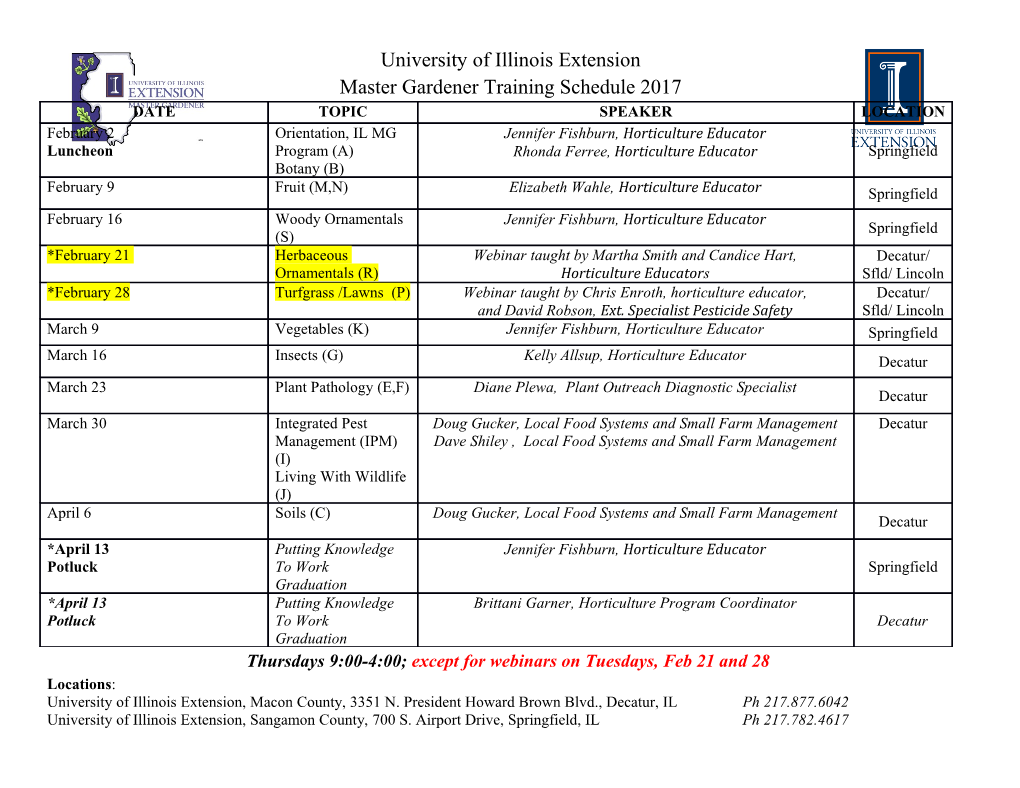
Empirical Processes with Applications in Statistics 3. Glivenko–Cantelli and Donsker Theorems Johan Segers UCLouvain (Belgium) Conférence Universitaire de Suisse Occidentale Programme Doctoral en Statistique et Probabilités Appliquées Les Diablerets, February 4–5, 2020 1 / 54 Set-up I i.i.d. random variables X1; X2;::: on some probability space Ω taking values in some measurable space (X; A) with common law P P(Xi 2 B) = P(B); 8B 2 A I Family F ⊂ L1(P) of P-integrable functions f : X! R I Empirical and population measures: for f 2 F , 1 Xn P f = f(X ) n n i i=1 Z Pf = f(x) dP(x) = E[f(Xi)] X I Empirical process: p Gn = n (Pn − P) 2 / 54 X e t z a à à I M 3 / 54 Bounded stochastic processes Assume that the following map is bounded almost surely: F! R : f 7! Pnf − Pf Then we can view Pn − P and Gn as (possibly non-measurable) maps Ω ! `1(F ) I Assume that supf2F jf(x)j < 1 for P-almost every x 2 X and supf2F jPfj < 1 I Often, we will assume that F has a P-integrable envelope F : X! [0; 1), i.e., 8x 2 X; 8f 2 F ; jf(x)j 6 F(x) 4 / 54 a q s f a o X z 5 / 54 Uniform law of large numbers: Glivenko–Cantelli Strong law of large numbers. For all f 2 F , Pnf ! Pf a.s.; n ! 1 Trivially, for every f1;:::; fk 2 F , max jPnf − Pfj ! 0 a.s.; n ! 1 j=1;:::;k Can we make this statement uniform in F ? Definition: Glivenko–Cantelli. F is a P-Glivenko–Cantelli class if as∗ sup jPnf − Pfj ! 0; n ! 1 f2F 6 / 54 Uniform central limit theorem: Donsker Multivariate central limit theorem Finite-dimensional distributions of Gn converge to those of a centered Gaussian process f 7! Gf on F with covariance function E[Gf1 Gf2] = Pf1f2 − Pf1 Pf2 = cov(f1(X); f2(X)) for f1; f2 2 F , where X ∼ P. Can we make this statement uniform in f 2 F ? Definition: Donsker class. 1 F is a P-Donsker class if Gn G as n ! 1 in ` (F ). To show: asymptotic tightness 7 / 54 A counterexample Let X = [0; 1] with the Borel σ-field and P the uniform distribution. Consider F = all continuous functions f : X! [0; 1] For any x1;:::; xn 2 X and any " > 0, we can find f 2 F such that I f(x1) = ::: = f(xn) = 1 R 1 I Pf = f(x) dx ". 0 6 As a consequence, sup jPnf − Pfj = 1 f2F The class F is therefore not P-Glivenko–Cantelli nor P-Donsker. By the Stone–Weierstrass theorem, the example extends to the subfamily F0 = fall polynomials f : X! [0; 1] with rational coefficientsg See lecture 1. 8 / 54 Complexity of a class of functions In general, how to put conditions on F limiting its complexity? The previous counterexample shows that it is not sufficient to impose that I functions f in F are bounded I functions f in F are smooth I the cardinality of F is not too large I ... Two techniques: controlling the I bracketing numbers I covering numbers These will provide sufficient but not necessary conditions Main sources for this lecture: van der Vaart and Wellner (1996), van der Vaart (1998) 9 / 54 Glivenko–Cantelli and Donsker Theorems Bracketing entropy Uniform entropy VC-classes Extension: Changing function classes 10 / 54 Definition: Bracketing numbers. I Bracket induced by functions l; u : X! R: [l; u] = ff : X! R j 8x 2 X : l(x) 6 f(x) 6 u(x)g An "-bracket [l; u]: if ku − lk 6 ", with k · k some norm Norm is usually L1(P) or L2(P) I Bracketing number: N[]("; F ; k · k) = the minimum number, N, of "-brackets needed to cover F , i.e., SN F ⊂ i=1[li; ui] and kui − lik 6 " for all i li and ui need not belong to F , but kuik and klik need to be finite. I Entropy with bracketing: log N[]("; F ; k · k) 11 / 54 D as t X 12 / 54 D ce - si T 13 / 54 Glivenko–Cantelli with bracketing I i.i.d. random elements X1; X2;::: in (X; A) with common law P I Family F ⊂ L1(P) of P-integrable functions f : X! R I Law of large numbers: 8f 2 F ; Pnf ! Pf a.s.; n ! 1 I Uniformly in f 2 F ? Glivenko–Cantelli theorem: bracketing. If 8" > 0; N[]("; F ; L1(P)) < 1 then F is P-Glivenko–Cantelli, i.e., as∗ sup jPnf − Pfj ! 0; n ! 1: f2F 14 / 54 Proof. Fix " > 0. SN I Consider a covering F ⊂ i=1[li; ui] ⊂ L1(P) with "-brackets: 8i = 1;:::; N; 0 6 P(ui − li) 6 " I For every f 2 F , find i 2 f1;:::; Ng such that f 2 [li; ui] and then Pnli 6 Pnf 6 Pnui Pli 6 Pf 6 Pui 6 Pli + " I Bound the supremum over f 2 F by a maximum over i = 1;:::; N: sup jPnf − Pfj 6 max jPnli − Plij _ jPnui − Puij + " f2F i=1;:::;N I Apply the law of large numbers to l1;:::; lN and u1;:::; uN. Let " # 0. 15 / 54 Donsker theorem with bracketing p 1 Recall Gn = n (Pn − P) seen as map Ω ! ` (F ), for F ⊂ L2(P) Donsker theorem: bracketing. If for some (and then for all) δ > 0 we have a finite bracketing integral Z δ q J[](δ; F ; L2(P)) := log N[]("; F ; L2(P)) d" < 1 0 then F is P-Donsker, i.e., 1 Gn G; n ! 1; in ` (F ) 2 2 I L2(P)-brackets [li; ui] satisfy P[(ui − li) ] 6 " and thus P(ui − li) 6 " =) L2(P) brackets are smaller than L1(P) brackets =) higher bracketing number N[]("; F ; L2(P)) I Finite bracketing integral =) N[]("; F ; L2(P)) cannot go to 1 too quickly as " # 0 16 / 54 Proof. Show asymptotic tightness of Gn via finite-partition criterion (part 2, p. 20). SN Construct partition F = i=1 Fi from δ-brackets [li; ui]. Use maximal inequality: there exists finite a(δ) > 0 such that 2 3 ∗ 6 G G 7 E 46 max sup j nf − ngj57 i=1;:::;N f;g2F i p −1 h 2 n oi . J[](δ; F ; L2(P)) + a(δ) P F 1 F > a(δ) n with F 2 L2(P) an envelope of F , constructed via 1-brackets. Let first n ! 1, then δ ! 0, and apply Markov’s inequality. Proof of the maximal inequality is difficult. Techniques: Bernstein’s inequality, Orlicz norms, chaining. See Lemmas 19.32–34 in van der Vaart (1998). 17 / 54 Example: weighted distribution function Suppose X = [0; 1] and P the uniform distribution. Weighted empirical distribution function: for w :[0; 1] ! [0; 1], let ft (x) = w(t)1[0;t](x); x; t 2 [0; 1] p Gnft = n Fn(t) − t w(t) Identify F = fft j t 2 [0; 1]g with [0; 1]. For a Brownian bridge B, do we have weak convergence in `1([0; 1]) p ? n Fn(t) − t w(t) (B(t)w(t)) ; n ! 1 t2[0;1] t2[0;1] If yes, then construct tail-sensitive Kolmogorov–Smirnov test statistics p sup n Fn(t) − t w(t) t2[0;1] via w such that w(t) ! 1 as t ! 0 or t ! 1 18 / 54 a T o a 8 ils Il c on T m D 1h Ë z a o o d a T 19 / 54 Assume w :[0; 1] ! [0; 1] is decreasing, w(0) = 1, w(1) = 0, and R 1 w2(t) dt < 1. 0 Fix " > 0. Find a sufficiently fine grid 0 = t0 < t1 < : : : < tm = 1 such that the brackets [li; ui] defined by 1 li(x) = w(ti) [0;ti−1](x) 1 1 ui(x) = w(ti−1) [0;ti−1](x) + w(x) (ti−1;ti ](x) R have L (P)-size [ (u − l )2]1=2 ". The number m of points t needed is 2 [0;1] i i 6 i 2 N[]("; F ; L2(P)) = O(1=" ) R δ p As log(1=") d" < 1, the bracketing integral J (δ; F ; L (P)) is finite. 0 [] 2 20 / 54 s a a f n z o 8 8 m m s 0 O a ï o x Il M C 21 / 54 Example: Parametric class d On general X, consider F = ffθ j θ 2 Θg for bounded Θ ⊂ R . Suppose there exists m 2 L2(P) such that 8x 2 X; 8θ1; θ2 2 Θ; fθ1 (x) − fθ2 (x) 6 m(x) kθ1 − θ2k Claim: There exists K > 0 depending only on Θ, d, and m such that diam Θ!d 80 < " < diam Θ; N ("; F ; L (P)) 6 K [] 2 " N Proof: Find grid fθigi=1 ⊂ Θ such that "-balls with centers θi cover Θ. For " > 0, cover F by brackets [li; ui] = [fθi − "m; fθi + "m]. Grid size N can be bounded by O (diam Θ=")d as " # 0. 22 / 54 a s AIM os a os 23 / 54 Mean absolute deviation 2 Let X1; X2;::: be iid P over X = R; assume E[X ] < 1. Mean absolute deviation: 1 Xn M = X − X n n i n i=1 p Asymptotic distribution of n(Mn − E[jX − µj])? Define 8θ; x 2 R; fθ(x) = jx − θj Writing µ = E[X], we have p p n (Mn − E[jX − µj]) = n Pnf − Pfµ X n p = Gnf + n Pf − Pfµ X n X n How to handle the two terms? 24 / 54 I q r Ils v X A f V o g N 25 / 54 1.
Details
-
File Typepdf
-
Upload Time-
-
Content LanguagesEnglish
-
Upload UserAnonymous/Not logged-in
-
File Pages54 Page
-
File Size-