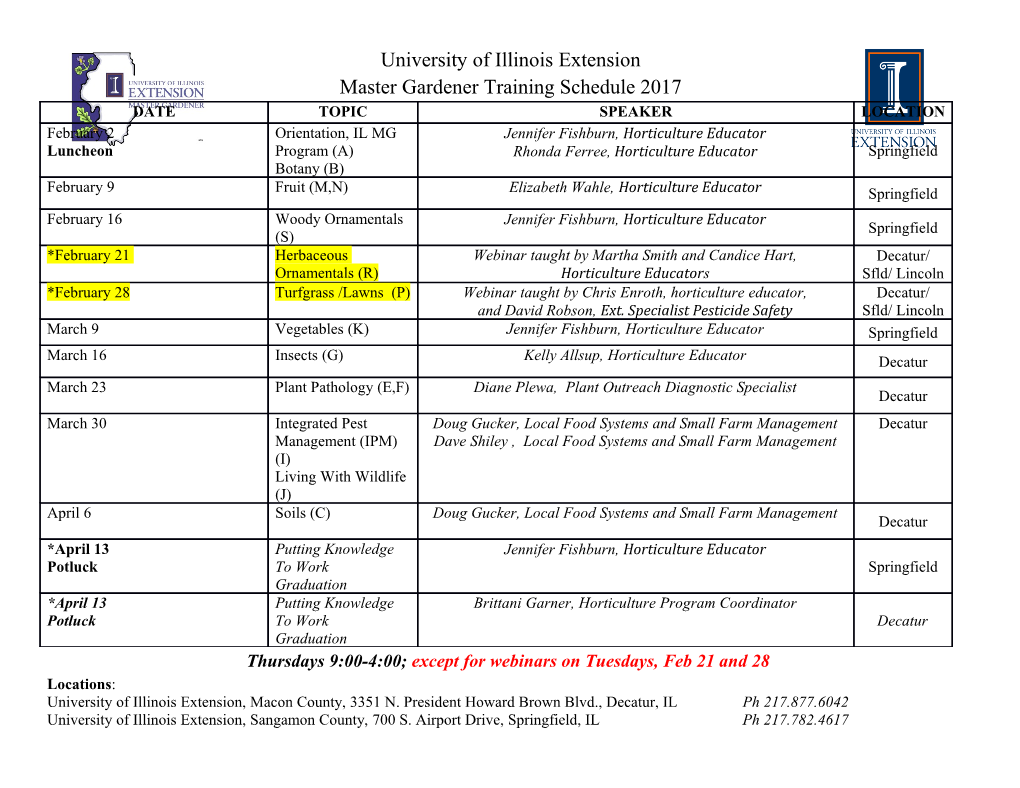
Journal of Hydrology 218 (1999) 13–34 Investigation and comparison of sampling properties of L-moments and conventional moments A. Sankarasubramanian1, K. Srinivasan* Department of Civil Engineering, Indian Institute of Technology Madras, Chennai 600 036, India Received 3 December 1997; accepted 26 January 1999 Abstract The first part of this article deals with fitting of regression equations for the sampling properties, variance of L-standard deviation (l2), and bias and variance of L-skewness (t3), based on Monte-Carlo simulation results, for generalised Normal (Lognormal-3) and Pearson-3 distributions. These fitted equations will be useful in formulating goodness-of-fit test statistics in regional frequency analysis. The second part presents a comparison of the sampling properties between L-moments and conventional product moments for generalised Normal, generalised Extreme Value, generalised Pareto and Pearson-3 distribu- tions, in a relative form. The comparison reveals that the bias in L-skewness is found to be insignificant up to a skewness of about 1.0, even for small samples. In case of higher skewness, for a reasonable sample size of 30, L-skewness is found to be nearly unbiased. However, the conventional skewness is found to be significantly biased, even for a low skewness of 0.5 and a reasonable sample size of 30. The overall performance evaluation in terms of “Relative-RMSE in third moment ratio” reveals that conventional moments are preferable at lower skewness, particularly for smaller samples, while L-moments are preferable at higher skewness, for all sample sizes. This point is illustrated through an application that seeks to obtain an appropriate regional flood frequency distribution for the 98 catchment areas located in the central region of India, spread over six hydrometeorologic subzones. ᭧ 1999 Elsevier Science B.V. All rights reserved. Keywords: Sampling methods; Generalised Normal, Pearson distributions; L-moments; L-skewness 1. Introduction distribution. Even though this method is conceptually simple, and the computations are straight-forward, it The method of moments has been one of the is found that the numerical values of the sample simplest and conventional parameter estimation tech- moments can be very different from those of the popu- niques used in statistical hydrology. In this method, lation from which the sample has been drawn, espe- while fitting a probability distribution to a sample, the cially when the sample size is small and/or the parameters are estimated by equating the sample skewness of the sample is considerable. If m, s 2, g, moments to those of the theoretical moments of the denote the mean, the variance and the skewness of the population, then the unbiased sample estimates of m, s 2, g, are expressed as: * Corresponding author. E-mail address: [email protected] (K. Srinivasan) 1 Xn 1 ; Presently Research Associate, Tufts University, MA 02155, m xi 1a USA. n i1 0022-1694/99/$ - see front matter ᭧ 1999 Elsevier Science B.V. All rights reserved. PII: S0022-1694(99)00018-9 14 A. Sankarasubramanian, K. Srinivasan / Journal of Hydrology 218 (1999) 13–34 Xn 1 estimated with M1,r,s considering x as linear. In fact, s2 x Ϫ m2; 1b Ϫ i either the quantity M1,0,s (a s: s 0,1,…) or M1,r,0 (b r: n 1 i 1 r 0,1,…) is sufficient to characterise a probability "#distribution. The quantity M which is known as b , 1 n Xn 1,r,0 r g x Ϫ m3 : 1c is more commonly used and can be defined as: 3 Ϫ Ϫ i s n 1 n 2 i 1 b r r E x F x 3 It is to be noted that Eq. 1(c) yields unbiased esti- b mate of skewness, only if the distribution is normal. The unbiased sample estimator (br)of r can be The attempts made to develop unbiased estimates of estimated using the expression given by Landwehr skewness for various non-normal distributions usually et al. (1979): result in a considerable increase in the variance of ! Xn Ϫ skewness (Vogel and Fennessey, 1993). Moreover, a i 1 x i notable drawback with conventional moment ratios 1 r b i 1 ! r 0; 1; …; n Ϫ 1 4 such as skewness and coefficient of variation, is that, r n n Ϫ 1 for finite samples, they are bounded. These bounds are shown to be dependent only on the sample size, irre- r spective of the population values (Kirby, 1974). where x is an ordered set of observations Wallis et al., 1974 have shown that the sample esti- (i) x Յ x Յ x Յ … Յ x . mates of skewness are highly biased for small 1 2 3 n The first four L-moments are given as: samples. Recently, Vogel and Fennessey (1993) have investigated the sampling properties of conven- l b 1 0 tional moment ratios for highly skewed Lognormal l b Ϫ b (LN-2) and generalised Pareto (GPA) distributions 2 2 1 0 for large samples, in terms of bias and root mean 5 l b Ϫ b ϩ b square error (RMSE) expressed in a relative form. 3 6 2 6 1 0 Their study reveals that sample estimates of coeffi- l b Ϫ b ϩ b Ϫ b cient of variation and skewness are remarkably biased 4 20 3 30 2 12 1 0 even for extremely large samples (n Ͼ 1000) drawn from highly skewed populations. The sample estimates (lr: r 1,2,3,…) of L- moments (l 1, l 2,…, l r) can be obtained by substitut- ing the unbiased sample estimates of b r given by Eq. 2. L-moments (4) into Eq. (5). More recently, Wang (1996) has derived direct esti- Recently, Hosking (1990) has defined L-moments, mators of L-moments by closely following the basic which are analogous to conventional moments, and definition of L-moments which is based on order can be expressed in terms of linear combinations of statistics. This eliminates the need to resort to order statistics. Basically, L-moments are linear func- PWMs, while defining the L-moments. However, tions of probability weighted moments (PWMs). the numerical values of the sample estimates from Greenwood et al. (1979) defined PWMs as the PWM-based indirect estimators and the order p: r Ϫ s statistics based direct estimators are the same. Mp;r;s E x F x 1 F x 2 where F(x) is the cumulative distribution function of x, with p,r,s being real numbers. 3. L-moment ratios This expression for Mp;r;s (Eq. (2)) is useful in char- acterising the statistical properties of an observed data Analogous to the conventional statistical moment ; ; ; ; set. The quantities {Mp;0;0 p 1 2 3 …} represent ratios, the L-moment ratios can be expressed as: the conventional non-central moments of x. But, in t l =l case of PWMs, the moments of a distribution are L-coefficient of variation 2 2 1 6a A. Sankarasubramanian, K. Srinivasan / Journal of Hydrology 218 (1999) 13–34 15 t l =l L-skewness 3 3 2 6b and L-moments can be obtained in terms of expecta- tions of quadratic functions of order statistics, t l =l ! ! L-kurtosis 4 4 2 6c Án Ϫ1 XX j Ϫ 1 Ϫ 2 E Xs:t Xr:t In general, 1ՅrϽsՅt t 1ՅjϽkՅn r Ϫ 1 8 t l =l r 3; 4; … 7 ! ! r r 2 k Ϫ j Ϫ 1 n Ϫ k × x Ϫ x 2; The sample estimates of L-moment ratios Ϫ Ϫ Ϫ k j ; ; ; s r 1 t s (t2 t3 … tr) can be obtained by replacing l ; l ; ; l ( 1 2 … r) by their respective sample estimates in a distribution free manner. But, these results depend ; ; ; l1 l2 … lr in the previous set of Eqs. (6a)–(6c) and on the joint distributions of order statistics, and hence (7). are extremely complex and the algebra of the same is L-moments are capable of characterising a wider not easily tractable. Such exact results are available range of distributions, compared to the conventional only for the uniform and the exponential distributions. moments. A distribution may be specified by its L- In contrast, the asymptotic distribution theory moments, even if some of its conventional moments provides an acceptable alternative to obtain practical, do not exist (Hosking, 1990). For example, in the case but slightly approximate estimates of variances of of the generalised Pareto distribution, the conven- sample PWMs and L-moments. If the underlying tional skewness is undefined beyond a value of 155 distribution has finite variance, then, it is possible to (shape parameter Ϫ 1/3), while the L-skewness can demonstrate asymptotic normality and also calculate be defined, even beyond that value. Further, L- the asymptotic bias and variance of the sample statis- moments are more robust to outliers in data than tics ar, br, lr and tr, using the set of expressions given conventional moments (Vogel and Fennessey, 1993) by Hosking (1986). The asymptotic theory usually and enable more reliable inferences to be made from provides a good approximation to the exact distribu- small samples about an underlying probability distri- tion of sample PWMs and L-moments for samples of bution. The advantages offered by L-moments over size n Ն 50 and in most cases adequate approximation conventional moments in hypothesis testing, bound- even for n 20 (Hosking, 1986). Using asymptotic edness of moment ratios and identification of distribu- theory, Hosking (1986) has derived approximate tions have been discussed in detail by Hosking (1986; analytical forms for the variance of L-moment and 1990).
Details
-
File Typepdf
-
Upload Time-
-
Content LanguagesEnglish
-
Upload UserAnonymous/Not logged-in
-
File Pages22 Page
-
File Size-