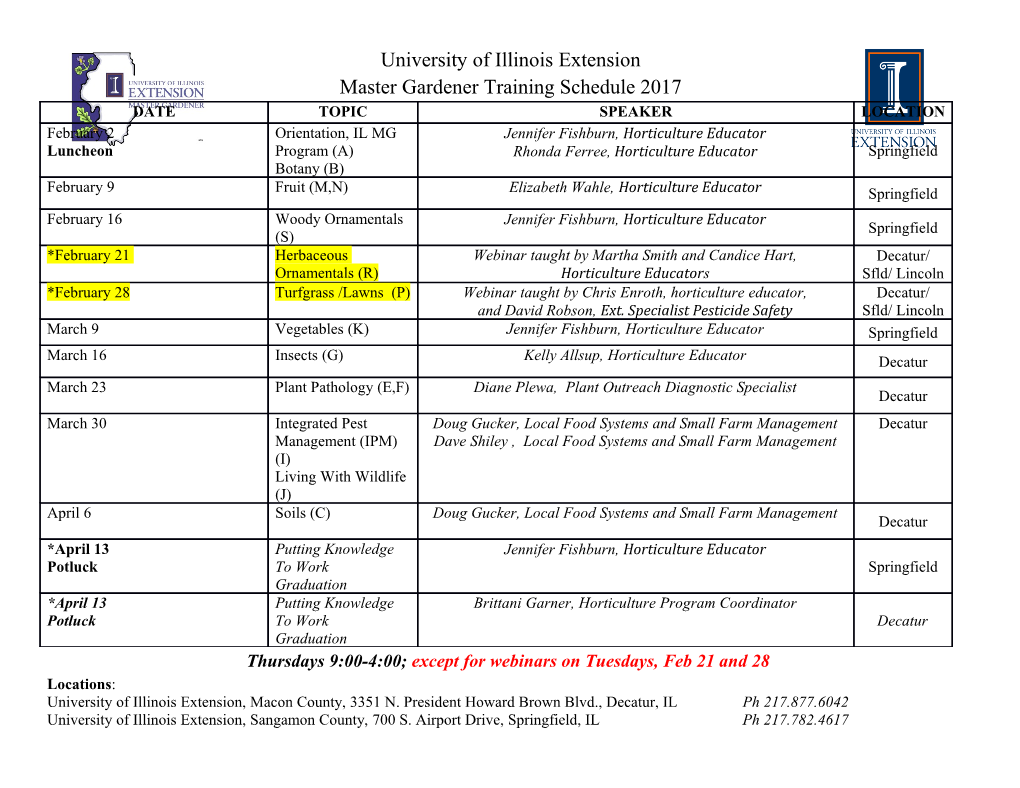
New hardware support in OSIRIS 4.0 1,2 R. A. Fonseca 1 GoLP/IPFN, Instituto Superior Técnico, Lisboa, Portugal 2 DCTI, ISCTE-Instituto Universitário de Lisboa, Portugal Ricardo Fonseca | OSIRIS Workshop 2017 Outline • The road to Exascale Systems – HPC system evolution – Current trends – OSIRIS support for top10 systems • The new Intel Knights Landing architecture – Architecture details – OSIRIS on the KNL • OSIRIS on modern CPUs – Overview of modern CPUs – Compiling and running for these • OSIRIS on GPUs 2 Ricardo Fonseca | OSIRIS Workshop 2017 The road to Exascale systems UNIVAC 1 - 1951 Internal view The Road to Exascale Computing High Performance Computing Power Evolution Exaflop/s 1012 Sunway Taihulight NRCPC, China Sunway TaihuLight #1 - TOP500 Jun/16 Tianhe-2 K Computer Titan Petaflop/s 9 Cray XT5-HE Tianhe-1A 10 IBM Blue Gene/L IBM RoadRunner IBM Blue Gene/L SGI Project Columbia NEC Earth Simulator IBM ASCI White 6 Teraflop/s Intel ASCI Red/9152 Intel ASCI Red/9632 10 - Fujitsu Numerical Hitachi/Tsukuba CP-PACS/2048 Wind Tunnel Intel Paragon XP/S 140 NEC SX-3/44R Cray-2/8 ETA10-G/8 Gigaflop/s M-13 103 Cray X-MP/4 Cray-1 CDC Cyber 205 CDC STAR-100 Burroughs ILLIAC IV Performance [ MFLOPs ] CDC 7600 CDC 6600 Megaflop/s 100 Sunway Taihulight Total system IBM 7030 Stretch UNIVAC LARC IBM 7090 • 40 960 compute nodes • 10 649 600 cores Node Configuration • 1.31 PB RAM IBM 709 IBM 704 kiloflop/s • 1× SW26010 manycore processor Performance 10-3 UNIVAC 1 • Rpeak 125.4 Pflop/s EDSAC 1 • 4×(64+1) cores @ 1.45 GHz • 4× 8 GB DDR3 • Rmax 93.0 Pflop/s 1940 1950 1960 1970 1980 1990 2000 2010 2020 Year (data from multiple sources) 4 Ricardo Fonseca | OSIRIS Workshop 2017 The Road to Power Efcient Computing Energy Requirement Evolution kiloJoule 6 10 Sunway Taihulight UNIVAC 1 NRCPC, China #1 - TOP500 Jun/16 IBM 704 Joule 103 IBM 7090 IBM 7030 Stretch CDC 6600 ] J m [ CDC 7600 miliJoule n o i 0 t 10 a Cray-1 r e p o Cray-2/8 r e Fujitsu Numerical p microJoule Wind Tunnel y g -3 r 10 e Intel ASCI Red/9152 Intel ASCI Red/9632 n IBM ASCI White E NEC Earth Simulator Sunway Taihulight IBM Blue Gene/L IBM Blue Gene/L IBM RoadRunner • Manycore architecture nanoJoule Cray XT5-HE 10-6 Tianhe-1A K Computer Titan Tianhe-2 • Peak performance 93 PFlop/s Sunway TaihuLight • Total power 15.3 MW picoJoule • 6.07 Gflop/W 10-9 • 165 pJ / flop 1940 1950 1960 1970 1980 1990 2000 2010 2020 Year (data from multiple sources) 5 Ricardo Fonseca | OSIRIS Workshop 2017 Petaflop systems firmly established Multicore systems • Maintain complex cores and replicate The drive towards Exaflop • 2 systems in the top 10 are based on multicore CPUs • Steady progress for over 60 years • 1× Fujitsu SPARK #8 (K-Computer) – 138 systems above 1 PFlop/s • 1× Intel Xeon E5 #10 (Trinity) • Supported by many computing paradigm Manicore evolutions • Use many lower power cores • Trend indicates Exaflop systems by next decade • 5 systems in the top 10 • Electric power is one of the limiting factors • #1 (Sunway Taihulight) – Target < 20 MW • 2× IBM BlueGene/Q in the top – Top system achieves ~ 6 Gflop/W • #5 (Sequoia) and #9 (Mira) • ~ 0.2 GW for 1 Exaflop • Seem to be the last of their kind • 2× Intel Knights Landing systems • Factor of 10× improvement still required • #6 (Cori) and #7 (Oakforest-PACS) – Best energy efciency • 14 Gflop/W Accelerator/co-processor technology • NVIDIA Tesla P100 • 3 systems in top 10 • #3 (Piz Daint) and #4 (Titan) use NVIDIA GPUs • #2 (Tianhe-2) uses Intel Knights Corner 6 Ricardo Fonseca | OSIRIS Workshop 2017 Which top 10 systems are currently supported by OSIRIS? Rank System Cores Rmax [TFlop/s] Rpeak [TFlop/s] Power [kW] OSIRIS support 1 Sunway TaihuLight,China 10649600 93014.6 125435.9 15371 No 2 Tianhe-2 (MilkyWay-2), China 3120000 33862.7 54902.4 17808 Full (KNC) 3 Piz Daint, Switzerland 361760 19590.0 25326.3 2272 Full (CUDA) 4 Titan, United States 560640 17590.0 27112.5 8209 Full (CUDA) 5 Sequoia, United States 1572864 17173.2 20132.7 7890 Full (QPX) 6 Cori, United States 622336 14014.7 27880.7 3939 Full (KNL) 7 Oakforest-PACS, Japan 556104 13554.6 24913.5 2719 Full (KNL) 8 K computer, Japan 705024 10510.0 11280.4 12660 Standard (Fortran) 9 Mira, United States 786432 8586.6 10066.3 3945 Full (QPX) 10 Trinity, United States 301056 8100.9 11078.9 4233 Full (AVX2) 7 Ricardo Fonseca | OSIRIS Workshop 2017 Intel Knights Landing Architecture Intel Xeon Phi 5110p die Intel Knights Landing Architecture NERSC Cori Intel Knights Landing (KNL) • Processing • 68 x86_64 cores @ 1.4 GHz • 4 threads / core • Scalar unit + 2× 512 bit vector unit • 32KB L1-I + 32KB L1-D cache • Organized in 36 tiles • Connected by 2D Mesh • 1 MB L2 cache • 2 cores/tile • Fully Intel Xeon ISA-compatible NERSC Cori • Binary compatible with other x86 code Cray XC40 • No L3 cache (MCDRAM can be configured as an L3 cache) #5 - TOP500 Nov/16 • Memory • Cray XC40 • 16 GB 8-channel 3D MCDRAM • 9152 Compute Nodes • 400+ GB/s • Total system • 3.9 MW • (Up to) 384 GB 6-channel DDR4 • 622 336 cores • Interconnect • 115.2 GB/s • 0.15 PB (MCDRAM) + 0.88 PB (RAM) • Aries Interconnect • 8×2 memory controllers • Performance • Node configuration • Up to 350 GB/s • RMAX = 14.0 PFlop/s • 1× Intel® Xeon® Phi 7250 @ • System Interface • RPEAK = 27.9 PFlop/s 1.4GHz (68 cores) • Standalone host processor • 16 GB (MCDRAM) + 96 GB (RAM) • Max power consumption 215W • 9 Ricardo Fonseca | OSIRIS Workshop 2017 KNL Memory Architecture Access Modes Clustering Modes • All2All • Cache mode • No afnity between tile, directory 16 GB and memory DDR • SW-Transparent MOCPDIORAM MOPCIDORAM PCIe MOCPDIORAM MOCPIDORAM MCDRAM • Most general, usually lower perf. • Covers whole DDR range EDC EDC IIO EDC EDC than other modes Tile Tile Tile Tile • Quadrant Tile Tile Tile Tile Tile Tile • Chip divided into 4 virtual quadrants Tile Tile Tile Tile Tile Tile • Afnity between directory and iMC Tile Tile Tile Tile iMC 16 GB memory, Lower latency and better DDR DDR MCDRAM bandwidth than All2All, SW- Tile Tile Tile Tile Tile Tile • Flat mode transparent • MCDRAM as regular memory Tile Tile Tile Tile Tile Tile • SW-Managed • Sub-NUMA clustering Tile Tile Tile Tile Tile Tile DDR • Same address space • Looks like 4-socket Xeon EDC EDC Misc EDC EDC Physical address • Afnity between tile, directory and memory, Lowest latency, requires MOCPDIORAM MOCPDIORAM MOCPDIORAM MOCPIDORAM NUMA optimize to get benefit KNL Mesh Interconnect • Also a hybrid Mode • Memory access / cluster modes must be configured at boot time • Use MCDRAM as part memory part cache • 25% or 50% as cache 10 Ricardo Fonseca | OSIRIS Workshop 2017 Logical view of a KNL node Intel KNL systems Logical View • The KNL node is simply a 68 core (4 threads per core) shared memory computer OpenMP region • Each core has dual 512 but VPUs OpenMP region N nodes core core core OpenMP region core core core OpenMP region • This can be viewed as small computer cluster with N SMP core corecore corecore core OpenMP regionM cores nodes with M cores per node core corecore corecore core OpenMP regionM cores core core core • Use a distributed memory algorithm (MPI) for core corecore corecore core M cores core core core parallelizing across nodes core corecore corecore Sharedcore memory M cores core core core • Use a shared memory algorithm (OpenMP) for core core Sharedcore memory M cores core core core parallelizing inside each node core core Sharedcore memory M cores core core core • The exact same code used in "standard" HPC systems can Shared memory core core core run on the KNL nodes Shared memory Shared memory • The vector units play a key role in system performance Intel KNL node • Vectorization for this architecture must be considered 11 Ricardo Fonseca | OSIRIS Workshop 2017 Parallelization for a KNL node Distributed Memory (MPI) • HPC systems present a hierarchy of distributed / shared memory parallelism • Top level is a network of computing nodes • Nodes exchange information through network messages Sim. Volume Parallel Domain • Each computing node is a set of CPUs / cores • Memory can be shared inside the node Shared Memory (OpenMP) • Parallelization of PIC algorithm Particle Bufer • Spatial decomposition across nodes: each node handles a specific region of simulation space • Split particles over cores inside node • Same as for standard CPU systems Thread 1 Thread 2 Thread 3 SMP node 12 Ricardo Fonseca | OSIRIS Workshop 2017 Vectorization of the PIC algorithm for KNL Particle Push Current Deposition Load nV virtual part. into Vector Load nV particles into Vector • The code spends ~80-90% time advancing Unit Unit particles and depositing current • Focus vectorization efort here Vectorized by particle Interpolate Fields Interpolate Fields InterpolateInterpolate Fields Fields Interpolate Fields • Vectors contain same Interpolate Fields InterpolateCalculate Currents Fields quantity for all particles • Previous strategy developed for vectorization works well Push Particles • Process nv (16) particles at a time for most of Push Particles PushPush Particles Particles Push Particles Transpose vectors the algorithm Push Particles TransposePush Particlescurrent to get 1 • Done through vector vector per current line operations • For current deposition vectorize by current line to avoid serialization Split Path / Create virtual particles • Critical because of large vector width Vectorized by current line Push Particles Push Particles • Use a vector transpose operation LoopPush over Particles vectors and • Vectors contain 1 line of accumulate current current for 1 particle Store Results 13 Ricardo Fonseca
Details
-
File Typepdf
-
Upload Time-
-
Content LanguagesEnglish
-
Upload UserAnonymous/Not logged-in
-
File Pages26 Page
-
File Size-