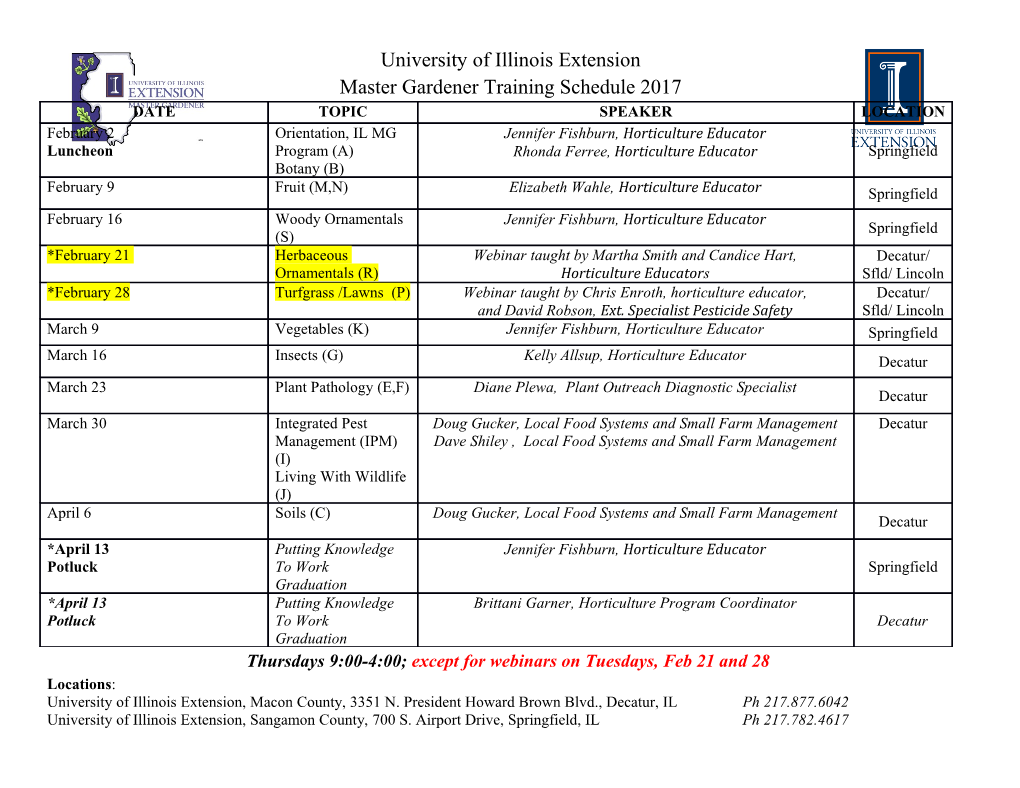
1 The need for spatio-temporal modeling to determine catch-per-unit effort based indices of 2 abundance and associated composition data for inclusion in stock assessment models. 3 Maunder, M.N.1,2, Thorson, J.T.3, Xu, H.,1, Oliveros-Ramos, R.1, Hoyle, S.D.4, Tremblay-Boyer, 4 L.5, Lee, H.H.,6, Kai, M.7, Chang, S.K.8, Kitakado, T.9, Albertsen, C.M.10, Minte‑Vera, C.V.1, 5 Lennert-Cody, C.E. 1, Aires-da-Silva, A.M. 1, and Piner, K.R.6 6 1: Inter-American Tropical Tuna Commission, 8901 La Jolla, Shores Dr., La Jolla, CA, 92037‑1508, USA 7 2: Center for the Advancement of Population Assessment, Methodology, La Jolla, CA, USA 8 3: Habitat and Ecosystem Process Research program, Alaska Fisheries Science Center, NMFS, NOAA, Seattle, WA, 9 USA 10 4: National Institute of Water and Atmospheric Research Ltd (NIWA), 217 Akersten Street, Port Nelson, New 11 Zealand 7011 12 5: Dragonfly Data Science, 158 Victoria St, Wellington, New Zealand 6011 13 6: Southwest Fisheries Science Center, National Marine Fisheries Service (NMFS), NOAA, La Jolla, CA, U.S.A. 14 7: National Research Institute of Far Seas, Fisheries (NRIFSF), Japan Fisheries, Research and Education Agency, 15 Shimizu, Shizuoka, Japan 16 8: Institute of Marine Affairs, National Sun Yat-sen University, Kaohsiung, Taiwan 17 9: Tokyo University of Marine Science and Technology, 5-7, Konan 4, Minato-ku, Tokyo 108-8477 Japan 18 10: National Institute of Aquatic Resources, Technical University of Denmark, Kemitorvet 201, DK-2800 Kgs. 19 Lyngby, Denmark 20 Abstract 21 We describe and illustrate a spatio-temporal modelling approach for analyzing age- or size- 22 specific catch-per-unit-effort (CPUE) data to develop indices of relative abundance and 23 associated composition data. The approach is based on three concepts: 1) composition data that 24 are used to determine the component of the population represented by the index should be 25 weighted by CPUE (abundance) while the composition data used to represent the fish removed 26 from the stock should be weighted by catch; 2) due to spatial non-randomness in fishing effort 27 and fish distribution, the index, index composition, and catch composition, should be calculated 28 at a fine spatial scale (e.g., 1°x1°) and summed using area weighting; and 3) fine-scale spatial 29 stratification will likely result in under-sampled and unsampled cells and some form of 30 smoothing method needs to be applied to inform these cells. We illustrate the concepts by 31 applying them to yellowfin tuna (Thunnus albacares) in the eastern Pacific Ocean. 32 Key Words: catch-per-unit-effort, CPUE, spatio-temporal model, index of abundance, catch-at- 33 age, length composition 34 1 35 1. Introduction 36 Fisheries stock assessment is the gold standard for providing management advice. Age- or size- 37 structured population dynamics models are fit to multiple data sets to estimate model parameters 38 and associated derived management quantities. The main data types, other than catch, are indices 39 of relative abundance and composition data representing the proportions of the sampled 40 population within different age, length, sex, and/or weight categories. The indices provide 41 information on trends in abundance. The composition data provide information on the 42 component of the population represented by the index, and the size or age of the fish removed by 43 the fishery. They both provide information on absolute abundance (Maunder and Piner, 2015). 44 Therefore, it is essential that the indices of relative abundance and composition data are analyzed 45 appropriately to ensure they are as precise and accurate as possible. 46 Preferably, indices of abundance are based on well-designed surveys, are proportional to 47 abundance, and are precise. Unfortunately, surveys are not possible for many stocks due to 48 logistical and funding limitations. Therefore, many stock assessments, such as those conducted 49 for tunas worldwide, rely on indices of relative abundance based on fishery catch-per-unit-of- 50 effort (CPUE) data. These fishery-dependent indices are influenced by several factors that may 51 invalidate the assumption that the index is proportional to abundance (Harley et al., 2001; 52 Maunder et al., 2006a; Thorson et al., 2017c). Of particular concern is that fishing effort is not 53 randomly or systematically distributed over the whole stock area, and is rather likely to be 54 concentrated where fish are abundant. Therefore, expanding indices from sampled to under- 55 sampled or unsampled areas may lead to positive bias. Similar issues also apply to the 56 composition data, but are typically not addressed. 57 There is a large body of literature describing alternative CPUE “standardization” approaches 58 to minimize the influence of factors other than abundance on the final index (Maunder and Punt, 59 2004). Typically, the CPUE is standardized using a Generalized Linear Model (GLM), or a 60 similar method, to account for factors that impact CPUE (e.g., vessel and gear characteristics, 61 season, location, and environmental conditions). There are also numerous examples of more 62 sophisticated approaches to deal with specific issues or fine-tune a component of the 63 standardization method. For example, Hinton and Nakano (1996) used a mechanistic model to 64 match the three-dimensional spatial distribution (latitude, longitude, and depth) of fishing effort, 65 with environmental conditions and fish habitat preference to standardize CPUE for blue marlin. 66 Many authors have focused on the fine scale spatial distribution of CPUE (e.g., Walters, 2003; 67 Carruthers et al., 2011; Thorson et al., 2017c), while others have used broader scale spatial strata 68 to standardize CPUE (e.g., Punt, et al., 2001; Gruss et al., 2019). 69 Historical approaches to deal with spatial variation in CPUE commonly used a simple GLM 70 that included location as a factor without an additional term for time-space interaction. This 71 approach implicitly assumes that the estimated year effect (i.e., the temporal trend), which is 72 assumed to be a proxy of relative abundance, is the same in each spatial stratum, and that only 73 the average CPUE differs among strata. The assumptions underlying this model can lead to bias 74 in the estimated index of relative abundance in several situations, including when the spatial 75 distribution of the stock changes over time (e.g., Punt et al., 2000b). Such a situation can 76 typically be identified when the interaction term between spatial stratum and year is statistically 77 significant, or the time series of year effects from different strata show different trends. 78 In general, statistically significant interaction terms between year and another categorical 79 variable that result in meaningful differences in standardized trends are problematic. Calculating 80 the index requires choosing a level for the variable interacting with year, and thus the estimated 2 81 trend will depend on the chosen level (Maunder and Punt, 2004). If the interaction is treated as a 82 random effect, calculating the index requires specifying the average value for the variable that is 83 treated as random. When the variable interacting with year is a spatial factor, a more appropriate 84 approach may be to use “area-weighting”, where the index is calculated as the weighted sum of 85 model predictions over the levels of the spatial factor. The weights are equal to the spatial area 86 associated with each factor. However, this approach assumes that the sampling represents all 87 locations in a spatial stratum, including poorly-sampled locations, which is unlikely to be even 88 approximately true for large spatial strata. Spatio-temporal modeling methods, which can use 89 information on CPUE from neighboring locations to improve estimation of the spatial effects 90 throughout the area occupied by the stock, can be based on finer spatial strata and should lead to 91 improved indices compared to those derived from simple area-weighting. In cases of large 92 differences in the year effect among spatial strata, the stock may be modelled as multiple 93 independent or interacting populations, and each population assessed based on its respective 94 stratum’s index of relative abundance (Punt, 2019). 95 CPUE-based indices of relative abundance used in stock assessment models are 96 representative of the population component caught in the fishery, rather than the entire 97 population. Typically, this issue is addressed by using an age- or size-based selectivity curve that 98 is estimated by fitting to fishery composition data. In most cases, the selectivity curve is used to 99 characterize both the catch and the index of abundance. Naively, this makes sense, since both 100 catch and the index of abundance are derived from the same fishery (e.g. gear). However, 101 selectivity in the stock assessment model does not simply represent contact selectivity (e.g., a 102 fish being trapped in a gillnet as it tries to pass through), but also availability, which can be a 103 consequence of the spatial structure of the fleet relative to the stock, and is likely to change over 104 time (Sampson, 2014; Waterhouse et al., 2014). The index is used in the assessment model to 105 represent changes in abundance while the catch represents mortality due to fishery removals. 106 Catch is not necessarily distributed spatially in proportion to abundance, and the “selectivity” in 107 the stock assessment will differ between the index and the catch when the composition data 108 differ systematically among spatial strata, and catch distribution among strata changes through 109 time. In general, the index selectivity should represent the total vulnerable (i.e. filtered through 110 the gear selectivity) abundance across the domain of the index, while the catch selectivity will 111 represent the vulnerable abundance available to the fishery adjusted as the fishery spatial 112 distribution changes over time.
Details
-
File Typepdf
-
Upload Time-
-
Content LanguagesEnglish
-
Upload UserAnonymous/Not logged-in
-
File Pages41 Page
-
File Size-