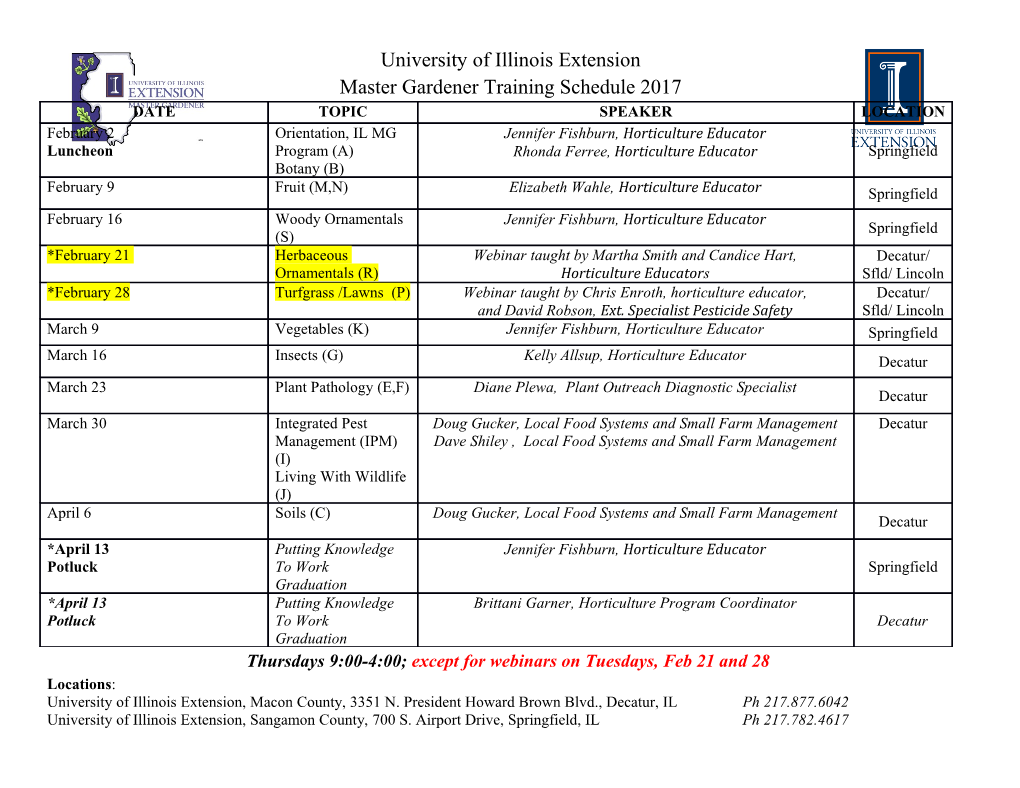
JOURNAL OF SCIENCE TECHNOLOGY AND EDUCATION 8(2), JUNE, 2020 ISSN: 2277-0011; Journal homepage: www.atbuftejoste.com Uncertainty Distribution Models for Key Input Parameters for Design and Analysis of Flexible Pavement in Nigeria Shuaibu, A. A., Kaura, J. M., Murana, A. A., Olowosulu, A. T. Department of Civil Engineering, Ahmadu Bello University, Zaria. ABSTRACT The road transportation sector is cardinal to accessing the ARTICLE INFO growth and socio-economic development of any country. This Article History Received: October, 2019 is because it contributes directly and immensely to support Received in revised form: Dec., 2019 other sectors of the economy to function properly. Flexible Accepted: April, 2020 pavement is a multi-layer system of which its design is mostly Published online: June, 2020 based on empiricism, assuming a deterministic process with final output as pavement thickness. Each layer thickness is KEYWORDS designed and placed in a manner that the wheel loading does Uncertainty, distribution model, layer not become excessive for the subgrade to bear. Various thickness, layer stiffness, variability studies have revealed uncertainties due to variations of thickness and layer modulus which could result in premature failure of the pavement. This variability could be as a result of poor design practice, inadequate quality control, complex traffic loading, complex material properties, lack of fit of design model as well as subgrade characteristic. To address these variabilities many highway agencies were forced into probabilistic design approach to accommodate uncertainties. Of crucial importance to this type of design is the characterization of material properties and establishment of appropriate probability distribution models. This study focused on the development of probability distribution models of key input pavement parameter to the design and analysis of flexible pavement. Data on the geometry and material properties were collected from Pavement Evaluation Unit (PEU), Kaduna and Federal Ministry of Power Works and Housing (FMPWH), Abuja. The probability distribution models of the variation of layer thicknesses, asphalt stiffness, base stiffness and subgrade stiffness were developed using Easy fit 5.5 statistical packages. The results of the studies revealed that the best distribution model for the layer thicknesses as well as stiffness considered in the study is the normal distribution. INTRODUCTION variability and uncertainty in pavement The large stochastic/random performance and may increase the cost of elements in pavement deterioration caused maintenance and rehabilitation over time. by immeasurable and unpredictable factors Analysis of data obtained by in situ core and (Harvey, 2012) introduces a measure of ground penetration radar indicates spatial Corresponding author: Shuaibu, A. A. [email protected], Department of Civil Engineering, Ahmadu Bello University, Zaria © 2020, Faculty of Technology Education, ATBU Bauchi. All rights reserved 320 JOURNAL OF SCIENCE TECHNOLOGY AND EDUCATION 8(2), JUNE, 2020 ISSN: 2277-0011; Journal homepage: www.atbuftejoste.com variation in pavement thickness layer. design inputs are, therefore fundamentals Likewise, the variation of layer modulus in developing a probabilistic-based design (Maji et al., 2016). that evaluates reliability (Dalla Valle and Uncertainty in engineering analysis Thom, 2016). An important aspect to this and design is commonly defined as approach is the appropriateness or fitness knowledge incompleteness due to inherent of distribution models to the substantive deficiencies in acquired knowledge. situation and/or the data sample of interest It can be used to characterize the state of a and this is the solution the test of goodness- system as being unsettled or in doubt, such of-fit (GOF) seek to proffer. as the uncertainty of the outcome (Ayyub The test is very relevant in risk and Klir, 2006). Because uncertainty is an analysis and is used to check how close the important dimension in the analysis of risks, data set distribution is to the hypothesized the nature and ways of dealing with these theoretical distribution (Babashamsi et al., uncertainties have been of interest to the 2016). The simplest case of a GOF test is a highway community for a long time. statistical null hypothesis of a completely Probability is the mechanism specified distribution against all possible employed to manage the uncertainty that alternative distributions (Anderson, 1995). underlies all real-world data and Thus, the outcome of the GOF test is used phenomena. It assists in gauging the belief to statistically verify an assumed theoretical and to quantify the lack of certitude that is distribution used in design and analysis or inherent in the process that generates the to disprove it. Fitting each random variable data been analyzed (Martinez and Martinez, into the best probability distribution model 2002). For example, to adequately simulate is key to performance prediction and a real system, one needs to understand the ultimately management decisions on any probability distributions that correctly road pavement under considerations. model the underlying processes. According to Kaura, (2015), in the Uncertainty of probabilistic input assignment of distribution models to a consists of specifying the types of random variable, two or more distribution probability distributions of the variables may appear to be plausible probability under consideration as well as the distribution models. In that case, the test of parameters of the distributions goodness-of-fit (GOF) can be used to (Oberguggenberger and Fellin, 2004). An delineate the relative degree of validity of adequate understanding of the influence of the different distributions. input parameter variability on the output of Goodness-of-Fit tests is a engineering computations requires that the procedure to determine whether a sample uncertainty itself be captured in n observation, x1, … xn of certain distribution mathematical terms (Oberguggengerger, is considered as a sample from a given 2004). In the specific area of pavement specified distribution (Anderson, 2011). engineering, techniques such as Calculating statistics of Goodness-of-Fit probabilistic analyses, general stochastic helps to rank the fitted distributions analysis and random fields have been used according to the quality of fit over the raw to explore the impact of material variability data (Mehrannia, and Pakgohar, 2014). The and uncertainty quantification in pavement ultimate ends for performing this GOF design and performance (Castillo and Caro, analysis is to obtain information which 2014). helps take best-informed decisions under Quantification and analyzing uncertainty conditions using best-fitted variability of pavement materials and distribution. This distribution is a model of Corresponding author: Shuaibu, A. A. [email protected], Department of Civil Engineering, Ahmadu Bello University, Zaria © 2020, Faculty of Technology Education, ATBU Bauchi. All rights reserved 321 JOURNAL OF SCIENCE TECHNOLOGY AND EDUCATION 8(2), JUNE, 2020 ISSN: 2277-0011; Journal homepage: www.atbuftejoste.com the stochastic process, which is faced in the parameters with great influence on the real world. variability of predicted fatigue performance Material variability can be without considering variable loads are; described in statistical terms such as mean, thickness and stiffness of asphalt concrete standard deviation together with its layer. Also, for rutting, the parameters of probability density distribution (Dalla Valle, great influence are; base thickness, asphalt 2005). A useful dimensionless way of concrete thickness, and stiffness of expressing the variability of a material’s subgrade. However, when the effect of property is to use the ratio of the standard variable load was considered, the output deviation over the mean, known as the variability for fatigue and rutting was coefficient of variation (COV). Knowledge of doubled. This is an indication that traffic is a the coefficient of variation of each design single most important parameter in input is extremely important to more predicting fatigue and rutting life of the accurately estimate their influence on the pavement. predicted pavement life (Dalla Valle and Dalla Valle and Thom, (2016), Thom, 2016). researched the reliability of pavement As earlier stated, the sources of design. The research revealed that the variability in pavement engineering have parameters with the greatest influence on been of interest to researchers for a very the variability of predicted fatigue long time. Researchers have carried out performance are the asphalt stiffness studies in the field of reliability analysis on modulus and thickness. The parameters pavement and have concluded on with the greatest influence on the parameters that are crucial to pavement variability of predicted deformation performance. Since these parameters are performance are the granular sub-base key, they must be treated as random thickness, the asphalt thickness and the variables in a probabilistic non-arbitrary subgrade stiffness. manner to account for variabilities that are Maji et al., (2016) developed a probabilistic associated with them to avoid the approach for asphaltic overlay design by premature failures and to maintain the considering variability in input parameter. A pavements at a satisfactory level service. sensitivity analysis of pavement layer Chou, (1990) carried out a study on moduli performed in this study revealed
Details
-
File Typepdf
-
Upload Time-
-
Content LanguagesEnglish
-
Upload UserAnonymous/Not logged-in
-
File Pages16 Page
-
File Size-