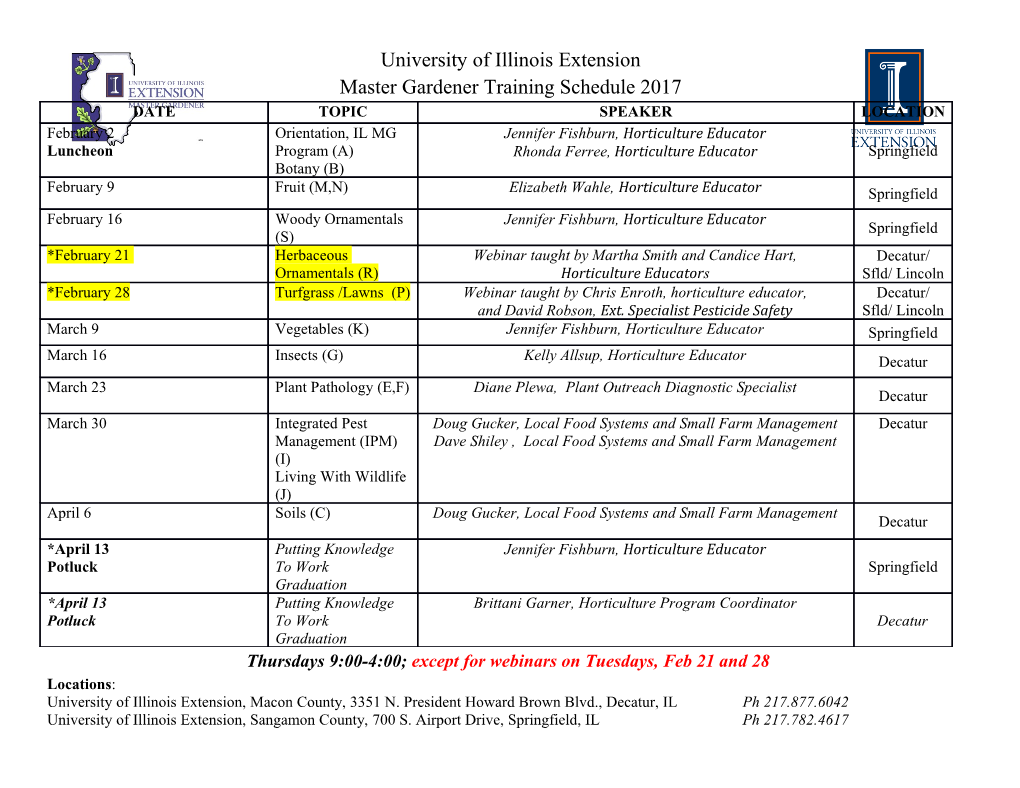
© 2000 Wiley-Liss, Inc. Cytometry 39:300–305 (2000) Mean and Variance of Ratio Estimators Used in Fluorescence Ratio Imaging G.M.P. van Kempen1* and L.J. van Vliet2 1Central Analytical Sciences, Unilever Research Vlaardingen, Vlaardingen, The Netherlands 2Pattern Recognition Group, Faculty of Applied Sciences, Delft University of Technology, Delft, The Netherlands Received 7 April 1999; Revision Received 14 October 1999; Accepted 26 October 1999 Background: The ratio of two measured fluorescence Results: We tested the three estimators on simulated data, signals (called x and y) is used in different applications in real-world fluorescence test images, and comparative ge- fluorescence microscopy. Multiple instances of both sig- nome hybridization (CGH) data. The results on the simu- nals can be combined in different ways to construct dif- lated and real-world test images confirm the presented ferent ratio estimators. theory. The CGH experiments show that our new estima- Methods: The mean and variance of three estimators for tor performs better than the existing estimators. the ratio between two random variables, x and y, are Conclusions: We have derived an unbiased ratio estima- discussed. Given n samples of x and y, we can intuitively tor that outperforms intuitive ratio estimators. Cytometry construct two different estimators: the mean of the ratio 39:300–305, 2000. © 2000 Wiley-Liss, Inc. of each x and y and the ratio between the mean of x and the mean of y. The former is biased and the latter is only asymptotically unbiased. Using the statistical characteris- tics of this estimator, a third, unbiased estimator can be Key terms: ratio imaging; CGH; ratio estimation; mean; constructed. variance In fluorescence microscopy, ratio imaging is applied to article. We will assume x and y to be stochastic variables ϭ ϭ ϭ ϭ a number of applications. In ratio labeling, the ratio be- with E{ x} x X and E{ y} y Y. In the second tween the intensities of different fluorophores is used to section we study the statistical properties of two estima- expand the number of labels for an in situ hybridization tors of the ratio R. Based on these results we show in the procedure (1). The number of fluorophores that can be third section that, under certain conditions (known vari- spectrally separated by fluorescence microscopy normally ance, covariance), a third, unbiased estimator can be con- restricts the total number of labels. structed. The simulations and experiments in the fourth Fluorescence ratio imaging is also used to measure spa- section support the presented theory. In the fifth section, tial and temporal differences in ion concentrations within we discuss the application of the presented theory on a single cell. This is achieved by using fluorophores whose CGH ratio imaging. We conclude in the sixth section. excitation or emission spectrum changes as a function of the Ca2ϩ or pH concentration (2). MEAN AND VARIANCE OF TWO In a third application of ratio imaging, known as com- RATIO ESTIMATORS parative genome hybridization (CGH) (3,4), one tries to Given n samples of x and y, we can obtain two obvious estimate the DNA sequence copy number as a function of estimators for the ratio R ϭ X/Y: the chromosomal location. This is achieved by measuring the hybridization between “tumor” DNA and “normal” x x r ϭ ͩ ͪ r ϭ (2) DNA to detect gene amplifications and deletions (5). 1 y 2 y In fluorescence ratio imaging, one is interested in the ratio R between two random variables X and Y, This research was presented at ASCI’95, First Annual Conference of the Advanced School for Computing and Imaging, Heijen, The Netherlands, 16–18 May 1995. X R ϭ . (1) The research was performed while G.M.P. van Kempen was a member Y of the Pattern Recognition Group. *Correspondence to: Dr. Ir. Geert M.P. van Kempen, Unilever Research Vlaardingen, Olivier van Noortlaan 120, 3133 AT Vlaardingen, P.O. Box In practice, one cannot measure X and Y, but only “noisy” 114, 3130 AC Vlaardingen, The Netherlands. realizations of X and Y, termed x and y in the present E-mail: [email protected]. FLUORESCENCE RATIO IMAGING 301 with x the average of x. To find an approximate expres- x x x 2 var͑r ͒ ϭ varͩ ͪ ϭ Eͭͩ Ϫ Eͭ ͮͪ ͮ sion for the expectation of r1 and r2, we have used a 2 y y y Taylor series expansion of x/y around x, y: x 2 Ϸ Ϫ x Eͭͩ ͪ ͮ x y y ץ x ץ x x Ϸ ͯ ϩ ͑x Ϫ ͒ ͩ ͪͯ ϩ ͑y Ϫ ͒ ͩ ͪͯ ͑ ͒ 2 ͑ ͒ ͑ ͒ var x x var y 2 x cov x, y ץ y ץ x y y , x y , y y , Ϸ ϩ Ϫ x y x y x y 2 4 3 y y y ͑ ͒ 2 ͑ ͒ ͑ ͒ x 1 var x x var y 2 x cov x, y 2ץ 1 2 Ϸ ͩ ϩ Ϫ ͪ ϩ ͑ x Ϫ ͒ ͩ ͪͯ 2 4 3 . (7) x2 y n y y y ץ x 2 x,y Estimators r1 and r2 have, only in first-order Taylor series x expansion, an equal variance, which diminishes for an 2ץ 1 ϩ ͑ y Ϫ ͒2 ͩ ͪͯ 3 ϱ y2 y infinite number of samples (n ). Therefore both ץ y 2 x,y estimators r1 and r2 are consistent. An expression similar to equations (6) and (7) for the variance of x/y was found .(x by Kendall and Stuart (6 2ץ ϩ ͑ Ϫ ͒͑ Ϫ ͒ ͩ ͪͯ ץ ץ x x y y x y y x, y AN UNBIASED RATIO ESTIMATOR In the previous section, we found that the estimator r 3 2 x is only asymptotically unbiased. However, having found ץ ץ ϩ Oͩͩ͑x Ϫ ͒ ϩ ͑y Ϫ ͒ ͪ ͩ ͪͪ. ͑3͒ y y an analytical expression of the bias, we can derive anץ x yץ x unbiased estimator for R based on r2: A Taylor series expansion of x/y around x, y is similar ͑ ͒ to equation (3). The mean of r1 and r2 can be found by x 1 x cov x, y r ϭ Ϫ ͩ var͑ y͒ Ϫ ͪ . (8) applying the expectation operator to the individual terms 3 y n 3 2 (ignoring all terms higher than two), y y The expectation for this estimator is, of course, ͑ ͒ x x x x cov x, y E͕r ͖ ϭ Eͭͩ ͪͮ ϭ Eͭ ͮ Ϸ ϩ var͑ y͒ Ϫ ͑ ͒ 1 3 2 x 1 x cov x, y x y y y y y E͕r ͖ ϭ Eͭ Ϫ ͩ var͑ y͒ Ϫ ͪͮ Ϸ . (9) 3 y n 3 2 (4) y y y and The variance of this estimator yields ͑ ͒ x x 2 x x x cov x, y x ͑ ͒ ϭ ͭͩ Ϫ ͭ ͮͪ ͮ ϭ ͑ ͒ ͕ ͖ ϭ ͭ ͮ ϭ ϩ ͑ ͒ Ϫ Ϸ var r3 E E var r2 . (10) E r2 E var y 3 2 y y y y y y y 1 cov͑ x, y͒ ϩ ͩ ͑ ͒ x Ϫ ͪ EXPERIMENTS var y 3 2 (5) n y y Some simulations were performed to support the ex- pressions for the mean and variance of r1, r2, and r3.In with E{r} the expectation of r, var( y) the variance of y, the first experiment we used computer-generated noise; and cov( x, y) the covariance of x and y. It is clear from in the second experiment we used noise images generated these two expressions that r2 is asymptotically unbiased in fluorescence image acquisition. 3 ϱ ϭ (lim n E{r2} x/ y) and that r1 is a biased Simulations estimator of R. An approximation of the variance of r1 and r2 is obtained by using the first-order terms of the Taylor An image of size n ϫ m was filled with m realizations series expansion: of x, N( x, x), each containing n samples (numerical recipes) (7) and another image was filled with realizations x x x 2 of y, N( y, y). Figure 1 shows the mean and variance of ͑ ͒ ϭ ͩͩ ͪͪ ϭ ͭͩͩ ͪ Ϫ ͭͩ ͪͮͪ ͮ var r1 var E E r1, r2, and r3 as a function of the number of samples n. y y y Note that r2 asymptotically converges to x/ y, r1 re- x 2 mains biased even for large values of n, whereas r is Ϸ ͩͩ ͪ Ϫ xͪ 3 E unbiased even for small number of samples. The means of y y r and r are in agreement with the predictions, whereas ͑ ͒ 2 ͑ ͒ ͑ ͒ 2 3 1 var x x var y 2 x cov x, y Ϸ ͩ ϩ Ϫ ͪ the mean of r1 converges to a slightly higher ratio than 2 4 3 (6) n y y y predicted when using a second-order Taylor approxima- tion, as in equation (5). The estimated variances of r1 and and r3 are in agreement with the theoretical variances as 302 VAN KEMPEN AND VAN VLIET ϭ ϭ ϭ ϭ FIG. 1. The mean and variance of r1, r2, and r3 measured as a function of n, with x y 40.0 and x y 8.0. Each point is an average of 10,000 realizations. ϭ ϭ FIG.
Details
-
File Typepdf
-
Upload Time-
-
Content LanguagesEnglish
-
Upload UserAnonymous/Not logged-in
-
File Pages6 Page
-
File Size-