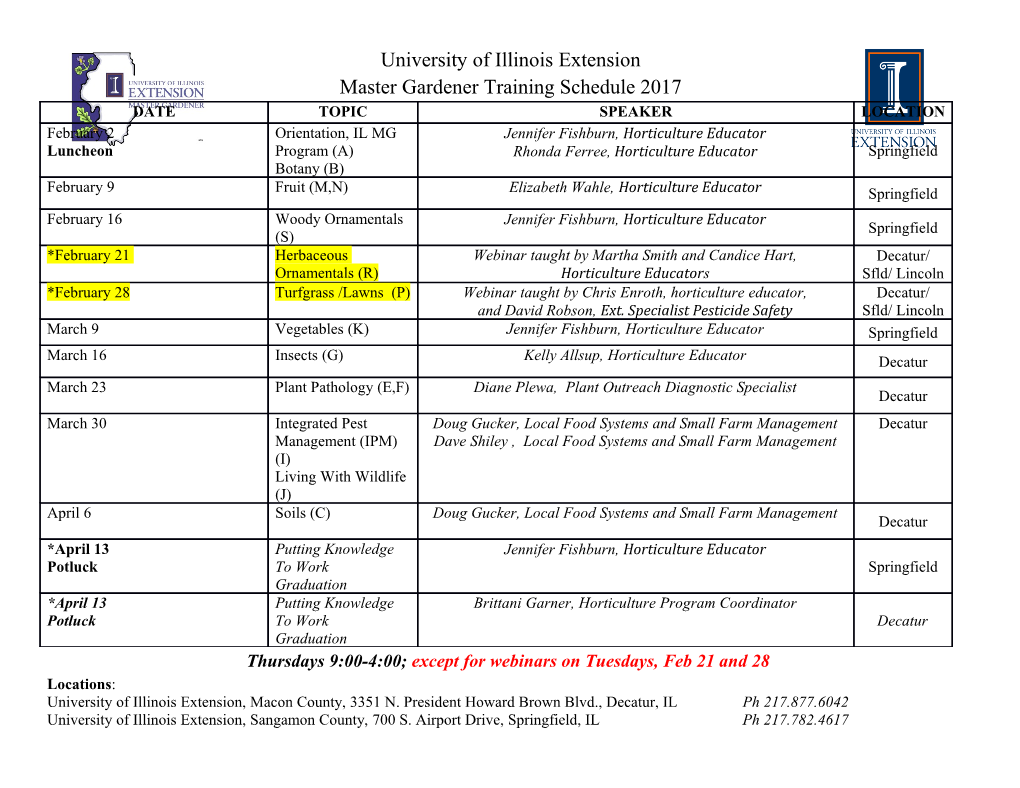
Computation of scalar accuracy metrics LE, CE, and SE as both predictive and sample- based statistics John Dolloff [C] and Jacqueline Carr [C] National Geospatial-Intelligence Agency (NGA) 7500 GEOINT Drive, Springfield, VA Government POC: Christopher O’Neill ([email protected]); PA case #: 16-277 ABSTRACT Scalar accuracy metrics often used to describe geospatial data quality are Linear Error (LE), Circular Error (CE), and Spherical Error (SE), which correspond to vertical, 2d horizontal, and 3d radial errors, respectively, in a local tangent plane coordinate system (e.g. East-North-Up). In addition, they also correspond to specifiable levels of probability; for example, CE_50 is Circular Error probable, or CE at the 50% probability level. Typical probability levels of interest are 50, 90, and 95%. Scalar accuracy metrics are very important for both near-real time accuracy predictions corresponding to derived location information, and for post-analysis verification and validation of system performance (location accuracy) requirements. The former normally correspond to predictive scalar accuracy metrics and the latter usually correspond to sample-based scalar accuracy metrics. Predictive metrics are generated from a priori error covariance matrices and mean-values, if non-zero, corresponding to the underlying (up to 3) error components. Sample-based metrics are generated from independent and identically distributed (i.i.d.) samples of error relative to “ground truth”. This paper details the proper but practical computation of both types of scalar accuracy metrics, including corresponding confidence intervals – techniques with non-trivial computational approximation errors are not included nor needed. Recommended sample-based metrics utilize order statistics which require no assumptions regarding the underlying probability distributions of errors. Keywords: Linear Error (LE), Circular Error (CE), Spherical Error (SE), probability, predictive statistics, sample statistics, order statistics 1.0 Introduction Scalar accuracy metrics are commonly used to express the accuracy or corresponding error in an estimate of geospatial location in a local tangent plane coordinate system (e.g. East-North-Up) in a way that is easy to visualize, comprehend, and communicate. Common scalar accuracy metrics used include Linear Error (LE) for describing vertical or elevation data accuracy and Circular Error (CE) for describing two dimensional horizontal data accuracy. Accuracy of geospatial location in three dimensions is often described using either a combination of the LE and CE scalar metrics into a representative CE-LE cylinder or by the single metric representation of Spherical Error (SE) providing a radial representation of accuracy in three dimensions. Representing accuracy through the use of a single number, or even pair of numbers, has many advantages as it provides simplified and intuitive representations, a clear means of comparison, and minimal bandwidth requirements. This simplification of sometimes complex information, however, is not without risk. There is not clear guidance or broad agreement on the best practices for calculation of these commonly desired metrics which results in representations which may not be reliably compared, such as to a desired requirement. Also, common computations of predictive statistics are typically excessive in their approximations. Similarly, computations based on sample-based statistics may rely on incorrect assumptions of error distribution and subsequently incorrectly reflect system or process performance. This paper considers these challenges in scalar accuracy metric representation and provides guidance to fast, rigorous, and accurate methods to robustly calculate these representative values. 2.0 Defining Scalar Accuracy Metrics LE, CE, and SE To clarify the discussion presented in this paper, it is important to set the stage with explicit descriptions of the popular scalar accuracy metrics, LE, CE, and SE. Linear Error (LE) - LE corresponds to the length of a vertical line (segment) such that there is a 90% probability that the absolute value of vertical error resides along the line. If the line is doubled in length and centered at the position solution, there is a 90% probability the true position vertical location resides along the line. LE_XX corresponds to LE at the XX % probability level. See Figure 2.0-1. 1 Circular Error (CE) - CE corresponds to the radius of a circle such that there is a 90% probability that the horizontal error resides within the circle, or equivalently, if the circle is centered at the position solution, there is a 90% probability the true position horizontal location resides within the circle. CE_XX corresponds to CE at the XX % probability level. See Figure 2.0-1. Spherical Error (SE) - SE corresponds to the radius of a 3D sphere such that there is a 90% probability that 3d error resides within, or equivalently, if the sphere is centered at the position solution, there is a 90% probability the true position location resides within the sphere. SE_XX corresponds to SE at the XX % probability level. Figure 2.0-1: Graphic depiction of CE (left) and LE (right) for examples where CE = 4m and LE = 5m For the above scalar accuracy metrics: It is assumed that the underlying x-y-z coordinate system is a local tangent plane system, i.e., x and y are horizontal components and z the vertical component. CE-LE corresponds to the CE-LE error cylinder. There is a probability between 81 to 90 percent that 3d radial error resides within the cylinder. The former value corresponds to uncorrelated horizontal and vertical errors, the latter value to highly correlated horizontal and vertical errors. See Figure 2.0-2. Figure 2.0-2: Example of CE-LE cylinder compared to corresponding error ellipsoid based on full covariance matrix References for the definitions of scalar accuracy metrics include [6-7]; an application of scalar accuracy metrics which also discusses error ellipsoids is [5]. 2 As important means of conveying both near-real time accuracy predictions corresponding to derived location information, and for post-analysis verification and validation of system performance (location accuracy) requirements, scalar accuracy metrics are developed based on both predictive statistics and sample statistics. Predictive statistics correspond to the mathematical modeling of assumed a priori error characteristics and are part of a statistical error model. Predictive scalar accuracy metrics are generated from a priori error covariance matrices and mean-values, if non-zero, corresponding to the underlying (up to 3) error components. Sample statistics correspond to the analysis of a collection of error samples. Sample-based scalar accuracy metrics are generated from independent and identically distributed (i.i.d.) samples of error relative to “ground truth”. The following sections of this paper detail the proper but practical computation of both types of scalar accuracy metrics, including corresponding confidence intervals – techniques with non-trivial computational approximation errors are not included nor needed with the availability of today’s computer systems. Sample-based techniques include the recommended use of order statistics which require no assumptions regarding the underlying probability distributions of errors. 3.0 Scalar Accuracy Metrics: Computations based on Predictive Statistics This section of the document presents the evaluation of the scalar accuracy metrics based on the predictive statistics: (1) 푛푥1 mean-value 휖푋̅̅̅̅, and (2) 푛푥푛 error covariance matrix 퐶푋; where 푛 = 1 for the computation of LE_XX, 푛 = 2 for the computation of CE_XX, and 푛 = 3 for the computation of SE_XX. The mean-value is typically zero for predictive statistics, although a non-zero mean-value is also accounted for in the following. A (multi-variate) Gaussian (normal) distribution is also assumed for the underlying random variables corresponding to location error (휖푋 = [휖푥 휖푦 휖푧]푇). This is necessary in order to assign probabilities; e.g., CE_90 corresponds to 90% probability. Note that radial error 휖푟 is defined as follows: 휖푟 = |휖푧|, 휖푟 = √휖푥2 + 휖푦2, ,휖푟 = √휖푥2 + 휖푦2 + 휖푧2, for LE, CE, and SE, respectively. (Note that confidence intervals are not computed for predictive-based scalar accuracy metrics as the underlying mean-value and error covariance matrix are assumed a “given”.) 3.1 LE LE_XX is defined as that line length L such that: 1 2 2 −1/2((푧−푧̅) /휎푧 ) 푝 = 1/2 ∫ 푒 푑푧, (3.1-1) (2휋) 휎푧 integrated over the region √푧2 ≤ 퐿, and where probability 푝 = 푋푋/100, 1d error 휖푋 = 휖푧 is defined as 푧 for notational convenience, with mean-value 휖푋̅̅̅̅ defined as 푧̅, and 1 × 1 error covariance matrix 퐶푋 about the mean 2 defined as 휎푧 . Note that if the mean-value is not zero, the length 퐿 is still relative to the origin per the standard definition of LE_XX. ∗ If we assume that the mean-value of error is zero, and change variables such that 푧/(휎푧√2) → 푧 , Equation 3.1-1 can be rewritten as: ∗ ∗ 2휎푧√2 퐿 −푧∗2 ∗ 2 퐿 −푧∗2 ∗ ∗ 푝 = 1/2 ∫ 푒 푑푧 = 1/2 ∫ 푒 푑푧 ≡ erf (푧 ∗), where 퐿 = 퐿/(휎푧√2). (3.1-2) (2휋) 휎푧 0 (휋) 0 Thus, since erf (Error Function) is a well-tabulated function and its inverse available via MATLAB and other programming languages, we have by definition, erf 푖푛푣(푝) = erf 푖푛푣(푋푋/100) = 퐿∗; thus, and accounting for the change of variables: 푋푋 퐿퐸_푋푋 = 휎 √2 × 푒푟푓푖푛푣( ), and specifically, (3.1-3) 푧 100 퐿퐸_푋푋 = 퐿(푝)휎푧, where 푝 = 푋푋/100 and the multiplier L(p) is listed in Table 5.1-1: (3.1-4) 3 Table 3.1-1: Linear Error (LE) multiplier L(p) versus probability level p Probabilities p=0.5 p=0.6827 p=0.90 p=0.95 p=9545 p=0.99 p=0.9973 p=0.999 L(p) 0.6745 1.0000 1.6499 1.9600 2.0000 2.5758 3.0000 3.2905 The light blue entries include the standard probability levels of interest. Thus, for example, L(p)=1.6499 is applicable for LE_90.
Details
-
File Typepdf
-
Upload Time-
-
Content LanguagesEnglish
-
Upload UserAnonymous/Not logged-in
-
File Pages15 Page
-
File Size-