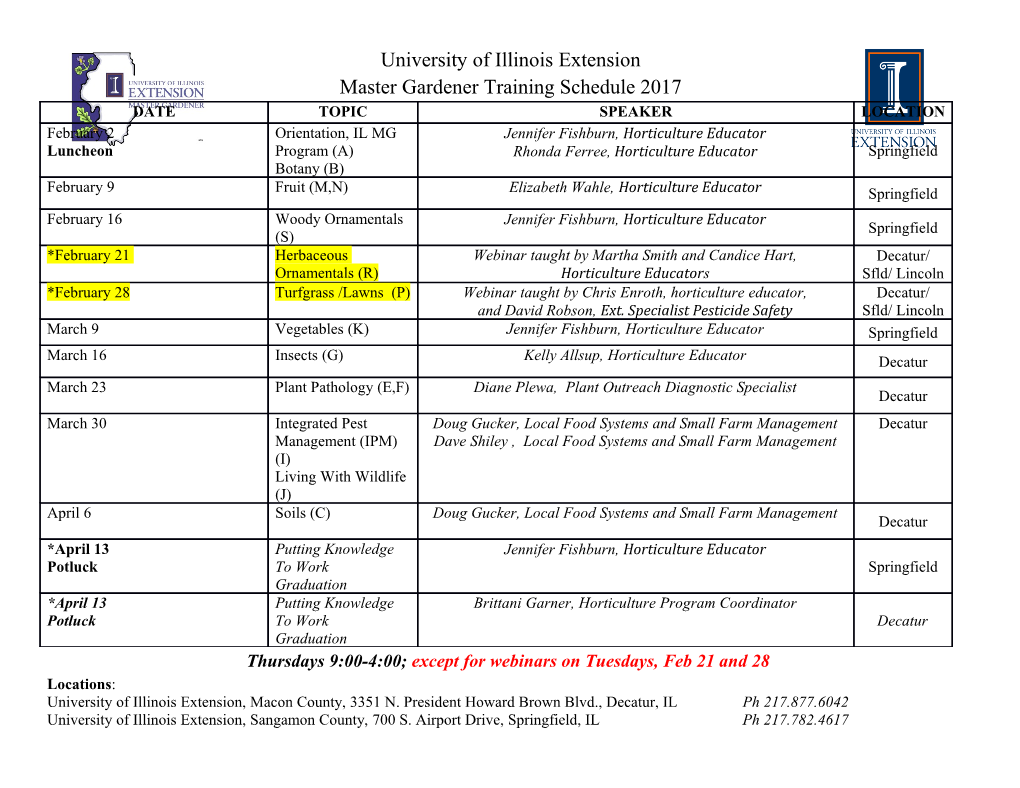
Toward de novo design of immune silent protein and peptide therapeutics Lance Stewart, Ph.D. MBA Chief Strategy and Operations Officer, UW IPD FDA – CERSI Collaborative Workshop “Predictive Immunogenicity for Better Clinical Outcomes” October 3 and 4, 2018 10-4-18 1 Design a new world of synthetic proteins • Founded in 2012 by Dr. David Baker • Organized within the School of Medicine, Biochemistry • ~140 Person Umbrella Organization • Faculty PIs (Baker, DiMaio, King, Gu, Bradley) • WRF Innovation Fellows (21) • Translational Investigators (1) • Research Staff (17) • Postdocs (27) • Graduate Students (36) • Admin (6) • Undergrads (27) • High School Student (1) • Using 10% of all UW Internet Traffic 10-4-18 2 PROTEIN STRUCTURE PREDICTION Amino Acid Sequence Protein Tertiary Structure PROTEIN DESIGN 3 The folded states of proteins are likely global energy minima for their sequences Unfolded Protein Structure Prediction: find lowest energy structure for fixed sequence Energy Protein Design: find a sequence for which desired structure has lowest energy Sample structures and sequences, and evaluate Native state energies using Rosetta molecular modeling suite 4 15 Years Ago (2003): First De Novo Design • First computational de novo design of a novel protein fold (Top 7) with atomic level accuracy. Model X-ray Structure Kuhlman et al, Science 2003 10-4-18 5 Today: The Coming of Age of De Novo Protein Design • Designed completely from scratch • Sequence unique from existing proteins in nature • Experimentally verified by high-resolution structure Top7 Kuhlman, Baker 1988 1997 2003 2011 2012 2013 2014 2015 2016 FSD-1 Mayo Coiled Coils: Degrado, Reagan, Kim, Harbury, Eisenberg, Alber, Woolfson Huang PS*, Boyken SE*, and Baker D. Nature 2016 10-4-18 6 De novo protein design Native sequences Neanderthal De novo protein design protein design Number of 100 residue amino sequences: 20100 = 1.3*10130 Number of naturally occurring proteins: ~1015 10-4-18 7 HISTORIC MOMENT IN PROTEIN DESIGN • We’ve learned how to design proteins from scratch. • There is finally enough computing power to do it. • Genomics enables building and testing designs in the lab. 10-4-18 8 De novo protein design method Design a Low Energy Define Design Strain-Free Select Sequences that Blueprint Backbones Sequences for Fold into Designed Backbones Structure Rosetta energy Rosetta RMSD Size and arrangement Backbones sampled VERY LARGE number of Selection of designed of secondary using fragments of possible amino acid sequences with lowest structures natural proteins sequences energies close to design model 10-4-18 9 De novo protein design method Gene Library Generate Yeast Surface FACS and Next-Gen DNA Select Individual Synthesis Display Libraries Sequencing Designs for Verification FACS: Fluorescein-Anti-Myc Ab to probe expression & R-Phycoerythrin Target of Limited Interest to probe binding activity Functional Myc-tag Protease FITC DESIGNED Binder PROTEIN Target Myc-tag Aga2 Aga1 DESIGNED Limited PROTEIN YEAST Aga2 Heat Aga1 CELL ofbinding Level YEAST Expression CELL Level of expression ~100,000 genes Transform yeast with Individual clones encoding mini- plasmids encoding Identify gene sequences expressing designed proteins ~60 aa minibinder design library, encoding functional minibinders are used to designed minibinders and treat with limited verify function protease and / or heat 10-4-18 10 Protein Design Takes Us Beyond Traditional Small Molecules and Antibodies • Computational design enables bottom up creation oF totally new Functional designer peptides, proteins, and nanomaterials. Traditional Computationally Traditional Computationally Small Molecule Designed Antibodies or Designed Drugs Mini-Proteins (<100 aa) Smaller Ab Smart Nanomaterials <1 KDa. Macrocycles (7-16 aa) Fragments 50 KDa. – 3 MDa. 1-12 KDa. 13-160 KDa. 10-4-18 11 Large Scale Design of Hyperstable Mini-Proteins Rocklin et al Science 2017 10-4-18 12 Design of Disulfide Stapled and Cyclic Mini-Proteins with Precise Control of Shape and Size αββ (0.99Å) βαβ (0.7Å) ββα (0.86Å) Mixed-chirality peptides stapled c(α α ) (0.79Å) - R L peptides Disulfide c(αα) (1.03Å) c(ααα) (1.06Å) c(ββ) (1.26Å) Design Models / NMR structure Cyclic peptides Bhardwaj, G*., Mulligan V.*, Bahl. C* et al., Nature (2016) 13 Rosetta can Design Peptide Macrocycles with Near Atomic Level Accuracy 7mer (rmsd: 0.8 Å) 7mer (rmsd: 1.1 Å) 8mer (rmsd: 0.3 Å) 9mer (rmsd: 1.2 Å) 10mer (rmsd: 0.8 Å) 10mer (rmsd: 0.5 Å) Hosseinzadeh, P. *, Bhardwaj, G*., Mulligan V.* et al., Science (2017) L-AA/D-AA computational model / NMR ensemble 14 Designed proteins show high thermal stability and resistance to chemical denaturation NC_HEE_D1 Bhardwaj, G*., Mulligan V.*, Bahl. C* et al., Nature (2016) 15 Immunogenicity ? 10-4-18 16 Causes of Immune Responses to Proteins 1. Multivalency = Aggregate Large Size Instability 2. MHC-II T-cell Epitopes 3. TCR - MHC-II T-cell Epitopes / Ag Primed B-Cell Synapse Sauerborn M, Brinks V, Jiskoot W, Schellekens H. Immunological mechanism underlying the immune response to recombinant human protein therapeutics. Trends Pharmacol Sci. 2010 Feb;31(2):53-9. 10-4-18 17 Features of Immunogenic Substances vs. De Novo Designed Mini-Binders ImmunogenicImmunogenic Protein Designed MiniDesigned-Binder / Macrocycle Mini-Binder / Protein Macrocycle Large size (> 10 KDa.) Small (< 10 KDa.) • Large size (> 10 KDa.) • Small (< 10 KDa.) Multivalent = B-cell receptor crosslinking Monomeric • Multivalent = B-cell receptor • Monomeric crosslinking • Hyper-Stable Poor• Poor stability stability = Denaturation = Denaturation = = Hyper-Stable• Not (>80 Self ˚C, Protease resistant) Aggregation = Multivalent Aggregation = Multivalent • Hard to digest or D-handed un- Not• SelfNot Self Not Self natural amino acids make it hard T•-cellT -epitopescell epitopes (MHC -(MHCII) require-II) require processing Hard to digestto or process. D-handed un-natural amino and presentation.processing and presentation. acids make• itRe hard-Designable to process. • Depends on route of entry Re• -designDepends (deimmunize) on route hardof entry Re-design (immune silence) easier delivery (e.g. mucosal, delivery (e.g. mucosal, Longsubcutaneous, T1/2 resident time intravenous) (Weeks) Short T1/2 (minutessubcutaneous, to hours) intravenous) • Short serum half-life Delivery• Adjuvants is often presentI.V. or S.C. to (systemic) trigger Delivery options, I.V., S.C., Aerosol (localized) • Simple formulations (PBS) Excipientinnate formulations immune function Simple formulations (PBS) 10-4-18 18 Designed Influenza Therapeutic Mini-Binder • Low cost potent, inhalable, long-lived, broadly neutralizing anti-viral therapeutic. • 40 amino acids (synthetic or recombinant) Heat Stable Aerosol • 2 disulfide bonds, Tm > 95˚C, Kd > ~5 nM. • In vitro Neutralization EC50 < 0.003 ug/ml. • Not immunogenic in mice. Flu Binder, 0.03 mg/kg All Mice Survive 100 80 Control 0.03mg/kg HB1.6928.2.3 60 40 Survival(%) Survival Control 20 0 02468101214 Time (d) Days After Flu Image by IPD and Cognition Studio Aaron Chevalier, Daniel Silva, Gabe Rocklin, David Baker et al., Nature 2017 10-4-18 19 Potent Anti-Flu Mini-binder is Hyperstable Pharmacokinetics Trypsin Resistant Heat Stable T1/2 = ~20 min. 10000 CS15134IV IV CS15134SQ SQ 1000 100 Plasma Conc (ng/mL) 10 1 0.0 4.0 8.0 12.0 16.0 20.0 24.0 Time (hrs) Aaron Chevalier, Daniel Silva, Gabe Rocklin, David Baker et al. , Nature 2017 10-4-18 20 Designed Mini-Binders Elicit Little or No Antibodies in Mice Dosing Protocols IgG Responses in ELISA (1:500 serum) Intravenous Intranasal Dosing 3mg/kg i.v. 1 2 3 hIgG Weeks 0 3 6 9 Blood Draws 1 2 3 BSA Dosing 3mg/kg i.n. 1 2 3 Weeks 0 2 4 6 Blood Draws 1 2 3 Designs are much less immunogenic than hIgG or BSA in mice ! Aaron Chevalier, Daniel Silva, Gabe Rocklin, David Baker et al., Nature 2017 10-4-18 21 Repeat Dosing of Mini-Binders Does Not Alter Prophylactic Efficacy in Mice Minibinder Dosing Flu virus 3mg/kg i.v. or i.n 2x MLD50 of H1N1 CA09 1 2 3 4 14 Days Trial 0 3 6 12 13.5 15.5 Weeks Minibinder 0.3 mg/kg i.n. Complete prophylactic protection after 4 repeated doses from 8 weeks to 1 week prior to lethal flu virus challenge ! Aaron Chevalier, Daniel Silva, Gabe Rocklin, David Baker with Deb Fuller’s Lab et al. , Nature 2017 10-4-18 22 ~Congruence Between Computational and Experimental Saturation Site Mutagenesis (SSM) AA Substitution AA Substitution Position in Designed Sequence Position in Designed Sequence Can Re-Design Sequence to Reduce T-Cell Epitope Liability if Needed ! Change Effect Enhance Function Neutral Destroy Function Aaron Chevalier, Daniel Silva, Gabe Rocklin, David Baker et al. , Nature 2017 10-4-18 23 Structure and Function By Design • Through design, we can maintain structural features through design of an astronomical number of different amino acid sequences. • As such, numerous desired target product profile features are achievable through de novo design. – Size – pI – Stability – H-bonding networks = hydration sphere – Others. • By definition de novo designed protein sequences do not exist in nature and could be recognized as foreign! • How can we design immune silence ? 10-4-18 24 MHC-II displays peptides on the surface of cells for T-cell receptors • Peptide binding cleft between 2 domains • binds 15-24mers, 9mer core • P1,P4,P6,P9 “pocket” positions 10-4-18 25 Reducing the Liability of T-cell Epitopes by Design • Through Rosetta design, we can maintain structural features and alter the amino acid sequence to “silence” predicted or known offending T-cell epitopes. 1. Host Genome Sequences 2. Known Epitope Sequences Machine Learning Predictions 3. King C, Garza EN, Mazor R, Linehan JL, Pastan I, Pepper M, Baker D. Removing T-cell epitopes with computational protein design. Proc Natl Acad Sci U S A. 2014 Jun 10;111(23):8577-82. 10-4-18 26 Current Immunogenicity Testing Paradigm for De Novo Designed Proteins • Step 1.
Details
-
File Typepdf
-
Upload Time-
-
Content LanguagesEnglish
-
Upload UserAnonymous/Not logged-in
-
File Pages31 Page
-
File Size-