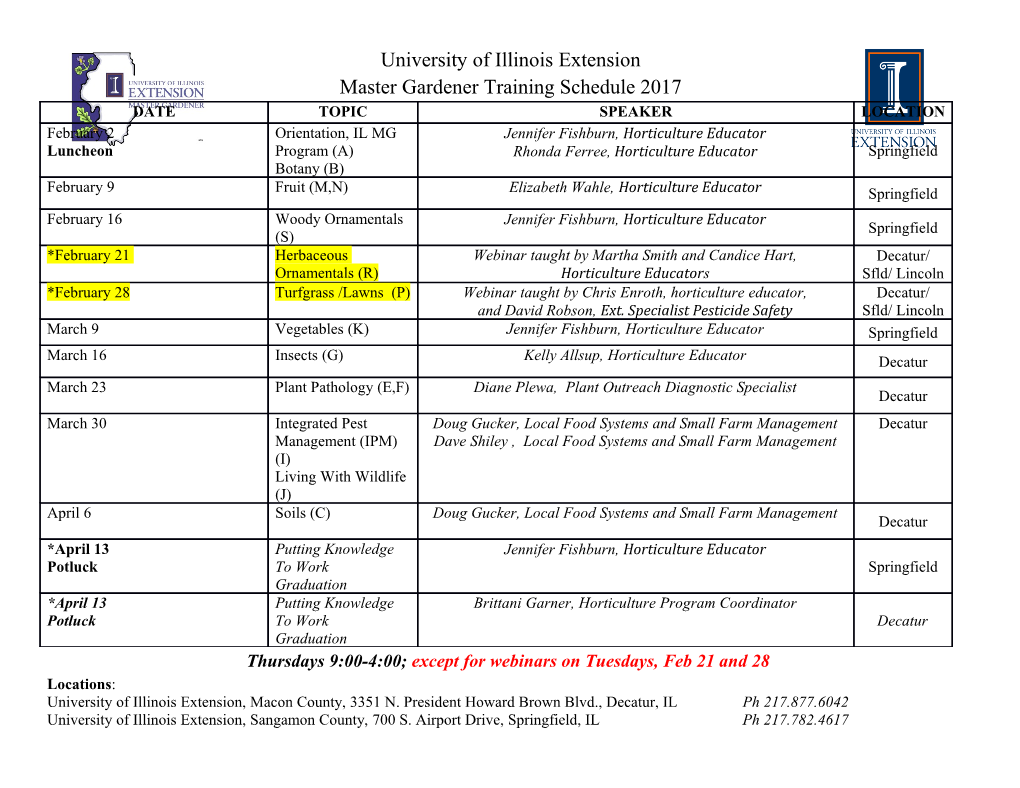
STATISTICAL SCIENCE Volume 31, Number 4 November 2016 ApproximateModelsandRobustDecisions..................James Watson and Chris Holmes 465 ModelUncertaintyFirst,NotAfterwards....................Ingrid Glad and Nils Lid Hjort 490 ContextualityofMisspecificationandData-DependentLosses..............Peter Grünwald 495 SelectionofKLNeighbourhoodinRobustBayesianInference..........Natalia A. Bochkina 499 IssuesinRobustnessAnalysis.............................................Michael Goldstein 503 Nonparametric Bayesian Clay for Robust Decision Bricks ..................................................Christian P. Robert and Judith Rousseau 506 Ambiguity Aversion and Model Misspecification: An Economic Perspective ................................................Lars Peter Hansen and Massimo Marinacci 511 Rejoinder: Approximate Models and Robust Decisions. .James Watson and Chris Holmes 516 Filtering and Tracking Survival Propensity (Reconsidering the Foundations of Reliability) .......................................................................Nozer D. Singpurwalla 521 On Software and System Reliability Growth and Testing. ..............FrankP.A.Coolen 541 HowAboutWearingTwoHats,FirstPopper’sandthendeFinetti’s?..............Elja Arjas 545 WhatDoes“Propensity”Add?...................................................Jane Hutton 549 Reconciling the Subjective and Objective Aspects of Probability ...............Glenn Shafer 552 Rejoinder:ConcertUnlikely,“Jugalbandi”Perhaps..................Nozer D. Singpurwalla 555 ChaosCommunication:ACaseofStatisticalEngineering...............Anthony J. Lawrance 558 Bayes, Reproducibility and the Quest for Truth ..............................D. A. S. Fraser, M. Bédard, A. Wong, Wei Lin and A. M. Fraser 578 A Review and Comparison of Age–Period–Cohort Models for Cancer Incidence .........................................................Theresa R. Smith and Jon Wakefield 591 Multiple Change-Point Detection: A Selective Overview .....................................................Yue S. Niu, Ning Hao and Heping Zhang 611 AConversationwithJeffWu.........................Hugh A. Chipman and V. Roshan Joseph 624 AConversationwithSamadHedayat..............Ryan Martin, John Stufken and Min Yang 637 Statistical Science [ISSN 0883-4237 (print); ISSN 2168-8745 (online)], Volume 31, Number 4, November 2016. Published quarterly by the Institute of Mathematical Statistics, 3163 Somerset Drive, Cleveland, OH 44122, USA. Periodicals postage paid at Cleveland, Ohio and at additional mailing offices. POSTMASTER: Send address changes to Statistical Science, Institute of Mathematical Statistics, Dues and Subscriptions Office, 9650 Rockville Pike—Suite L2310, Bethesda, MD 20814-3998, USA. Copyright © 2016 by the Institute of Mathematical Statistics Printed in the United States of America EDITOR Peter Green University of Bristol and University of Technology, Sydney ASSOCIATE EDITORS Steve Buckland Byron Morgan Eric Tchetgen Tchetgen University of St. Andrews University of Kent Harvard School of Public Jiahua Chen Peter Müller Health University of British Columbia University of Texas Yee Whye Teh Rong Chen Sonia Petrone University of Oxford Rutgers University Bocconi University Jon Wakefield Rainer Dahlhaus Nancy Reid University of Washington University of Heidelberg University of Toronto Guenther Walther Peter J. Diggle Glenn Shafer Stanford University Lancaster University Rutgers Business Jon Wellner Robin Evans School–Newark and University of Washington University of Oxford New Brunswick Martin Wells Michael Friendly Royal Holloway College, Cornell University York University University of London Tong Zhang Edward I. George Michael Stein Rutgers University University of Pennsylvania University of Chicago MANAGING EDITOR T. N. Sriram University of Georgia PRODUCTION EDITOR Patrick Kelly EDITORIAL COORDINATOR Kristina Mattson PAST EXECUTIVE EDITORS Morris H. DeGroot, 1986–1988 Morris Eaton, 2001 Carl N. Morris, 1989–1991 George Casella, 2002–2004 Robert E. Kass, 1992–1994 Edward I. George, 2005–2007 Paul Switzer, 1995–1997 David Madigan, 2008–2010 Leon J. Gleser, 1998–2000 Jon A. Wellner, 2011–2013 Richard Tweedie, 2001 Statistical Science 2016, Vol. 31, No. 4, 465–489 DOI: 10.1214/16-STS592 © Institute of Mathematical Statistics, 2016 Approximate Models and Robust Decisions James Watson and Chris Holmes Abstract. Decisions based partly or solely on predictions from probabilis- tic models may be sensitive to model misspecification. Statisticians are taught from an early stage that “all models are wrong, but some are useful”; how- ever, little formal guidance exists on how to assess the impact of model ap- proximation on decision making, or how to proceed when optimal actions appear sensitive to model fidelity. This article presents an overview of re- cent developments across different disciplines to address this. We review diagnostic techniques, including graphical approaches and summary statis- tics, to help highlight decisions made through minimised expected loss that are sensitive to model misspecification. We then consider formal methods for decision making under model misspecification by quantifying stability of optimal actions to perturbations to the model within a neighbourhood of model space. This neighbourhood is defined in either one of two ways. First, in a strong sense via an information (Kullback–Leibler) divergence around the approximating model. Second, using a Bayesian nonparametric model (prior) centred on the approximating model, in order to “average out” over possible misspecifications. This is presented in the context of recent work in the robust control, macroeconomics and financial mathematics literature. We adopt a Bayesian approach throughout although the presentation is agnostic to this position. Key words and phrases: Computational decision theory, model misspecifi- cation, D-open problem, Kullback–Leibler divergence, robustness, Bayesian nonparametrics. REFERENCES BEAUMONT,M.A.,ZHANG,W.andBALDING, D. J. (2002). Ap- proximate Bayesian computation in population genetics. Genet- AHMADI-JAV I D , A. (2011). An information-theoretic approach ics 162 2025–2035. to constructing coherent risk measures. In IEEE International BELSLEY,D.A.,KUH,E.andWELSCH, R. E. (1980). Regres- Symposium on Information Theory Proceedings (ISIT) 2125– sion Diagnostics: Identifying Influential Data and Sources of 2127. IEEE, New York. Collinearity. Wiley, New York. MR0576408 AHMADI-JAV I D , A. (2012). Entropic value-at-risk: A new co- herent risk measure. J. Optim. Theory Appl. 155 1105–1123. BERGER, J. O. (1984). The robust Bayesian viewpoint. In Ro- MR3000633 bustness of Bayesian Analyses (J. Kadane, ed.) 63–144. North- Holland, Amsterdam. MR0785367 ARTZNER,P.,DELBAEN,F.,EBER,J.-M.andHEATH,D. (1999). Coherent measures of risk. Math. Finance 9 203–228. BERGER, J. O. (1985). Statistical Decision Theory and Bayesian MR1850791 Analysis, 2nd ed. Springer, New York. MR0804611 AUTIER, P. (2015). Breast cancer: Doubtful health benefit of BERGER, J. O. (1994). An overview of robust Bayesian analysis. screening from 40 years of age. Nat. Rev. Clin. Oncol. 12 570– TEST 3 5–124. MR1293110 572. BERGER,J.andBERLINER, L. M. (1986). Robust Bayes and em- BAIO,G.andDAWID, A. P. (2015). Probabilistic sensitivity anal- pirical Bayes analysis with ε-contaminated priors. Ann. Statist. ysis in health economics. Stat. Methods Med. Res. 24 615–634. 14 461–486. MR0840509 James Watson is a Postdoctoral Researcher at the Mahidol–Oxford Research Unit, Centre for Tropical Medicine and Global Health, Nuffield Department of Clinical Medicine, University of Oxford, Oxford, United Kingdom (e-mail: [email protected]). Chris Holmes is Professor of Biostatistics, Department of Statistics, University of Oxford, United Kingdom (e-mail: [email protected]). BERNARDO,J.-M.andSMITH, A. F. M. (1994). Bayesian The- GRÜNWALD,P.andVA N OMMEN, T. (2014). Inconsistency of ory. Wiley, Chichester. MR1274699 Bayesian inference for misspecified linear models, and a pro- BISSIRI,P.G.,HOLMES,C.C.andWALKER, S. G. (2013). posal for repairing it. Preprint. Available at arXiv:1412.3730. A general framework for updating belief distributions. J. R. HAND, D. J. (2006). Classifier technology and the illusion of Stat. Soc. Ser. B. Stat. Methodol. Preprint. Available at progress. Statist. Sci. 21 1–34. MR2275965 arXiv:1306.6430. HANSEN,L.P.andSARGENT, T. J. (2001a). Acknowledging mis- BISSIRI,P.G.andWALKER, S. G. (2012). Converting informa- specification in macroeconomic theory. Rev. Econ. Dyn. 4 519– tion into probability measures with the Kullback-Leibler diver- 535. gence. Ann. Inst. Statist. Math. 64 1139–1160. MR2981617 HANSEN,L.P.andSARGENT, T. J. (2001b). Robust control and model uncertainty. Am. Econ. Rev. 91 60–66. BOX,G.E.P.andDRAPER, N. R. (1987). Empirical HANSEN,L.P.andSARGENT, T. J. (2008). Robustness. Princeton Model-Building and Response Surfaces. Wiley, New York. Univ. Press, Princeton, NJ. MR0861118 HANSEN,L.P.,SARGENT,T.J.,TURMUHAMBETOVA,G.and BREUER,T.andCSISZÁR, I. (2013). Systematic stress tests with WILLIAMS, N. (2006). Robust control and model misspecifica- entropic plausibility constraints. J. Bank. Financ. 37 1552– tion. J. Econom. Theory 128 45–90. MR2229772 1559. HASTIE,T.andTIBSHIRANI, R. (1993). Varying-coefficient mod- BREUER,T.andCSISZÁR, I. (2016). Measuring distribution els. J. R. Stat. Soc. Ser. B. Stat. Methodol. 55 757–796. model risk. Math. Finance 26 395–411. MR3481309 MR1229881 CAROTA,C.,PARMIGIANI,G.andPOLSON, N. G. (1996). Diag- HJORT,N.L.,HOLMES,C.C.,MÜLLER,P.andWALKER,S.G.
Details
-
File Typepdf
-
Upload Time-
-
Content LanguagesEnglish
-
Upload UserAnonymous/Not logged-in
-
File Pages34 Page
-
File Size-