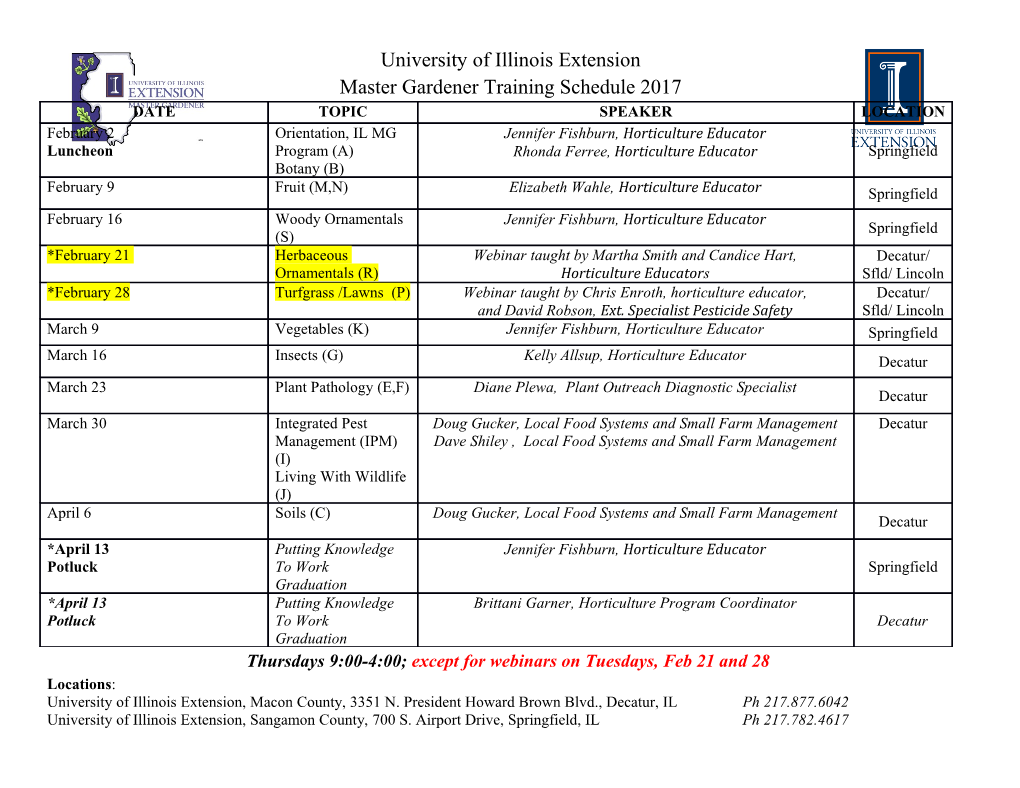
Temporal models of streaming social media data Daniel Preot¸iuc-Pietro Department of Computer Science The University of Sheffield A thesis submitted for the degree of Doctor of Philosophy June 2014 Abstract There are significant temporal dependencies between online behaviour and occurring real world activities. Particularly in text modelling, these are usually ignored or at best dealt with in overly simplistic ways such as assuming smooth variation with time. Social media is a new data source which present collective behaviour much more richly than tradi- tional sources, such as newswire, with a finer time granularity, timely reflection of activities, multiple modalities and large volume. Analysing temporal patterns in this data is important in order to discover newly emerging topics, periodic occurrences and correlation or causality to real world indicators or human behaviour patterns. With these opportunities come many challenges, both engineering (i.e. data volume and process- ing) and algorithmic, namely the inconsistency and short length of the messages and the presence of large amounts of irrelevant messages to our goal. Equipped with a better understanding of the dynamics of the com- plex temporal dependencies, tasks such as classification can be augmented to provide temporally aware responses. In this thesis we model the temporal dynamics of social media data. We first show that temporality is an important characteristic of this type of data. Further comparisons and correlation to real world indicators show that this data gives a timely reflection of real world events. Our goal is to use these variations to discover emerging or recurring user behaviours. We consider both the use of words and user behaviour in social media. With these goals in mind, we adapt existing and build novel machine learning techniques. These span a wide range of models: from Markov models to regularised regression models and from evolutionary spectral clustering which models smooth temporal variation to Gaussian Process regression which can identify more complex temporal patterns. We introduce approaches which discover and predict words, topics or be- haviours that change over time or occur with some regularity. These are modeled for the first time in the NLP literature by using Gaussian Processes. We demonstrate that we can effectively pick out patterns, in- cluding periodicities, and achieve state-of-the-art forecasting results. We show that this performance gain transfers to improve tasks which do not take temporal information in account. Further analysed is how temporal variation in the text can be used to discover and track new content. We develop a model that exploits the variation in word co-occurrences for clustering over time. Different collection and processing tools, as well as several datasets of social media data have been developed and published as open-source software. The thesis posits that temporal analysis of data, from social media in par- ticular, provides us with insights into real-world dynamics. Incorporating this temporal information into other applications can benefit standard tasks in natural language processing and beyond. Acknowledgements I would first like to thank my supervisor, Trevor Cohn, for guiding me through this hard but rewarding process. His expertise, vision and drive had a major impact in my career as a researcher. He has provided me patience, understanding and great feedback when I needed it most. The Department of Computer Science at the University of Sheffield and the Trendminer project offered me a great environment for research. I had the privilege to collaborate with great colleagues such as Vasileios Lampos, Sina Samangooei, Nikolaos Aletras, Andrea Varga, Kalina Bontcheva, and Dominic Rout. I would also like to thank my thesis examiners, Lucia Specia and Nello Cristianini, for their expertise and attention to detail. Without their help, my thesis would be poorer. A special thanks is also for Noah Smith and Chris Dyer which have kindly invited me to visit Carnegie Mellon University during my studies. My experience there was invaluable and has heavily and positively impacted my research. However, I would have never chosen this path without the time I spent at the University of Bucharest. Responsible for my career path was mostly Florentina Hristea who introduced me to AI and NLP in particular. The enthusiasm of my professors there, especially Marius Popescu, Liviu Dinu and Horia Georgescu, provided an inspiration for me. On a different, personal scale, none of this was possible without the help of my parents, Valeriu Preot¸iuc-Pietro and Ana-Romanit¸a Preot¸iuc-Pietro, and my girlfriend Ioana Pal. They have given me the support I needed while I was away for my studies. Contents 1 Introduction 1 1.1 Aims . .3 1.2 Thesis structure . .4 1.3 Published material . .5 2 Online Social Networks 7 2.1 Microblogging . .7 2.1.1 Conventions . .9 2.1.2 Quantitative analysis . 11 2.1.3 Data . 12 2.2 Location Based Social Networks . 15 2.2.1 Quantitative analysis . 16 2.2.2 Data . 18 2.2.3 Data analysis . 19 2.3 Conclusion . 23 3 Architecture for experimental setup 25 3.1 Components . 25 3.1.1 Tokenisation . 26 3.1.2 Language detector . 27 3.1.3 Stemmer . 28 3.1.4 Local time . 28 3.1.5 Deduplicator . 28 3.1.6 Sentiment detector . 29 3.1.7 Geolocator . 30 3.1.8 Possible extensions . 33 3.2 Open-source tool . 35 3.2.1 Aims . 35 i 3.2.2 Architecture . 36 3.2.3 Data format . 37 3.2.4 Running times . 38 3.3 Conclusions . 38 4 Temporality and social media 40 4.1 Non-temporal models . 41 4.1.1 Timestamped data analysis . 41 4.1.2 Forecasting . 44 4.2 Temporal models . 47 4.2.1 Online learning . 47 4.2.2 Online clustering and event detection . 48 4.2.3 Regularised learning . 50 4.2.4 Generative models . 51 4.3 Forecasting political voting intention . 52 4.3.1 Voting intention scores . 52 4.3.2 Linear regression . 54 4.3.3 Bilinear regression . 55 4.3.4 Experimental setup . 60 4.3.5 Quantitative results . 62 4.3.6 Qualitative analysis . 62 4.4 Non-stationary models of forecasting . 66 4.4.1 Online bilinear learning . 66 4.4.2 Non-stationarity . 71 4.5 Conclusion . 73 5 Temporal prediction 75 5.1 Gaussian Processes . 75 5.1.1 Gaussian Process regression . 76 5.1.2 Kernels . 78 5.1.3 Model selection and optimisation . 82 5.2 Temporal modelling of words . 84 5.2.1 Task . 85 5.2.2 Related work . 86 5.2.3 Experimental setup . 86 5.2.4 Methods . 87 5.2.5 Results . 88 ii 5.2.6 Discussion . 92 5.2.7 Text based prediction . 92 5.3 Temporal modelling of user behaviour . 94 5.3.1 Venue categories . 95 5.3.2 Experimental setup . 99 5.3.3 Methods . 100 5.3.4 Results . 101 5.4 Conclusion . 102 6 Detection of timely events 104 6.1 Word co-occurrence . 105 6.1.1 Pointwise mutual information . 106 6.1.2 Temporal evolution . 108 6.1.3 Applications . 109 6.2 Methods . 110 6.2.1 K-means clustering . 111 6.2.2 Spectral clustering . 112 6.2.3 Evolutionary spectral clustering . 114 6.3 Experiments . 115 6.3.1 Data . 116 6.3.2 Cluster measures . 117 6.3.3 Gardenhose experiments . 117 6.3.4 Austrian Politics experiments . 126 6.3.5 Application to summarisation . 130 6.4 Temporal experiments . 131 6.5 Conclusion . 132 7 Conclusions 134 7.1 Further research . 136 7.2 Summary . 138 A Event related clusters 139 B Aggregated clusters 141 Bibliography 144 iii Chapter 1 Introduction In an Internet where user generated content is ubiquitous, systems that are aware of the temporal evolution of data are paramount for a better understanding of real world phenomena. Thus, in this thesis, we aim to develop machine learning models for streaming user generated social media data which incorporate the temporal com- ponent in order to discover topic emergence, recurrence or correlation to underlying events. The Internet has become immense1 and plays a large part in the life of most people. This is mainly due to its widespread and proliferation of devices that can access it. Many people have shifted much of their daily personal activities to the online medium like socialising, work or information gathering. This is particularly obvious by the recent burst in usage of social media and user-generated content. Reportedly, social media usage became the single most time-consuming activity on the web as measured within the U.S., overtaking search, e-mail or gaming.2 With social media, we have seen a shift in paradigms for posting online content. Before social media, and the so-called Web 2.0 in general, the content was static; there were a few hubs of information which were updated only by those who had the authority, curating the content in the process. Now, also with the aid of the technical advances, user-generated content has become prevalent online. Thus, every person using social media can be thought as being a `journalist' [Murthy, 2011] on his daily life, exhibited by him sharing news, events, moods or images. For many, these sources have become the primary source of information for time sensitive or local events. This is mostly because people trust their peers more for giving accurate more insights, comments and a complementary view compared to traditional editorial sources. The lack of curation tends to lead to swifter responses. 1http://archive.org/ 2http://cn.nielsen.com/documents/Nielsen-Social-Media-Report_FINAL_090911.pdf 1 A notorious example illustrating the timely nature of social media (i.e.
Details
-
File Typepdf
-
Upload Time-
-
Content LanguagesEnglish
-
Upload UserAnonymous/Not logged-in
-
File Pages174 Page
-
File Size-