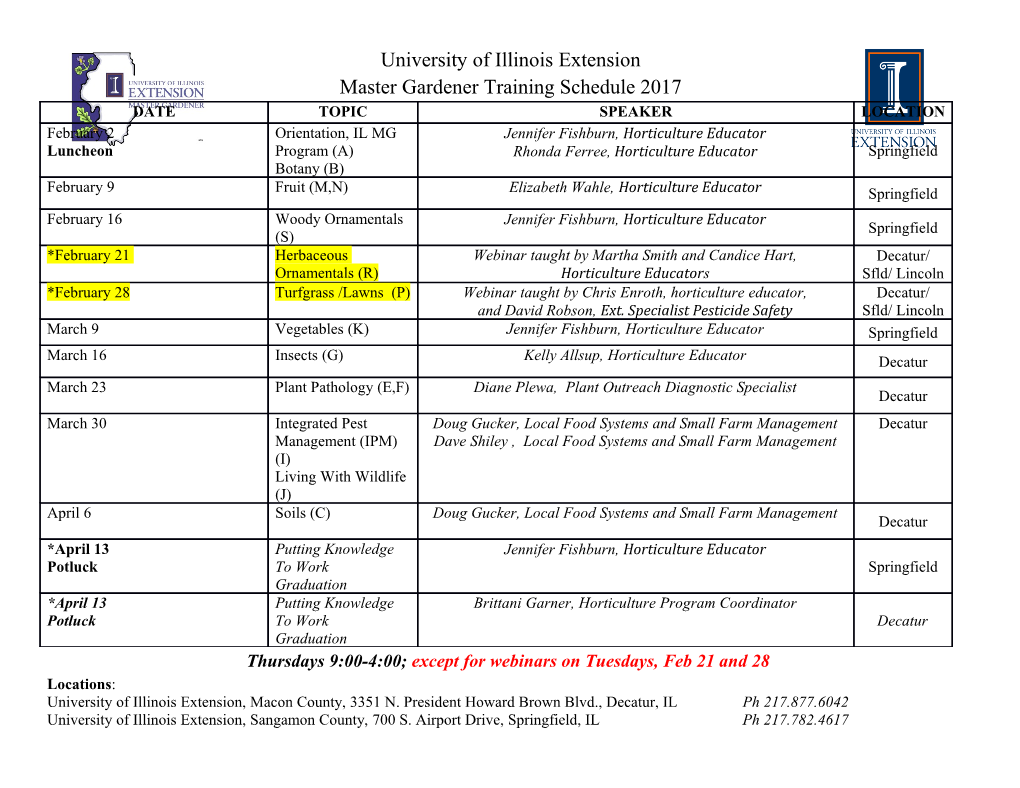
Post Hoc Power: Tables and Commentary Russell V. Lenth July, 2007 The University of Iowa Department of Statistics and Actuarial Science Technical Report No. 378 Abstract Post hoc power is the retrospective power of an observed effect based on the sample size and parameter estimates derived from a given data set. Many scientists recommend using post hoc power as a follow-up analysis, especially if a finding is nonsignificant. This article presents tables of post hoc power for common t and F tests. These tables make it explicitly clear that for a given significance level, post hoc power depends only on the P value and the degrees of freedom. It is hoped that this article will lead to greater understanding of what post hoc power is—and is not. We also present a “grand unified formula” for post hoc power based on a reformulation of the problem, and a discussion of alternative views. Key words: Post hoc power, Observed power, P value, Grand unified formula 1 Introduction Power analysis has received an increasing amount of attention in the social-science literature (e.g., Cohen, 1988; Bausell and Li, 2002; Murphy and Myors, 2004). Used prospectively, it is used to determine an adequate sample size for a planned study (see, for example, Kraemer and Thiemann, 1987); for a stated effect size and significance level for a statistical test, one finds the sample size for which the power of the test will achieve a specified value. Many studies are not planned with such a prospective power calculation, however; and there is substantial evidence (e.g., Mone et al., 1996; Maxwell, 2004) that many published studies in the social sciences are under-powered. Perhaps in response to this, some researchers (e.g., Fagley, 1985; Hallahan and Rosenthal, 1996; Onwuegbuzie and Leech, 2004) recommend that power be computed retrospectively. There are differing approaches to retrospective power, but the one of interest in this article is a power calculation based on the observed value of the effect size, as well as other auxiliary quantities such as the error standard deviation, while the significance level of the test is held at a specified value. We will refer to such power calculations as “post hoc power” (PHP). Advocates of PHP recommend its use especially when a statistically nonsignificant result is obtained. The thinking here is that such a lack of significance could be due either to low power or to a truly small effect; if the post hoc power is found to be high, then the argument is made that the nonsignificance must then be due to a small effect size. There is substantial literature, much of it outside of the social sciences (e.g., Goodman and Berlin, 1994; Zumbo and Hubley, 1998; Levine and Ensom, 2001; Hoenig and Heisey, 2001), that takes an opposing view to PHP practices. Lenth (2001) points out that PHP is simply a function of the P value of the test, and thus adds no new information. Yuan and Maxwell (2005) show that PHP does not necessarily provide an accurate estimate of true power. Hoenig and Heisey (2001) discuss several misconceptions connected with retrospective power. Among other things, they demonstrate that when a test is nonsignificant, then the higher the PHP, the more evidence there is against the null hypothesis. They also point out that, in lieu of PHP, a correct and effective way to establish that an effect is small is to use an equivalence test (Schuirmann, 1987). In this article, we derive and present new tables that directly give exact PHP for all standard scenarios involving t tests (Section 2) and F tests (Section 3). (The PHP of certain z tests and c2 tests can also be obtained as limiting cases.) All that is needed to obtain PHP in these settings is the significance level, the P value of the test, and the degrees of freedom. If one desires a PHP calculation, this is obviously a convenient resource for obtaining exact power with very little effort; however, the broader goal is to demonstrate explicitly what PHP is, and what it is not. In Section 4, we present a slight reformulation of the PHP problem that leads to a “grand unified formula” for post hoc power that is universal to all tests and is a simple head calculation. The results are discussed in Section 5, along with possible alternative practices regarding retrospective power. 2 t tests Table 1 may be used to obtain the post hoc power (PHP) for most common one-and two-tailed t tests, when the significance level is a = .05. The only required information (beyond a) is the P value of the test and the degrees of freedom. Computational details are provided later in this section; for now, here is an illustration based on an example in Hallahan and Rosenthal (1996). They discuss the results of a hypothetical study where a new treatment is tested to see if it improves cognitive functioning of stroke victims. There are 20 patients in the control group and 20 in the treatment group, and the observed difference between the groups is .4 standard deviations—somewhat short of a “medium” effect on the scale proposed by Cohen (1988)—with a P value of .225 (two-sample pooled t test, two-tailed). In this case, we have n = 38 degrees of freedom. Referring to the bottom half of Table 1 (for 2-sided tests) and linearly interpolating, we obtain a post hoc power of about .234 (the exact value, using the algorithm used to produce Table 1, is .2251.) This agrees with the value of .23 reported in the article. We briefly discuss some patterns in these tables. First, PHP is a decreasing function of 2 Table 1: Post hoc power of a t test when the significance level is a = .05. It depends on the P value, the degrees of freedom n, and whether it is one- or two-tailed. Post hoc power of a z test may be obtained using the entries for n = ¥. P value of test Alternative n 0.001 0.01 0.05 0.1 0.25 0.5 0.75 One-tailed 1 1.0000 1.0000 0.6767 0.3698 0.1348 0.0500 0.0105 2 1.0000 0.9910 0.5996 0.3571 0.1434 0.0500 0.0118 5 0.9995 0.8899 0.5365 0.3565 0.1557 0.0500 0.0112 10 0.9860 0.8225 0.5174 0.3573 0.1607 0.0500 0.0107 20 0.9627 0.7870 0.5084 0.3578 0.1633 0.0500 0.0105 50 0.9420 0.7660 0.5033 0.3580 0.1649 0.0500 0.0103 200 0.9300 0.7556 0.5008 0.3582 0.1657 0.0500 0.0102 1000 0.9267 0.7529 0.5002 0.3582 0.1659 0.0500 0.0102 ¥ 0.9258 0.7522 0.5000 0.3582 0.1659 0.0500 0.0102 Two-tailed 1 1.0000 1.0000 0.6812 0.3797 0.1506 0.0730 0.0542 2 1.0000 0.9922 0.6147 0.3731 0.1619 0.0804 0.0562 5 0.9996 0.8919 0.5446 0.3727 0.1864 0.0918 0.0589 10 0.9844 0.8145 0.5210 0.3744 0.1978 0.0973 0.0602 20 0.9553 0.7723 0.5102 0.3754 0.2038 0.1003 0.0609 50 0.9290 0.7473 0.5040 0.3761 0.2075 0.1022 0.0614 200 0.9137 0.7351 0.5010 0.3764 0.2094 0.1032 0.0616 1000 0.9094 0.7318 0.5002 0.3765 0.2099 0.1035 0.0617 ¥ 0.9083 0.7310 0.5000 0.3765 0.2100 0.1035 0.0617 P value, for any number of degrees of freedom and alternative. In general, except for very small degrees of freedom, the power of a marginally significant test (P = a = .05) is around one half, with the two-tailed powers generally higher than the one-tailed results. If the test is significant, the power is higher than .5; and when the test is nonsignificant, the power is usually less than .5. Thus, it is an empty question whether the PHP is high when significance is not achieved. 2.1 Derivation of the tables Consider the null hypothesis H0 : q = q0, where q is some parameter and q0 is a specified null value (often zero). We have available an estimator qˆ, and the t statistic has the form qˆ − q t = 0 (1) se(qˆ) where se(qˆ) is an estimate of the standard error of qˆ when H0 is true. Assume that: 1. For all q, qˆ is normally distributed with mean q; its standard deviation will be denoted t. 3 2. For all q, n · se(qˆ)2/t2 has a c2 distribution with n degrees of freedom. The value of n is known. 3. qˆ and se(qˆ) are independent. These conditions hold for most common t-test settings, such as a one-sample test of a mean, pooled or paired comparisons of two means, and tests of regression coefficients under standard homogeneity assumptions. Let us re-write (1) in the form [(qˆ − q)/t] + [(q − q )/t] Z + d t = 0 = p (2) se(qˆ)/t Q/n where d = (q − q0)/t. According to the stated assumptions, Z and Q are independent, Z is standard normal, and Q is c2 with n degrees of freedom. This characterizes the noncentral t distribution with n degrees of freedom and noncentrality parameter d. (See, for example, Hogg et al., 2005, page 442).
Details
-
File Typepdf
-
Upload Time-
-
Content LanguagesEnglish
-
Upload UserAnonymous/Not logged-in
-
File Pages13 Page
-
File Size-