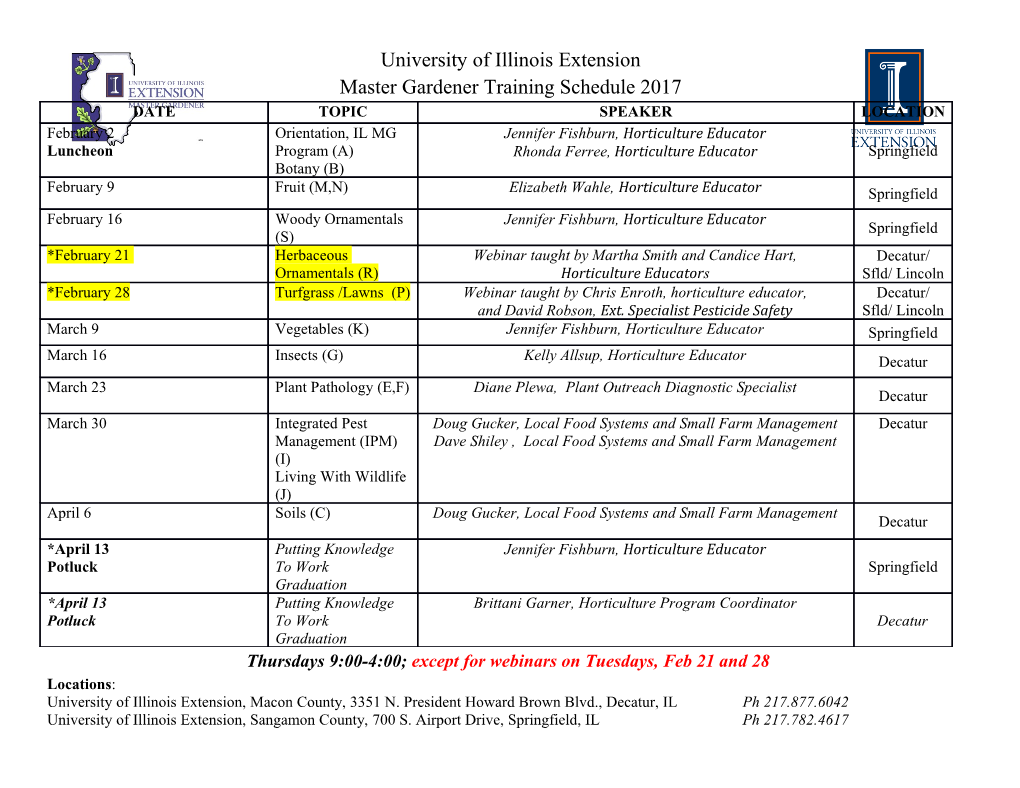
Principle of Statistics Michael Li March 28, 2017 e Likelihood Principle Basic inferential principles. Likelihood and score functions, Fisher information, Cramer-Rao lower bound, review of multivariate normal distribution. Maximum likelihood estimators and their asymptotic properties: stochastic convergence concepts, consistency, eciency, asymp- totic normality. Wald, score and likelihood ratio tests, condence sets, Wilks theorem, prole likelihood. Examples. [8] Bayesian Inference Prior and posterior distributions. Conjugate families, improper priors, predictive distributions. Asymptotic theory for posterior distributions. Point estimation, credible regions, hypothesis testing and Bayes factors. [3] Decision eory & Multivariate Analysis Basic elements of a decision problem, including loss and risk functions. Decision rules, admissi- bility, minimax and Bayes rules. Finite decision problems, risk set. Stein estimator.Correlation coecient and distribution of its sample version in a bivariate normal population. Partial corre- lation coecients. Classication problems, linear discriminant analysis. Principal component analysis. [9] Nonparametric Inference and Monte Carlo Techniques Glivenko-Cantelli theorem, KolmogorovSmirnov tests and condence bands. Bootstrap meth- ods: jackknife, roots (pivots), parametric and nonparametric bootstrap. Monte Carlo simulation and the Gibbs sampler. [4] 1 Contents Contents 2 1 Maximum Likelihood Principle 3 1.1 Information Geometry and e Likelihood Function . .3 1.2 Denitions and Elementary eorems . .4 1.3 Cramer-Rao Lower Bound . .4 1.3.1 Multivariate Cramer-Rao lower bound . .5 2 Asymptotic eory (for MLE) 5 2.1 Law of Large Numbers and Central Limit eorem . .6 2.2 Consistency of MLE . .7 2.3 Plug-in MLE and Delta Method . .8 3 Asymptotic Inference with MLE 9 3.1 Hypothesis Testing . .9 4 Bayesian Inference 9 4.1 Basic Ideas, Prior, and Posterior Distribution . .9 5 Decision eory 10 5.1 Admissability . 11 6 Classication Problems 13 7 Further Topics 14 7.1 Multivariate Analysis for Statistics . 14 7.2 Resampling Techniques and the Bootstrap . 14 7.2.1 Bootstrap . 14 7.3 Monte-Carlo Methods . 15 7.4 Nonparametric Models . 15 2 1 Maximum Likelihood Principle Denition. Let ff(·; θ): θ 2 Θg be a statistical model of pdf for the law P of X, and consider observing X1; ··· ;Xn independent observations of X. e likelihood function of the model is: n Y ln(θ) = f(Xi; θ) i=1 e log-likelihood function is: X ln(θ) = log Ln(θ) = log L(xi; θ) ¯ 1 e normalized log-likelihood function is ln(θ) = n ln(θ). Denition. A maximum likelihood estimator (MLE) is any value θ^ 2 Θ for which ^ Ln(θ) = maxθ2Θ Ln(θ). To solve for MLE,we take the zero of the score function, which is dened as: Sn(θ) = rθ ln(θ) 1.1 Information Geometry and e Likelihood Function d For X a random variable of law Pθ on X ⊆ R and g : X ! R. We write: Z Eθg(X) = EPθ g(x) = g(x)dPθ(x) Z = g(x)f(x; θ)dx X = g(x)f(x; θ) where the last equality only holds if X is discrete. ¯ Maximizing Ln(θ) is equivalent to maximizing ln(θ), which is an approximation of Z l(θ) = Eθ0 [log(f(X; θ))] = log(f(X; θ))f(X; θ0)dx We have f(X; θ) l(θ) − l(θ0) = Eθ0 log( ) f(X; θ0) Recall Jensen’s inequality. Since log is concave, f(X; θ) Z f(X; θ) l(θ) − l(θ0) ≤ log Eθ0 = log f(X; θ0)dX = log(1) = 0 f(X; θ0) f(X; θ0) If we make the assumption of strict identiability, as in l(θ) = l(θ0) ) θ = θ0, then by the strict version of Jenson’s inequality, we have l(θ) < l(θ0), so θ0 is the unique maximizer. Remark. l(θ0)−l(θ) can be interpreted as a distance between θ and θ0. is is called the Kulbach-Leibler distance or divergence, or the entropy distance between f(X; θ) and f(X; θ0). 3 1.2 Denitions and Elementary eorems @ R Denition. In a parametric model, if @θ and integration ·dx can be interchanged, we say that the model is regular. In a regular model,we have: @ @ Z E [ log f(X; θ)] = f(X; θ)dx = 0 θ @θ @θ Now we dene an important concept in this course: R p Denition. For θ 2 (θ), we set for θ ⊂ R @ @ I(θ) = E [[ log f(x; θ)][ log f(x; θ)]T ] θ @θ @θ We call I(θ), the p × p matrix, the Fisher Information Matrix. Proposition. For all θ 2 R (θ), in a regular model, we have: @2 I(θ) = −E [ log f(x; θ)] θ @θ@θT Proof. @2 Z 1 @2 1 @ @ −E [ log f(x; θ)] = − f(x; θ) − f(x; θ) f(x; θ) f(x; θ)dθ θ @θ@θT f(x; θ) @θ@θT f(x; θ)2 @θ @θT Z @2 1 @ @ = − f(x; θ)dθ + E [ f(x; θ) f(x; θ)] @θ@θT θ f(x; θ)2 @θ @θT = I(θ) For the last step, the rst integral is just 0 aer taking about the derivative, and the second integral is just I(θ). 1.3 Cramer-Rao Lower Bound eorem. Let ff(x; θ); θ 2 Θg be a regular statistical model, and θ^ an unbiased estimator 2 R. en: 1 Var(θ^) ≥ 8θ 2 Θ nI(θ) Proof. Remember the Cauchy-Schwarz inequality: Cov2(Y; Z) ≤ Var(Y ) Var(Z) ^ @ Let Y = θ and Z = @θ log f(X; θ). en Cov(Y; Z) = E[YZ] (E[Z] = 0) and Var(Z) = I(θ). en: Z @ @ Z E[YZ] = θ^(x) f(x; θ)dx = θ^(x)f(x; θ)dx = 1 @θ @θ As the integral is just the expectation of θ^. en the CS inequality rearranges to the required form. Now you ask, where is the n? is comes from the n samples, and we have to use @ Q Z = @θ log i f(Xi; θ), and Var(Z) = nI(θ) here. Of course we also have a easy corollary: ( @ E θ^)2 ^ ^ @θ θ Corollary. If θ is not unbiased, we have Var(θ) ≥ nI(θ) . 4 1.3.1 Multivariate Cramer-Rao lower bound eorem. For θ 2 Θ ⊆ Rp, p ≥ 1, consider functionals of the parameter Φ: θ ! R. One shows in a similar manner that for any unbiased estimator Φ~ based on n iid observations X1; ··· ;Xn has a lower bound: 1 @Φ @Φ Var (Φ)~ ≥ (θ)T I−1(θ) (θ) θ n @θ @θ For example consider: X @ Φ(θ) = αT θ = α θ Φ(θ) = α i i @θ ~ 1 T −1 en Varθ(Φ) ≥ n α I (θ)α. Example. Let x1 = X ∼ N(θ; Σ) where θ = θ1 and where θ is known. For a x2 θ2 sample size fo 1: Case 1 Consider estimation of θ1, when θ2 is known. en, the model is one-dimensional with parameter θ1 and the Fisher information is I1(θ1). Case 2 When θ2 is unknown, we have a two dimensional model and we care about θ1 = Φ(θ). Applyingt he Cramer-Rao lower bound: @ΦT @Φ Var (θ~ ) = (θ)I−1(θ) (θ) = I (θ ) θ 1 @θ @θ Φ 1 2 Asymptotic eory (for MLE) ~ At the very least, we need E(θn) ! θ as n ! 1. We would like the unbiased estimator θ~ to converge to θ when n ! 1 as we take X1; ··· Xn samples from Pθ. is is called consistency. ~ −1 e best we can hope for is that n Varθ(θn) ! I (θ) as n ! 1. is is called asymptotic eciency. Denition. Let (Xn; n ≥ 0), X be random vectors in a probability space (Ω; A; P). a:s: - Xn converges to X almost surely, denoted Xn −−! X if: P(w 2 Ω: kXn(w) − X(w)k ! 0asn ! 1) = 1 P - Xn converges to X in probability, denoted Xn −! X if: P(kXn − Xk > ) ! 0 as n ! 1 for all > 0. p Remark. For a vector Xn 2 R . It is equivalent to have Xn(j) ! X(j) for all 1 ≤ j ≤ p and to have Xn ! X. k d Denition. Let Xn;X be random vectors in R . Xn −! X as n ! 1 or Xn converges to Xin distribution if P(Xn ≤ t) ! P(X ≤ t) for all t where the map t ! P(X ≤ t) is continuous. 5 Proposition. a:s: P d - Xn −−! X implies Xn −! X implies Xn −! X as n ! 1. d - Xn;X with values in X ⊆ R and g : X! R a continuous function. en Xn ! X a.s./in P/in d implies g(Xn) ! g(X) a.s./in P/in d. is is called the continuous mapping theorem. d d Slutsky’s Lemma Suppose Xn −! X and Yn −! c, where c is a deterministic random variable that takes the same value with probability 1. en as N ! 1: P (i) Yn −! c d (ii) Xn + Yn −! X + c d Xn d X (iii) XnYn −! cX and if c 6= 0, −! . Yn c P (iv) If An are random matrices such that (An)ij −! Aij where Aij are eter- ministic, then d AnXn −! AX d (v) If Xn −! X as n ! 1, then (Xn)n2N is bounded in probability, or Xn = Op(1). is means that for all > 0, there exists M() < 1 such that P(kXnk > M()) < . 2.1 Law of Large Numbers and Central Limit eorem Proposition (Weak law of Large Numbers (WLLN)). For Xa; ··· Xn iid copies from X ∼ P with Var(X) < 1, we have: 1 X P X¯ − X −! E(X) n n i 1 P Var(x) Proof.
Details
-
File Typepdf
-
Upload Time-
-
Content LanguagesEnglish
-
Upload UserAnonymous/Not logged-in
-
File Pages16 Page
-
File Size-