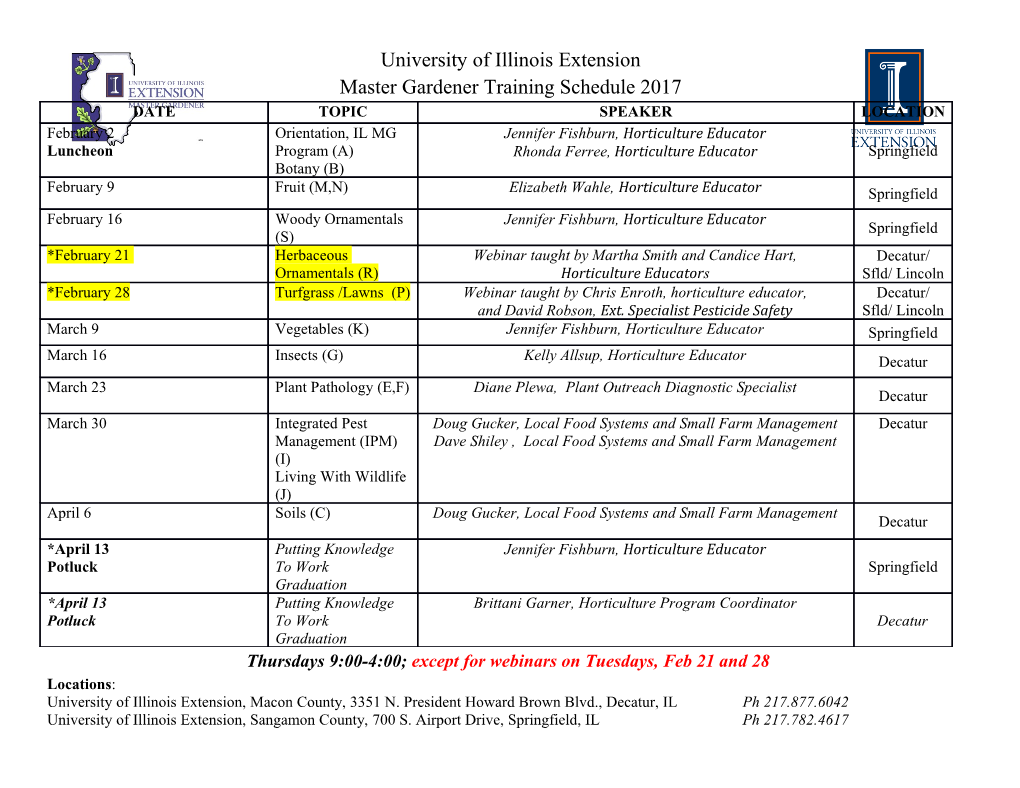
Xu et al. EURASIP Journal on Image and Video EURASIP Journal on Image Processing (2018) 2018:136 https://doi.org/10.1186/s13640-018-0376-5 and Video Processing RESEARCH Open Access Online multi-frame super-resolution of image sequences Jieping Xu1* , Yonghui Liang2,JinLiu2, Zongfu Huang2 and Xuewen Liu2 Abstract Multi-frame super-resolution recovers a high-resolution (HR) image from a sequence of low-resolution (LR) images. In this paper, we propose an algorithm that performs multi-frame super-resolution in an online fashion. This algorithm processes only one low-resolution image at a time instead of co-processing all LR images which is adopted by state- of-the-art super-resolution techniques. Our algorithm is very fast and memory efficient, and simple to implement. In addition, we employ a noise-adaptive parameter in the classical steepest gradient optimization method to avoid noise amplification and overfitting LR images. Experiments with simulated and real-image sequences yield promising results. Keywords: Super-resolution, Image processing, Image sequences, Online image processing 1 Introduction There are a variety of SR techniques, including multi- Images with higher resolution are required in most elec- frame SR and single-frame SR. Readers can refer to Refs. tronic imaging applications such as remote sensing, med- [1, 5, 6] for an overview of this issue. Our discussion below ical diagnostics, and video surveillance. For the past is limited to work related to fast multi-frame SR method, decades, considerable advancement has been realized in as it is the focus of our paper. imaging system. However, the quality of images is still lim- One SR category close to our alogorithm is dynamic SR ited by the cost and manufacturing technology [1]. Super- which means estimating a sequence of HR images from a resolution (SR) is a promising digital image processing sequence of LR images. A natural approach is to make an technique to obtain a single high-resolution image (or extension of the static SR methods, as is adopted by some sequence) from multiple blurred low-resolution images. video SR algorithms [7, 8]. Although these batch-based The basic idea of SR is that the low-resolution (LR) dynamic SR algorithms have the ability to enhance the images of the same scene contain different information resolution of images/videos, the high memory and com- because of relative subpixel shifts; thus, a high-resolution putational requirements restrict their practical use. Farsiu (HR) image with higher spatial information can be recon- et al. [9] proposed a fast and memory-efficient algorithm structed by image fusion. Subpixel motion can occur due for dynamic demosaicing and color SR of video sequences. to movement of local objects or vibrating of imaging sys- They first got a blurry HR image by shifting and adding tem, or even controlled micro-scanning [2, 3]. Numerous each LR frame recursively based on the Kalman filter, SR algorithms have been proposed since the concept was then the blurry HR image was deconvolved based on max- introduced by Tsai and Huang [4] in the year of 1984. imum a posteriori (MAP) technique. To make Farsiu’s Most of them operate in batch mode, i.e., a sequence of algorithm more robust, Kim et al. [10]proposedamea- images are co-processed at the same time. Thus, these surement validation method based on Mahalanobis dis- algorithms require a high memory resource to store the tance to eliminate the LR images whose motion estimation LR images and temporary data, and need a high com- errors were larger than a threshold. Recently, researchers puting resource as well. These disadvantages limit their pay more attention to SR methods based on neural net- practical application. work (NC)[11, 12], which can be trained from data to approximate complex nonlinear functions, while these *Correspondence: [email protected] algorithms require significant computational resources to 1Space Engineering University, No. 1, Bayi Road, Beijing, 101416 China achieve real-time throughput. Graphic processing unit Full list of author information is available at the end of the article (GPU)isusedtoaccelerate,asdonebyHuetal.[13–16]. © The Author(s). 2018 Open Access This article is distributed under the terms of the Creative Commons Attribution 4.0 International License (http://creativecommons.org/licenses/by/4.0/), which permits unrestricted use, distribution, and reproduction in any medium, provided you give appropriate credit to the original author(s) and the source, provide a link to the Creative Commons license, and indicate if changes were made. Xu et al. EURASIP Journal on Image and Video Processing (2018) 2018:136 Page 2 of 10 2 2 Stefan Harmeling et al. [17] are the first to propose a size L MN × L MN, Bt represents blur matrix of size 2 2 blind deconvolution algorithm for astronomical imaging L MN × L MN, Dt denotes the down-sampling matrix of 2 working in online mode. Instead of minimizing the total size MN ×L MN, nt is the lexicographically ordered noise overall cost function for all blurry images, they just mini- vector, and yt represents the lexicographically ordered LR mized the cost function for one image at each time and the imagevectorofsizeMN × 1. current deblurred image was used as the initial estimate Mathematically, the SR problem is an inverse problem for the next time recursively. Thus, the deblurred image whose objective is to estimate an HR image from multiple was refined gradually along with the image capturing. observed blurry LR images. MAP estimator is a powerful Hirsch et al. [18] extended the online blind deconvolution tool to solve this type of problem. The MAP optimization algorithm by incorporating super-resolution. The differ- problem can be represented by ent information contained in different LR images results ˆ = ( | ) + ( ) from blurring with different blur kernels. Both online xMAP arg max ln P yt x ln P x (2) blind deconvolution (OBD) and its SR extension (OBDSR) where yt means all LR images, and P(x) represents a pri- get the HR image estimate by minimizing an auxiliary ori knowledge of the HR image. However, some complex function rather than minimizing the cost function directly priori models used by the MAP estimator may increase to avoid image overfitting, while sometimes the strategy the computational burden remarkably. If we omit the failed, especially when the noise of LR images is large. priori term, the MAP estimator degenerates to a maxi- Stimulated by the online blind deconvolution algorithm mum likelihood (ML) estimator. Under the assumption proposed by Stefan Harmeling et al. [17], we propose of independent and identical Gaussian distributed image a multi-frame super-resolution algorithm operating in noise with zero mean, ML optimization problem can be online mode which we name as online SR (OSR). Differ- simplified as a least square problem, i.e., ent from the OBDSR, the different information provided by different LR images in our algorithm results from rel- T ˆ = − 2 ative motion between each other instead of different blur xML arg min yt DtBtMtx (3) kernels. Another difference is that we employ a modified t=1 steepest gradient optimization method to estimate the HR Generally, due to the ill-posed nature of SR inverse prob- image instead of an auxiliary function, which is proved to lem, the MAP estimator is superior to the ML estima- be more robust to noise. In this paper, the LR images are tor. For both MAP estimator and ML estimator, all LR assumed to be obtained in a streaming fashion with a com- images should be stored in memory; thus, a high memory mon space-invariant blur, and the relative motion between resource is required. each other can be modeled as translation. At each time, we get an LR image, and we immediately use it to improve 3 The proposed method the current HR image estimate. 3.1 The loss function The rest of this paper is organized as follows. Section 2 In our OSR algorithm, when we get a new LR image in addresses the formulation of the SR problem. In Section 3, the data stream, we retrieve the new information immedi- we lay out the details of our algorithm and make it com- ately and add it to the HR image estimate. The process is patible with color images. In Section 4, we present exper- realized by minimizing the loss function incurred at time imental results with simulated and real-image sequences. t instead of overall loss. The problem can be expressed as The paper will conclude in Section 5 with a brief summary a non-negatively constrained problem: and future work plan. xˆ = min J (x; y ) = min y − D B M x2 (4) t ≥ t t ≥ t t t t 2 Problem statement x 0 x 0 To study the super-resolution problem, we first formu- The motion matrix Mt could vary in time, while the down- late the observation model. For notational simplicity, the sampling matrix Dt and blur matrix Bt remain constant image in our method is represented as a lexicographically over time for most situations (i.e., B = Bt, D = Dt). We ordered vector. Let us consider the desired HR image of further assume the relative motion between LR images size LM × LN,whereL denotes the down-sampling factor can be modeled as pure translation. Above assumptions in the observation model and M and N are the number of are valid in staring imaging and some videos during a cer- rows and columns of the LR images. The most commonly tain period of time. The matrices D, B,andM are quite used observation model is given by huge with few non-zero elements, thus can be constructed as sparse matrices.
Details
-
File Typepdf
-
Upload Time-
-
Content LanguagesEnglish
-
Upload UserAnonymous/Not logged-in
-
File Pages10 Page
-
File Size-