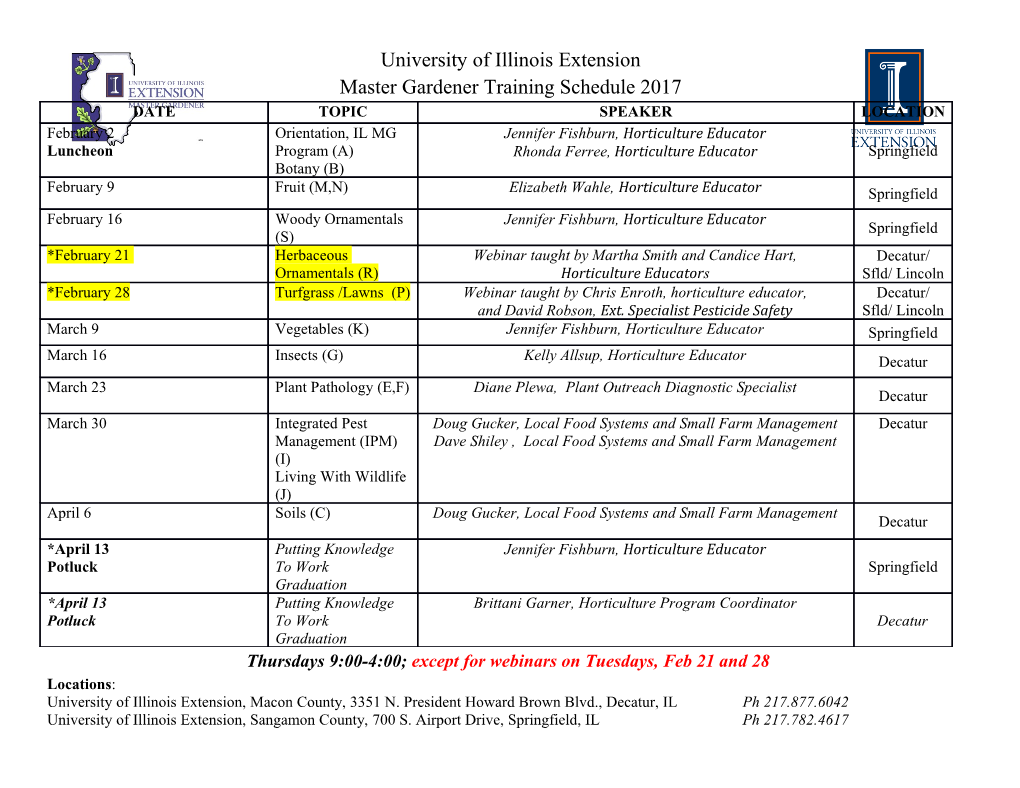
applied sciences Article Which Local Search Operator Works Best for the Open-Loop TSP? Lahari Sengupta *, Radu Mariescu-Istodor and Pasi Fränti Machine Learning Group, School of Computing, University of Eastern Finland, 80101 Joensuu, Finland; [email protected].fi (R.M.-I.); [email protected].fi (P.F.) * Correspondence: [email protected].fi; Tel.: +358-417232752 Received: 6 August 2019; Accepted: 17 September 2019; Published: 23 September 2019 Abstract: The traveling salesman problem (TSP) has been widely studied for the classical closed-loop variant. However, very little attention has been paid to the open-loop variant. Most of the existing studies also focus merely on presenting the overall optimization results (gap) or focus on processing time, but do not reveal much about which operators are more efficient to achieve the result. In this paper, we present two new operators (link swap and 3–permute) and study their efficiency against existing operators, both analytically and experimentally. Results show that while 2-opt and relocate contribute equally in the closed-loop case, the situation changes dramatically in the open-loop case where the new operator, link swap, dominates the search; it contributes by 50% to all improvements, while 2-opt and relocate have a 25% share each. The results are also generalized to tabu search and simulated annealing. Keywords: traveling salesman problem; open-loop TSP; randomized algorithms; local search; NP–hard; O–Mopsi game 1. Introduction The traveling salesman problem (TSP) aims to find the shortest tour for a salesperson to visit N number of cities. In graph theory, a cycle including every vertex of a graph makes a Hamiltonian cycle. Therefore, in the context of graph theory, the solution to a TSP is defined as the minimum-weight Hamiltonian cycle in a weighted graph. In this paper, we consider the symmetric Euclidean TSP variant, where the distance between two cities is calculated by their distance in the Euclidean space. Both the original problem [1] and its Euclidean variant are NP–hard [2]. Finding the optimum solution efficiently is therefore not realistic, even for the relatively small size of the input. O–Mopsi (http://cs.uef.fi/o-mopsi/) is a mobile-based orienteering game where the player needs to find some real-world targets, with the help of GPS navigation [3]. Unlike a prior instruction of visiting order in the classical orienteering, O–Mopsi players have to decide the order of visiting targets by themselves. The game finishes immediately when the last target is reached. The player does not need to return to the start target. This corresponds to a special case of the orienteering problem [4]. Finding the optimum order by minimizing the tour length corresponds to solving open-loop TSP [5], because the players are not required to return to the start position. The open-loop variant is also NP–hard [2]. The solution to a closed-loop TSP is a tour; however, the solution to an open-loop TSP is an open path. Hence, a TSP with the size N contains N links in its closed-loop solution and N-1 in its open-loop solution (Figure1). However, the optimum solution to an open-loop TSP is not usually achievable by removing the largest link from the optimum solution to the closed-loop TSP (Figure1). Appl. Sci. 2019, 9, 3985; doi:10.3390/app9193985 www.mdpi.com/journal/applsci Appl. Sci. 2019, 9, 3985x FOR PEER REVIEW 22 of of 2426 Appl. Sci. 2019, 9, x FOR PEER REVIEW 2 of 26 Figure 1. Difference between open- and closed-loop traveling salesman problem (TSP). FigureFigure 1. 1. DifferenceDifference between between open open-- and and closed closed-loop-loop traveling traveling salesman salesman problem problem ( (TSP).TSP). In O–Mopsi, players are not required to solve the optimum tour, but finding a good tour is still In O–Mopsi, players are not required to solve the optimum tour, but finding a good tour is still an essentialIn O–Mopsi, part ofplayers the game. are not Experiments required to showed solve the that, optimum among tourthe players, but finding who completeda good tour a isgame, still an essential part of the game. Experiments showed that, among the players who completed a game, anonly essential 14% had part found of the the game. optimum Experiments tour, even showed if the that, number among of targetsthe players was aboutwho completed 10, on average a game, [3]. only 14% had found the optimum tour, even if the number of targets was about 10, on average [3]. onlyThe optimum14% had foundtour is the still opt neededimum fortour reference, even if whenthe number analyzing of targets the performance was about of10, the on players.average This[3]. The optimum tour is still needed for reference when analyzing the performance of the players. This is Theis usually optimum made tour as is a stillpost needed-game analysisfor reference but can when also analyzing happen duringthe performance real-time ofplay the. A players. comparison This usually made as a post-game analysis but can also happen during real-time play. A comparison can be iscan usually be made made with as a thepost tour-game length analysis or withbut can the also visiting happen order. during The real game-time creator play. Aalso comparison needs an made with the tour length or with the visiting order. The game creator also needs an estimator for the canestimator be made for thewith tour the length, tour lengthas it will or be with published the visiting as a part order. of the The game game info. creator If the gamealso needsis created an tour length, as it will be published as a part of the game info. If the game is created automatically on estimatorautomatically for the on tour the length,demand, as TSPit will must be published be solved asreal a part-time. of Thethe gameoptimality info. Ifis thehighly game desired is created, but the demand, TSP must be solved real-time. The optimality is highly desired, but can sometimes be automaticallycan sometimes on be the compromised demand, TSP for must the sake be solvedof fast processing.real-time. The optimality is highly desired, but compromised for the sake of fast processing. can sometimesThe number be compromised of targets in for the the current sake of O fast–Mopsi processing. instances varies from 4 to 27, but larger The number of targets in the current O–Mopsi instances varies from 4 to 27, but larger instances instancesThe number may appear. of targets For the in smaller the current instances, O–Mopsi the rela instancestively simple varies branch from- and4 to-bound 27, but algorithm larger may appear. For the smaller instances, the relatively simple branch-and-bound algorithm is fast enough instancesis fast enough may appear.to produc Fore the smalleroptimum instances, tour. However, the rela tivelyfor the simple longer branch instances,-and it-bound might algorithm take from to produce the optimum tour. However, for the longer instances, it might take from minutes to hours. isminutes fast enough to hours. to produc Alonge thewith optimum the exact tour. solver, However, a faster for heuristic the longer algorithm instances, is therefore it might takeneeded from to Along with the exact solver, a faster heuristic algorithm is therefore needed to find the solution more minutesfind the tosolution hours. Alongmore quickly, with the in exact spite solver, of the adanger faster heuristicof occasionally algorithm resulting is therefore in a sub needed-optimal to quickly, in spite of the danger of occasionally resulting in a sub-optimal solution. Besides O–Mopsi, findsolution. the solution Besides more O–Mopsi, quickly, TSP in arisesspite ofin the route danger planning of occasionally [6], orienteering resulting problem in a subs -optimal[7], and TSP arises in route planning [6], orienteering problems [7], and automated map generation [8]. solution.automated Besides map generation O–Mopsi, [8]. TSP arises in route planning [6], orienteering problems [7], and An example of O–Mopsi play is shown in Figure2, with a comparison to the optimum tour automatedAn example map generation of O–Mopsi [8]. play is shown in Figure 2, with a comparison to the optimum tour (reference). For analyzing, we calculate several reference tours: optimum, fixed-start, and dynamic. (reference).An example For analyzing,of O–Mopsi we play calculate is shown several in Figure reference 2, with tours: a comparisonoptimum, fixed to -thestart, optimum and dynamic tour . The optimum tour takes into account the fact that the players can freely choose their starting point. (reference).The optimum For tour analyzing, takes into we account calculate the several fact that reference the players tours: can optimum freely choose, fixed- start,their startingand dynamic point.. Since selecting the best starting point is challenging [9], we also calculate two alternative reference TheSince optimum selectin gtour the takesbest startinginto account point the is challengingfact that the [9], players we also can calculatefreely choose two alternativetheir starting reference point. tours from the location where the player started. Fixed-start is the optimum tour using this starting Sincetours selectinfrom theg thelocation best startingwhere the point player is challenging started. Fixed [9],- startwe also is the calculate optimum two tour alternative using this reference starting point. The dynamic tour follows the player’s choices from the starting point but re-calculates the new tourspoint. from The thedynamic location tour where follows the playerthe player’s started. choices Fixed -fromstart isthe the st optimumarting point tour but using re-calculates this starting the optimum every time the player deviates from optimum path. Three different evaluation measures are point.new optimumThe dynamic every tour time follows the playerthe player’s deviates choices from from optimum the st artingpath.
Details
-
File Typepdf
-
Upload Time-
-
Content LanguagesEnglish
-
Upload UserAnonymous/Not logged-in
-
File Pages24 Page
-
File Size-